Immune abnormalities and differential gene expression in the hippocampus and peripheral blood of patients with Alzheimer’s disease
Introduction
Alzheimer’s disease (AD) is a progressive neurodegenerative disease with primary clinical manifestations, including memory decline, cognitive impairment, and behavioral abnormalities (1). In 2018, AD was diagnosed in one individual every 3 seconds worldwide. Fifty million people are currently living with AD, and this number will be three times as high by the year 2050. The global cost of AD care in the year 2018 was $1 trillion, which is anticipated to rise to $2 trillion by 2030 (2). Considering the high morbidity and economic burden of AD, disentangling the etiology, and finding preventive measures are urgently needed.
Despite decades of research, no precise mechanisms of AD development have been elucidated. Hypotheses include the aggregation of amyloid-β proteins into senile plaques (3), abnormal phosphorylation of τ protein to form neurofibrillary tangles (4), and early loss of synapses (5). Early detection methods were based on biomarkers expressed in the brain tissue and cerebrospinal fluid, which present challenges due to the highly invasive collection process and high cost. Therefore, obtaining peripheral blood biomarkers, which is non-invasive and cost-effective, is more applicable. Trying to find common genes differentially expressed in the peripheral blood cells and brain tissue is one of the most interesting aspects of investigating AD. Various diagnostic methods for AD have been studied, including cognitive tests (6), structural magnetic resonance imaging (7), electroencephalogram (8), 18F-fluorodeoxyglucose positron emission tomography (9), and cerebrospinal fluid biomarkers (10); however, each method has its own limitations. Therefore, we performed a bioinformatic analysis using human blood gene expression data from the Gene Expression Omnibus (GEO) database to explore more suitable detection methods.
In addition to the classical pathological hallmarks, neuroinflammation has recently been observed in AD (11). Neuroinflammation is not restricted to the microglia and astrocytes, which are the brain’s resident immune cells, but includes strong interactions with peripheral immune cells including neutrophils (12), macrophages (13), and lymphocytes (14). Specifically, T-lymphocytes have been detected in human AD post-mortem specimens (15). In this study, we addressed the role of gene expression in AD and focused on the possibility that peripheral immune cells serve as hematological signatures that could aid in both diagnosis and management. We present the following article in accordance with the MDAR reporting checklist (available at https://atm.amegroups.com/article/view/10.21037/atm-21-4974/rc).
Methods
Data acquisition
The microarray datasets used in this study were selected from the GEO database of the National Center for Biotechnology Information (http://www.ncbi.nlm.gov/geo). For the selection of expression profiling datasets, the following search terms were used: {“Alzheimer disease” [MeSH Terms] OR Alzheimer’s disease [All Fields]} AND {“Homo sapiens” [porgn] AND “Expression profiling by array” [DataSet Type]}. These search criteria allowed for the identification of different mRNA expression datasets, of which only those with >20 samples (total of control and AD samples) were selected for further computational analyses. Among them, two databases of hippocampus samples (GSE1297 and GSE5281) (16) and two databases of peripheral blood mononuclear cells (PBMCs) (GSE63060 and GSE63061) (17) were selected (Table 1). This research did not involve a human subject trial. Instead, the data came exclusively from the GEO. The study was conducted in accordance with the Declaration of Helsinki (as revised in 2013).
Table 1
GEO ID | Platform | Normal-aging sample | AD sample | Resource |
---|---|---|---|---|
GSE1297 | GPL96 [HG-U133A] Affymetrix Human Genome U133A Array | 9 | 22 | Hippocampus |
GSE5281 | GPL570 [HG-U133_Plus_2] Affymetrix Human Genome U133 Plus 2.0 Array | 13 | 10 | Hippocampus |
GSE63060 | GPL6947 Illumina HumanHT-12 V3.0 Expression BeadChip | 104 | 145 | Peripheral blood |
GSE63061 | GPL10558 Illumina HumanHT-12 V4.0 Expression BeadChip | 134 | 139 | Peripheral blood |
GEO, Gene Expression Omnibus; ID, identification; AD, Alzheimer’s disease.
