Ferroptosis-related genes identify tumor immune microenvironment characterization for the prediction of prognosis in cervical cancer
Introduction
Cervical squamous cell carcinoma and endocervical adenocarcinoma (CESC) are the fourth most common cancer among women worldwide. However, the lack of vaccination and screening programs in many developing countries has added to the difficulty in diagnosis (1). According to the International Agency for Research on Cancer (IARC), there are approximately 570,000 new cervical cancer (CC) cases and 311,400 deaths each year (2). CC accounts for 10–15% of all cancer-related deaths in women, second only to breast cancer (BC) in its mortality rate (3). Although current treatment strategies, such as surgery, radiation, and chemotherapy, show promise for CC patients, approximately 75% of patients experience disease progression and/or recurrence (4,5). Therefore, there is an urgent need to explore the carcinogenic mechanism of CESC and develop a new prognostic model of CESC.
The tumor microenvironment (TME) is closely related to CESC development, confirmed by numerous studies (6-8). For instance, de Vos van Steenwijk et al. found that mature M1 macrophages in tumors were associated with better survival, regardless of CD8+ T cell infiltration (6). In addition, the expression of low-risk cytochrome b (-245) beta subunit (CYBB) is also positively correlated with the degree of M1 macrophage infiltration. Meanwhile, treatment strategies targeting the TME are booming (9,10). But the lack of integration between our knowledge of tumor biology and the TME has hindered innovation in cancer therapy. It is becoming clear that not only cancer cells but also stromal cells should be targeted in carcinoma treatment (11). In addition, cancer cells have been shown to produce immunosuppression in the TME and then achieve immune escape (12). Therefore, a greater in-depth understanding of the correlation between the TME and cancer prognosis and the exploration of new treatment strategies for CC are urgently needed to improve targeted treatment.
In addition, cell death plays a critical role in the development of cancer. Cell death can be classified into two types, apoptosis and programmed necrosis (13). The type of ferroptosis studied in this work is an iron-dependent, non-apoptotic form of regulated cell death (RCD) characterized by iron-dependent lipid peroxidation (14). There are two critical events of the induction of ferroptosis which are Fe2+ accumulation and lipid peroxidation. Also, ferroptosis can be inhibited by iron chelators and lipophilic. Fe3+ is imported into cells by the transferrin receptor, then it will be converted to Fe2+. However, excess Fe2+ leads to the Fenton reaction which will produce lipid reactive oxygen species (ROS). The ROS will cause ferroptosis (15). The challenge of cancer treatment is to kill cancer cells while ensuring the safety of normal cells. Recent research has identified that cancer cells absorb more iron than normal cells, and the iron dependence of cancer cells makes them vulnerable to iron-catalyzed necrosis (16). Therefore, several studies are currently investigating the regulation of ferroptosis in various cancers, including pancreatic cancer, brain tumors, ovarian cancer, and BC (17-20) because ferroptosis is seen as a promising mechanism for killing drug-resistant cancer cells. For instance, the inactivation of (Glutathione Peroxidase 4) GPX4 is fatal to cancer cells in the mesenchymal state (21). An increasing number of ferroptosis-related genes (FRGs) have been discovered recently, and researchers hope to analyze the expression of FRGs to predict the overall survival (OS) of cancer patients. Several cancers, such as liver cancer, BC, and glioma, have been extensively studied (22-24), but CESC is rarely studied. So, confirming the role of ferroptosis in CESC is needed.
However, the relationship between ferroptosis and TME is unclear. Understanding the interactions may provide novel strategies to treating cancer (25). In a recent study, researchers found that ferroptosis can promote tumor growth by driving the polarization of macrophages in the TME (26). Another recent study showed that hypoxia-inducible factor (HIF) pathways is a positive trigger for ferroptosis in clear-cell carcinoma (CCC) (27). So, different with other reports which focused on constructing FRGs, we not only built a solid prediction model, but also tried to explain the connection between TME and ferroptosis by analyzing CYBB.
As with most solid tumors, CC has always been classified by the clinical staging classification system. As recommended by the CC staging guidelines from the Federation International of Gynecology and Obstetrics (FIGO), imaging modalities (e.g., chest X-rays) are initially used to evaluate the cancer stage, followed by the development of an appropriate treatment plan (28,29). However, the guidelines have limited usefulness in assessing the prognosis of patients with positive lymph nodes (30). Currently, with the accumulation of large-scale cancer genome data, the identification of cancer molecular subtypes using multi-omics data is approaching the goal of personalized medicine (31). Therefore, we made some classified innovations based on the TCGA-derived patient data. This study performed classification using the “consensus clustering” method and demonstrated the classification reliability by evaluating survival in different subtypes, cluster heat map, and silhouette width.
This study employed the ESTIMATE and CIBERSORT algorithms to analyze CESC tissue samples from TCGA-CESC database and to calculate the proportion of tumor-infiltrating immune cells (TICs) and stromal components in the samples. We also used a single-sample Gene Set Enrichment Analysis (ssGSEA) to assess the enrichment scores of particular gene sets in every single sample (32). Moreover, a series of analyses on FRGs in CESC was conducted, including univariate and multivariate Cox regressions, and a prognostic model consisting of phosphatidylethanolamine-binding protein 1 (PEBP1), dual oxidase 1 (DUOX1), iron-sulfur cluster assembly enzyme (ISCU), and CYBB was established. We also performed immune cell infiltration analysis to explore how ferroptosis effects on TME. In the crossover analysis with the immune microenvironment, CYBB was identified as a predictive biomarker and was used in the follow-up GSEA and immune infiltration analysis.
