Voice parameters for difficult mask ventilation evaluation: an observational study
Introduction
Mask ventilation (MV) is a crucial aspect of airway management in general anesthesia (1). Successful MV is extremely important to maintain airway patency for optimal oxygenation of the tissues. Ability to ventilate the patient with a mask is also a core rescue skill during difficult or failed tracheal intubation situations (2). Difficult mask ventilation (DMV) is defined as a situation in which it is not possible for the anesthesiologist to provide adequate ventilation, due to inadequate mask seal, excessive gas leak, or excessive resistance to the ingress or egress of gas, according to the American Society of Anesthesiologists (ASA) Task Force on Management of the Difficult Airway (3). The reported incidence of DMV varies widely (0.08–15%) due to the lack of a precise and objective definition (4-9). It is believed that DMV is the major contributing factor for severe anesthesia-related complications including death or hypoxic brain injury (10,11). Therefore, the accurate prediction of DMV is of significant importance. Previous studies have reported that age >55 years, body mass index (BMI) >26 kg/m2, presence of a beard, lack of teeth, and history of snoring are 5 independent risk factors for DMV (4,6,7,12). In addition, previous neck radiation, thick or short neck, modified Mallampati class 3 or 4, and limited mandibular protrusion are also known as predictors for DMV (13). Unfortunately, the diagnostic accuracy of the above predictors has remained poor. In a large cohort study, DMV was unanticipated in 808 of 857 (94%) cases (14). Nørskov et al. (15) developed a scoring system [named the difficult facemask (DIFFMASK) score] based on 13 different predictive factors of DMV, which yielded a sensitivity of 85% and specificity of just 59%. Thus, further studies are needed to investigate accurate predictors for DMV.
The human voice is complex signal of sound emanating from our vocal cords, which is not only a primary mean of communicating with each other, but also contains a large amount of characteristic information of the human body (16). Prior studies have suggested that voice parameters can reflect anatomical features of the upper airway, including the opening of the mandible, the length of mandible, maxilla, and the laryngeal tube, and the volume of the oral and pharyngeal cavities (17,18). Some studies have found that patients with obstructive sleep apnea (OSA; a disease associated with DMV) show significant changes in acoustic parameters compared to healthy controls, and acoustic analysis showed potential in the assessment of OSA (19-21).
Formant is one of the most important parameters in the acoustic analysis, representing the vocal tract resonance, and relating to the upper airway anatomy (18,19). At present, only studies reported by de Carvalho et al. (22,23) have provided some evidence supporting an association between formant frequencies and difficult airway. Considering the influence of different races on pronunciation manner and the incidence of DMV, and the scarcity of literature on the voice features used for MV evaluation, we conducted this study in the Chinese population to confirm the initial findings by de Carvalho et al. (22), and to further clarify the potential value of voice analysis of both vowels and consonants to predict DMV. We hypothesized that voice features may be considered an alternative valuable predictor, in addition to already known factors for DMV in patients scheduled for general anesthesia. We present the following article in accordance with the STROBE reporting checklist (available at https://dx.doi.org/10.21037/atm-21-6274).
Methods
Patients
This observational study was conducted between December 2020 and July 2021 after obtaining approval from the Ethics Committee of Shanghai Ninth People’s Hospital (No. SH9H-2020-T386-1). The protocol is registered in ClinicalTrials.gov (trial registration No. NCT 04458220). The study was conducted in accordance with the Declaration of Helsinki (as revised in 2013).
The inclusion criteria for the study were patients scheduled for elective surgery under general anesthesia, aged 18 years and above and native Mandarin speakers. The exclusion criteria were as follows: ASA physical status class IV; contraindications for MV (rapid sequence induction, awake intubation in the case of expected difficult airway); participants with a previous history of speech disorders, vocal cord disease, cleft palate, craniofacial syndromes, hearing or perception abnormalities, mental or central nervous system disease, or those who had participated in other relevant clinical investigation in the past 3 months. Informed consent was provided by each participant before their inclusion.
