Prediction of distant metastasis and survival prediction of gastric cancer patients with metastasis to the liver, lung, bone, and brain: research based on the SEER database
Introduction
Gastric cancer (GC) is a globally important disease. It is the 5th most common malignancy, and the 4th most common cause of death from cancer in the world (1). In 2020, 769,000 people died of GC worldwide, and it has been estimated that there are >1 million new cases of GC each year (2,3). As patients with GC are often at an advanced stage when they are first diagnosed, the mortality rate of GC is extremely high, and the median survival rate of advanced stage GC patients is <12 months (4). Most newly diagnosed cases of GC involve locally advanced tumor growth or distant metastasis (5). According to a GC analysis in Sweden, >40% of GC patients had metastatic disease (6).
Some previous reports indicate that gender, age, race, TNM staging, lung metastasis, and tumor size are considered to be significantly related to the survival of elderly patients with gastric cancer (7). Tumor size and TMN stage are significantly related to the survival of young gastric cancer patients (8). Many studies have proposed that age, race, tumor size, and depth may be risk factors for distant metastasis of gastric cancer (9).
At present, the nomogram of distant metastasis and prognosis of GC has not been fully developed and verified. Compared with the previous prognostic analysis for different gastric cancer subtypes, we believed that GC patients need to establish a reliable clinical model with good performance.
The Surveillance, Epidemiology, and End Results (SEER) is the authoritative source of cancer statistics in the United States (US). In this study, we used data from SEER cancer registry of patients diagnosed with GC from 2010 to 2015 to establish a survival prediction model. On this basis, we had also established prediction models for distant metastasis in patients with GC.
We present the following article in accordance with the TRIPOD reporting checklist (available at https://dx.doi.org/10.21037/atm-21-6295).
Methods
The study was conducted in accordance with the Declaration of Helsinki (as revised in 2013).
SEER database
The SEER program provides cancer statistics in an effort to reduce the cancer burden in the US population. SEER is supported by the Surveillance Research Program of the Division of Cancer Control and Population Sciences (DCCPS) of the National Cancer Institute. The SEER database released information on metastases in the liver, lungs, bones, and brain in 2010 (10). Public original data were obtained from the SEER database. The data were downloaded by SEER*Stat Software (version 8.3.9). The exact data used were extracted from the “Incidence–SEER Research Data, 18 Registries, Nov 2020 Sub (2000–2018)—Linked to County Attributes—Time Dependent (1990–2018) Income/Rurality, 1969–2019 Counties, National Cancer Institute, DCCPS, Surveillance Research Program”, released April 2021, based on the November 2020 submission.
Patients
Histological types were defined by the following ICD-O-3 codes: 8140 to 8147, 8210 to 8211, 8220 to 8221, and 8260 to 8263 for adenocarcinoma, 8480 and 8481 for mucinous adenocarcinoma, and 8490 for Signet ring cell carcinoma.
The primary site was defined by the site recode ICD-O-3/WHO 2008: Stomach.
Patients were excluded if they met any of the following exclusion criteria: (I) it was unknown whether metastasis had occurred; (II) their survival time was “0” or unknown; (III) the patient did not have a tumor, node, metastasis (TMN) stage record; and/or (IV) information about collaborative stage (CS) extension or CS tumor size was missing or incomplete.
Statistical analysis
The categorized data are described as numbers and percentages (N, %). All the statistical analyses were performed using the R programming language and environment (http://www.r-project.org/). Univariate and multivariate logistic regression analysis were conducted to identify the risk and prognostic factors of GC patients with metastasis. A 2-tailed P value <0.05 was considered statistically significant (11). Factors that are statistically significant in univariate and multivariate analysis were included in the construction of nomograms. The “regplot” software package was used to construct nomograms to predict the probability of distant metastasis and the survival time of GC patients (12). The median overall survival (OS) time was determined using Kaplan-Meier survival curves, and differences were assessed using the log-rank test. The outcomes of the prediction models include liver metastasis, lung metastasis, brain metastasis, bone metastasis and OS time of GC patients.
