Development and external validation of a prognostic nomogram for patients with gastric cancer after radical gastrectomy
Introduction
Gastric cancer (GC) is a significant health problem and one of the major causes of death worldwide. According to a report by Global Cancer Statistics, over 1 million new cases of GC were diagnosed throughout the world and about 783,000 people died of this disease in 2018 (1). In China, the incidence rate of GC was 679 per million in 2015, and Chinese cases accounted for more than 60% of all global GC cases, making it the second most common malignant tumor and the third leading cause of tumor death worldwide (2). Although recent decades have witnessed the development of a diversity of innovations and novel intervention strategies, the outlook for GC in China is not good, with GC incurring a heavy burden on the Chinese health care system.
GC can be divided into many pathological types, including adenocarcinoma, squamous cell carcinoma, carcinoid, and others, but the vast majority of cases are gastric adenocarcinoma (3,4). GC is usually diagnosed histologically following endoscopic biopsy and is staging by laparoscopy and imaging examinations, such as computed tomography (CT), endoscopic ultrasound, magnetic resonance imaging (MRI), and positron emission tomography (PET) (5). Over the years, numerous clinical studies have been carried to further optimize the treatment mode of GC, including preoperative neoadjuvant therapy (NAT), surgical modality selection, postoperative chemoradiotherapy, biologically targeted therapy, and immunotherapy. The most effective curative therapeutic option for early GC (EGC) is endoscopic resection (6), and patients with non-EGC are mainly treated by surgical resection with lymphadenectomy to achieve local radical resection (7). Adjuvant or perioperative chemotherapy can improve survival in those who are at stage 1B GC or higher (8-10), and the 5-year overall survival (OS) rate of patients with GC can reach more than 90% after early diagnosis with subsequent standardized treatment. However, due to the lack of the specific early symptoms, numerous GC patients are diagnosed late and thus have a poor 5-year OS rate of less than 29% (4,11). Consequently, the treatment of GC needs to be based on a reliable risk stratification system of prognosis that can help predict the survival of the patients and allow for radical interventions to be avoided or implemented at an appropriate time.
Thus far, the identification of prognosis for patients with GC has mainly depended on TNM staging, but owing to its inaccuracy and incompleteness, its prognostic value remains controversial. Therefore, the requirement for effective prognostic criteria has prompted researchers to explore new prognostic models for GC from multiple perspectives to help in the diagnosis of high-risk patients with poor prognoses (12-22). Identifying this proportion of patients would allow clinicians to adjust treatment strategies at an appropriate time, achieve effective intervention to control the progression of tumor, and extend patients’ survival as far as possible. However, most of the previous prognostic nomograms ignored the inclusion of clinical symptoms and procedure-related parameters, and thus the correlations between these accessible factors in the clinic and the prognosis of GC remain to be examined. Moreover, the previous prognostic models were tailored for specific populations and lacked of the risk stratification prediction for prognosis, thus restricting their more widespread application in clinical practice.
We therefore conducted this retrospective follow-up study to develop and validate a new prognostic nomogram of GC, incorporating general demographic characteristics, clinical symptoms, serological markers, surgery-related indicators, and other clinicopathological parameters. We hope to explore the clinically accessible GC-related prognostic factors and provide corresponding clinical evidence for improving postoperative survival in patients with GC. We present the following article in accordance with the TRIPOD reporting checklist (available at https://dx.doi.org/10.21037/atm-21-6359).
Methods
Patients
This study retrospectively included patients with pathologically confirmed GC who underwent radical gastrectomy (curative R0 resection). The training cohort consisted of GC patients from Tangdu Hospital (the Second Affiliated Hospital of Air Force Medical University) who were diagnosed from January 1, 2010, to January 1, 2014. The validation cohort included GC patients from Xijing Hospital (the First Affiliated Hospital of Air Force Medical University) between January 1, 2015, to January 1, 2016. The basic demographic characteristics, clinical symptoms, serological markers, treatment-related indicators, pathological parameters, and other clinical data were obtained from clinical medical records of the database in the hospital. The exclusion criteria were the following: (I) patients with remnant GC; (II) patients who refused follow-up or whose death or survival data was missing; and (III) patients from whom the clinical symptoms, complete clinicopathological data, or surgery-related information could not be obtained. Multiple imputations were performed by Stata 15.0 (StataCorp., College Station, TX, USA). All procedures performed in this study involving human participants were in accordance with the Declaration of Helsinki (as revised in 2013). The study was approved by Ethics Committee of Tangdu Hospital (No. K202011-18). Individual consent for this retrospective analysis was waived.