Preoperative airway assessment
During the preoperative visit, the demographic properties of age, gender, weight, height, and body mass index (BMI) and the following information were recorded: lack of teeth, presence of beard, history of snoring, history of neck radiotherapy, inter-incisor gap (IIG), modified Mallampati test (MMT), thyromental distance (TMD), mandibular protrusion, neck circumference (NC), and cervical spine mobility. To reduce measurement bias, all researchers received repeated educational sessions prior to this trial.
The IIG refers to the maximal distance between the upper and lower incisors, which is used to assess the mouth opening capacity. The MMT was performed with the patient seated and their head in full extension, tongue out, and without pronunciation (24). The TMD refers to the distance between the uppermost border of the thyroid cartilage and the mentum, and was measured with the neck extended and the mouth closed (25). Mandibular protrusion assesses the range of movement of the mandible by asking patients to move their lower teeth past their upper teeth (25). The NC was measured at the level of the cricothyroid membrane. Cervical spine mobility (25) was measured by asking the patient to fully extend and flex the head and neck, and classified as grade 1 >90°, grade 2 =90°, or grade 3 <90°.
Voice data and analysis
Recording
The voice samples were selected from the Chinese phonetic alphabet (https://github.com/Samurais/DaCiDian). All participants were asked to phonate the Chinese vowels: [a], [o], [e], [i], [u], and [ü]; consonants: interdentals [ci], post-alveolar [chi], alveolar [le], alveo-palatals [qi], velars [ke]; and nasal vowel [en] (based on the anteroposterior position of the tongue and the location of air friction distributed along the vocal tract). All recordings were performed with the participant in a seated position in a quiet room in comfortable conditions. A 16-bit hand-held recorder with a sampling frequency of 44.1 kHz (Sony, ICD-PX470) was used to record the participants’ speech. A distance of 5 cm was maintained between the mouth and the recorder for each recording. The duration of each sound and the interval between sounds was approximately 1 second.
Voice parameters abstraction
The voice samples were first identified and aligned using a Kaldi chain (26) model trained on the AISHELL-2 (27) dataset, which enabled the exact position of syllable in the speech samples to be determined. Conventionally, formant frequencies refer to more intense frequencies within the envelope of the spectrum, which are computed based on the number of energy maxima per period, and bandwidth is measured as the width of a formant between 2 points that are 3 dB below the peak on either side of it. In this study, the Praat software program (https://www.praat.org/) was used to extract the pitch (fundamental frequency), the first 4 formant frequencies (f1–f4), and bandwidths (bw1–bw4). For all the above features, the mean value along with the time of the clip where the syllable was located was taken as the final result. This voice analysis was conducted by a specialist in speech technology who was blinded to the airway evaluation and MV classifications.
Induction of anesthesia and MV evaluation
An anesthesiologist with more than 3 years of experience was assigned to airway management. During induction of anesthesia, the patient’s head and neck were placed in a sniffing position. Preoxygenation of each patient was performed for 4 min by mask with 100% O2. Each patient was routinely monitored during the whole procedure by electrocardiography, peripheral oxygen saturation (SpO2), and end-tidal carbon dioxide. General anesthesia was induced with a combination of midazolam 0.05 mg/kg, fentanyl 2–4 µg/kg, propofol 2–2.5 mg/kg, and rocuronium 0.6 mg/kg. Evaluation of MV was made after induction of anesthesia. According to Langeron et al., MV is considered difficult in the case of: (I) inability for the unassisted anesthesiologist to maintain oxygen saturation >92% using 100% oxygen and positive pressure ventilation, (II) important gas flow leak, (III) necessity to increase the gas flow to greater than 15 L/min and to use the oxygen flush valve more than twice, (IV) no perceptible chest movement, (V) performing a 2-handed MV technique, and (VI) operator change.
Statistical analysis
Measurement data were expressed as mean ± standard deviation, and categorical variables were expressed as frequency (%). The hypothesis was tested using one-way analysis of variance (ANOVA), the Mann-Whitney U test, and Fisher’s exact probability method. Univariate and multivariate logistic regression models were established using the general linear model to evaluate the relationship between the voice features and DMV grade. Covariables for multivariate regression including gender (male, female), age (as a continuous variable), height, weight, BMI, IIG, NC, and snoring history. Stepwise logistic model and the ROC curve was used to analyze the predictive value of voice parameters to predict DMV, expressed as the AUC with its 95% confidence interval (95% CI). A P<0.05 indicated statistical significance. The dataset was randomly split into the training and testing sets at a ratio of 8:2, and the random seed was 2021. The data analysis was performed using the R project software program (R 4.0.4; https://cran.r-project.org/bin/windows/base/old/4.0.4/).
