Exploration of potential therapeutic targets for stroke based on the GEO database
Introduction
Stroke threatens the life and health of patients and causes long-term disability, which seriously reduces patients’ quality of life. In recent years, stroke has risen from the second leading cause of death to China’s leading cause of death. Ischemic stroke accounts for 70–85% of all strokes. At present, the only standard treatment for acute ischemic stroke is intravenous thrombolysis and mechanical thrombectomy with intravenous tissue plasminogen activator within 3 to 4.5 hours after the onset of symptoms. However, the narrow therapeutic time window of intravenous tissue plasminogen activator thrombolysis has prompted researchers to seek new therapeutic strategies and drugs. In recent years, due to the wide application of high-throughput sequencing technology, some substantial progress has been made in the study of the pathological mechanism of stroke. Studies have jointly analyzed mRNA and miRNA sequencing data sets, identified 6 core mRNAs and 2 regulated miRNAs in the pathogenesis of stroke, and explained the impact of systemic lupus erythematosus and atypical infections on stroke (1). Therefore, further studying the pathophysiological mechanisms of stroke development and recovery may provide a new and more effective approach to improving stroke patients’ prognoses. In general, non-coding RNAs, including long non-coding RNAs (lncRNAs) and microRNAs (miRNAs), are widely expressed in the human central nervous system and are related to a variety of nervous system diseases (2). Current research shows that lncRNAs play an important role in regulating gene expression (3) and participate in many vital biological processes, such as differentiation, organogenesis, apoptosis, genomic imprinting, regulation of mRNA splicing, and translation control (4). In recent years, many researchers have shown great interest in exploring the relationship between the lncRNA spectrum and stroke, and confirmed the key pathogenic role of lncRNA (5-7). A clinical study confirmed that lncRNA SNHG15 can be used as a diagnostic marker for ischemic stroke (5). Wang et al. (6) showed that H19 is associated with a good prognosis in stroke patients and may have a protective effect on brain tissue and nerves. Moskowitz et al. (7) showed that lncRNA is involved in neuronal cell regulation in stroke patients. Therefore, the purpose of this study is to jointly analyze the sequencing results of 2 groups of non-coding RNAs, screen the differentially expressed lncRNAs, and predict the target genes of the lncRNAs. This study aims to clarify the potential functions of lncRNAs and explore the potential biomarkers and therapeutic targets of stroke.
We present the following article in accordance with the MDAR checklist (available at https://dx.doi.org/10.21037/atm-21-5815).
Methods
Data download
The study was conducted in accordance with the Declaration of Helsinki (as revised in 2013). In this study, lncRNA sequencing data (GSE102541, GSE140275) of blood samples from stroke patients and healthy subjects were downloaded from the Gene Expression Omnibus (GEO) database. A total of 15 samples of lncRNA sequencing data were downloaded in this study, including 9 samples of stroke patients and 6 samples of healthy subjects. Among the 9 stroke patients, 6 cases were ischemic stroke and 3 cases were hemorrhagic stroke. A batch correction was performed on the 2 data groups to remove the impact of the experimental environment or experimental conditions on lncRNA sequencing results. The 2 data groups were normalized through standard correction and logarithmic operation and combined into a data matrix. The data matrix was the expression amount of lncRNAs in each sample. Since the lncRNA sequencing data in this study was open source, it did not need to be reviewed by the hospital ethics committee.
Difference analysis
The lncRNA sequencing (lncRNA-seq) data sets was analyzed by R software (V3.5.1) and the R package edgeR. Fold change (FC) = lncRNA expression in stroke samples/lncRNA expression in healthy control samples. The difference multiple was expressed in the form of log2FC, and a t-test was used. LncRNAs with significant differences were screened based on |log2FC| >1 and false discovery rate (FDR) <0.05.
Correlation analysis
R software and the R package corrplot were used to calculate the correlations between significantly different lncRNAs. The R package cyclize was used for visualization. Correlations between lncRNAs were tested by the Pearson test.
Target gene prediction and enrichment analysis
This study used the StarBase database (http://starbase.sysu.edu.cn/). Target genes were predicted for differential lncRNAs. Gene ontology (GO) functional enrichment analysis was carried out for related target genes. GO functional enrichment analysis was performed using DAVID data, and FDR <0.05 was used as the screening condition. The results of enrichment analysis were visualized by the R package ggplot.
Principal component analysis
Principal component analysis was performed based on the lncRNAs with the most significant expression in stroke blood samples. Through the dimensionality reduction of the data matrix, this study explored the differentiation of the most significant lncRNAs between stroke and healthy control samples and the characterization performance of the essential characteristics of stroke samples.
Statistical analysis
The data were statistically analyzed using R software and related R packages. P<0.05 was considered statistically significant.