Model validation
Receiver operating characteristic (ROC) curves were used to verify the diagnostic accuracy and sensitivity of the nomograms, which are useful for organizing classifiers and visualizing their performance (13). The higher the area under the curve (AUC), the higher the accuracy of the nomogram (14). Calibration plots (graphical tools for investigating the reliability of prediction models) were used to verify the prediction ability of the survival nomogram (15). Decision curve analyses (DCAs) and clinical impact curves (CICs) were used to examine the discrimination and calibration of the model, and the clinical impact of the model was quantified using R package “rmda” (16,17).
Results
Clinical characteristics of GC patients in the SEER cohort
From 2010 to 2015, 18,752 GC patients in the SEER database met the inclusion criteria for this study (see Figure 1). Among them, 1,775 GC patients had liver metastasis, 594 had lung metastasis, 479 had bone metastasis, and 73 had brain metastasis.
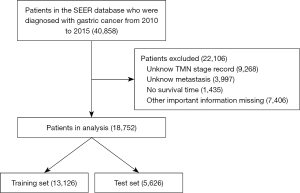
The patients were randomly divided into training and validation sets according to the ratio of 7:3. Table 1 sets out the demographic and clinicopathological characteristics of the patients in the training cohort (n=13,126) and the validation cohort (n=5,626). 60% of the patients were aged >60 years. We used the 8th edition of American Joint Committee on Cancer (AJCC) to classify the TMN stages of GC patients. Under the AJCC (8th ed.) 26%, 20%, 29%, and 25% of the patients had stage I, stage II, stage II and stage IV TNM GC, respectively. More than 70% of the patients had adenocarcinoma. The incidence of lung, liver, brain, and bone metastasis was 3%, 9%, 3%, and 0.4%, respectively. The incidence of patients with distant metastases to 1 of these 4 sites was 12.7%.
Table 1
Variable | All subjects, N=18,752 | Training cohort, N=13,126 | Validation cohort, N=5,626 | |||||
---|---|---|---|---|---|---|---|---|
N | % | N | % | N | % | |||
Age | ||||||||
<40 | 629 | 3 | 437 | 3 | 192 | 3 | ||
40–49 | 1,447 | 8 | 1,006 | 8 | 441 | 8 | ||
50–59 | 3,578 | 19 | 2,512 | 19 | 1,066 | 19 | ||
60–69 | 5,413 | 29 | 3,783 | 29 | 1,630 | 29 | ||
70–79 | 5,528 | 29 | 3,849 | 29 | 1,679 | 30 | ||
≥80 | 2,157 | 12 | 1,539 | 12 | 618 | 11 | ||
AJCC stage | ||||||||
IA | 3,337 | 18 | 2,332 | 18 | 1,005 | 18 | ||
IB | 1,527 | 8 | 1,058 | 8 | 469 | 8 | ||
IIA | 1,389 | 7 | 988 | 8 | 401 | 7 | ||
IIB | 2,354 | 13 | 1,687 | 13 | 667 | 12 | ||
IIIA | 2,847 | 15 | 1,963 | 15 | 884 | 16 | ||
IIIB | 1,549 | 8 | 1,103 | 8 | 446 | 8 | ||
IIIC | 1,034 | 6 | 725 | 6 | 309 | 5 | ||
IV | 4,715 | 25 | 3,270 | 25 | 1,445 | 26 | ||
Histology | ||||||||
Adenocarcinoma | 14,423 | 77 | 10,109 | 77 | 4,314 | 77 | ||
Mucinous adenocarcinoma | 394 | 2 | 255 | 2 | 139 | 2 | ||
Signet ring cell carcinoma | 3,935 | 21 | 2,762 | 21 | 1,173 | 21 | ||
Tumor size | ||||||||
0 | 1,969 | 11 | 1,346 | 10 | 623 | 11 | ||
1 | 5,923 | 32 | 4,177 | 32 | 1,746 | 31 | ||