Results
Baseline characteristics
A total of 1,270 eligible patients were initially recruited. After excluding cases with missing data related to voice parameters and with contraindications for MV, 1,160 cases were subjected to statistical analysis (Figure 1). A summary of perioperative baseline characteristics by group is presented in Table 1. Difficulties of MV were encountered in 218 of 1,160 (18.8%) participants. Between 2 groups, statistically significant differences were revealed in age, gender, BMI, MMT, NC, mandibular protrusion, cervical spine mobility, history of snoring, and neck radiotherapy, while no difference were observed in IIG, TMD, beard, and no teeth.
Table 1
Risk factors | Overall (n=1,160) | Easy MV (n=942) | Difficult MV (n=218) | P value |
---|---|---|---|---|
Age (years) | 34.62±12.72 | 33.18±11.79 | 40.81±14.62 | <0.001 |
Gender | <0.001 | |||
Female | 702 (60.5) | 634 (67.3) | 68 (31.2) | |
Male | 458 (39.5) | 308 (32.7) | 150 (68.8) | |
BMI (kg/m2) | 21.86±3.30 | 21.34±3.02 | 24.12±3.50 | <0.001 |
Snoring history | <0.001 | |||
Yes | 447 (39.0) | 309 (33.3) | 138 (63.9) | |
No | 698 (61.0) | 620 (66.7) | 78 (36.1) | |
Neck radiotherapy | 0.001 | |||
Yes | 11 (1.0) | 4 (0.4) | 7 (3.2) | |
No | 1,144 (99.0) | 934 (99.6) | 210 (96.8) | |
Beard | 0.464 | |||
Yes | 3 (0.3) | 2 (0.2) | 1 (0.5) | |
No | 1,155 (99.7) | 939 (99.8) | 216 (99.5) | |
No teeth | 0.163 | |||
Yes | 4 (0.3) | 2 (0.2) | 2 (0.9) | |
No | 1,153 (99.7) | 937 (99.8) | 216 (99.1) | |
IIG (cm) | 4.06±0.98 | 4.06±0.97 | 4.03±1.03 | 0.656 |
TMD (cm) | 9.36±1.61 | 9.38±1.61 | 9.28±1.65 | 0.401 |
NC (cm) | 34.85±3.84 | 34.14±3.47 | 37.89±3.88 | <0.001 |
MMT | 0.001 | |||
1 | 358 (31.2) | 311 (33.4) | 47 (21.8) | |
2 | 274 (23.9) | 226 (24.3) | 48 (22.2) | |
3 | 395 (34.5) | 308 (33.1) | 87 (40.3) | |
4 | 119 (10.4) | 85 (9.1) | 34 (15.7) | |
Mandibular protrusion | 0.010 | |||
1 | 1,045 (90.9) | 860 (92.2) | 185 (85.6) | |
2 | 81 (7.0) | 56 (6.0) | 25 (11.6) | |
3 | 23 (2.0) | 17 (1.8) | 6 (2.8) | |
Cervical spine mobility | <0.001 | |||
1 | 1,121 (98.0) | 919 (98.9) | 202 (94.0) | |
2 | 21 (1.8) | 9 (1.0) | 12 (5.6) | |
3 | 2 (0.2) | 1 (0.1) | 1 (0.5) |
Data are shown as number (percentage) or mean ± SD. MV, mask ventilation; SD, standard deviation; BMI, body mass index; MMT, modified Mallampati test; NC, neck circumference; IIG; inter-incisor gap; TMD, thyromental distance.
Univariate and multivariate logistic regression
Univariate logistic regression analysis revealed that 69 voice parameters differed significantly between the difficult group and easy group with P values <0.05 (Table S1).
Univariate logistic regression analysis of clinical airway assessment variables and DMV (Table 2) showed that age, gender (male), BMI, snoring history, neck radiotherapy, NC, MMT (grade 3/4), mandibular protrusion (grade 2), and cervical spine mobility (grade 2) were significantly associated with DMV (P<0.01).