According to the Japanese guidelines for GC treatment, all patients in our study received standard follow-up after radical gastrectomy: every 3 to 6 months for the first 2 years postoperatively; every 6 to 12 months from the third to fifth years, and annually thereafter (23). The evaluation of follow-up comprised the patient’s history (especially the detailed symptoms), physical examination, and laboratory biochemical test results including the level of carcinoembryonic antigen (CEA), carbohydrate antigen 125 (CA 125) and carbohydrate antigen 19-9 (CA 19-9), tumor metastasis evaluated by chest radiography, CT, bone scintigraphy, and endoscopy. A retrospective review of medical records collected the patient’s demographic characteristics, including age and gender, treatment process (including various surgery-related information), and pathological features of the tumor. The final follow-up of the disease condition was based on medical records or telephone interviews. In this paper, the survival referred to the OS of patients from the time of diagnosis of GC to death.
Nomogram development
Various indicators, including the demographic characteristics, clinical symptom-related parameters, laboratory analysis results, and treatment process (including surgery-related information) were considered as candidate variables of the prognostic model. Except for CEA, CA19-9, and CA125, which were continuous variables, all the other variables were considered to be categorical variables (Table S1). The least absolute shrinkage and selection operator (LASSO) algorithm method was applied to select the most significant predictive features from these variables in the training cohort (24,25). In the LASSO algorithm, the negative log-likelihood was applied to represent the residual sum of the square for the Cox proportional hazards regression model (Cox regression). If lambda (λ) becomes smaller, it does not affect the estimated regression parameters, but as λ becomes larger, some coefficients might shrink to zero (24,25). Therefore, we chose λ with the minor cross-validation error to develop the model. We then used all available variables and selected λ to refit the model. As a result, most of the covariate coefficients shrank to zero; and the nonzero coefficients remaining, which were considered as the favorable parameters of the model, were screened out by LASSO. Next, we applied the multivariate Cox regression method to establish the prognostic model with these indicators. R version 4.0.3 software (The R Foundation for Statistical Computing, Vienna, Austria) was used for model visualization.
Internal validation
The predictive performance was quantified from the 3 perspectives of accuracy (calibration), discrimination, and clinical utility. Accuracy was evaluated by plotting the calibration curve of 1,000 bootstrap samples and calculating the concordance index (C-index). We plotted the calibration curves for 1-, 2-, and 3-year survival to compare the concordance between the predicted survival probability calculated by the nomogram and the patients’ actual survival probability. Time-dependent receiver operating characteristic curve (ROC) analysis was applied to assess the discrimination of the model. Finally, the decision curve analysis (DCA) method was used to estimate the potential clinical value of the nomogram with quantitative analysis of the net benefits being applied at different threshold probabilities. This method is used to evaluate the benefits of a model and incorporate the series of patients’ preferences for the risks of undertreatment and overtreatment to promote more appropriate model selection and inform decision usage (26,27).
External validation
The external validation of the model was conducted in patients from a different center (the validation cohort) in terms of calibration, discrimination, and clinical usefulness, with the same methods as those applied in the internal validation.
Prognostic risk stratification
We used X-tile software (version 3.6.1, http://www.tissuearray.org) to determine the prognostic risk cutoff points for low-, moderate-, and high-risk cancer-related death (28). X-tile software provides a convenient and comprehensive evaluation method that can stratify the population according to prognosis risk stratifications.
Statistical analysis
Descriptive statistics were applied to describe the basic demographic and clinicopathological characteristics of the study population. If the continuous variable conformed to the normal distribution, it is described by the mean ± standard deviation (SD); otherwise, it is expressed by the median and interquartile range (IQR). The categorical variables are presented as counts and percentages. Our research data were processed in Stata version 15.0 (RRID: SCR_012763; StataCorp) and R version 4.0.3 (RRID: SCR_001905; http://www.r-project.org). A 2-sided P value <0.05 was considered to be statistically significant.
Results
Demographic and clinicopathologic characteristics
According to the inclusion and exclusion criteria, 1,424 patients with GC were eligible in this study, including 1,010 in the training cohort and 414 in the validation cohort. The sample size of this study met the criterion of 10 outcome events for each predictor variable (29,30). A flowchart of the detailed research process is shown in Figure 1. The patients were middle-aged and older adult individuals, and ranged from 51 to 66 years of age in the training set and 50 to 65 years in the validation set. The ratio of males to females in the patients was approximately 4:1. In both the training and validation cohorts, many patients had a history of smoking and drinking. Most patients received gastrectomy were negative for human epidermal growth factor receptor 2 (HER2), and were at the T3 stage. Only a few patients (2.0% and 2.7% in the training and the validation set, respectively) received NAT. The detailed demographic and clinicopathologic characteristics are summarized in Table S1.