3 | 7,926 | 42 | 5,570 | 42 | 2,356 | 42 | ||
5 | 1,538 | 8 | 1,061 | 8 | 477 | 8 | ||
6 | 1,396 | 7 | 972 | 7 | 424 | 8 | ||
Extension | ||||||||
<300 | 5,485 | 29 | 3,856 | 29 | 1,629 | 29 | ||
300–600 | 11,244 | 60 | 7,841 | 60 | 3,403 | 60 | ||
>600 | 2,023 | 11 | 1,429 | 11 | 594 | 11 | ||
N stage | ||||||||
N0 | 8,803 | 47 | 6,114 | 47 | 2,689 | 48 | ||
N1 | 5,353 | 29 | 3,785 | 29 | 1,568 | 28 | ||
N2 | 2,319 | 12 | 1,617 | 12 | 702 | 12 | ||
N3 | 2,277 | 12 | 1,610 | 12 | 667 | 12 | ||
Lung Met | ||||||||
Yes | 594 | 3 | 418 | 3 | 176 | 3 | ||
No | 18,158 | 97 | 12,708 | 97 | 5,450 | 97 | ||
Liver Met | ||||||||
Yes | 1,775 | 9 | 1,227 | 9 | 548 | 10 | ||
No | 16,977 | 91 | 11,899 | 91 | 5,078 | 90 | ||
Bone Met | ||||||||
Yes | 479 | 3 | 332 | 3 | 147 | 3 | ||
No | 18,273 | 97 | 12,794 | 97 | 5,479 | 97 | ||
Brain Met | ||||||||
Yes | 73 | 0.4 | 49 | 0.4 | 24 | 0.4 | ||
No | 18,679 | 99.6 | 13,077 | 99.6 | 5,602 | 99.6 |
Prediction of distant metastasis in patients with GC
We analyzed the risk factors of distant metastasis in patients with GC. The univariate analysis and the multivariate analysis showed that tumor size, N stage, histological type, and extension were related to liver metastasis, tumor size, N stage, age, and extension were related to lung metastasis and bone metastasis, and tumor size, N stage, T stage, and extension were related to brain metastasis. Using the risk factors identified in the multivariate logistic regression analysis model, we constructed 4 nomograms for distant metastasis of the liver, lung, bone, and brain (see Figure 2). The total number of points can be attached to the probability of distant metastasis by calculating each variable point.
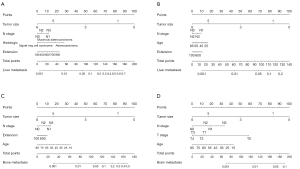
The ROC curves used to assess the nomogram of distant metastasis are shown in Figure 3. The area under the curve (AUC) of liver metastasis was 0.817 (see Figure 3A), the AUC of lung metastasis was 0.811 (see Figure 3B), the AUC of bone metastasis was 0.818 (see Figure 3C), and the AUC of brain metastasis was 0.784 (see Figure 3D). The AUCs show that the nomograms had a good predictive performance. We also constructed forest plots of lung metastasis and liver metastasis (see Figure 4).
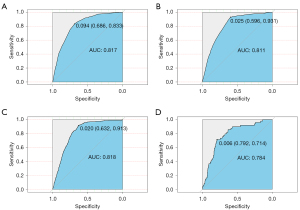
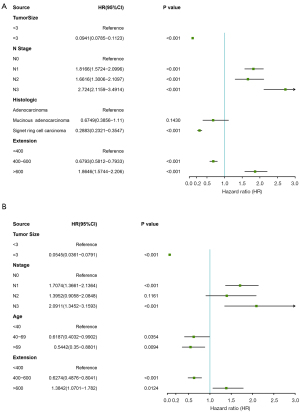
In this study, the median OS for patients was 20 months for the whole cohort. The median OS time of patients with liver metastasis was 7 months. The median OS time of patients with lung metastasis was 5 months. The median OS time of patients with brain metastasis was 5 months. The median OS time of patients with bone metastasis was 6 months. The median OS times of patients with different types of metastasis are shown in Figure 5. The decision curve and clinical impact curve analyses showed that within a large interval, the benefits of the 4 metastasis prediction models were higher than the extreme curve, which proves that the prediction models had good clinical utility (see Figures 6,7).