Batch normalization and differential expression analysis
The multiple dataset analyses were based on different instruments with different handlers or in different groups. First, we integrated GSE1297 and GSE5281 using R software with the “sva” package (version 4.0.3; R Foundation for Statistical Computing, Vienna, Austria). The combat approach was used to eliminate batch effects from different studies. Then, we used the “limma” package to identify differentially expressed genes (DEGs); value fold changes (FC) with |logFC| >2 and adjusted P value <0.05 as cutoff values, false discovery rate <0.05). The batch normalization of GSE63060 and GSE63061 used the same method. DEG pheatmaps were created using the trimmed mean of the M value normalization factors.
Enrichment analysis and protein-protein interaction (PPI)
Gene Ontology (GO) and Kyoto Encyclopedia of Genes and Genomes (KEGG) pathway enrichment analyses of DEGs were used in the “clusterProfiler” package in R. GO information was screened with adjusted P value <0.05, including the function of DEGs from three aspects: cellular component, molecular function (MF), and biological process (BP). The KEGG pathway terms with adjusted P value <0.05 were significantly enriched, depicting utilities of the biological system and high-level functions. STRING (version 11.0; https://string-db.org) is an online tool that provides visualization of PPI networks. Networks were performed based on gene expression, and the minimum required interaction score of 0.7 was considered statistically significant.
Exploring immune infiltration
CIBERSORT (https://cibersort.stanford.edu/) is a versatile computer algorithm that quantifies immunological characteristics based on gene expression signatures. We downloaded a gene signature matrix from CIBERSORT that included 22 immune cell types. These cells are a recategorization of 10 major cell types, including naive and memory B, 7 kinds of T (CD8+ T; activated memory, native, and resting memory CD4+ T; and follicular helper, regulatory, and γδ T), 3 kinds of macrophages (M0, M1, and M2), resting and activated dendritic, resting and activated mast, resting and activated natural killer, monocytes, eosinophils, neutrophils, and plasma cells. Then, we predicted the proportions of the 22 types of infiltrating immune cells in the AD and normal-aging samples. The number of permutations was set at 100 to improve the deconvolution algorithm’s accuracy, and P<0.05 was regarded as statistically significant.
Statistical analysis
All analyses were performed using R v.4.0.3 (https://www.R-project.org). All data were expressed as means ± standard deviations (SDs). The Wilcoxon rank-sum test was used for comparison between two groups. All tests were two-sided, and P<0.05 was considered statistically significant.
Results
A total of 576 samples, comprising 54 hippocampal tissue expression samples and 522 PBMC expression samples, were included in the current study. Among the hippocampus tissue expression subset, 9 normal-aging individuals and 22 patients with AD were obtained from GSE1297, and 13 normal-aging individuals and 10 patients with AD were obtained from GSE5281. For PBMCs, 104 normal-aging individuals and 145 patients with AD were obtained from GSE1297, and 134 normal-aging individuals and 139 patients with AD were obtained from GSE5281 (Table 1). In the hippocampus tissue expression subset, the mean age of the 22 normal-aging individuals was 81.95±9.12 years, of whom 7 (31.82%) were female; the mean age for the 32 patients with AD was 83.63±8.19 years, of whom 20 (62.5%) were female. In the PBMC subset, the mean age at onset of the 238 normal-aging individuals in the was 74.02±6.32 years, of whom 143 (60.08%) were female; the mean age at onset for the 284 patients with AD was 76.62±6.73 years, of whom 184 (64.79%) were female (Table S1).
After batch normalization, the DEG analysis was performed using R. A total of 1,261 DEGs were found in the hippocampus tissue subset by matching GSE1297 and GSE5281, including 120 upregulated and 1,141 downregulated DEGs (Figure 1A). A total of 290 DEGs were found in the PBMC subset by matching GSE63060 and GSE63061, including 4 upregulated and 286 downregulated DEGs (Figure 1B). The DEGs identified in each subset are shown in Figure 1C,1D. The results showed 28 differential mRNA expressions among both hippocampus tissue and peripheral blood sets (Figure 2, Table S2).