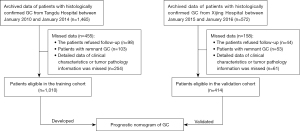
Nomogram development
We applied the LASSO method to select the reliable variables of the model. As a result, most of the covariate coefficients shrank to zero; and only 7 remaining nonzero parameters were selected (Figure 2) as independent prognostic factors of the model. These included the number of positive lymph nodes [P=0.001; hazard ratio (HR) 1.03; 95% CI: 1.01–1.04, tumor size (P=0.004; HR 1.07; 95% CI: 1.02–1.11)], adjacent organs invaded (P=0.011; HR 1.34; 95% CI: 1.07–1.74), vascular invasion (P=0.007; HR 1.40; 95% CI: 1.10–1.78), CA 125 (P<0.001; HR 1.00; 95% CI: 1.00–1.01), depth of invasion (T stage) (P<0.001; HR 1.84; 95% CI: 1.54–2.19), and HER2 status (P<0.001; HR 1.28; 95% CI: 1.11–1.47), as depicted in Table 1. Based on these indicators, we developed a prognostic predictive nomogram of GC (Figure 3). In the nomogram plot, each variable was assigned a corresponding point according to its HR; then, by adding up the total points for each variable and positioning the points on the total point scale, we could obtain the probabilities of 1-, 2-, and 3-year OS.
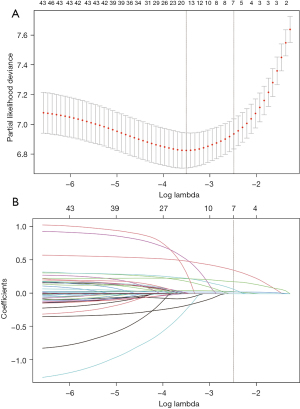
Table 1
Prognostic predictors | P value | HR | 95% CI |
---|---|---|---|
Positive LN (for each additional LN involved) | 0.001 | 1.03 | 1.01–1.04 |
Tumor size (per increase in 0.1 cm) | 0.004 | 1.07 | 1.02–1.11 |
Adjacent organs invasion (yes vs. no) | 0.011 | 1.34 | 1.07–1.74 |
Vascular invasion (yes vs. no) | 0.007 | 1.40 | 1.10–1.78 |
CA 125 (per increase in 0.1 IU/mL) | <0.001 | 1.01 | 1.00–1.01 |
Depth of invasion (T stage*) | <0.001 | 1.84 | 1.54–2.19 |
HER2 status# | <0.001 | 1.28 | 1.11–1.47 |
*, T stage was confirmed by postoperative pathological diagnosis; #, HER2 status was examined by immunohistochemistry. LN, lymph node; CA 125, carbohydrate antigen 125. HR, hazard ratio; HER2, human epidermal growth factor receptor 2.
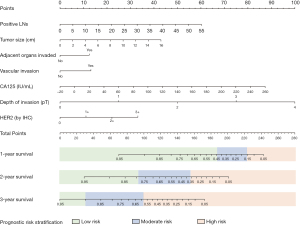
Nomogram validation
The calibration of the nomogram
The internal calibrations of the nomogram demonstrated good agreement with the actual survival in the predictions of 1-, 2- and 3-year survival, with a C-index of 0.76 (95% CI: 0.74–0.79), 0.79 (95% CI: 0.77–0.81), and 0.82 (95% CI: 0.76–0.88), respectively (Figure 4A-4C). Similarly, the calibration plot in the external cohort graphically also showed good agreement between the prediction by the nomogram and the actual survival in 1-, 2-, and 3-year survival with a C-index of 0.76 (95% CI: 0.68–0.82), 0.78 (95% CI: 0.73–0.83), and 0.75 (95% CI: 0.65–0.85), respectively (Figure 4A-4C).
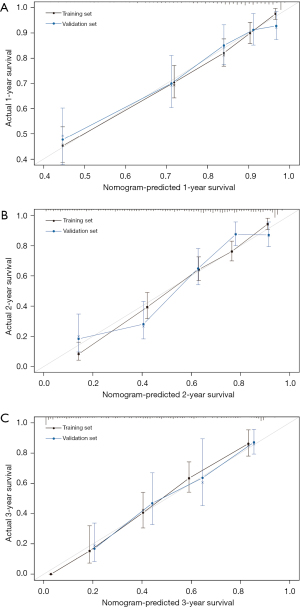
The accuracy of the nomogram
The time-dependent ROC (Figure 5A) in the internal validation showed favorable discriminations; and the area under the curve (AUC) of these were 0.75 (95% CI: 0.72–0.79), 0.80 (95% CI: 0.77–0.83), and 0.82 (95% CI: 0.74–0.89), respectively. In addition, that in 1-, 2-, and 3-year survival were 0.75 (95% CI: 0.69–0.80), 0.79 (95% CI: 0.74–0.84), and 0.76 (95% CI: 0.67–0.85), respectively (Figure 5B), which revealed a good discrimination of the nomogram.