Table 2
Variables | OR | 95% CI | P value |
---|---|---|---|
Age (years) | 1.043 | 1.032–1.055 | <0.001 |
Gender | |||
Female | Reference | ||
Male | 4.541 | 3.320–6.266 | <0.001 |
BMI (kg/m2) | 1.290 | 1.230–1.355 | <0.001 |
Snoring history | 3.550 | 2.612–4.854 | <0.001 |
Neck radiotherapy | 7.783 | 2.329–29.941 | 0.001 |
Beard | 2.174 | 0.101–22.792 | 0.527 |
No teeth | 4.338 | 0.518–36.319 | 0.143 |
IIG (cm) | 0.966 | 0.832–1.124 | 0.655 |
TMD (cm) | 0.961 | 0.877–1.053 | 0.400 |
NC (cm) | 1.295 | 1.241–1.353 | <0.001 |
MMT | |||
1 | Reference | ||
2 | 1.405 | 0.907–2.179 | 0.127 |
3 | 1.869 | 1.273–2.772 | 0.002 |
4 | 2.647 | 1.595–4.367 | <0.001 |
Mandibular protrusion | |||
1 | Reference | ||
2 | 2.075 | 1.244–3.377 | 0.004 |
3 | 1.641 | 0.585–4.009 | 0.304 |
Cervical spine mobility | |||
1 | Reference | ||
2 | 6.066 | 2.534–15.045 | <0.001 |
3 | 4.550 | 0.179–115.369 | 0.285 |
DMV, difficult mask ventilation; OR, odds ratio; CI, confidence interval. BMI, body mass index; MMT, modified Mallampati test; NC, neck circumference; IIG; inter-incisor gap; TMD, thyromental distance.
All the significant risk factors with P values <0.05 found by univariate analysis were subjected to multivariate analysis to identify independent predictors for DMV. Table 3 shows that 9 voice parameters (o_f3, e_f3, e_f4, i_f2, i_f3, u_f4, ci_f3, ci_f4, en_f3) were independently related to DMV (P<0.05), after adjusting for gender, age, height, weight, BMI, IIG, NC, and snoring history.
Table 3
Voice parameters | OR | 95% CI | P value |
---|---|---|---|
o_f3 | 0.999 | 0.997–1.000 | 0.031 |
e_f3 | 0.999 | 0.997–1.000 | 0.019 |
e_f4 | 0.999 | 0.998–1.000 | 0.040 |
i_f2 | 0.999 | 0.998–1.000 | 0.019 |
i_f3 | 0.998 | 0.997–0.999 | 0.002 |
u_f4 | 0.998 | 0.997–1.000 | 0.009 |
ci_f3 | 0.998 | 0.997–1.000 | 0.018 |
ci_f4 | 0.998 | 0.997–1.000 | 0.023 |
en_f3 | 0.999 | 0.998–1.000 | 0.029 |
DMV, difficult mask ventilation; OR, odds ratio; CI, confidence interval.
Establishment of the assessment model
We used forward stepwise logistic regression for the whole sample to further construct the predictive model for DMV. The 20 selected voice parameters (including o_f4, e_bw2, i_f3, u_pitch, u_f1, u_f4, ü_bw4, ci_f1, qi_f1, qi_f4, qi_bw4, chi_f1, chi_bw2, chi_bw4, le_pitch, le_bw3, ke_bw2, en_pitch, en_f2, en_bw4) are presented in Table 4.