Results
Differentially expressed lncRNAs
The research aimed to explore the correlation between lncRNA and stroke, so it focused on the screening of differentially expressed lncRNA in blood samples of stroke patients. A total of 239 differentially expressed lncRNAs were screened in 9 stroke blood samples and 6 healthy control blood samples. The results showed that 146 genes were upregulated, while 93 genes were downregulated, as shown in Figure 1. According to the |log2FC| value, the top 10 lncRNAs with the most significant enrichment were selected. The up-regulated lncRNAs were LINC02334, TARID, MRGPRF-AS1, CAI2, LINC00189, TUG1, and RNF5P1, respectively. The top downregulated lncRNAs were AC005180.2, ADAMTS9-AS1, and AC036108.3, respectively, as shown in Figure 2.
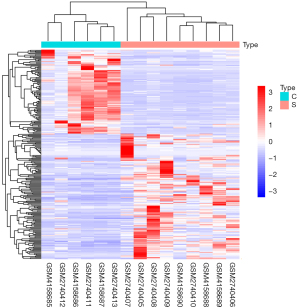
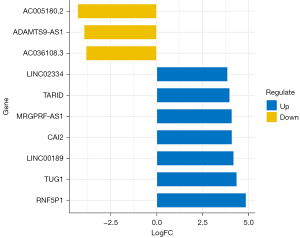
Correlation analysis
The 10 lncRNAs with the most significant differences were used for correlation analysis. There was a significant positive correlation between the downregulated genes and upregulated genes. A strong correlation was found among AC005180.2, ADAMTS9-AS1, and AC036108.3. Meanwhile, a strong correlation was observed between TARID, MRGPRF-AS1, LINC02334, and TUG1, as shown in Figure 3. Based on the correlation network diagram in Figure 4, CAI2, LINC00189, and RNF5P1 were at the core of the correlation network, indicating that they might be the critical lncRNAs for the pathogenesis of stroke.
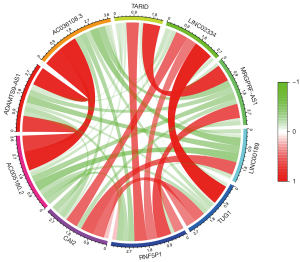
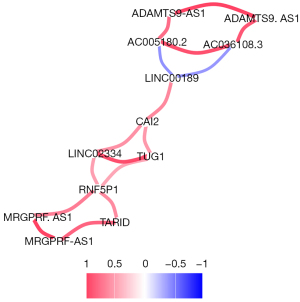
Target gene prediction
This study was based on StarBase (http://starbase.sysu.edu.cn/). The target genes of differential lncRNAs were predicted, and the relationships between mRNAs and lncRNAs are shown in Figure 5.
GO enrichment analysis
As shown in Figure 6, the results showed that the genes related to muscle contraction, positive regulation of RNA polymerase II promoter transcription, muscle structural components, focal adhesion, and endothelial cell chemotaxis were significantly enriched. Meanwhile, genes related to actin binding, actin cytoskeleton, actin filament binding, blood lipid regulation, positive regulation of smooth muscle contraction, and skeletal muscle cell differentiation increased in expression.
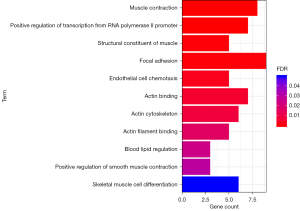
Principal component analysis
Principal component analysis showed that the 10 lncRNAs with significant differences could distinguish between stroke blood samples and healthy control blood samples. The significantly different lncRNAs identified in this study could characterize the essential characteristics of stroke, as shown in Figure 7.
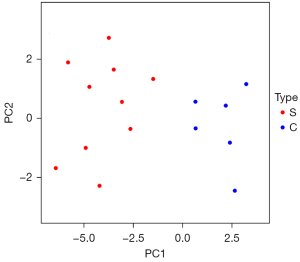
Discussion
Studies have shown that lncRNAs play an important role in the pathogenesis of stroke (6,8). The specific mechanisms and functions of most lncRNAs in neuroprotection and stroke diseases need to be further studied and clarified. In non-coding RNA sequencing analysis, abnormal expression of lncRNAs is closely related to the occurrence and progression of stroke. Other research confirmed that lncRNAs usually play essential roles in the occurrence and progression of stroke and the recovery of stroke (8,9). At present, research on the functions and mechanisms of lncRNAs is mainly focused on animal models of stroke. However, due to the noticeable biological and genetic differences between animals and humans, the human lncRNA spectrum must be further studied. In addition, the current research on this issue only focuses on a few lncRNAs, such as GAS5, MALAT1, SNHG1, and ANRIL (6,10,11). Therefore, the functions of most lncRNAs remain unclear.