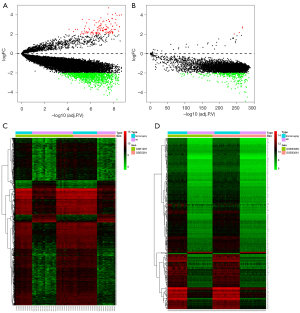
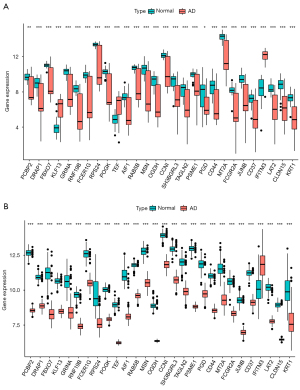
To explore the potential biological functions and signaling pathways of DEGs in patients with AD, we further enriched and analyzed the two subsets. GO enrichment analysis is useful for uncovering the significantly integrated DEGs. Results of the three most significant BP terms, including biological process, cellular component, and MF, in hippocampus tissue are shown in Figure 3. As for BP terms, neutrophil activation involved in immune response was the most significant term, followed by gliogenesis, neutrophil degranulation, glial cell differentiation, and glial cell development. Regarding cellular components (CC), the DEGs were enriched mainly in the collagen-containing extracellular matrix, external side of plasma membrane, vesicle lumen, secretory granule lumen, and cytoplasmic vesicle lumen. Besides the enrichment levels of MF, the BPs were primarily associated with immune receptor activity, carbohydrate binding, and actin binding. In PBMCs (Figure 4), the top three BP terms were neutrophil degranulation, neutrophil activation involved in immune response, and T cell activation. As for cellular component terms, the DEGs were enriched mainly in the mitochondrial matrix, cytoplasmic vesicle lumen, and vesicle lumen. Regarding MF, the DEGs were enriched mainly in protein serine/threonine kinase activity and ubiquitin-like protein ligase binding. KEGG analysis showed the possible signaling pathways of DEGs. We also analyzed the KEGG pathways with an adjusted P value <0.05 that were enriched in hippocampal tissue and PBMC subsets. The KEGG pathways are shown in Figure S1. Although the biological function enrichment of the hippocampus was not identical to PBMCs after screening, there was a close association between the death of neurons, immune response, and mitochondrial function in the hippocampus and PBMCs.
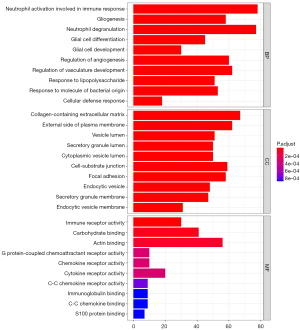
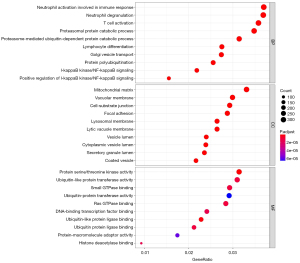
In addition to gene set enrichment analysis, we performed PPI network analysis through the hub DEGs using the STRING interactome database. We performed a zero-order interaction analysis to simplify the network to a more manageable size. As a result, we detected a PPI network, including 668 nodes and 3,121 edges in the hippocampal tissue subset and 142 nodes and 389 edges in the PBMC subset (Figure 5). Figure S2 shows the top 40 hub genes, ranked according to the link in the PPI network (the number of nodes they connect to). According to the enrichment analyses, patients with AD demonstrated close associations with the immune response. Therefore, we further explored the immune cell infiltration patterns of PBMCs to investigate the role of inflammation in the pathogenesis of AD. As shown in Figure 6, fractions of some immune cells varied significantly between normal-aging individuals and patients with AD. In all, four immune cells in the patients with AD group showed significantly higher infiltration levels, including naive CD4+ T cells, resting natural killer cells, M0 macrophages, and activated mast cells, while resting memory CD4+ T cells were significantly lower in the patients with AD group. Although the proportions of the six immune cells were significantly different between the two groups (including native B cells, follicular helper T cells, γδ T cells, resting dendritic cells, resting mast cells, and eosinophils) at a false detection rate <0.05, they were significantly lower in all samples. Importantly, it was difficult to identify if they truly matched the patients with AD group.