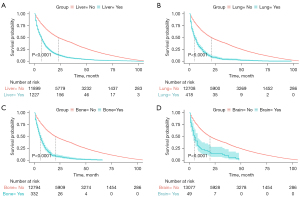
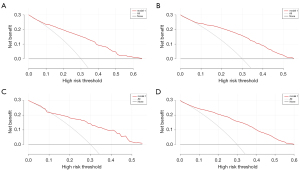
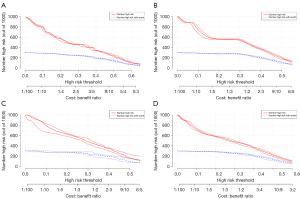
Construction and validation of the OS nomogram
The survival-related factors of the SEER cohort were determined based on the multivariate logistic regression analysis model. A forest plot was constructed to show the survival-related factors and their P value (see Figure 8). All of these factors were used to construct a survival prediction nomogram for GC patients at 12, 24, and 36 months (see Figure 9). By adding up the total scores shown in the bottom scale, the nomogram could predict the OS for individual patients at 12, 24, and 36 months. The C-index of the survival prediction nomogram was 0.701. In the test cohort, the C-index of the survival prediction nomogram was 0.703. The ROC curves were used to evaluate the survival prediction nomogram (see Figure 10). The calibration plots of the model showed that the 24-month survival time predicted was consistent with the actual value (see Figure 11).
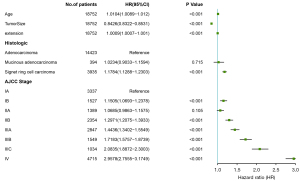
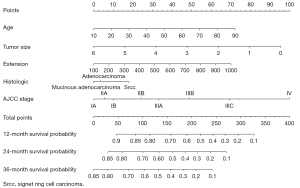
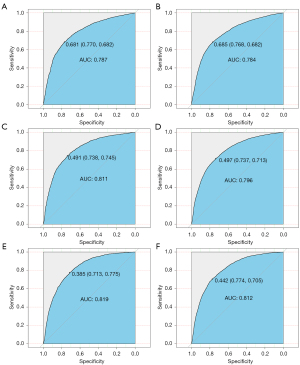
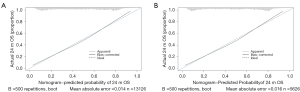
Discussion
At present, due to the early diagnosis and standardized treatment of GC, the survival time of patients is significantly longer than it was previously; however, overall it is still relatively poor (18). Distant organ metastasis is a sign of poor prognosis in patients with GC. Thus, a prediction model for distant organ metastasis in patients with GC could help to identify patients who are prone to distant metastasis based on clinical characteristics. Following the in-depth study of GC in recent years, many clinical molecular markers had been identified that can be used in the prediction of distant metastasis and the survival time of GC patients. Further, such molecular markers may help in the early diagnosis of metastasis and the development of individualized treatment strategies.
In the distant metastasis data of GC patients, we found that the probability of liver metastasis was 9%, which was the highest among the 4 organs. The probability of liver metastasis was the highest among the 4 organs may be due to differences in the metastasis pathways and the popularity of the inspection measures. The common ways for patients with GC to develop distant metastasis are lymph node metastasis and abdominal cavity metastasis (19,20). Conversely, the common ways of liver metastasis are direct infiltration, blood metastasis, lymphatic metastasis, and planting metastasis (19). The liver is connected to digestive organs, such as the stomach, through the hepatic portal vein, which is conducive to the blood metastasis of GC (21). Studies have shown that GC cells spread to various organs through the portal vein, and the liver becomes the first filter for GC cells (22). This may be why the liver is the first organ of distant metastasis in patients with GC (6). Due to advancements in medical technologies and the continuous improvement of detection methods, cases of liver metastasis can be detected in early or even asymptomatic stages (23).
