A deep convolutional neural network-based method for laryngeal squamous cell carcinoma diagnosis
Introduction
Laryngeal cancer (LC) is one of the most common cancers of the respiratory tract. In 2020, there were an estimated 184,404 new LC cases and 99,840 LC deaths worldwide, which accounted for 1.0% of all new cancer cases and 1.0% of all cancer deaths worldwide (1). In eastern Asia, there were an estimated 36,851 new LC cases and 17798 LC deaths in 2021 (1). More than 98% of LC cases are laryngeal squamous cell carcinomas (LSCCs), which is the second most common type of head and neck squamous cell carcinoma. The clinical outcomes vary and are dependent on the cancer stage. Disease-specific survival ranges from 47.6% (T4 supraglottic LSCC) to 98.1% (T1a glottic LSCC), and disease-free survival (DFS) ranges from 23.0% (T3 glottic LSCC) to 69.1% (T1a glottic LSCC) according to the T-stages (2-4). As DFS is not favorable for patients diagnosed with LSCC, the early detection and accurate diagnosis of the cancer is critical to achieve the best clinical outcomes.
To diagnose LSCC, clinicians check the throat with laryngoscope or endoscopy. Narrow-band imaging (NBI) endoscopy is widely used to diagnose variable laryngeal diseases (5). NBI is based on the depth of light penetration, which depends on the wavelength—the longer the wavelength, the deeper the penetration. The NBI endoscope uses a filter to modify the wide-band white light emitted by the endoscope xenon light source (6). A blue-and-green light source with a wavelength of 415 and 541 nm can only penetrate the mucosa and submucosa. A narrow band of blue light (41.5 nm) will show the superficial capillary vessel network, and a narrow band of green light (540 nm) (7-9) will show the subcutaneous vessel. When the 2 wavelengths are combined, the blood vessels on the tissue surface can be observed. Many studies have confirmed the value of NBI endoscopy in the diagnosis of laryngeal diseases (10-14). It is especially superior to fibrolaryngoscope, stroboscope, and other examination methods in the diagnosis of benign and malignant lesions. Laryngeal lesions may exhibit different features of an intraepithelial papillary capillary loop (IPCL) under a NBI laryngoscope. Ni et al. compared the sensitivity and specificity of white-light and NBI imaging (sensitivity: 68.9% vs. 88.9%; specificity: 89.8% vs. 93.2%) (15). NBI has obvious advantages in the early diagnosis of laryngeal malignant diseases and in guiding biopsy sites; however, lengthy training and extensive experience is required to acquire the skill necessary to review NBI scans. Indeed, this is not a skill that can be acquired in a short time (9).
If recommended by a clinician, a biopsy will be taken and viewed under a microscope by a pathologist for a definitive diagnosis. This process is also dependent on the pathologists’ expertise and human cognition of histological texture and morphological features (16). Pathologists need to consider numerous features and organelles, such as special cells, the shape of the nucleus, mitosis, the cytoplasm, the distance between cells, nucleolus patterns, and the epithelium (16). An immediate and accurate diagnosis is needed during surgery; however, it can take a long time for pathologists to identify specific features to make the right decision. In addition, to gain expertise, pathologists require long-term training, and in most parts of world, skilled pathologists are lacking (17). According to the data in this study, the CNN model is comparable to clinical experts in terms of its accuracy, sensitivity, and specificity. Further, the CNN model can acquire the ability to make such diagnoses in a much shorter time than clinical experts. Thus, this model may help with the diagnosis of LSCC in the future (17).
Deep learning refers to a machine learning method that is based on a neural network model with multiple levels of data representation. Convolutional neural networks (CNNs) are feedforward neural networks with deep structure and convolution calculation (18). It is a model that can addresses classification and detection problems. Compared with conventional image processing algorithms, CNN has high potential for feature extraction and analysis (19). Recently, artificial intelligence (AI) based on deep CNNs has been applied to skin cancer classification, congenital cataracts, diabetic retinopathy diagnosis, magnetic resonance imaging (MRI), and pathology (20-24). By applying these cutting-edge deep-learning techniques, AI systems can immediately provide accurate diagnoses based on image data, which could potentially contribute to the earlier detection of diseases and increase patients’ survival rates (25). Our previous single center study preliminarily verified the effectiveness of a CNN model in the laryngeal NBI image diagnosis (26). In this study, we also applied CNN technology to interpret pathology images, we trained, validated, and tested the CNN model using NBI scans and pathology images of LSCC from multiple centers, so as to further verify the application value of AI technology in the diagnosis of LSCC.