This study combined 2 groups of lncRNA sequencing results (GSE102541 and GSE140275) for bioinformatics analysis. We screened 239 differentially expressed lncRNAs, of which 146 were upregulated and 93 were downregulated. The top upregulated lncRNAs with the most significant differences were LINC02334, TARID, MRGPRF-AS1, CAI2, LINC00189, TUG1, and RNF5P1. In contrast, AC005180.2, ADAMTS9-AS1, and AC036108.3 were downregulated. Most of these lncRNAs have been proven to play an important role in the pathogenesis of stroke. Previous studies found that RNF5P1 was highly expressed in patients with ischemic stroke while its expression was low in healthy people (12). RNF5P1 is an independent risk factor for ischemic stroke. It has a certain diagnostic value and is a potential biomarker for ischemic stroke. Wei et al. measured the content of lncRNA TUG1 in serum samples of stroke patients and healthy controls and found that TUG1 was highly expressed in blood samples of stroke patients and was correlated with an increased risk of ischemic stroke (13). Serum lncRNA TUG1 therefore has potential diagnostic value. Previous studies found that the expression level of lncRNA CAI2 in patients with acute ischemic stroke was higher than that in healthy controls (14). CAI2 was considered to correlate with the severity of acute stroke and is effective in diagnosing acute stroke. The change in CAI2 is a potential diagnostic marker (14).
This study further explored the correlations between the 10 most significant lncRNAs. We found a strong correlation among AC005180.2, ADAMTS9-AS1, and AC036108.3. Meanwhile, there was a strong correlation between TARID, MRGPRF-AS1, LINC02334, and TUG1, which were at the core of the correlation network and may be the key lncRNAs for the pathogenesis of stroke. The diagnostic value of CAI2 and RNF5P1 in stroke has been confirmed. Zhang et al. (15) found that lncRNA LINC00189 may be related to the occurrence and progression of squamous urothelial carcinoma by single-cell sequencing. Four lncRNAs, including HCG11, CASC15, LINC00189, and LINC00905, were shown to be significantly correlated with the deterioration of recurrence-free survival of cervical cancer (16). In addition, there are few related studies on other lncRNAs found in this study, and some functions have not been fully elucidated, which needs further experimental exploration.
To further explore the biological functions of lncRNAs with the most significant differences, this study predicted the target genes of lncRNAs through the StarBase database and performed GO functional enrichment analysis. The results showed that the target genes were enriched in muscle contraction, positive transcriptional regulation of RNA polymerase II promoter, muscle structural components, focal adhesion, endothelial chemotaxis, actin binding, actin cytoskeleton, actin filament binding, and blood lipid regulation. Other upregulated genes were related to positive regulation of smooth muscle contraction and skeletal muscle cell differentiation, among others. It is worth noting that of these functional items, blood lipid regulation and endothelial cell chemotaxis are closely related to the pathological basis of stroke. At the same time, focal adhesion is related to the adhesion and migration of vascular smooth muscle cells, which may lead to the occurrence and development of stroke. GO functional enrichment further confirmed that the most significant lncRNAs that were found in this study may play a vital role in the pathogenesis of stroke and are potential therapeutic targets. Based on the results of this study, we can further explore the diagnostic efficacy of differentially expressed lncRNA and its correlation with prognosis in clinical samples, and even further explore the significance of its targeted therapy.
There are some shortcomings in this study. First, the sample size is small, and the sample size needs to be further expanded to more comprehensively screen lncRNAs with significant differences in stroke. Second, due to the limitations of this study and the lack of sample size and clinical data, it is impossible to further explore the relationship between lncRNA and the prognosis of stroke patients. The clinical relevance of the differential lncRNA identified in this study, especially the impact on the prognosis of stroke patients, still needs to be confirmed by further clinical studies. The third is that this study lacks in vivo and in vitro experimental confirmation, as well as further exploration of the mechanism of differential lncRNA based on experiments.
This study conducted a principal component analysis based on the expression of the most significantly different lncRNAs, reduced the dimension of the data, and further explored the roles of these lncRNAs in stroke. The results showed that the 10 lncRNAs with significant differences could distinguish between stroke blood samples and healthy control blood samples. In other words, they could characterize the essential characteristics of stroke to a certain extent.
Conclusions
In conclusion, genes including LINC02334, TARID, MRGPRF-AS1, CAI2, LINC00189, TUG1, RNF5P1, AC005180.2, ADAMTS9-AS1, and AC036108.3 play an important role in the pathogenesis of stroke and may be potential therapeutic targets.
Acknowledgments
Funding: This research was supported by the National Natural Science Foundation of China Youth Science Foundation Project (No. 81804185) and the Zhejiang Provincial “Thirteenth Five-Year Plan” Key Specialty Construction Project Fund of Traditional Chinese Medicine [Zhejiang Health Office Traditional Chinese Medicine (2019) No. 1].