We present the following article in accordance with the REMARK reporting checklist (available at https://atm.amegroups.com/article/view/10.21037/atm-21-6265/rc).
Methods
Data collection
The Cancer Genome Atlas (TCGA, https://cancergenome.nih.gov/) was used to download CESC RNA sequencing (RNA-seq) transcriptome data. A total of 309 samples, including 306 tumor samples and 3 normal samples, were obtained. We obtained 60 FRGs from previous papers (22), and 259 from an authoritative public database from the website (https://www.zhounan.org/ferrdb/operations/download.html) (https://cdn.amegroups.cn/static/public/atm-21-6265-1.pdf). Finally, 267 FRGs were identified after intersection (Figure S1). The present study did not require approval from an ethics committee because TCGA and FerrDb are publicly accessible databases. The study was conducted in accordance with the Declaration of Helsinki (as revised in 2013).
Calculation of immune, stromal, and estimate scores
The ESTIMATE package (version 1.0.13) in R (version 4.0.5) was used to predict the proportion of immune mechanism components in the TME for each sample. The package generates immune scores, stromal scores, and estimate scores, where higher scores indicate a greater proportion of components corresponding to the TME.
Differentially expressed genes (DEGs) selected between the high and low groups identified by immune and stromal scores
The 306 tumor samples were labeled ‘high’ or ‘low’ based on a comparison with the immune and stromal score median scores. The gene expression was differentiated by the “limma” R package. DEGs were generated by comparison between the high- and low-score samples. DEGs required a fold change greater than 1 and a false discovery rate (FDR) <0.05 to be considered statistically significant.
Survival analyses
The “survival” (version 3.2-13) and “survminer” packages (version 0.4.9) in R were used to perform the survival analyses. Kaplan-Meier survival curves and log-rank tests were used to explore the differences in scores between the high and low groups.
Functional enrichment analysis and protein-protein interaction (PPI) network
The “clusterProfiler” (version 3.18.1), “enrichplot” (version 1.10.2), and “ggplot2” (version 3.3.5) packages were used for the analyses, and items with an FDR <0.05 were considered significantly enriched. The STRING database was used to construct a PPI network.
Relationship between CYBB and tumor immunoreaction
Two gene sets, Hallmark and C7, were used for the analysis, and the entire transcriptome of all tumor samples was used for the GSEA. The gene sets with an FDR <0.05 were considered significant. In addition, to understand the proportion of immune cells in each sample, the CIBERSORT algorithm was used to estimate the degree of infiltration. Furthermore, the correlation between the expression level of CYBB and the infiltration level of different immune cell types was calculated.
Establishment and validation of the prognostic model
We used a univariate Cox regression to identify FRGs with potential prognostic significance in the CESC samples. We then identified DEGs in the immune score and stromal score groups. CYBB was identified after intersection. Subsequently, we performed a multivariate Cox regression analysis for each prognostic gene associated with ferroptosis, and four genes were identified as candidates for a prognostic model. The risk score formula for each sample was presented as follows: risk score= β1x1 + β2x2 + … + βpxp (where βp denotes the coefficient and x p represents the gene expression level). To verify the accuracy and the sensitivity of the model, we used the “timeROC” package (version 0.4) in R to draw a time-dependent receiver operating characteristic curve (ROC). A Principal Component Analysis (PCA) was performed using the function “prcomp” in R to illustrate the accuracy of the assessment based on the high- and low-risk scores. Additionally, t-distributed Stochastic Neighbor Embedding (t-SNE) was performed using the “Rtsne” R package (version 0.15) to investigate the distribution of different groups.
Univariate and multivariate Cox regression analyses
A univariate regression analysis was used to assess the prognostic value of the risk score and clinical characteristics (age, grade, tumor, and metastasis). Subsequently, variables with P<0.05 were identified, and a multiple Cox regression analysis was performed to determine which variables could independently predict patient survival.
Consensus clustering (CC) for cancer typing
The consensus clustering (CC) algorithm is a method for cancer typing (33). It is performed by the “Execute CC” function built into the “CancerSubtypes” R package (version 1.12.1 for version 3.10 of Bioconductor). This cancer typing software uses multi-omics data to identify cancer subtypes and provides a standard framework for data preprocessing, feature selection, cancer subtype recognition, result validation, and visualization (31). To verify the results, we used clustering heat maps and the cumulative distribution function (CDF) to select the most appropriate number of clusters. Based on the number of candidate clusters, survival analysis was performed. The number of clusters with the most significant differences in survival was selected as the final number for CESC typing.
Statistical analysis
Pearson’s correlational analysis was performed to explore the relationships among the variables. Paired independent sample t-tests were conducted using the Mann-Whitney test to assess differences between the high- and low-risk groups. Statistical analyses were performed and visualized using R version 4.0.5.
Results
The workflow scheme
The analysis approach of the present study is shown in Figure 1. Firstly, the transcriptomic RNA-seq data of 309 samples (Table S1) were downloaded from the TCGA database, and then the prognostic signature was established using a multiple Cox regression. Additionally, we used ESTIMATE, CIBERSORT, and consensus clustering algorithms to analyze the data. The CYBB and IFNG (Interferon gamma) genes were obtained by the intersection of the FRGs following the univariate Cox analysis, and the DEGs were obtained by using the ESTIMATE algorithm. Then, we focused on CYBB for the subsequent analyses, including the survival analysis, Gene Set Enrichment Analysis (GSEA), and the related TICs. A prognostic model was subsequently established based on the identification of four FRGs, including CYBB, and evaluated using various methods. Finally, the consensus clustering algorithm was used to classify the obtained data.