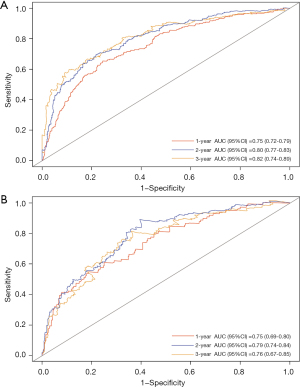
The clinical utility of the nomogram
The DCA was also applied to validate the clinical utility of the model by evaluating the net benefit. DCA plotting had demonstrated that the nomogram used in the current study was effective in the training cohort when the threshold probability was less than 72.0% (Figure 6A), greater than 9.9% (Figure 6B), and greater than 18.5% (Figure 6C) in the 1-, 2- and 3-year survival prediction, respectively. Additionally, the DCA curve showed that the nomogram was also useful in clinic when the threshold probability was 4.8–62.2% (Figure 6D), greater than 4.9% (Figure 6E), and greater than 8.8% (Figure 6F) in the 1-, 2- and 3-year survival predictions, respectively.
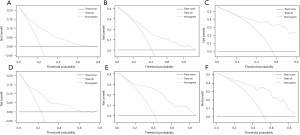
Prognostic risk stratification
We use the 2 best cutoff points of the total score of 1-, 2-, and 3-year survival in the training set was generated by the X-tile software. According to these 2 cutoff points, the study population was divided into 3 groups with entirely different risks of survival probability (Figure 3): the low-risk group (1-year survival: total points ≤187, 496 cases in the training set and 380 cases in the verification set; 2-year survival: total points ≤93, 502 cases in the training set and 101 cases in the verification set; 3-year survival: total points ≤30, 491 cases in the training set and 276 cases in the verification set), the moderate-risk group (1-year survival: 187< total points ≤223, 370 patients in the training set and 24 cases in the verification set; 2-year survival: 93< total points ≤153, 365 cases in the training set and 215 cases in the verification set; 3-year survival: 30< total points ≤98, 306 cases in training set and 129 cases in verification set), and the high-risk group (1-year survival: total points >223, 144 patients in the training set and 4 cases in the verification set; 2-year survival: total points >153, 143 patients in the training set and 92 cases in the verification set; 3-year survival: total points >98, 213 patients in the training set and 3 cases in the verification set). Figure 7 shows the survival curves before and after risk stratifications in the population of the training and validation set, respectively; the HR is 2.88 for high- vs. low-risk and 4.42 for moderate- vs. low-risk with both P values <0.0001. The statistical differences in the prognosis among the 3 risk stratification groups further showed that our model had an excellent risk stratification performance.
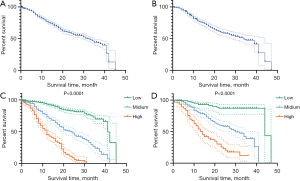
Discussion
In recent years, researchers have spared no effort in developing the individualized treatment of GC, and new targeted therapies have been administered to patients (31,32); however, the overall survival of GC patients is still not satisfactory. As clinical symptoms appear, the lesions often progress into an advanced stage; thus, a considerable portion of GC patients lose the opportunity for surgery and have an unfavorable outcome (33). Accordingly, it is worth exploring prognostic factors to facilitate a more feasible and reliable prognostic tool for risk stratification and further clinical decision-making.
In this study, we incorporated various factors, including clinical characteristics, pathological parameters, and surgery- and treatment-related indicators, and applied the LASSO method to select variables among them. We finally established a reliable prognosis nomogram to predict 1-, 2- and 3-year survival, which contained 7 indicators: lymph node involvement, tumor size, adjacent organs invaded, vascular invasion, CA 125, depth of tumor invasion (T stage), and HER2 status. Considering that some factors (such as the differences of the medical equipment, surgical skills and postoperative patient care strategies) might cause differences in the prognosis of patients after radical GC surgery in different centers, therefore, we decided to conduct both internal and external validations to evaluate the performance of our model more comprehensively. After internal and external validations, the model demonstrated a robust predictive capacity with satisfying calibration, discrimination, and clinical utility. Furthermore, we divided the population into 3 risk groups of survival used the cutoff points generated by X-tile; in this way, the nomogram was further improved into a risk-stratified prognosis model. We hope the prognostic risk stratification nomogram can provide guidance for clinical practice and help facilitate tailored decision-making, thus avoiding overtreatment or undertreatment in GC patients and improving communication between clinicians and patients.
At present, the most commonly accepted prognostic risk prediction system in clinical practice is TNM staging, but the accuracy and stability it offers are limited, and thus its value in precision medicine has increasingly declined (34-37). Several studies of prognostic nomograms have emphasized their potential advantages to patients and a reliable risk-based predictive tool that can be used widely in cancer-related research (38). Researchers have investigated a number of prognostic factors for GC, such as age, sex, tumor size, node status, depth of invasion, tumor site, Lauren type, histologic type, distant metastasis, and biological markers, with various prognostic models being established accordingly (12-21,37,39-42); however, none of these involved the value of clinical symptoms, HER2 status, and adjacent organ invasion. Additionally, only a few of these nomogram studies conducted validation in an external center, and some only focused on specific subgroups of patients (18); consequently, it remains elusive for the application of nomograms for a large population of GC patients. To address this, we established a model in this study that was validated externally, and it demonstrated its applicability for a wider range of populations with GC. Moreover, as has been done in only a few other studies, we also investigated the relevant clinical symptoms and tumor molecular markers.