Table 4
Voice parameters | OR | 95% CI | P value |
---|---|---|---|
o_f4 | 1.002 | 1.000–1.004 | 0.015 |
e_bw2 | 0.999 | 0.997–1.000 | 0.032 |
i_f3 | 0.998 | 0.997–0.999 | 0.001 |
u_pitch | 0.989 | 0.980 –0.999 | 0.026 |
u_f1 | 0.996 | 0.993–0.999 | 0.004 |
u_f4 | 0.998 | 0.997–1.000 | 0.023 |
ü_bw4 | 1.001 | 1.000–1.003 | 0.036 |
ci_f1 | 0.995 | 0.992–0.998 | 0.001 |
qi_f1 | 1.004 | 1.001–1.007 | 0.006 |
qi_f4 | 0.999 | 0.997–1.000 | 0.070 |
qi_bw4 | 1.002 | 1.000–1.003 | 0.032 |
chi_f1 | 1.004 | 1.002–1.007 | 0.002 |
chi_bw2 | 0.999 | 0.997–1.000 | 0.055 |
chi_bw4 | 0.999 | 0.997–1.000 | 0.021 |
le_bw3 | 0.999 | 0.998–1.000 | 0.096 |
le_pitch | 1.014 | 1.007–1.023 | < 0.001 |
ke_bw2 | 1.002 | 1.000–1.003 | 0.022 |
en_pitch | 0.987 | 0.977–0.997 | 0.010 |
en_f2 | 0.999 | 0.998–1.000 | 0.127 |
en_bw4 | 0.999 | 0.998–1.000 | 0.046 |
DMV, difficult mask ventilation; OR, odds ratio; CI, confidence interval.
The ROC curve analysis showed that the AUC of the forward stepwise model for predicting DMV was 0.779 (95% CI: 0.710–0.848) (Figure 2), The highest point of the Youden index was designated as the threshold, which revealed the sensitivity and specificity of 71.0% (95% CI: 63.5–86.5%) and 75.0% (95% CI: 63.9–77.6%), respectively.
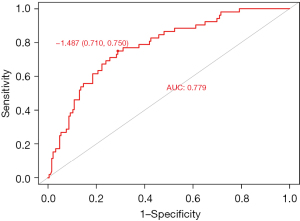
Discussion
Ventilation is frequently initiated manually with bag and face mask in the delivery of general anesthesia, which is the most basic, yet the most essential technique of ventilation before endotracheal intubation or insertion of any airway device. However, the prediction of DMV is still poorly addressed in current airway management research (12,14,28). Meanwhile, previous studies have focused primarily on physical signs of difficult airway without analyzing changes in voice. The main result of this study was that voice features can have a role in the prediction of DMV. The experimental results showed that both formant frequencies and bandwidths can make significant contributions to the evaluation of MV difficulty.
Regarding formant frequencies, our results showed that the first 4 formants presented a correlation with DMV. Formant frequencies are the distinguishing frequency components of human articulation that are dependent on the shape and length of the vocal tract in addition to the movement of the articulators (29,30). The OSA, known as an independent risk factor for DMV, has been revealed to display obvious alterations in formant frequencies due to the deposition of redundant tissues in the upper airway and reduced posterior airway space behind the base of the tongue (19,21). Macari et al. (18) previously reported a significant negative relation between the formant frequencies F3 and F4 and the length of the mandible and maxilla. This may be the underlying theoretical basis for the relationship between formant frequencies and MV difficulty.
When considering the results for bandwidths, we found there is an association between bw2/bw3/bw4 and DMV. Based on the pronunciation process, excessive tissue compliance would tend to increase the sound damping within the vocal tract. The measurement of formant bandwidths would provide an estimate of vocal tract compliance (20). The most common pathogenesis of the DMV is excessive pharyngeal compliance which would be expected to result in upper airway collapse or obstruction occurring after induction of general anesthesia (2). Results in the study by Robb et al. (20), which were extracted from the analysis of sustained phonations of vowel sounds, showed a significantly wider formant bandwidth in OSA patients compared with non-OSA participants, which provides an anatomic interpretation for our results.
Similar to other studies, our study also suggested that age, gender, BMI, and snoring are risk factors related to DMV. These risk factors also significantly affect the pronunciation process. Different speech features have been reported to be correlated with age, gender, height, and weight, due to the changes in the anatomy and physiology of the speech production system. Most reports have agreed that formant frequencies are negatively correlated with age and body size (31-33). For obese patients, abnormal fat deposition in the upper airway may interfere with voice production (34). Moreover, previous studies have provided evidence for an association between OSA and abnormal or particular speech features, which has mainly been attributed to alterations in upper airway anatomy such as craniofacial abnormalities, dental occlusion, relaxed pharyngeal soft tissues, large tongue base, and so on (19,21). Hence, when evaluating the association between voice parameters and DMV, we adjusted for age, gender, height, weight, BMI, IIG, NC, and snoring history in the multivariate regression. The results showed that only formants of [o], [e], [i], [u], [en], and [ci] were independently correlated with DMV.