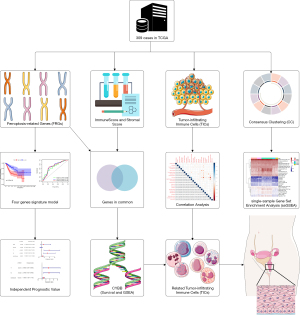
Survival analysis based on immune score, stromal score, and estimate score
The data from the TCGA database were scored, and the higher the score, the higher the relative content of specific cells. After scoring each sample, we divided the immune scores, stromal scores, and estimate scores into high and low groups, aiming to explore the association between scores and survival time. The immune score and stromal score represent the ratio of immune cells and stromal cells in the entire TME, respectively, while the estimate score is the sum of the immune and stromal scores. As shown in Figure 2A, the high immune score group had a higher five-year survival rate than the low immune score group. Although there was no significant relationship between the proportion of stromal cells and the survival rate, as shown in Figure 2B, the estimate score was positively correlated with the 5-year survival rate (Figure 2C). Furthermore, the results showed that the estimate score was a better prognostic predictor of CESC than the immune score or the stromal score.
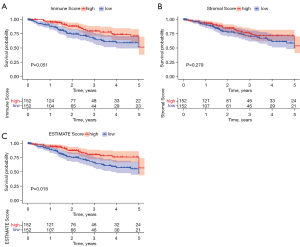
DEGs of the immune score and stromal score and their enriched immune function
Heat maps were drawn to describe the difference in gene expression between different groups divided by the median (Figure 3A,3B). A total of 1,067 DEGs were identified in the immune score group. Among them, 643 genes were upregulated, and 424 were downregulated (Figure 3A,3C). In the stromal score group, a total of 947 DEGs were identified, of which 917 genes were upregulated and 30 were downregulated (FDR <0.05, logFC >1), as shown in Figure 3B and Figure 3D. The DEGs from the different subgroups were intersected in upregulation and downregulation categories.
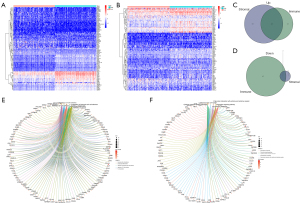
Specifically, there were 408 identical genes in upregulation and 17 identical genes in downregulation. In total, 425 DEGs were identified as potential key factors in determining the CESC TME. Gene Ontology (GO) and Kyoto Encyclopedia of Genes and Genomes (KEGG) enrichment analyses were performed on these DEGs. The GO analysis demonstrated that the DEGs mainly mapped onto leukocyte cell-cell adhesion, lymphocyte differentiation, regulation of leukocyte cell-cell adhesion, regulation of T cell activation, T cell activation, and other immune-related functions (Figure 3E). In contrast, the KEGG analysis revealed that many DEGs mapped to cytokine-cytokine receptor interaction, chemokine signaling pathway, and viral protein interaction with cytokine and cytokine receptor pathway (Figure 3F). Overall, the primary functions of the identified DEGs appeared to be related to immune activity, also illustrating the importance of these DEGs in the CESC TME.
Intersection analysis of FRGs and the TME
A total of 267 FRGs were included in this study, 53 of which were identified as significant by univariate Cox analysis (Figure 4A). To further explore the underlying mechanism, we used the STRING database to build a PPI network (Figure 4B). Then, these 53 genes were intersected with the 425 previously identified TME-related DEGs, and finally, two genes, CYBB and IFNG, were found (Figure 4C). After survival analysis, we found a significant correlation between CYBB and prognosis (Figure 4D).
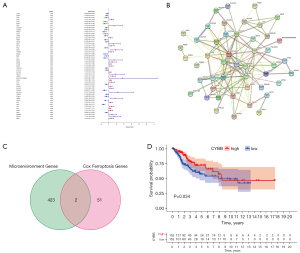
Establishment of the FRG prognostic risk model
After selecting CYBB, we found three other factors, namely PEBP1, DUOX1, and ISCU, through the multivariate Cox regression analysis (P<0.05). Subsequently, we constructed the following prognostic model: (–0.3149) × CYBB expression + (–1.1396) × DUOX1 expression + (–0.8738) × PEBP1 expression + (–0.9232) × ISCU expression. After the prognostic model was established, each sample was assigned a risk score. To perform the survival analysis, all samples were divided into high and low groups based on their risk score (Figure 5A). We found that low-risk patients lived significantly longer than high-risk patients. As the risk score increased, the number of deaths increased (Figure 5B-D). The results of “PCA” and “t-SNE” confirmed the accuracy of our CESC sample classification (Figure 5E,5F). Additionally, a ROC analysis was conducted to verify the accuracy and sensitivity of the model. The AUC of the prognostic model was greater than 0.7 for the 3-year survival predictions in all groups (Figure 5G), indicating that the model demonstrated excellent accuracy. Then, we obtained data from the Human Protein Atlas (HPA) (https://www.proteinatlas.org/) to demonstrate the expression of PEBP1, ISCU, CYBB, and DUOX1 at the protein level in cervical and CESC tissues (Figure 5H-5O).