Clinical symptoms are often the earliest and most significant reasons for patients to seek medical treatment. Therefore, if certain symptoms are demonstrated to be associated with GC prognosis, this may prove to be considerably valuable in guiding clinicians to provide timely clinical interventions for patients and may allow for appropriate treatment intensification and the prolonging of survival as much as possible. Based on this concept, we incorporated multiple possible symptoms of stomach diseases into this study, including pain, flatulence, deglutition disorders, vomiting, acid regurgitation, and weight loss (Table S1). In order to better quantify the degree of the performance of these symptoms, we designed a symptom score table. Regarding the index of pain, we converted values from light to heavy into a scale from 0 to 10, with 0–5 being mild pain and 6–10 being severe pain. For weight loss, we measure the exact value. The other factors were measured according their presence or absence. However, unfortunately, we did not find these symptoms to be indicative of survival outcomes in GC. We surmised that this may be related to the subjectivity of the actual symptoms of the individuals and the inaccuracy of the retrospective data to a certain extent. Although the result was negative, as far as we know, this is the first report to explore the prognostic value of clinical symptoms in GC and may potentially provide guidance for other related future research.
HER2 is a member of the epidermal growth factor receptor family. It is a type I receptor tyrosine kinase, located on human chromosome 17q21, encodes transmembrane protein p185, and exerts tyrosine protein kinase activity (43). Its abnormal amplification and overexpression can lead to malignant transformation in cells (44). It has also been reported that the detection rates of HER2-positive cases varies greatly across different countries, from 2% to 45% (45), which may be influenced not only by the heterogeneity of GC, but also by the differences in other factors, such as histological type, primary site, scoring system, specimen samplings fixation solutions, and HER2 antibodies. Retrospective studies have suggested that HER2-positive expression is related to older age, male gender, intestinal subtype in Lauren classification, and tumors located in the upper third of the stomach (46-48), but the relationship between the expression of HER2 and the prognosis of GC patients remains controversial. A meta-analysis that evaluated 41 studies with a total of 17,494 patients demonstrated HER2 overexpression to be associated with poor prognosis in GC (49). However, in a study by Uprak et al. (50), no significant relationship of HER2 status with clinicopathological parameters and the prognosis of GC patients was found. Hence, more research into this issue is still required, and perhaps, the combination of HER2 levels and other clinical indicators could potentially have predictive value for the prognosis of GC. For instance, Qiu et al. (47) showed that patients with HER2-negative intestinal GC had a better prognosis than did those with HER2-positive GC, suggesting that HER2 combined with Lauren type could better predict the prognosis in patients with GC. In the current study, we retrospectively investigated the various clinical characteristics of more than 1,400 patients and applied reasonable statistical methods, which indicated HER2 to be an independent factor associated with the prognosis of G; specifically, we found that patients with HER2-positive expression had a 1.28-fold higher risk of death compared to patients with HER2- negative expression (P<0.001). This was combined with other factors to establish the model examined in this study, revealing that targeted therapy could improve outcomes in HER2-positive patients.
A large number of studies have focused on the importance of lymph node metastasis on the prognosis of GC, but few have analyzed the prognostic value of adjacent organ involvement in GC (51,52). It is well-known that the local infiltration and lymphatic metastasis are the main diffusion routes of GC. In this study, we investigated the prognostic value of the involvement of adjacent organs in GC patients. We found that 21.6% and 17.9% patients in the training and the validation cohort, respectively, had surrounding organ invasion, including of the liver, pancreas, spleen, diaphragm, transverse colon, and mesocolon. Compared with patients without invasion of adjacent organs, these patients had a 1.34-time lower risk of survival. This study is the first to quantify the significance of adjacent organ invasion on the prognosis of GC, laying the foundation for future clinical research in this area.
CA 125 is one of the more well-known serum tumor markers and is commonly used in cancer diagnosis, treatment monitoring, and prognosis evaluation (53). Previous studies have suggested serum CA 125 to be more reliable in the diagnosis of peritoneal metastases in GC than other are imaging examines, such as ultrasound, computed tomography, and other tumor serum markers. Additionally, CA 125 level has been shown to be significantly related to the degree of peritoneal spread and survival of patients (39,54-56). However, most research into CA 125 has treated it as a categorical variable (negative or positive), and thus an examination of its more subtle impacts on GC prognosis is lacking. In this study, we evaluated the effect of each 0.1 IU/mL increase of CA 125 on the prognosis of patients with GC, which revealed it to be independent risk factor (HR =1.01; 95% CI: 1.00–1.01; P<0.001).