We found that patients with GC have a low rate of bone metastasis (the incidence of bone metastasis was 3%). The occurrence of GC bone metastasis may be underestimated because GC bone metastasis is rare, and bone metastasis is usually not included in routine examinations (24). Using the prediction model for GC bone metastasis, patients at high risk of bone metastasis could be detected in advance. It would be unreasonable to suggest that all parts of their body be checked in all GC patients. However, those at high risk of metastasis could be identified using the metastasis prediction model, and targeted inspection measures and feasible medical solutions could be formulated.
In this study, the incidence of brain metastases was 0.4%. In stage-IV patients, the probability of brain metastasis was 1.5%. As many patients with brain metastasis have a short survival time and rapid disease progression, there is a lack of clinical information about these patients, and these patients could not be included in our model. Thus, the incidence of brain metastasis in this study may be underestimated. The actual incidence of brain metastasis from GC is not low. Indeed, about 10% of cancer patients will develop brain metastasis during advanced disease progression (25). When brain metastasis occurs, the patient’s survival period is significantly shortened (11,25). Even if more and more targeted drugs or chemotherapeutic drugs are developed to prolong the survival time of patients, the existence of the blood-brain barrier makes it difficult to increase the concentration of drugs in the brain, resulting in poor therapeutic effects (26). Through this prediction model, we can identify patients at high risk of brain metastasis early and implement corresponding measures as early as possible to reduce or even block the occurrence of brain metastasis.
In our study, the younger the age, the higher the probability of bone metastasis and brain metastasis. This may be due to differences in lymph node involvement in different age groups. Among GC patients, the proportion of patients with >15 lymph node metastases decreases significantly with age (27). Many studies have also shown that a younger age is positively correlated with distant metastasis in GC patients (28). Palliative chemotherapy has been reported to improve the survival rate of GC patients with bone metastasis (29).
In this study, we first downloaded data from the SEER public database on GC patients. Studies have shown that a number of factors, including histological type, TMN staging, and age, may be signs of distant metastasis (10,11,30). Through single-factor and multi-factor analyses, we selected factors, such as N stage, tumor invasion, and tumor size, and established a predictive model for distant metastasis. Many studies have evaluated the survival rate and related factors of patients with GC metastasis, and found that age, tumor stage, and tumor histological type, are independent factors related to the survival of GC patients (28,31,32). Our prediction model included tumor stage, tumor histology type, tumor invasion depth, tumor size and age. However, many other factors, such as marital status, sex and insurance, are also considered independent factors for the survival of GC patients (28,33,34). We did not include all such factors in our prediction model, as including too many variables may lead to the over-fitting of models, which in turn may lead to falsely high ROC results. The results of the present study have been detailed above. We made individualized predictions based on the different clinical characteristics of each patient, which is somewhat better than previous studies that have only compared the possible metastasis of different types of GC patients.
In our study, we conducted a quantitative evaluation of the incidence of distant metastasis in patients with different clinical characteristics, established 4 prediction models for distant metastasis in the liver, lung, brain, and bone, and established ROC curves to evaluate their predictive efficacy. Among them, the predictive performance of bone metastasis model was the best, and had an AUC was as high as 0.818. This may be because bone metastasis patients has good homogeneity.
We also established a survival prediction nomogram to establish a prediction model for the survival of different types of patients. We used ROC curves to evaluate the predictive efficacy of the survival prediction model, and found that the 36-month prediction model performed the best, and had an AUC as high as 0.819 and 0.812 in train set and test set. Yu et al. also constructed a nomogram for young GC patients, and their 3-year OS AUC was 0.763 (8). Similarly, Zhang et al. constructed a nomogram for the survival rate of elderly GC patients after surgery (7), and their 3-year OS had a c-index of 0.765. Our c-index was 0.811. We drew a calibration curve to prove that its performance was very good, and that the prediction model has good clinical value. Survival prediction models could be used to effectively prevent excessive treatment, prevent the wastage of medical resources, and provide a scientific basis for medical staff and patients and their families to make medical decisions.