We present the following article in accordance with the STARD reporting checklist (available at https://dx.doi.org/10.21037/atm-21-6458).
Methods
Study type
A retrospective, multicenter, experimental study was conducted.
Patient characteristics
To diagnose LSCC, clinicians generally check the throat using NBI technology. If necessary, a biopsy is performed before or during the surgery to confirm the diagnosis (see Figure 1A). To test the potential of a deep-learning algorithm on LSCC diagnosis, we collected 4,591 NBI scans of 4,591 patients at the Department of Otolaryngology Head and Neck Surgery at Beijing Tongren Hospital. All these NBI scans can show the laryngeal lesions clearly, the scans showing incomplete lesions due to the patient's inability to cooperate with the examination have been excluded. Patients’ ages ranged from 21 to 87 years old (mean ± standard deviation: 59.36±10.25 years). Among them, 2,664 of the patients had already been diagnosed with LSCC, and 1,927 laryngeal lesions had already been diagnosed as benign based on the pathology results. An independent testing cohort (287 benign laryngeal lesions and 235 LSCC NBI scans) from The Affiliated Hospital of Southwest Medical University and The First Hospital of China Medical University was used to test the performance of this algorithm. They used the same NBI endoscopy system as Beijing Tongren Hospital.
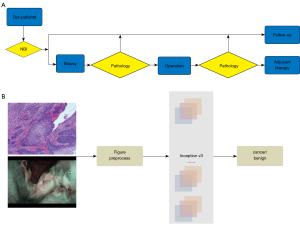
NBI scan data set
A retrospective study of 4,591 patients’ NBI scans was carried out at the Department of Otolaryngology Head and Neck Surgery at the Beijing Tongren Hospital from January 2016 to December 2017. All the patients underwent NBI endoscopy, and had their diagnoses confirmed pathologically. Data for the independent testing cohort was collected from Beijing Tongren Hospital, Southwest Medical University and The First Hospital of China Medical University (287 benign laryngeal lesion and 235 LSCC NBI scans). The medical procedure used at each of the centers was approved by the Beijing Tongren Hospital Review Board.
Endoscopy (Olympus CV-170 for NBI and Olympus CLV-S40Pro for white light, Olympus Medical Systems Corp., Tokyo, Japan) was used to evaluate patients’ laryngeal lesions in both white-light and NBI modes. First, the entire larynx was observed with standard white light, and the capillaries on the mucosal surface were then observed in NBI mode. The anatomical locations of patients’ laryngeal lesions were documented as follows: epiglottis, ventricular folds, vocal folds, arytenoids, aryepiglottic folds, subglottic region, anterior and the posterior commissure. Representative images were recorded for analysis. According to Ni’s study (15), endoscopic NBI findings can be classified into 5 types (I to V) based on the features of the mucosal IPCLs. Based on the characteristics of the NBI findings, we divided the patients into 2 groups: (I) the benign laryngeal lesion group (IPCL I–III); and (II) the LSCC group (IPCL IV–V).
Training and validation of the pathology image data set
A total of 3,458 pathological images were taken from 1,228 patients’ hematoxylin and eosin (H&E) staining biopsy slides (318 benign patients and 910 LSCC patients). These pathological images were collected from the Beijing Tongren Hospital and Southwest Medical University. To test the ability and reliability of the CNN model, we took different magnification images, including ×20 (288 images), ×40 (742 images), ×100 (845 images) and ×200 (1,583 images). The H&E-stained sections were reviewed by 2 pathologists to confirm the diagnoses, the patient information was treated as confidential by all the specialists involved.
Development of the CNN algorithm
InceptionV3 (27) was developed by Google and inherited from the GoogLeNet model (28), which was the state-of-the-art image recognition net in 2014. It has shown the performance of human experts on medical image recognition (20,21). Thus, we selected Inception V3 (27) as our prediction model. The Inception v3 network, which was trained using the ImageNet 2012 Challenge training data set, was first downloaded (https://github.com/tensorflow/models/tree/master/research/inception). The parameters of data augmentation were adopted from the literature (29,30). All the experiments were based on Tensorflow 1.6, and trained/tested on an Ubuntu 16.04 desktop system with Intel Core i7-6700k CPU and a NVIDIA GXT 1,080 8Gb GPU. We conducted 2 experiments to evaluate the performance of our CNN algorithm on NBI scans and pathological images. First, 4,591 patients’ NBI scans (1,927 benign and 2,664 LSCC NBI scans) were randomly divided into training, validation and test data sets at a ratio of 70:15:15. Second, we fine-tuned the model based on the pathological images. The training, validation, and testing process for the pathological images was the same as that for the NBI scans. The pathological images were also randomly divided into 3 data sets at the same ratio.