Despite the promising findings, the current study has several limitations that should be noted. First , it was based on a retrospective analysis, and 17.34% (254/1,465) and 10.66% (61/572) of patients who lacked clinicopathological information in the training and validation sets, respectively, were not included in the study; thus, selection bias could not be avoided. Second, despite the importance of the clinical symptoms, patient’s understanding of symptoms might affect their contributions to the prediction model, resulting in a negative conclusion; thus, the accurate quantitative description of subjective symptoms, as well as the strengthening of training and education for patients on symptom awareness, needs to be improved in further clinical research. Third, we excluded patients with remnant GC, so these patients may not be applicable for our model. Therefore, a well-designed multisampling, multicenter cohort study is still required.
In summary, we constructed a postoperative risk stratification nomogram of OS for GC patients, which has the potential to identify those patients at high-risk of short survival after operation. Application of this nomogram may allow for more radical interventions to be avoided and only implemented at the appropriate time. Moreover, this nomogram may also be used to screen comparable study groups for related clinical trials. We hope it can provide a reference for the prognostic prediction and clinical treatment for GC and further facilitate the individualization of the clinic process.
Acknowledgments
The authors would like to acknowledge all the participants involved in this study.
Funding: This work was supported by the National Natural Science Foundation of China (No. 81572916, No. 81502424).
Footnote
Reporting Checklist: The authors have completed the TRIPOD reporting checklist. Available at https://dx.doi.org/10.21037/atm-21-6359
Data Sharing Statement: Available at https://dx.doi.org/10.21037/atm-21-6359
Conflicts of Interest: All authors have completed the ICMJE uniform disclosure form (available at https://dx.doi.org/10.21037/atm-21-6359). The authors have no conflicts of interest to declare.
Ethical Statement: The authors are accountable for all aspects of the work in ensuring that questions related to the accuracy or integrity of any part of the work are appropriately investigated and resolved. All procedures performed in this study involving human participants were in accordance with the Declaration of Helsinki (as revised in 2013). The study was approved by Ethics Committee of Tangdu Hospital (No. K202011-18). Individual consent for this retrospective analysis was waived.
Open Access Statement: This is an Open Access article distributed in accordance with the Creative Commons Attribution-NonCommercial-NoDerivs 4.0 International License (CC BY-NC-ND 4.0), which permits the non-commercial replication and distribution of the article with the strict proviso that no changes or edits are made and the original work is properly cited (including links to both the formal publication through the relevant DOI and the license). See: https://creativecommons.org/licenses/by-nc-nd/4.0/.
References
- Bray F, Ferlay J, Soerjomataram I, et al. Global cancer statistics 2018: GLOBOCAN estimates of incidence and mortality worldwide for 36 cancers in 185 countries. CA Cancer J Clin 2018;68:394-424. [Crossref] [PubMed]
- Chen W, Zheng R, Baade PD, et al. Cancer statistics in China, 2015. CA Cancer J Clin 2016;66:115-32. [Crossref] [PubMed]
- Dicken BJ, Bigam DL, Cass C, et al. Gastric adenocarcinoma: review and considerations for future directions. Ann Surg 2005;241:27-39. [Crossref] [PubMed]
- Yang B, Lu X. The malignancy among gastric submucosal tumor. Transl Cancer Res 2019;8:2654-66. [Crossref]
- Smyth EC, Nilsson M, Grabsch HI, et al. Gastric cancer. Lancet 2020;396:635-48. [Crossref] [PubMed]
- Hatta W, Gotoda T, Koike T, et al. History and future perspectives in Japanese guidelines for endoscopic resection of early gastric cancer. Dig Endosc 2020;32:180-90. [Crossref] [PubMed]
- Cohen DJ, Leichman L. Controversies in the treatment of local and locally advanced gastric and esophageal cancers. J Clin Oncol 2015;33:1754-9. [Crossref] [PubMed]
- Glimelius B, Ekström K, Hoffman K, et al. Randomized comparison between chemotherapy plus best supportive care with best supportive care in advanced gastric cancer. Ann Oncol 1997;8:163-8. [Crossref] [PubMed]