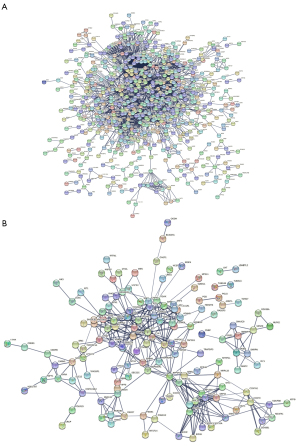
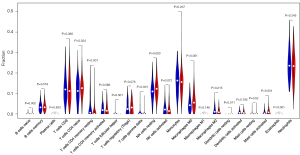
Discussion
With the development of microarrays and high-throughput sequencing technologies, a bioinformatic analysis of AD has been recently investigated; however, most of the current research is based on invasive methods and a single array, leading to poor acceptance and a lack of multiple cohorts for joint research. In our current study, we used both the hippocampus and PBMCs from AD and normal-aging samples to identify blood biomarkers that predicted brain tissue biomarkers to diagnose AD without brain biopsy or a cerebrospinal fluid analysis. By using GO and KEGG analyses, we found that DEGs from the AD and normal-aging samples were related to neuronal death, mitochondrial function, and most importantly, immune response. We further explored the immune cell infiltration patterns of PBMCs to investigate the role of inflammation in the pathogenesis of AD.
Various prior methods have shown that peripheral blood gene expression can be used for the diagnosis of multiple brain disorders despite the effects of the blood-brain barrier (18,19). Moradi et al. (20) used a linear discriminant analysis method to study blood gene expression profiles that shows peripheral blood genes have a potential application value to classify healthy and AD; however the study did not mention the association between peripheral blood genes and brain tissue genes. Voyle et al. (21) applied a pathway-based interpretation of gene expression to diagnosis AD, but the model only performed similarly to a model based on demographic information. Our study used a novel approach by extracting data from the hippocampus and PBMCs of patients with AD and normal-aging samples, and 28 genes were obtained in the intersection between the two groups of DEGs, to link peripheral blood and brain tissue.
RAB5B is among the important GTPases of the RAB protein family (22,23). We found that RAB5B was significantly downregulated in the hippocampus in GSE1297 and GSE5281, and the expression of RAB5B was also downregulated in PBMCs in GSE63060 and GSE63061. N-methyl-D-aspartate (NMDA) receptors have an important role in nerve cell death associated with activation of surface receptors sensitive to glutamate (24). Previous studies showed that NMDA receptors are internalized by a clathrin-dependent mechanism (25,26). A decrease in RAB5B could lead to abnormal internalization of NMDA receptors and induce excitatory toxicity mediated by the receptor (27,28). Considering the dysregulation of the NMDA pathway, RAB5B may be a potential target in AD.
It has been argued that the pathogenesis of AD is associated with abnormal mitochondria that produce excessive neuronal oxidative stress in the surrounding cytoplasm, causing damage to susceptible neurons (29-31). PCBP2, the RPS gene family, and the RAB gene family (32-34) have been implicated in mitochondrial dysfunction and oxidative damage in the pathogenesis of AD in past research, which is consistent with our present results. Consistent with our study, Lunnon et al. (35) also validated mitochondrial dysfunction and inflammatory changes occurring very early in AD. These results will provide a reference for further investigation of early treatment and detection methods.
In addition, through the PPI network, we found that some other hub genes are closely related to AD occurrence. RPS27A, the gene with the largest number of connecting nodes in hippocampal tissue, has not only been shown to be a bridge gene for the progression of AD pathology, but has been widely studied in multiple neurodegenerative diseases (36). APOB has 52 node genes and has been widely used in studies of AD. For example, Di Paolo et al. (37) revealed APOB as a new link between cholesterol and AD and may underlie AD pathogenesis common to both late-onset AD and early-onset AD. VAMP8 was considered the most core gene in peripheral blood consistent with the results of a previous neuronal models study showing that VAMP8 could increase tau secretion (38). Neuroinflammation mediated by microglia and astrocytes, as well as by peripheral immune cells, has been described as an important contributor to AD (39,40). CD44, an inflammation-related gene identified as a binding partner in cell adhesion and migration of immune cells (41,42), may contribute to AD pathology. In our current study, expression of CD44 was significantly downregulated in the hippocampus following changes in PBMCs. Consistent with our results, Uberti et al. (43) reported that CD44 was abnormally expressed in lymphocytes of patients with AD compared with healthy participants, which may be driving the immune response into infected tissues, including the central nervous system. Previous studies have also shown that the expression patterns of the CD44 gene family are different between patients with AD and matched non-AD controls in postmortem hippocampal samples (44). Some CD44 splice variants were localized to neuritic plaques and astrocytes, while some were localized in neuroblastoma cells and primary neurons. In addition, CD44 levels were increased in the frontal cortex of patients with severe AD who died with an antemortem clinical diagnosis compared with that of those with mild cognitive impairment (45). Considering the significant changes in CD44 expression and role of CD44 protein, CD44 may be a therapeutic target in AD.