In our survival prediction model, the median OS time was 20 months, and the median OS time of patients with liver, lung, bone, and brain metastasis was 7, 5, 6, and 5 months, respectively. In our data, the 1-year survival rate of patients with liver metastasis was 29%. Some reports have indicated that the median survival time after diagnosis of liver metastasis is 4–34 months; however, if there are other distant metastases at the same time, the survival time is shorter (10,22,31,35). The median survival time of patients diagnosed with bone metastasis is approximately 4–7 months (10,36). In general, our findings did not differ greatly from the findings of other studies.
According to previous studies, surgery is the only possible cure for GC (37). D2 lymphadenectomy with spleen and pancreas preservation may reduce the possibility of recurrence and distant metastasis after surgery, so that GC patients can obtain better survival benefits (38). Compared with chemotherapy alone, adding trastuzumab to the treatment of patients with HER2 receptor overexpression can improve overall survival and progression-free survival (37).
Many studies had shown that biomarkers play a key mechanism in angiogenesis and cancer metastasis (39). miR-375 partially inhibits the migration and invasion of GC cells by targeting JAK2 oncogene (40), and miR-10b activates RhoC-AKT signaling by targeting HOXD10 Conduction to promote the invasion of gastric cells (41). Targeted therapies for these potential molecular targets may have important implications for the distant metastasis of gastric cancer.
Our models have certain limitations, which we hope to resolve in our future work. First, we only selected the SEER database for GC patients from 2010–2015; thus, we had a small sample size, and we did not compare the data with data from other databases. Second, we only had information about the transfer of the liver, lung, bone and brain, and no information about other body parts. Third, the question of whether our models are reliable requires further verification by prospective cohort studies or case-control studies. Fourth, the data in this study came from American patients, and there are differences between Eastern and Western populations. Whether the model can be used for patients in other regions requires further research. In the future, we hope to conduct research on data in other regions to further improve the models. In addition, the question of how to more scientifically screen for a lack of information is a problem that limited our accuracy when constructing the models for patients.
In general, we established a model for predicting distant metastasis and the survival of GC patients using a GC patient data set on the SEER database. The models were verified to have a good predictive performance and can provide some references for formulating treatment plans for patients with GC.
Conclusions
Establishing a prediction model for distant metastasis and the survival of GC patients is of great clinical significance. The prediction of distant metastasis could help clinicians to conduct individualized assessments of patients and formulate individualized examination measures. Survival prediction models could also help oncologists formulate good treatment strategies and provide hospice care.
Acknowledgments
Funding: None.
Footnote
Reporting Checklist: The authors have completed the TRIPOD reporting checklist. Available at https://dx.doi.org/10.21037/atm-21-6295
Conflicts of Interest: All authors have completed the ICMJE uniform disclosure form (available at https://dx.doi.org/10.21037/atm-21-6295). The authors have no conflicts of interest to declare.
Ethical Statement: The authors are accountable for all aspects of the work in ensuring that questions related to the accuracy or integrity of any part of the work are appropriately investigated and resolved. The study was conducted in accordance with the Declaration of Helsinki (as revised in 2013).
Open Access Statement: This is an Open Access article distributed in accordance with the Creative Commons Attribution-NonCommercial-NoDerivs 4.0 International License (CC BY-NC-ND 4.0), which permits the non-commercial replication and distribution of the article with the strict proviso that no changes or edits are made and the original work is properly cited (including links to both the formal publication through the relevant DOI and the license). See: https://creativecommons.org/licenses/by-nc-nd/4.0/.