Our study differs from the previous work by de Carvalho et al. (22). To our knowledge, this study was the first attempt to analyze the association between voice features and DMV in a Chinese population. There are discernably different cranial and facial structures between Western and Asian populations, which would lead to variations in vocal tract structure and pronunciation manners. Our results showed the applicability of acoustic evaluation in the prediction of DMV among Chinese participants. Furthermore, our speech samples consisted of both vowels and consonants. Vowels are the most frequently discussed syllables in the literature, which are produced without obvious tongue movement, representing resonances of the vocal tract, whereas the consonants are primarily determined by the relative positions of articulator including the tongue, teeth, lip, and soft palate (35,36). Our results demonstrated that both vowels ([o], [e], [i], [u], [ü], and [en]) and consonants ([ci], [chi], [le], [qi], and [ke]) are important predictors for DMV. Up to now, there is no universal definition and detection equipment for DMV, because DMV is not a disease, neither is it just one particular anatomical characteristic of patient physiognomy, it is a clinical situation mostly requiring a clinician’s judgment. Considering that ASA Task Force’s general definition (3) was vague and partly subjective, while the definition by Han et al. (37) was too stringent that it may result in underestimating the incidence of clinically significant difficult ventilation, in this study we rated MV according to Langeron et al. (4). Thus, different DMV definitions will lead to variations in incidence among different studies, and also affect the comparability of the model performance.
There were still some deficiencies in this study. The results presented in this paper were limited to the voices of Chinese speakers, so comparisons with other languages will require a more careful analysis of language-dependent acoustic traits. Another limitation in our study was that all patients’ voice were recorded in the sitting position. It is known that the patients were mostly placed in a supine position during induction of anesthesia, therefore changes of voice parameters measured in 2 distinct positions should be considered in further study to improve the accuracy of the predictive model. Moreover, our study was conducted in the context of elective intubation in the operating room, and voice recording requires the patients to be awake and cooperative, thus our method cannot be used to predict difficult airway in critically ill patients and in the case of emergency surgery. As the study was conducted on a general adult population, further studies including a varied age groups are needed to determine whether the relationship between DMV and voice parameters found in this study applies to other groups. In terms of technical factors, values of acoustic measures may vary with the software used. In these cases, other acoustic software programs would have to be used to analyze voice data.
In summary, the voice parameters may prove valuable information in MV evaluation of patients scheduled for general anesthesia. Compared to other currently used methods, voice parameter-based methods are promising as they are nonintrusive and can work as an automated and objective method to simultaneously analyze several voice parameters and thus may promote the development of and telematic airway evaluation. However, external, prospective validation is needed to fully realize the applicability of this novel approach in the assessment of difficult airway.
Acknowledgments
Funding: This study was supported by the Interdisciplinary Program of Shanghai Jiao Tong University (ZH2018ZDA14), the Health Industry Clinical Research Specialized Project of Shanghai Municipal Health Commission (202140410), the Clinical Research Plan of SHDC (SHDC2020CR3043B) and Clinical Research Program of 9th People’s Hospital, Shanghai Jiao Tong University School of Medicine (JYLJ202013).
Footnote
Reporting Checklist: The authors have completed the STROBE reporting checklist. Available at https://dx.doi.org/10.21037/atm-21-6274
Data Sharing Statement: Available at https://dx.doi.org/10.21037/atm-21-6274
Conflicts of Interest: All authors have completed the ICMJE uniform disclosure form (available at https://dx.doi.org/10.21037/atm-21-6274). The authors have no conflicts of interest to declare.
Ethical Statement: The authors are accountable for all aspects of the work in ensuring that questions related to the accuracy or integrity of any part of the work are appropriately investigated and resolved. The study was conducted in accordance with the Declaration of Helsinki (as revised in 2013). This observational study was approved by the Ethics Committee of Shanghai Ninth People’s Hospital (No. SH9H-2020-T386-1). Informed consent was provided by each participant before their inclusion.