Statistical analysis
The area under the curve (AUC) of receiver operating curve (ROC) was used to evaluate the effectiveness of our CNN algorithm (29,30). A ROC is a comprehensive indicator that reflects the sensitivity and specificity of continuous variables. The curve is drawn with the sensitivity as the ordinate and the specificity as the abscissa. The larger the AUC, the higher the diagnostic accuracy.
Performance comparison of the CNN model and human specialists in relation to the NBI scans
A validation data set of the NBI scans (1,276 benign and 1,096 LSCC NBI scans) was used to compare the performance of the CNN model and the human specialists. Four human specialists with around 10 years’ clinical experience were invited to review our validation data cohorts. The patient information was treated as confidential by all the specialists involved.
Ethics
All procedures performed in this study involving human participants were in accordance with the Declaration of Helsinki (as revised in 2013). The study was approved by ethics committee of Beijing Tongren Hospital, Capital Medical University (No. TRECKY2014-027). Individual consent for this retrospective analysis was waived.
Results
Performance of CNN
Our algorithm was trained to determine whether a lesion was benign or malignant based on the NBI scans and pathology images (see Figure 1B). 70% of the benign and LSCC NBI scans were used in the training data set (see Figure 2A). A transfer learning strategy was adopted to ensure the performance of the CNN model. The trained CNN algorithm was further used for our validation set, which comprised 15% of the benign and LSCC NBI scans. The remaining 15% of the scans were used as the testing cohort. ROCs were then used to evaluate the model’s ability to distinguish between benign and malignant laryngeal NBI scans. The AUC of the ROC of the validation cohort was 0.966 (see Figure 2B). The AUC of the ROC of the testing cohort was 0.964 (see Figure 2C). There was also an independent cohort with images from 3 different centers. With an AUC of 0.873, the performance of the algorithm was still very high (see Figure 2D).
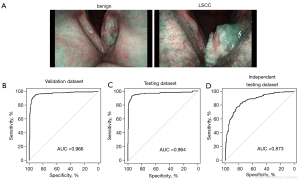
Comparison between CNN and human specialists
To compare the accuracy between the CNN model and the human specialists, the validation cohort images were further independently reviewed by 4 specially invited specialists. The average rates of accuracy, sensitivity, and specificity were 90.6%, 88.8%, and 92.2% respectively (see Figure 3A). The algorithm had a diagnosis time of only 0.01 s for each image. Conversely, human specialists with >10 years’ experience took 5.5 s (on average) to interpret each image. The CNN model had a 45% accuracy rate for the 20 NBI scans misdiagnosed by specialists, which also shows that the model performed better than the experts (see Figure 3B).
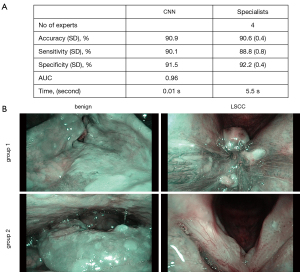
Application of the CNN model to LSCC pathology images
Based on the NBI scans, patients were recommended for biopsy for further diagnosis as necessary. A pathological diagnosis is the gold standard for cancer diagnosis and should accurately guide future treatment. Thus, pathologists are required to have extensive experience and undertake lengthy clinical training. To further investigate the use of the CNN model on LSCC pathology images in making fast and accurate diagnoses, we collected 3,458 pathology images (hematoxylin and eosin) from 1,228 patients (see Figure 4A) with different magnifications (×20, ×40, ×100, and ×200). The pathology images were randomly divided into a training data set, a validation data set, and a testing data set (ratio: 70:15:15). First, we trained our CNN model with a training cohort as described in the methods section above. A validation and testing cohort was further used with the trained pathology CNN algorithm. The AUC was 0.994 for the validation data set (see Figure 4B), and 0.981 for the testing data set (see Figure 4C). We also validated our algorithm with another independent data set from The Affiliated Hospital of Southwest Medical University and The First Hospital of China Medical University, and the performance of our algorithm remained high (AUC =0.982; see Figure 4D). Our trained pathology algorithm demonstrated a high performance for pathological diagnoses, even of pathology images of different magnifications.