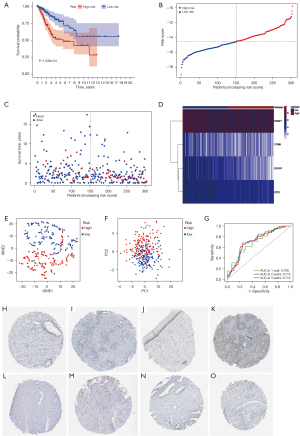
Independent factor analysis of the prognostic model and immune cell infiltration analysis
To analyze whether the model could predict prognosis independently, we firstly conducted a univariate Cox regression analysis. Results showed that T stage (HR: 3.897, 95% CI: 1.908–7.961, P<0.001), N stage (HR: 3.222, 95% CI: 1.685–6.162, P<0.001), and risk score (HR: 1.390, 95% CI: 1.142–1.692, P=0.001) were significantly associated with CESC prognosis (Figure 6A) and all three were identified as risk factors. Subsequently, we performed a multivariate Cox regression analysis, finding that risk score was an independent risk factor for CESC (HR: 1.404, 95% CI: 1.150–1.715, P<0.001). Although not as significant as the risk score, T stage (HR: 2.680, 95% CI: 1.264–5.683, P=0.010) and N stage (HR: 2.790, 95% CI: 1.424–5.465, P=0.003) were also identified as independent prognostic risk factors (Figure 6B). In addition, ssGSEA analyses were performed to show the differences in immune cells and related immune functions between the high and low risk groups. We found that aDCs (activated dendritic cells) (P<0.001), iDCs (interdigitating dendritic cells) (P<0.001), pDCs (plasmacytoid dendritic cells) (P<0.001), DCs (dendritic cells) (P<0.001), and APC (antigen presenting cells) co-inhibition (P<0.05), all of which are responsible for antigen processing and presentation, were significantly inhibited in the high-risk group (Figure 6C). Furthermore, excluding Th1 cells, Th2 cells and Treg, the other nine types of immune cells (B cells, CD8+ T cells, macrophages, mast cells, neutrophils, T helper cells, NK cells, Tfh, and TIL) in the low-risk group were significantly higher than those in the high-risk group (all P<0.05). In terms of immune function, all functions were inhibited in the high-risk group, among which 10 types showed significant differences, including APC co-inhibition, CCR, check-in point, cytolytic activity, HLA, inflammation-promoting, MHC class I, parainflammation, T cell co-inhibition, T cell co-stimulation, and Type I IFN response (Figure 6D).
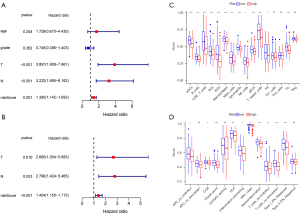
CYBB as a potential regulator of TME status
We divided the samples into high- and low-CYBB expression groups according to the CYBB expression level. Next, we used the HALLMARK and C7 gene set collections to perform GSEA on the samples to identify the functions that might be affected. We found when using HALLMARK in the high-CYBB expression group (Figure 7A) that genes were mainly enriched in immune-related processes, such as allograft rejection, apoptosis, complement, and interferon response (alpha and gamma). When C7 (related to the immune system) was used, 3,717 gene sets were identified as significant at FDR <25%, and 2,526 gene sets were significantly enriched at a nominal P<0.01 (Figure 7B). However, in the low-expression group using the two collections, it remained almost impossible to identify a statistically significant gene set.
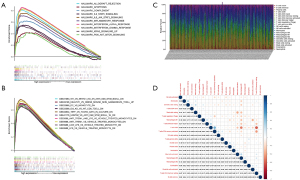
The relationship between CYBB and immune cell infiltration
To further demonstrate the correlation between CYBB expression levels and the immune microenvironment, the CIBERSORT algorithm was used to analyze the proportion of tumor-infiltrating cells in each sample and the correlation of 21 immune cells in the CESC samples (Figure 7C,7D). After conducting the difference and correlational analyses, 11 immune cells related to CYBB expression were obtained from the intersection. Among them, seven CYBB expression levels (T cells CD8, T cells CD4 memory activated, T cells gamma delta, macrophages M1, macrophages M2, dendritic cells resting, and mast cells resting) were positively correlated, and four (NK cells activated, dendritic cells activated, mast cells activated, and eosinophils) were negatively correlated (Figure 8A-8C). These results indicate that the expression level of CYBB can affect immune cells.
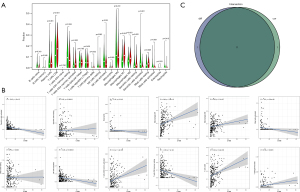
The CC algorithm used for CESC subtyping and the analysis of the differences in immune cell infiltration
The “CancerSubtypes” package in R was used for the cancer typing. The CC algorithm was adopted to build a typing model with K=2–10. The results identified that the model was most robust when the grouping number was limited to five (Figure 9A) and showed a significant difference in the survival of patients when divided into five groups (P=8.64E–05). Therefore, the results of the five-group comparisons were used in the final analysis. The five groups contained 68 patients, 50 patients, 121 patients, 35 patients, and 30 patients, respectively. To illustrate whether immune cell infiltration also differed among the five groups, an ssGSEA was conducted and heat maps were used to visualize the results of 16 immune cells and 13 immune-related biological processes (Figure 9B). The results showed that there were significant differences in immune cells and related biological processes among the five groups. The heat maps showed that the enrichment degree of almost all immune cells and immune-related biological processes was the highest in group 3, and lowest in groups 2 and 5, while that of the first and fourth groups was relatively low. Interestingly, DCs, iDCs, pDCs, aDCs, TIL, CD8+ T cells, and the related process T cell co-stimulation were found to be the most highly expressed in group 3, which also explained the better survival outcomes of patients in group 3 in the CESC sub-typing analysis (Figure 9C).