Open Access Statement: This is an Open Access article distributed in accordance with the Creative Commons Attribution-NonCommercial-NoDerivs 4.0 International License (CC BY-NC-ND 4.0), which permits the non-commercial replication and distribution of the article with the strict proviso that no changes or edits are made and the original work is properly cited (including links to both the formal publication through the relevant DOI and the license). See: https://creativecommons.org/licenses/by-nc-nd/4.0/.
References
- Heidegger T. Management of the Difficult Airway. N Engl J Med 2021;384:1836-47. [Crossref] [PubMed]
- El-Orbany M, Woehlck HJ. Difficult mask ventilation. Anesth Analg 2009;109:1870-80. [Crossref] [PubMed]
- Apfelbaum JL, Hagberg CA, Caplan RA, et al. Practice guidelines for management of the difficult airway: an updated report by the American Society of Anesthesiologists Task Force on Management of the Difficult Airway. Anesthesiology 2013;118:251-70. [Crossref] [PubMed]
- Langeron O, Masso E, Huraux C, et al. Prediction of difficult mask ventilation. Anesthesiology 2000;92:1229-36. [Crossref] [PubMed]
- Yildiz TS, Solak M, Toker K. The incidence and risk factors of difficult mask ventilation. J Anesth 2005;19:7-11. [Crossref] [PubMed]
- Kheterpal S, Han R, Tremper KK, et al. Incidence and predictors of difficult and impossible mask ventilation. Anesthesiology 2006;105:885-91. [Crossref] [PubMed]
- Kheterpal S, Healy D, Aziz MF, et al. Incidence, predictors, and outcome of difficult mask ventilation combined with difficult laryngoscopy: a report from the multicenter perioperative outcomes group. Anesthesiology 2013;119:1360-9. [Crossref] [PubMed]
- el-Ganzouri AR, McCarthy RJ, Tuman KJ, et al. Preoperative airway assessment: predictive value of a multivariate risk index. Anesth Analg 1996;82:1197-204. [PubMed]
- Williamson JA, Webb RK, Szekely S, et al. The Australian Incident Monitoring Study. Difficult intubation: an analysis of 2000 incident reports. Anaesth Intensive Care 1993;21:602-7. [Crossref] [PubMed]
- Cook TM, Woodall N, Frerk C, et al. Major complications of airway management in the UK: results of the Fourth National Audit Project of the Royal College of Anaesthetists and the Difficult Airway Society. Part 1: anaesthesia. Br J Anaesth 2011;106:617-31. [Crossref] [PubMed]
- Cheney FW, Posner KL, Lee LA, et al. Trends in anesthesia-related death and brain damage: A closed claims analysis. Anesthesiology 2006;105:1081-6. [Crossref] [PubMed]
- Heinrich S, Birkholz T, Irouschek A, et al. Incidences and predictors of difficult laryngoscopy in adult patients undergoing general anesthesia: a single-center analysis of 102,305 cases. J Anesth 2013;27:815-21. [Crossref] [PubMed]
- Law JA, Duggan LV, Asselin M, et al. Canadian Airway Focus Group updated consensus-based recommendations for management of the difficult airway: part 2. Planning and implementing safe management of the patient with an anticipated difficult airway. Can J Anaesth 2021;68:1405-36. [Crossref] [PubMed]
- Nørskov AK, Rosenstock CV, Wetterslev J, et al. Diagnostic accuracy of anaesthesiologists' prediction of difficult airway management in daily clinical practice: a cohort study of 188 064 patients registered in the Danish Anaesthesia Database. Anaesthesia 2015;70:272-81. [Crossref] [PubMed]
- Nørskov AK, Wetterslev J, Rosenstock CV, et al. Prediction of difficult mask ventilation using a systematic assessment of risk factors vs. existing practice - a cluster randomised clinical trial in 94,006 patients. Anaesthesia 2017;72:296-308. [Crossref] [PubMed]
- Sataloff RT, Heman-Ackah YD, Hawkshaw MJ. Clinical anatomy and physiology of the voice. Otolaryngol Clin North Am 2007;40:909-29. v. [Crossref] [PubMed]