References
- Ferlay J, Colombet M, Soerjomataram I, et al. Cancer statistics for the year 2020: An overview. Int J Cancer 2021; Epub ahead of print. [Crossref] [PubMed]
- Sung H, Ferlay J, Siegel RL, et al. Global Cancer Statistics 2020: GLOBOCAN Estimates of Incidence and Mortality Worldwide for 36 Cancers in 185 Countries. CA Cancer J Clin 2021;71:209-49. [Crossref] [PubMed]
- Smyth EC, Nilsson M, Grabsch HI, et al. Gastric cancer. Lancet 2020;396:635-48. [Crossref] [PubMed]
- Zhang XY, Zhang PY. Gastric cancer: somatic genetics as a guide to therapy. J Med Genet 2017;54:305-12. [Crossref] [PubMed]
- Dicken BJ, Bigam DL, Cass C, et al. Gastric adenocarcinoma: review and considerations for future directions. Ann Surg 2005;241:27-39. [Crossref] [PubMed]
- Riihimäki M, Hemminki A, Sundquist K, et al. Metastatic spread in patients with gastric cancer. Oncotarget 2016;7:52307-16. [Crossref] [PubMed]
- Zhang Y, Yu C. Development and validation of a Surveillance, Epidemiology, and End Results (SEER)-based prognostic nomogram for predicting survival in elderly patients with gastric cancer after surgery. J Gastrointest Oncol 2021;12:278-96. [Crossref] [PubMed]
- Yu C, Zhang Y. Development and validation of prognostic nomogram for young patients with gastric cancer. Ann Transl Med 2019;7:641. [Crossref] [PubMed]
- Chen J, Wu L, Zhang Z, et al. A clinical model to predict distant metastasis in patients with superficial gastric cancer with negative lymph node metastasis and a survival analysis for patients with metastasis. Cancer Med 2021;10:944-55. [Crossref] [PubMed]
- Qiu MZ, Shi SM, Chen ZH, et al. Frequency and clinicopathological features of metastasis to liver, lung, bone, and brain from gastric cancer: A SEER-based study. Cancer Med 2018;7:3662-72. [Crossref] [PubMed]
- Zhu Y, Fang X, Wang L, et al. A Predictive Nomogram for Early Death of Metastatic Gastric Cancer: A Retrospective Study in the SEER Database and China. J Cancer 2020;11:5527-35. [Crossref] [PubMed]
- Shen H, Deng G, Chen Q, et al. The incidence, risk factors and predictive nomograms for early death of lung cancer with synchronous brain metastasis: a retrospective study in the SEER database. BMC Cancer 2021;21:825. [Crossref] [PubMed]
- Fawcett T. An introduction to ROC analysis. Pattern Recognition Letters 2006;27:861-74. [Crossref]
- Wu J, Zhang H, Li L, et al. A nomogram for predicting overall survival in patients with low-grade endometrial stromal sarcoma: A population-based analysis. Cancer Commun (Lond) 2020;40:301-12. [Crossref] [PubMed]
- Gerds TA, Andersen PK, Kattan MW. Calibration plots for risk prediction models in the presence of competing risks. Stat Med 2014;33:3191-203. [Crossref] [PubMed]
- Kastrinos F, Uno H, Ukaegbu C, et al. Development and Validation of the PREMM5 Model for Comprehensive Risk Assessment of Lynch Syndrome. J Clin Oncol 2017;35:2165-72. [Crossref] [PubMed]
- Kerr KF, Brown MD, Zhu K, et al. Assessing the Clinical Impact of Risk Prediction Models With Decision Curves: Guidance for Correct Interpretation and Appropriate Use. J Clin Oncol 2016;34:2534-40. [Crossref] [PubMed]
- Jin X, Zhu Z, Shi Y. Metastasis mechanism and gene/protein expression in gastric cancer with distant organs metastasis. Bull Cancer 2014;101:E1-12. [Crossref] [PubMed]
- Maehara Y, Moriguchi S, Kakeji Y, et al. Pertinent risk factors and gastric carcinoma with synchronous peritoneal dissemination or liver metastasis. Surgery 1991;110:820-3. [PubMed]
- Esaki Y, Hirayama R, Hirokawa K. A comparison of patterns of metastasis in gastric cancer by histologic type and age. Cancer 1990;65:2086-90. [Crossref] [PubMed]