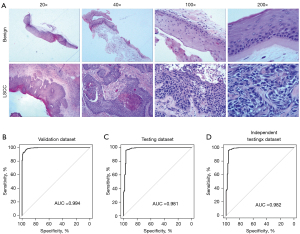
Discussion
At present, AI has been used for repetitive tasks in medical practice. Deep learning based on CNN can automatically extract important feature from data set, and its performance is more systematic and easier to adjust, many studies have found its considerable effect in lesion segmentation and classification. Esteva et al. demonstrated that AI based on CNN can be used to classify skin cancer with a level of competence comparable to that of dermatologists (20). Ting et al. also showed that a deep-learning algorithm had high sensitivity and specificity at detecting referable diabetic retinopathy (21). Chang et al. developed a deep-learning technique based on residual CNN to non-invasively predict the IDH genotype in grade IIIV– glioma using conventional MRI in a multi-institutional data set (31). Xi et al. showed that deep learning can distinguish between benign and malignant renal lesions based on routine MRI scans (23). A study also reported that some deep-learning algorithms achieved better diagnostic performance at detecting metastases in H&E-stained tissue sections of the lymph nodes of women with breast cancers than a panel of 11 pathologists participating in a simulation exercise designed to mimic routine pathology workflow (32).
The accurate and fast diagnosis for LSCC is crucial for clinicians to make treatment decisions, which improve patients’ survival rates and reduce medical costs. The diagnostic process for LSCC includes a physical examination, laryngoscopy, videostroboscopy, biopsy, and the molecular testing of the tumor, or even computed tomography (CT) and MRI. If necessary, a positron emission tomography-CT scan is used to determine if and how far the cancer has spread. In this process, numerous images are taken and reviewed by clinicians, pathologists, and radiologists. This repetitive work is tedious, but requires extensive experience. This is exactly where AI can help. Recently, AI has been applied to diagnosis using white-light laryngoscopy (29,30).
In this study, the CNN model showed high performance in the diagnosis of LSCC using an NBI image data set. NBI has been widely used for LSCC detection because the use of white-light imaging is limited when it comes to detecting small lesions <1 cm, especially in the setting of recurrent disease (33,34). Pathology diagnoses differs to diagnoses based on NBI scans, as pathologists need to be able to identify histological texture and morphological features (16). Notably, our trained algorithm also showed high accuracy in pathological diagnoses, even when assessing pathology images of different magnifications. The algorithm was validated with images from different centers. Our data revealed the potential wide application of this CNN model in relation to medical and pathological images (35).
In this study, our algorithm had several advantages over the human experts. First, the average review time for the CNN model was much shorter than that of the human experts. Second, the accuracy of the CNN model was higher than that of the human experts. Third, as the cut-off value CNN algorithm is adjustable, different cut-off values can be chosen to achieve higher sensitivity or specificity based on clinical demands. At this stage, we only verified the effectiveness of the CNN model in static NBI scans and pathology diagnosis, we are interested in whether this algorithm could recognize more features related to clinical outcomes or molecular genotypes (31,36). We intend to train this algorithm with more NBI scans to increase its accuracy, explore whether it could be used to make real-time diagnoses based on live-images during endoscopic examinations, and further explore whether more LSCC clinical data and biological sample database information can be integrated to provide wise decision support for precision head and neck medicine. CNN-based AI method has a broad research space and has a better clinical application prospect in head and neck medicine.
Conclusions
In conclusion, we found that that diagnostic ability of the CNN model was comparable to that of experienced specialists and pathologists. Our models for NBI and pathology images have clinical applications and could potentially assist in the fast and accurate diagnosis of LSCC.
Acknowledgments
Funding: None.
Footnote
Reporting Checklist: The authors have completed the STARD reporting checklist. Available at https://dx.doi.org/10.21037/atm-21-6458
Data Sharing Statement: Available at https://dx.doi.org/10.21037/atm-21-6458
Conflicts of Interest: All authors have completed the ICMJE uniform disclosure form (available at https://dx.doi.org/10.21037/atm-21-6458). The authors have no conflicts of interest to declare.
Ethical Statement: The authors are accountable for all aspects of the work in ensuring that questions related to the accuracy or integrity of any part of the work are appropriately investigated and resolved. All procedures performed in this study involving human participants were in accordance with the Declaration of Helsinki (as revised in 2013). The study was approved by ethics committee of Beijing Tongren Hospital, Capital Medical University (No. TRECKY2014-027). Individual consent for this retrospective analysis was waived.