- Fuchs CS, Shitara K, Di Bartolomeo M, et al. Ramucirumab with cisplatin and fluoropyrimidine as first-line therapy in patients with metastatic gastric or junctional adenocarcinoma (RAINFALL): a double-blind, randomised, placebo-controlled, phase 3 trial. Lancet Oncol 2019;20:420-35. [Crossref] [PubMed]
- Lordick F. Chemotherapy for resectable microsatellite instability-high gastric cancer. Lancet Oncol 2020;21:203. [Crossref] [PubMed]
- Miyahara R, Niwa Y, Matsuura T, et al. Prevalence and prognosis of gastric cancer detected by screening in a large Japanese population: data from a single institute over 30 years. J Gastroenterol Hepatol 2007;22:1435-42. [Crossref] [PubMed]
- Dikken JL, Baser RE, Gonen M, et al. Conditional probability of survival nomogram for 1-, 2-, and 3-year survivors after an R0 resection for gastric cancer. Ann Surg Oncol 2013;20:1623-30. [Crossref] [PubMed]
- Song KY, Park YG, Jeon HM, et al. A nomogram for predicting individual survival of patients with gastric cancer who underwent radical surgery with extended lymph node dissection. Gastric Cancer 2014;17:287-93. [Crossref] [PubMed]
- Muneoka Y, Akazawa K, Ishikawa T, et al. Nomogram for 5-year relapse-free survival of a patient with advanced gastric cancer after surgery. Int J Surg 2016;35:153-9. [Crossref] [PubMed]
- Jiang Y, Chen C, Xie J, et al. Radiomics signature of computed tomography imaging for prediction of survival and chemotherapeutic benefits in gastric cancer. EBioMedicine 2018;36:171-82. [Crossref] [PubMed]
- Jiang Y, Zhang Q, Hu Y, et al. ImmunoScore Signature: A Prognostic and Predictive Tool in Gastric Cancer. Ann Surg 2018;267:504-13. [Crossref] [PubMed]
- Roberto M, Botticelli A, Strigari L, et al. Prognosis of elderly gastric cancer patients after surgery: a nomogram to predict survival. Med Oncol 2018;35:111. [Crossref] [PubMed]
- Chen QY, Zhong Q, Wang W, et al. Development and external validation of a nomogram for predicting the conditional probability of survival after D2 lymphadenectomy for gastric cancer: A multicentre study. Eur J Surg Oncol 2019;45:1934-42. [Crossref] [PubMed]
- Dong D, Tang L, Li ZY, et al. Development and validation of an individualized nomogram to identify occult peritoneal metastasis in patients with advanced gastric cancer. Ann Oncol 2019;30:431-8. [Crossref] [PubMed]
- Shi X, Xu L, Ma B, et al. Development and validation of a nomogram to predict the prognosis of patients with gastric cardia cancer. Sci Rep 2020;10:14143. [Crossref] [PubMed]
- Wang CY, Yang J, Zi H, et al. Nomogram for predicting the survival of gastric adenocarcinoma patients who receive surgery and chemotherapy. BMC Cancer 2020;20:10. [Crossref] [PubMed]
- Barchi LC, Yagi OK, Jacob CE, et al. Predicting recurrence after curative resection for gastric cancer: External validation of the Italian Research Group for Gastric Cancer (GIRCG) prognostic scoring system. Eur J Surg Oncol 2016;42:123-31. [Crossref] [PubMed]
- Japanese Gastric Cancer Association. Japanese gastric cancer treatment guidelines 2014 (ver. 4). Gastric Cancer 2017;20:1-19. [Crossref] [PubMed]
- Tibshirani R. Regression Shrinkage and Selection Via the Lasso. J R Stat Soc Series B Stat Methodol 1996;58:267-88.
- Tibshirani R. The lasso method for variable selection in the Cox model. Stat Med 1997;16:385-95. [Crossref] [PubMed]
- Vickers AJ, Elkin EB. Decision curve analysis: a novel method for evaluating prediction models. Med Decis Making 2006;26:565-74. [Crossref] [PubMed]
- Vickers AJ, Cronin AM, Elkin EB, et al. Extensions to decision curve analysis, a novel method for evaluating diagnostic tests, prediction models and molecular markers. BMC Med Inform Decis Mak 2008;8:53. [Crossref] [PubMed]
- Camp RL, Dolled-Filhart M, Rimm DL. X-tile: a new bio-informatics tool for biomarker assessment and outcome-based cut-point optimization. Clin Cancer Res 2004;10:7252-9. [Crossref] [PubMed]
- Concato J, Peduzzi P, Holford TR, et al. Importance of events per independent variable in proportional hazards analysis. I. Background, goals, and general strategy. J Clin Epidemiol 1995;48:1495-501. [Crossref] [PubMed]
- Peduzzi P, Concato J, Feinstein AR, et al. Importance of events per independent variable in proportional hazards regression analysis. II. Accuracy and precision of regression estimates. J Clin Epidemiol 1995;48:1503-10. [Crossref] [PubMed]
- Ng K, Meyerhardt JA, Fuchs CS. Adjuvant and neoadjuvant approaches in gastric cancer. Cancer J 2007;13:168-74. [Crossref] [PubMed]
- Knight G, Earle CC, Cosby R, et al. Neoadjuvant or adjuvant therapy for resectable gastric cancer: a systematic review and practice guideline for North America. Gastric Cancer 2013;16:28-40. [Crossref] [PubMed]