- Lim M, Lin E, Bones P. Vowel effect on glottal parameters and the magnitude of jaw opening. J Voice 2006;20:46-54. [Crossref] [PubMed]
- Macari AT, Karam IA, Tabri D, et al. Formants frequency and dispersion in relation to the length and projection of the upper and lower jaws. J Voice 2015;29:83-90. [Crossref] [PubMed]
- Montero Benavides A, Blanco Murillo JL, Fernández Pozo R, et al. Formant Frequencies and Bandwidths in Relation to Clinical Variables in an Obstructive Sleep Apnea Population. J Voice 2016;30:21-9. [Crossref] [PubMed]
- Robb MP, Yates J, Morgan EJ. Vocal tract resonance characteristics of adults with obstructive sleep apnea. Acta Otolaryngol 1997;117:760-3. [Crossref] [PubMed]
- Wei M, Du J, Wang X, et al. Voice disorders in severe obstructive sleep apnea patients and comparison of two acoustic analysis software programs: MDVP and Praat. Sleep Breath 2021;25:433-9. [Crossref] [PubMed]
- de Carvalho CC, da Silva DM, de Carvalho AD Jr, et al. Evaluation of the association between voice formants and difficult facemask ventilation. Eur J Anaesthesiol 2019;36:972-3. [Crossref] [PubMed]
- de Carvalho CC, da Silva DM, de Carvalho AD Junior, et al. Pre-operative voice evaluation as a hypothetical predictor of difficult laryngoscopy. Anaesthesia 2019;74:1147-52. [Crossref] [PubMed]
- Samsoon GL, Young JR. Difficult tracheal intubation: a retrospective study. Anaesthesia 1987;42:487-90. [Crossref] [PubMed]
- Detsky ME, Jivraj N, Adhikari NK, et al. Will This Patient Be Difficult to Intubate?: The Rational Clinical Examination Systematic Review. Jama 2019;321:493-503. [Crossref] [PubMed]
- Povey D, Ghoshal A, Boulianne G, et al. The Kaldi speech recognition toolkit. IEEE 2011 workshop on automatic speech recognition and understanding. IEEE Signal Processing Society, 2011 (CONF).
- Du J, Na X, Liu X, et al. Aishell-2: Transforming mandarin asr research into industrial scale. arXiv preprint arXiv:1808.10583, 2018.
- Lundstrøm LH, Rosenstock CV, Wetterslev J, et al. The DIFFMASK score for predicting difficult facemask ventilation: a cohort study of 46,804 patients. Anaesthesia 2019;74:1267-76. [Crossref] [PubMed]
- Macari AT, Ziade G, Turfe Z, et al. Correlation Between the Position of the Hyoid Bone on Lateral Cephalographs and Formant Frequencies. J Voice 2016;30:757.e21-.e26.
- Ladefoged P, Harshman R, Goldstein L, et al. Generating vocal tract shapes from formant frequencies. J Acoust Soc Am 1978;64:1027-35. [Crossref] [PubMed]
- Torre P 3rd, Barlow JA. Age-related changes in acoustic characteristics of adult speech. J Commun Disord 2009;42:324-33. [Crossref] [PubMed]
- Xue SA, Hao GJ. Changes in the human vocal tract due to aging and the acoustic correlates of speech production: a pilot study. J Speech Lang Hear Res 2003;46:689-701. [Crossref] [PubMed]
- Evans S, Neave N, Wakelin D. Relationships between vocal characteristics and body size and shape in human males: an evolutionary explanation for a deep male voice. Biol Psychol 2006;72:160-3. [Crossref] [PubMed]
- Takaki PB, Vieira MM, Said AV, et al. Does Body Mass Index Interfere in the Formation of Speech Formants? Int Arch Otorhinolaryngol 2018;22:45-9. [Crossref] [PubMed]
- Arnela M, Dabbaghchian S, Blandin R, et al. Influence of vocal tract geometry simplifications on the numerical simulation of vowel sounds. J Acoust Soc Am 2016;140:1707. [Crossref] [PubMed]
- Ghaemi H, Khoddami SM, Soleymani Z, et al. The Vocal Fold Dysfunction Questionnaire: Validity and Reliability of the Persian Version. J Voice 2018;32:710-4. [Crossref] [PubMed]
- Han R, Tremper KK, Kheterpal S, et al. Grading scale for mask ventilation. Anesthesiology 2004;101:267. [Crossref] [PubMed]
(English Language Editor: J. Jones)