Open Access Statement: This is an Open Access article distributed in accordance with the Creative Commons Attribution-NonCommercial-NoDerivs 4.0 International License (CC BY-NC-ND 4.0), which permits the non-commercial replication and distribution of the article with the strict proviso that no changes or edits are made and the original work is properly cited (including links to both the formal publication through the relevant DOI and the license). See: https://creativecommons.org/licenses/by-nc-nd/4.0/.
References
- Sung H, Ferlay J, Siegel RL, et al. Global Cancer Statistics 2020: GLOBOCAN Estimates of Incidence and Mortality Worldwide for 36 Cancers in 185 Countries. CA Cancer J Clin 2021;71:209-49. [Crossref] [PubMed]
- Brandstorp-Boesen J, Sørum Falk R, Boysen M, et al. Impact of stage, management and recurrence on survival rates in laryngeal cancer. PLoS One 2017;12:e0179371. [Crossref] [PubMed]
- Timmermans AJ, van Dijk BA, Overbeek LI, et al. Trends in treatment and survival for advanced laryngeal cancer: A 20-year population-based study in The Netherlands. Head Neck 2016;38:E1247-55. [Crossref] [PubMed]
- Zhang H, Sheng X, Zhang S, et al. The prognostic value of tumor budding in laryngeal squamous cell carcinoma. Transl Cancer Res 2020;9:119-27. [Crossref]
- Kraft M, Fostiropoulos K, Gürtler N, et al. Value of narrow band imaging in the early diagnosis of laryngeal cancer. Head Neck 2016;38:15-20. [Crossref] [PubMed]
- Gono K, Obi T, Yamaguchi M, et al. Appearance of enhanced tissue features in narrow-band endoscopic imaging. J Biomed Opt 2004;9:568-77. [Crossref] [PubMed]
- Larghi A, Lecca PG, Costamagna G. High-resolution narrow band imaging endoscopy. Gut 2008;57:976-86. [Crossref] [PubMed]
- Piazza C, Dessouky O, Peretti G, et al. Narrow-band imaging: a new tool for evaluation of head and neck squamous cell carcinomas. Review of the literature. Acta Otorhinolaryngol Ital 2008;28:49-54. [PubMed]
- Uedo N, Ishihara R, Iishi H, et al. A new method of diagnosing gastric intestinal metaplasia: narrow-band imaging with magnifying endoscopy. Endoscopy 2006;38:819-24. [Crossref] [PubMed]
- Staníková L, Šatanková J, Kučová H, et al. The role of narrow-band imaging (NBI) endoscopy in optical biopsy of vocal cord leukoplakia. Eur Arch Otorhinolaryngol 2017;274:355-9. [Crossref] [PubMed]
- Mascharak S, Baird BJ, Holsinger FC. Detecting oropharyngeal carcinoma using multispectral, narrow-band imaging and machine learning. Laryngoscope 2018;128:2514-20. [Crossref] [PubMed]
- Paderno A, Piazza C, Del Bon F, et al. Deep Learning for Automatic Segmentation of Oral and Oropharyngeal Cancer Using Narrow Band Imaging: Preliminary Experience in a Clinical Perspective. Front Oncol 2021;11:626602. [Crossref] [PubMed]
- Esmaeili N, Illanes A, Boese A, et al. Laryngeal Lesion Classification Based on Vascular Patterns in Contact Endoscopy and Narrow Band Imaging: Manual Versus Automatic Approach. Sensors (Basel) 2020;20:4018. [Crossref] [PubMed]
- Staníková L, Walderová R, Jančatová D, et al. Comparison of narrow band imaging and the Storz Professional Image Enhancement System for detection of laryngeal and hypopharyngeal pathologies. Eur Arch Otorhinolaryngol 2018;275:1819-25. [Crossref] [PubMed]
- Ni XG, He S, Xu ZG, et al. Endoscopic diagnosis of laryngeal cancer and precancerous lesions by narrow band imaging. J Laryngol Otol 2011;125:288-96. [Crossref] [PubMed]
- AlZubaidi AK, Sideseq FB, Faeq A, et al. Computer aided diagnosis in digital pathology application: Review and perspective approach in lung cancer classification. 2017 Annual Conference on New Trends in Information & Communications Technology Applications (NTICT), 2017:219-24.