Footnote
Reporting Checklist: The authors have completed the MDAR checklist. Available at https://dx.doi.org/10.21037/atm-21-5815
Conflicts of Interest: All authors have completed the ICMJE uniform disclosure form (available at https://dx.doi.org/10.21037/atm-21-5815). The authors have no conflicts of interest to declare.
Ethical Statement: The authors are accountable for all aspects of the work in ensuring that questions related to the accuracy or integrity of any part of the work are appropriately investigated and resolved. The study was conducted in accordance with the Declaration of Helsinki (as revised in 2013).
Open Access Statement: This is an Open Access article distributed in accordance with the Creative Commons Attribution-NonCommercial-NoDerivs 4.0 International License (CC BY-NC-ND 4.0), which permits the non-commercial replication and distribution of the article with the strict proviso that no changes or edits are made and the original work is properly cited (including links to both the formal publication through the relevant DOI and the license). See: https://creativecommons.org/licenses/by-nc-nd/4.0/.
References
- Xie Q, Zhang X, Peng S, et al. Identification of novel biomarkers in ischemic stroke: a genome-wide integrated analysis. BMC Med Genet 2020;21:66. [Crossref] [PubMed]
- Qureshi IA, Mattick JS, Mehler MF. Long non-coding RNAs in nervous system function and disease. Brain Res 2010;1338:20-35. [Crossref] [PubMed]
- Martianov I, Ramadass A, Serra Barros A, et al. Repression of the human dihydrofolate reductase gene by a non-coding interfering transcript. Nature 2007;445:666-70. [Crossref] [PubMed]
- Mercer TR, Dinger ME, Mattick JS. Long non-coding RNAs: insights into functions. Nat Rev Genet 2009;10:155-9. [Crossref] [PubMed]
- Deng QW, Li S, Wang H, et al. Differential long noncoding RNA expressions in peripheral blood mononuclear cells for detection of acute ischemic stroke. Clin Sci (Lond) 2018;132:1597-614. [Crossref] [PubMed]
- Wang J, Zhao H, Fan Z, et al. Long Noncoding RNA H19 Promotes Neuroinflammation in Ischemic Stroke by Driving Histone Deacetylase 1-Dependent M1 Microglial Polarization. Stroke 2017;48:2211-2221. [Crossref] [PubMed]
- Moskowitz MA, Lo EH, Iadecola C. The science of stroke: mechanisms in search of treatments. Neuron 2010;67:181-98. Erratum in: Neuron 2010;68:161. [Crossref] [PubMed]
- Liu B, Cao W, Xue J. LncRNA ANRIL protects against oxygen and glucose deprivation (OGD)-induced injury in PC-12 cells: potential role in ischaemic stroke. Artif Cells Nanomed Biotechnol 2019;47:1384-95. [Crossref] [PubMed]
- Zhang L, Luo X, Chen F, et al. LncRNA SNHG1 regulates cerebrovascular pathologies as a competing endogenous RNA through HIF-1α/VEGF signaling in ischemic stroke. J Cell Biochem 2018;119:5460-72. [Crossref] [PubMed]
- Guo D, Ma J, Yan L, et al. Down-Regulation of Lncrna MALAT1 Attenuates Neuronal Cell Death Through Suppressing Beclin1-Dependent Autophagy by Regulating Mir-30a in Cerebral Ischemic Stroke. Cell Physiol Biochem 2017;43:182-94. [Crossref] [PubMed]
- Kopp F, Mendell JT. Functional Classification and Experimental Dissection of Long Noncoding RNAs. Cell 2018;172:393-407. [Crossref] [PubMed]
- Bao MH, Szeto V, Yang BB, et al. Long non-coding RNAs in ischemic stroke. Cell Death Dis 2018;9:281. [Crossref] [PubMed]
- Wei YS, Yang J, He YL, et al. A functional polymorphism in the promoter of TUG1 is associated with an increased risk of ischaemic stroke. J Cell Mol Med 2019;23:6173-81. [Crossref] [PubMed]
- Wang SW, Liu Z, Shi ZS. Non-Coding RNA in Acute Ischemic Stroke: Mechanisms, Biomarkers and Therapeutic Targets. Cell Transplant 2018;27:1763-77. [Crossref] [PubMed]
- Zhang X, Zhang M, Hou Y, et al. Single-cell analyses of transcriptional heterogeneity in squamous cell carcinoma of urinary bladder. Oncotarget 2016;7:66069-76. [Crossref] [PubMed]
- Zhang Y, Zhang X, Zhu H, et al. Identification of Potential Prognostic Long Non-Coding RNA Biomarkers for Predicting Recurrence in Patients with Cervical Cancer. Cancer Manag Res 2020;12:719-30. [Crossref] [PubMed]
(English Language Editor: C. Betlzar)