- Zhang H, Deng T, Liu R, et al. Exosome-delivered EGFR regulates liver microenvironment to promote gastric cancer liver metastasis. Nat Commun 2017;8:15016. [Crossref] [PubMed]
- Liu J, Chen L. Current status and progress in gastric cancer with liver metastasis. Chin Med J (Engl) 2011;124:445-56. [PubMed]
- Li Y, Xie D, Chen X, et al. Prognostic Value of the Site of Distant Metastasis and Surgical Interventions in Metastatic Gastric Cancer: A Population-Based Study. Technol Cancer Res Treat 2020;19:1533033820964131. [Crossref] [PubMed]
- Mikami J, Kimura Y, Makari Y, et al. Clinical outcomes and prognostic factors for gastric cancer patients with bone metastasis. World J Surg Oncol 2017;15:8. [Crossref] [PubMed]
- Lemke J, Scheele J, Kapapa T, et al. Brain metastases in gastrointestinal cancers: is there a role for surgery? Int J Mol Sci 2014;15:16816-30. [Crossref] [PubMed]
- Daneman R, Prat A. The blood-brain barrier. Cold Spring Harb Perspect Biol 2015;7:a020412. [Crossref] [PubMed]
- Ahmad A, Khan H, Cholankeril G, et al. The impact of age on nodal metastases and survival in gastric cancer. J Surg Res 2016;202:428-35. [Crossref] [PubMed]
- Zhang Y, Lin Y, Duan J, et al. A Population-Based Analysis of Distant Metastasis in Stage IV Gastric Cancer. Med Sci Monit 2020;26:e923867. [Crossref] [PubMed]
- Turkoz FP, Solak M, Kilickap S, et al. Bone metastasis from gastric cancer: the incidence, clinicopathological features, and influence on survival. J Gastric Cancer 2014;14:164-72. [Crossref] [PubMed]
- Maehara Y, Tomisaki S, Oda S, et al. Lymphatic advancement to peritoneal dissemination and liver metastasis in gastric cancer patients. Anticancer Res 1994;14:2755-7. [PubMed]
- Shiraishi N, Sato K, Yasuda K, et al. Multivariate prognostic study on large gastric cancer. J Surg Oncol 2007;96:14-8. [Crossref] [PubMed]
- Ma T, Wu ZJ, Xu H, et al. Nomograms for predicting survival in patients with metastatic gastric adenocarcinoma who undergo palliative gastrectomy. BMC Cancer 2019;19:852. [Crossref] [PubMed]
- Gadde R, Tamariz L, Hanna M, et al. Metastatic gastric cancer (MGC) patients: Can we improve survival by metastasectomy? A systematic review and meta-analysis. J Surg Oncol 2015;112:38-45. [Crossref] [PubMed]
- Qiu M, Yang D, Xu R. Impact of marital status on survival of gastric adenocarcinoma patients: Results from the Surveillance Epidemiology and End Results (SEER) Database. Sci Rep 2016;6:21098. [Crossref] [PubMed]
- Tiberio GA, Coniglio A, Marchet A, et al. Metachronous hepatic metastases from gastric carcinoma: a multicentric survey. Eur J Surg Oncol 2009;35:486-91. [Crossref] [PubMed]
- Ahn JB, Ha TK, Kwon SJ. Bone metastasis in gastric cancer patients. J Gastric Cancer 2011;11:38-45. [Crossref] [PubMed]
- Orditura M, Galizia G, Sforza V, et al. Treatment of gastric cancer. World J Gastroenterol 2014;20:1635-49. [Crossref] [PubMed]
- Jiang L, Yang KH, Guan QL, et al. Survival and recurrence free benefits with different lymphadenectomy for resectable gastric cancer: a meta-analysis. J Surg Oncol 2013;107:807-14. [Crossref] [PubMed]
- Zhong J, Chen Y, Wang LJ. Emerging molecular basis of hematogenous metastasis in gastric cancer. World J Gastroenterol 2016;22:2434-40. [Crossref] [PubMed]
- Xu Y, Jin J, Liu Y, et al. Snail-regulated MiR-375 inhibits migration and invasion of gastric cancer cells by targeting JAK2. PLoS One 2014;9:e99516. [Crossref] [PubMed]
- Liu Z, Zhu J, Cao H, et al. miR-10b promotes cell invasion through RhoC-AKT signaling pathway by targeting HOXD10 in gastric cancer. Int J Oncol 2012;40:1553-60. [PubMed]