- Robertson S, Azizpour H, Smith K, et al. Digital image analysis in breast pathology-from image processing techniques to artificial intelligence. Transl Res 2018;194:19-35. [Crossref] [PubMed]
- Liu C, Pang M. Extracting Lungs from CT Images via Deep Convolutional Neural Network Based Segmentation and Two-Pass Contour Refinement. J Digit Imaging 2020;33:1465-78. [Crossref] [PubMed]
- Ren Y, He Y. J Healthc Eng 2021;2021:6076135. [PubMed]
- Esteva A, Kuprel B, Novoa RA, et al. Dermatologist-level classification of skin cancer with deep neural networks. Nature 2017;542:115-8. [Crossref] [PubMed]
- Ting DSW, Cheung CY, Lim G, et al. Development and Validation of a Deep Learning System for Diabetic Retinopathy and Related Eye Diseases Using Retinal Images From Multiethnic Populations With Diabetes. JAMA 2017;318:2211-23. [Crossref] [PubMed]
- Long E, Lin H, Liu Z, et al. An artificial intelligence platform for the multihospital collaborative management of congenital cataracts. Nat Biomed Eng 2017;1:0024.
- Xi IL, Zhao Y, Wang R, et al. Deep Learning to Distinguish Benign from Malignant Renal Lesions Based on Routine MR Imaging. Clin Cancer Res 2020;26:1944-52. [Crossref] [PubMed]
- Kulkarni PM, Robinson EJ, Sarin Pradhan J, et al. Deep Learning Based on Standard H&E Images of Primary Melanoma Tumors Identifies Patients at Risk for Visceral Recurrence and Death. Clin Cancer Res 2020;26:1126-34. [Crossref] [PubMed]
- He J, Baxter SL, Xu J, et al. The practical implementation of artificial intelligence technologies in medicine. Nat Med 2019;25:30-6. [Crossref] [PubMed]
- Hu R, Zhong Q, Xu ZG, et al. Application of deep convolutional neural networks in the diagnosis of laryngeal squamous cell carcinoma based on narrow band imaging endoscopy. Zhonghua Er Bi Yan Hou Tou Jing Wai Ke Za Zhi 2021;56:454-8. [PubMed]
- Szegedy C, Vanhoucke V, Ioffe S, et al. Rethinking the inception architecture for computer vision. 2016 IEEE Conference on Computer Vision and Pattern Recognition (CVPR), 2016:2818-26.
- Szegedy C, Liu W, Jia Y, et al. Going deeper with convolutions. 2015 IEEE Conference on Computer Vision and Pattern Recognition (CVPR), 2015:1-9.
- Xiong H, Lin P, Yu JG, et al. Computer-aided diagnosis of laryngeal cancer via deep learning based on laryngoscopic images. EBioMedicine 2019;48:92-9. [Crossref] [PubMed]
- Ren J, Jing X, Wang J, et al. Automatic Recognition of Laryngoscopic Images Using a Deep-Learning Technique. Laryngoscope 2020;130:E686-93. [Crossref] [PubMed]
- Chang K, Bai HX, Zhou H, et al. Residual Convolutional Neural Network for the Determination of IDH Status in Low- and High-Grade Gliomas from MR Imaging. Clin Cancer Res 2018;24:1073-81. [Crossref] [PubMed]
- Ehteshami Bejnordi B, Veta M, Johannes van Diest P, et al. Diagnostic Assessment of Deep Learning Algorithms for Detection of Lymph Node Metastases in Women With Breast Cancer. JAMA 2017;318:2199-210. [Crossref] [PubMed]
- Cosway B, Drinnan M, Paleri V. Narrow band imaging for the diagnosis of head and neck squamous cell carcinoma: A systematic review. Head Neck 2016;38:E2358-67. [Crossref] [PubMed]
- Ni XG, Wang GQ. The Role of Narrow Band Imaging in Head and Neck Cancers. Curr Oncol Rep 2016;18:10. [Crossref] [PubMed]
- Ting DSW, Liu Y, Burlina P, et al. AI for medical imaging goes deep. Nat Med 2018;24:539-40. [Crossref] [PubMed]
- Bychkov D, Linder N, Turkki R, et al. Deep learning based tissue analysis predicts outcome in colorectal cancer. Sci Rep 2018;8:3395. [Crossref] [PubMed]
(English Language Editor: L. Huleatt)