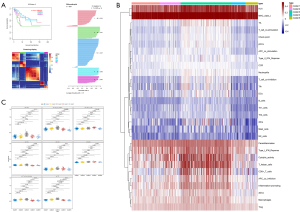
Discussion
In the present study, we used the TCGA database to identify and analyze relevant genes that could potentially play an important role in both the CESC TME and ferroptosis. After performing a series of bioinformatics analyses, we found that CYBB is an indicator of the TME status in CESC patients, which may be of great significance for future research.
It is well known that the TME plays an essential role in the occurrence and metastasis of tumors. Exploring the composition of the TME is extremely useful for finding new targets for tumor therapy. Our analysis of transcriptome data from CESC patients in the TCGA-CESC database suggests that the immune component of the TME contributes to CESC patients’ prognosis. In particular, the estimate score (the proportion of immune and stromal components in the TME) was significantly correlated with tumor development, indicating the importance of the interaction between cancer cells and immune cells. Meanwhile, we also found that ferroptosis is closely related to the TME in CESC. Ferroptosis, a form of RCD, is distinct from apoptosis, necrosis, pyroptosis, and autophagy (34). In addition, an increasing amount of evidence shows that ferroptosis is closely related to the occurrence, progression, and inhibition of cancer (35). However, the role of ferroptosis in patients with CESC is not well understood, especially the mechanism of the association and interaction between ferroptosis and the TME. In this study, we established a four-gene ferroptosis-associated model to predict the prognosis of patients with CESC, aim to illustrate the relationship between ferroptosis and the TME with CYBB.
CYBB encodes the β chain of cytochrome B. At present, only a few articles have focused on the role of CYBB in the immune microenvironment and ferroptosis. For instance, Long et al. suggested that the expression level of CYBB in lung adenocarcinoma was higher in normal tissues than in cancer tissues, and a high expression of CYBB predicted better patient survival (36). CYBB is also widely believed to be associated with chronic granulomatous disease (37). The phagocytic NADPH oxidase consists of five subunits, of which the enzyme component is GP91-phox, or NOX2, encoded by the CYBB gene on the X chromosome (38,39). Moreover, when NOX2 is assembled on the phagolysosome, it produces intracellular ROS, and assembly on the plasma membrane leads to extracellular ROS formation (40). As we know, ROS plays an important role in the ferroptosis mechanism (15) insofar as ROS leads to lipid peroxidation, which in turn results in ferroptosis (41). Interestingly, the presence of ROS in myeloid-derived suppressor cells (MDSCs) may have undesirable consequences. In addition, studies have shown that cancer-associated fibroblasts can induce a high expression of CYBB in MDSC to produce ROS and inhibit T cell proliferation (42). Furthermore, NOX2 composed of CYBB regulates adaptive immunity in several ways, including antigen presentation and cross signaling between T cells and B cells. NOX2 is recruited to the early phagosomes of dendritic cells and ensures the expression of ROS, otherwise the over-acidified phagosomes lead to excessive antigen degradation, resulting in impaired crosspresentation (43-45).
Using the HALLMARK and C7 gene set collections to perform GSEA to analyze CYBB, we found that genes mainly enriched in immune-related processes. We also performed an immune infiltration analysis of CYBB, and our results are worthy of attention. An increase in cytotoxic T lymphocyte (expressing CD8) and helper T cells (expressing CD4) that were positively correlated with CYBB expression was associated with improved prognosis (7). As CYBB is an important part of NADPH oxidase, the positive correlation between CYBB and subtypes M1-like and M2-like macrophages can be explained to a certain extent. Phenotype M1 is pro-inflammatory and anti-tumorigenesis, while phenotype M2 is anti-inflammatory and pro-tumorigenesis (46). Furthermore, increased infiltration of M1 macrophages is a favorable prognostic factor for survival of HPV-related CC patients (6). In summary, the role of CYBB in the TME is more subtle and complex than in ferroptosis. Our data analysis shows that CYBB is a low-risk gene, and its high expression in tumors indicates better patient survival.
Three other genes, DUOX1, PEBP1, and ISCU, were included in the prognostic model established in this study. Similar to CYBB, DUOX1, a type of NADPH oxidase, also regulates ROS (47). Studies have revealed that DUOX1 has the potential to become a key factor in the prognosis and treatment of liver cancer, and high levels of DUOX1 contribute to a better prognosis for liver cancer patients (48). In addition, a previous study demonstrated that DUOX1 is downregulated in BC and seems to play a role in breast carcinogenesis (49), and another recent study demonstrated that a high expression of DUOX1 is beneficial for CESC patients’ survival (50). These findings suggest that using DUOX1 knockout animal models will be important in the further exploration of the mechanism of tumor inhibition or promotion. PEBP1 is considered a metastasis suppressor gene (51), and its decreased expression is often associated with cancer invasion and metastasis (52). PEBP1 also forms complexes with 15-Lipoxygenases (15-LO), resulting in the production of hydroperoxy-PE, which ultimately leads to ferroptosis (53). The expression of ISCU has been reported to help regulate iron metabolism (54). ISCU has also been identified as a low-risk FRG in another study on papillary thyroid carcinoma (55).