- Wagner AD, Syn NL, Moehler M, et al. Chemotherapy for advanced gastric cancer. Cochrane Database Syst Rev 2017;8:CD004064. [PubMed]
- Taniguchi K, Ota M, Yamada T, et al. Staging of gastric cancer with the Clinical Stage Prediction score. World J Surg Oncol 2019;17:47. [Crossref] [PubMed]
- Zhou YY, Kang YT, Chen C, et al. Combination of TNM staging and pathway based risk score models in patients with gastric cancer. J Cell Biochem 2018;119:3608-17. [Crossref] [PubMed]
- Ye J, Ren Y, Wei Z, et al. External validation of a modified 8th AJCC TNM system for advanced gastric cancer: Long-term results in southern China. Surg Oncol 2018;27:146-53. [Crossref] [PubMed]
- Jiao XG, Deng JY, Zhang RP, et al. Prognostic value of number of examined lymph nodes in patients with node-negative gastric cancer. World J Gastroenterol 2014;20:3640-8. [Crossref] [PubMed]
- Iasonos A, Schrag D, Raj GV, et al. How to build and interpret a nomogram for cancer prognosis. J Clin Oncol 2008;26:1364-70. [Crossref] [PubMed]
- Kim DH, Yun HY, Ryu DH, et al. Preoperative CA 125 is significant indicator of curative resection in gastric cancer patients. World J Gastroenterol 2015;21:1216-21. [Crossref] [PubMed]
- Zhang D, He W, Wu C, et al. Scoring System for Tumor-Infiltrating Lymphocytes and Its Prognostic Value for Gastric Cancer. Front Immunol 2019;10:71. [Crossref] [PubMed]
- He Y, Mao M, Shi W, et al. Development and validation of a prognostic nomogram in gastric cancer with hepatitis B virus infection. J Transl Med 2019;17:98. [Crossref] [PubMed]
- Lin JX, Lin JP, Xie JW, et al. Prognostic Value and Association of Sarcopenia and Systemic Inflammation for Patients with Gastric Cancer Following Radical Gastrectomy. Oncologist 2019;24:e1091-1091e1101. [Crossref] [PubMed]
- Dowdy SC, Mariani A, Janknecht R. HER2/Neu- and TAK1-mediated up-regulation of the transforming growth factor beta inhibitor Smad7 via the ETS protein ER81. J Biol Chem 2003;278:44377-84. [Crossref] [PubMed]
- Tai W, Mahato R, Cheng K. The role of HER2 in cancer therapy and targeted drug delivery. J Control Release 2010;146:264-75. [Crossref] [PubMed]
- Laboissiere RS, Buzelin MA, Balabram D, et al. Association between HER2 status in gastric cancer and clinicopathological features: a retrospective study using whole-tissue sections. BMC Gastroenterol 2015;15:157. [Crossref] [PubMed]
- Pereira MA, Ramos M, Dias AR, et al. Expression Profile of Markers for Targeted Therapy in Gastric Cancer Patients: HER-2, Microsatellite Instability and PD-L1. Mol Diagn Ther 2019;23:761-71. [Crossref] [PubMed]
- Qiu M, Zhou Y, Zhang X, et al. Lauren classification combined with HER2 status is a better prognostic factor in Chinese gastric cancer patients. BMC Cancer 2014;14:823. [Crossref] [PubMed]
- Yıldız Y, Sokmensuer C, Yalcin S. Evaluation of c-Met, HGF, and HER-2 expressions in gastric carcinoma and their association with other clinicopathological factors. Onco Targets Ther 2016;9:5809-17. [Crossref] [PubMed]
- Lei YY, Huang JY, Zhao QR, et al. The clinicopathological parameters and prognostic significance of HER2 expression in gastric cancer patients: a meta-analysis of literature. World J Surg Oncol 2017;15:68. [Crossref] [PubMed]
- Uprak TK, Attaallah W, Çelikel ÇA, et al. HER-2 incidence in gastric cancer, its association with prognosis and clinicopathological parameters. Ulus Cerrahi Derg 2015;31:207-13. [Crossref] [PubMed]
- Zhang LX, Wei ZJ, Xu AM, et al. Can the neutrophil-lymphocyte ratio and platelet-lymphocyte ratio be beneficial in predicting lymph node metastasis and promising prognostic markers of gastric cancer patients? Tumor maker retrospective study. Int J Surg 2018;56:320-7. [Crossref] [PubMed]
- Pang L, Wang J, Fan Y, et al. Correlations of TNM staging and lymph node metastasis of gastric cancer with MRI features and VEGF expression. Cancer Biomark 2018;23:53-9. [Crossref] [PubMed]
- Rodríguez-Enríquez S, Pacheco-Velázquez SC, Gallardo-Pérez JC, et al. Multi-biomarker pattern for tumor identification and prognosis. J Cell Biochem 2011;112:2703-15. [Crossref] [PubMed]
- Huang C, Liu Z, Xiao L, et al. Clinical Significance of Serum CA125, CA19-9, CA72-4, and Fibrinogen-to-Lymphocyte Ratio in Gastric Cancer With Peritoneal Dissemination. Front Oncol 2019;9:1159. [Crossref] [PubMed]
- Emoto S, Ishigami H, Yamashita H, et al. Clinical significance of CA125 and CA72-4 in gastric cancer with peritoneal dissemination. Gastric Cancer 2012;15:154-61. [Crossref] [PubMed]
- Nakata B. Serum CA 125 level as a predictor of peritoneal dissemination in patients with gastric carcinoma. Cancer 1998;83:2488-92. [Crossref] [PubMed]