ISCU plays a role in protecting tumor cells from ferroptosis, while the other three genes (CYBB, DUOX1, and PEBP1) promote ferroptosis. Our results demonstrated that all four genes were positively correlated with the prognosis of CESC patients. However, the effect of these genes on patient survival needs to be further verified. For example, the complex roles of ROS regulated by DUOX1 and CYBB in the TME have not been fully elucidated.
What also interested us was the obvious difference in DCs between the two risk groups in the ferroptosis prognostic model, indicating that there were significant differences in antigen processing and presentation between them. Furthermore, patients in the low-risk group also had a higher percentage of B cells, T helper cells, CD8+ T cells, and NK cells than those in the high-risk group, which is consistent with their ability to kill tumor cells (56,57). Additionally, activation of HLA, T cell co-stimulation, and Type I IFN response processes in the low-risk group also explains the more favorable prognosis in low-risk CESC patients.
Although we have identified many important findings, the present study has several limitations. All the prognostic model information in this paper came from TCGA database, while other data validation was lacking. TCGA database is limited by numerous factors, such as region, race, and sample size. Although it is representative to some extent, it is not comprehensive. As a result, the prognostic model and the hypothesis proposed in this paper need to be verified in future experiments.
Conclusions
The expression profiles of FRGs are closely related to the TME and the prognosis of CESC patients. The interaction between ferroptosis and immunity in the development of CC provides a new insight into the molecular mechanisms underlying this disease.
Acknowledgments
Funding: This research was funded by the Fundamental Research Funds for the Central Universities [grant number: DUT19RC (3) 076], the National Natural Science Foundation of China (grant number: 81600370), and the China Postdoctoral Science Foundation (grant number: 2018M640270) to Yanshuo Han; also, by the Natural Science Foundation of Liaoning Province (grant number: 2021-YGJC-15) to Zhuo Yang.
Footnote
Provenance and Peer Review: This article was commissioned by the Guest Editor (Danbo Wang) for the series “New Progress and Challenge in Gynecological Cancer” published in Annals of Translational Medicine. The article has undergone external peer review.
Reporting Checklist: The authors have completed the REMARK reporting checklist. Available at https://atm.amegroups.com/article/view/10.21037/atm-21-6265/rc
Conflicts of Interest: All authors have completed the ICMJE uniform disclosure form (available at https://atm.amegroups.com/article/view/10.21037/atm-21-6265/coif). The series “New Progress and Challenge in Gynecological Cancer” was commissioned by the editorial office without any funding or sponsorship. The authors have no other conflicts of interest to declare.
Ethical Statement: The authors are accountable for all aspects of the work in ensuring that questions related to the accuracy or integrity of any part of the work are appropriately investigated and resolved. The study was conducted in accordance with the Declaration of Helsinki (as revised in 2013).
Open Access Statement: This is an Open Access article distributed in accordance with the Creative Commons Attribution-NonCommercial-NoDerivs 4.0 International License (CC BY-NC-ND 4.0), which permits the non-commercial replication and distribution of the article with the strict proviso that no changes or edits are made and the original work is properly cited (including links to both the formal publication through the relevant DOI and the license). See: https://creativecommons.org/licenses/by-nc-nd/4.0/.
References
- Vu M, Yu J, Awolude OA, et al. Cervical cancer worldwide. Curr Probl Cancer 2018;42:457-65. [Crossref] [PubMed]
- Ferlay J, Colombet M, Soerjomataram I, et al. Estimating the global cancer incidence and mortality in 2018: GLOBOCAN sources and methods. Int J Cancer 2019;144:1941-53. [Crossref] [PubMed]
- Ojesina AI, Lichtenstein L, Freeman SS, et al. Landscape of genomic alterations in cervical carcinomas. Nature 2014;506:371-5. [Crossref] [PubMed]
- Kim SW, Chun M, Ryu HS, et al. Salvage radiotherapy with or without concurrent chemotherapy for pelvic recurrence after hysterectomy alone for early-stage uterine cervical cancer. Strahlenther Onkol 2017;193:534-42. [Crossref] [PubMed]
- Fuller CD, Wang SJ, Thomas CR Jr, et al. Conditional survival in head and neck squamous cell carcinoma: results from the SEER dataset 1973-1998. Cancer 2007;109:1331-43. [Crossref] [PubMed]
- de Vos van Steenwijk PJ, Ramwadhdoebe TH, Goedemans R, et al. Tumor-infiltrating CD14-positive myeloid cells and CD8-positive T-cells prolong survival in patients with cervical carcinoma. Int J Cancer 2013;133:2884-94. [Crossref] [PubMed]
- Litwin TR, Irvin SR, Chornock RL, et al. Infiltrating T-cell markers in cervical carcinogenesis: a systematic review and meta-analysis. Br J Cancer 2021;124:831-41. [Crossref] [PubMed]
- Monnier-Benoit S, Mauny F, Riethmuller D, et al. Immunohistochemical analysis of CD4+ and CD8+ T-cell subsets in high risk human papillomavirus-associated pre-malignant and malignant lesions of the uterine cervix. Gynecol Oncol 2006;102:22-31. [Crossref] [PubMed]
- Mittal V, El Rayes T, Narula N, et al. The Microenvironment of Lung Cancer and Therapeutic Implications. Adv Exp Med Biol 2016;890:75-110. [Crossref] [PubMed]
- Quail DF, Joyce JA. Microenvironmental regulation of tumor progression and metastasis. Nat Med 2013;19:1423-37. [Crossref] [PubMed]
- Dzobo K. Taking a Full Snapshot of Cancer Biology: Deciphering the Tumor Microenvironment for Effective Cancer Therapy in the Oncology Clinic. OMICS 2020;24:175-9. [Crossref] [PubMed]
- Teng MW, Galon J, Fridman WH, et al. From mice to humans: developments in cancer immunoediting. J Clin Invest 2015;125:3338-46. [Crossref] [PubMed]
- Radogna F, Dicato M, Diederich M. Cancer-type-specific crosstalk between autophagy, necroptosis and apoptosis as a pharmacological target. Biochem Pharmacol 2015;94:1-11. [Crossref] [PubMed]
- Cao JY, Dixon SJ. Mechanisms of ferroptosis. Cell Mol Life Sci 2016;73:2195-209. [Crossref] [PubMed]
- Su Y, Zhao B, Zhou L, et al. Ferroptosis, a novel pharmacological mechanism of anti-cancer drugs. Cancer Lett 2020;483:127-36. [Crossref] [PubMed]
- Hassannia B, Vandenabeele P, Vanden Berghe T. Targeting Ferroptosis to Iron Out Cancer. Cancer Cell 2019;35:830-49. [Crossref] [PubMed]
- Dong S, Li X, Jiang W, et al. Current understanding of ferroptosis in the progression and treatment of pancreatic cancer. Cancer Cell Int 2021;21:480. [Crossref] [PubMed]
- Basuli D, Tesfay L, Deng Z, et al. Iron addiction: a novel therapeutic target in ovarian cancer. Oncogene 2017;36:4089-99. [Crossref] [PubMed]
- Je EM, An CH, Yoo NJ, et al. Mutational and expressional analyses of NRF2 and KEAP1 in sarcomas. Tumori 2012;98:510-5. [Crossref] [PubMed]
- Ma S, Henson ES, Chen Y, et al. Ferroptosis is induced following siramesine and lapatinib treatment of breast cancer cells. Cell Death Dis 2016;7:e2307. [Crossref] [PubMed]
- Hangauer MJ, Viswanathan VS, Ryan MJ, et al. Drug-tolerant persister cancer cells are vulnerable to GPX4 inhibition. Nature 2017;551:247-50. [Crossref] [PubMed]
- Liang JY, Wang DS, Lin HC, et al. A Novel Ferroptosis-related Gene Signature for Overall Survival Prediction in Patients with Hepatocellular Carcinoma. Int J Biol Sci 2020;16:2430-41. [Crossref] [PubMed]
- Zhu L, Tian Q, Jiang S, et al. A Novel Ferroptosis-Related Gene Signature for Overall Survival Prediction in Patients With Breast Cancer. Front Cell Dev Biol 2021;9:670184. [Crossref] [PubMed]
- Liu HJ, Hu HM, Li GZ, et al. Ferroptosis-Related Gene Signature Predicts Glioma Cell Death and Glioma Patient Progression. Front Cell Dev Biol 2020;8:538. [Crossref] [PubMed]
- Binnewies M, Roberts EW, Kersten K, et al. Understanding the tumor immune microenvironment (TIME) for effective therapy. Nat Med 2018;24:541-50. [Crossref] [PubMed]
- Dai E, Han L, Liu J, et al. Autophagy-dependent ferroptosis drives tumor-associated macrophage polarization via release and uptake of oncogenic KRAS protein. Autophagy 2020;16:2069-83. [Crossref] [PubMed]
- Zou Y, Palte MJ, Deik AA, et al. A GPX4-dependent cancer cell state underlies the clear-cell morphology and confers sensitivity to ferroptosis. Nat Commun 2019;10:1617. [Crossref] [PubMed]
- FIGO Committee on Gynecologic Oncology. FIGO staging for carcinoma of the vulva, cervix, and corpus uteri. Int J Gynaecol Obstet 2014;125:97-8. [Crossref] [PubMed]
- Pecorelli S. Revised FIGO staging for carcinoma of the vulva, cervix, and endometrium. Int J Gynaecol Obstet 2009;105:103-4. [Crossref] [PubMed]
- Wright JD, Matsuo K, Huang Y, et al. Prognostic Performance of the 2018 International Federation of Gynecology and Obstetrics Cervical Cancer Staging Guidelines. Obstet Gynecol 2019;134:49-57. [Crossref] [PubMed]
- Xu T, Le TD, Liu L, et al. CancerSubtypes: an R/Bioconductor package for molecular cancer subtype identification, validation and visualization. Bioinformatics 2017;33:3131-3. [Crossref] [PubMed]
- Zhao L, Zhang J, Liu Z, et al. Comprehensive Characterization of Alternative mRNA Splicing Events in Glioblastoma: Implications for Prognosis, Molecular Subtypes, and Immune Microenvironment Remodeling. Front Oncol 2021;10:555632. [Crossref] [PubMed]
- Monti S, Tamayo P, Mesirov J, et al. Consensus Clustering: A Resampling-Based Method for Class Discovery and Visualization of Gene Expression Microarray Data. Machine Learning 2003;52:91-118. [Crossref]
- Galluzzi L, Vitale I, Aaronson SA, et al. Molecular mechanisms of cell death: recommendations of the Nomenclature Committee on Cell Death 2018. Cell Death Differ 2018;25:486-541. [Crossref] [PubMed]
- Wang Y, Wei Z, Pan K, et al. The function and mechanism of ferroptosis in cancer. Apoptosis 2020;25:786-98. [Crossref] [PubMed]
- Long W, Li Q, Zhang J, et al. Identification of key genes in the tumor microenvironment of lung adenocarcinoma. Med Oncol 2021;38:83. [Crossref] [PubMed]
- Stasia MJ, Cathebras P, Lutz MF, et al. Chronic-granulomatous disease. Rev Med Interne 2009;30:221-32. [Crossref] [PubMed]
- Roos D, Kuhns DB, Maddalena A, et al. Hematologically important mutations: X-linked chronic granulomatous disease (third update). Blood Cells Mol Dis 2010;45:246-65. [Crossref] [PubMed]
- Roos D, van Leeuwen K, Hsu AP, et al. Hematologically important mutations: X-linked chronic granulomatous disease (fourth update). Blood Cells Mol Dis 2021;90:102587. [Crossref] [PubMed]
- Panday A, Sahoo MK, Osorio D, et al. NADPH oxidases: an overview from structure to innate immunity-associated pathologies. Cell Mol Immunol 2015;12:5-23. [Crossref] [PubMed]
- Latunde-Dada GO. Ferroptosis: Role of lipid peroxidation, iron and ferritinophagy. Biochim Biophys Acta Gen Subj 2017;1861:1893-900. [Crossref] [PubMed]
- Xiang H, Ramil CP, Hai J, et al. Cancer-Associated Fibroblasts Promote Immunosuppression by Inducing ROS-Generating Monocytic MDSCs in Lung Squamous Cell Carcinoma. Cancer Immunol Res 2020;8:436-50. [Crossref] [PubMed]
- Savina A, Jancic C, Hugues S, et al. NOX2 controls phagosomal pH to regulate antigen processing during crosspresentation by dendritic cells. Cell 2006;126:205-18. [Crossref] [PubMed]
- Jancic C, Savina A, Wasmeier C, et al. Rab27a regulates phagosomal pH and NADPH oxidase recruitment to dendritic cell phagosomes. Nat Cell Biol 2007;9:367-78. [Crossref] [PubMed]
- Graham DB, Stephenson LM, Lam SK, et al. An ITAM-signaling pathway controls cross-presentation of particulate but not soluble antigens in dendritic cells. J Exp Med 2007;204:2889-97. [Crossref] [PubMed]
- Jayshree RS. The Immune Microenvironment in Human Papilloma Virus-Induced Cervical Lesions-Evidence for Estrogen as an Immunomodulator. Front Cell Infect Microbiol 2021;11:649815. [Crossref] [PubMed]
- Ashtiwi NM, Sarr D, Rada B. DUOX1 in mammalian disease pathophysiology. J Mol Med (Berl) 2021;99:743-54. [Crossref] [PubMed]
- Ostrakhovitch EA, Li SS. NIP1/DUOXA1 expression in epithelial breast cancer cells: regulation of cell adhesion and actin dynamics. Breast Cancer Res Treat 2010;119:773-86. [Crossref] [PubMed]
- Fortunato RS, Gomes LR, Munford V, et al. DUOX1 Silencing in Mammary Cell Alters the Response to Genotoxic Stress. Oxid Med Cell Longev 2018;2018:3570526. [Crossref] [PubMed]
- Cho SY, Kim S, Son MJ, et al. Dual oxidase 1 and NADPH oxidase 2 exert favorable effects in cervical cancer patients by activating immune response. BMC Cancer 2019;19:1078. [Crossref] [PubMed]
- Martinho O, Simões K, Longatto-Filho A, et al. Absence of RKIP expression is an independent prognostic biomarker for gastric cancer patients. Oncol Rep 2013;29:690-6. [Crossref] [PubMed]
- Yun J, Frankenberger CA, Kuo WL, et al. Signalling pathway for RKIP and Let-7 regulates and predicts metastatic breast cancer. EMBO J 2011;30:4500-14. [Crossref] [PubMed]
- Wenzel SE, Tyurina YY, Zhao J, et al. PEBP1 Wardens Ferroptosis by Enabling Lipoxygenase Generation of Lipid Death Signals. Cell 2017;171:628-641.e26. [Crossref] [PubMed]
- Du J, Wang T, Li Y, et al. DHA inhibits proliferation and induces ferroptosis of leukemia cells through autophagy dependent degradation of ferritin. Free Radic Biol Med 2019;131:356-69. [Crossref] [PubMed]
- Yang D, Wang J, Li C, et al. Ferroptosis-related gene model to predict overall survival of papillary thyroid carcinoma. Am J Otolaryngol 2021;42:103163. [Crossref] [PubMed]
- Knutson KL, Disis ML. Tumor antigen-specific T helper cells in cancer immunity and immunotherapy. Cancer Immunol Immunother 2005;54:721-8. [Crossref] [PubMed]
- Kennedy R, Celis E. Multiple roles for CD4+ T cells in anti-tumor immune responses. Immunol Rev 2008;222:129-44. [Crossref] [PubMed]
(English Language Editor: D. Fitzgerald)