Current research suggests that there are strong inflammatory reactions mediated by resident brain cells and through peripheral immune cells, which infiltrate the brain at various stages of disease progression (40). As the immune environment in the peripheral blood changes, brain tissue metabolism, signaling, and BPs also change. We believe that adverse indicators in PBMCs are a signal of early pathological changes in AD. If long-term immune system abnormalities are found in elderly people, especially in CD4+ T cells, natural killer cells, macrophages, and mast cells, early cognitive screening and intervention for AD are warranted; however, our current study did not determine abnormal cut-off values, and large-sample data comparing normal-aging individuals and patients with AD are necessary for future studies. Although immune abnormalities do not necessarily lead to AD, we speculate that elderly people with long-term immune system abnormalities have a much higher risk of AD than the general older adult population. Treatment of early inflammatory reactions may delay the onset and progression of AD.
According to this study, key genes are potential biomarkers to predict and to diagnose AD. Thus, AD could be diagnosed by testing the patient’s peripheral blood, without brain tissue biopsy or cerebrospinal fluid testing. These genes suggest that AD onset may be associated with the immune pathway; therefore, we must further explore the effects of immunity on AD. We recommend routine blood testing for older adults annually. If peripheral blood immune cell abnormalities are found over time, patients should have cognitive examinations or early interventions. This study may provide noninvasive methods for early identification of AD or possible targets for future AD treatment.
Conclusions
In conclusion, our current study found that the expression of several key genes changes in both the hippocampus and PBMCs of patients with AD, strongly suggesting their utility as disease biomarkers. In addition, immune abnormalities may have an important role in AD pathophysiology. When patients exhibit long-term peripheral blood immune abnormalities, they may be recognized as being at high risk for AD, leading to closer observed medical treatment.
Acknowledgments
We would like to thank Editage (https://www.editage.cn) for English language editing.
Funding: This study was supported by the National Natural Science Foundation of China (grant number: 81771826).
Footnote
Reporting Checklist: The authors have completed the MDAR reporting checklist. Available at https://atm.amegroups.com/article/view/10.21037/atm-21-4974/rc
Conflicts of Interest: All authors have completed the ICMJE uniform disclosure form (available at https://atm.amegroups.com/article/view/10.21037/atm-21-4974/coif). The authors have no conflicts of interest to declare.
Ethical Statement: The authors are accountable for all aspects of the work in ensuring that questions related to the accuracy or integrity of any part of the work are appropriately investigated and resolved. This research did not involve a human subject trial. Instead, the data came exclusively from the GEO. The study was conducted in accordance with the Declaration of Helsinki (as revised in 2013).
Open Access Statement: This is an Open Access article distributed in accordance with the Creative Commons Attribution-NonCommercial-NoDerivs 4.0 International License (CC BY-NC-ND 4.0), which permits the non-commercial replication and distribution of the article with the strict proviso that no changes or edits are made and the original work is properly cited (including links to both the formal publication through the relevant DOI and the license). See: https://creativecommons.org/licenses/by-nc-nd/4.0/.
References
- Isaev NK, Stelmashook EV, Genrikhs EE, et al. Alzheimer's Disease: An Exacerbation of Senile Phenoptosis. Biochemistry (Mosc) 2015;80:1578-81. [Crossref] [PubMed]
- Alzheimer’s Disease International. World Alzheimer Report 2018: The state of the art of dementia research: New frontiers 2018. Available online: https://www.alzint.org/resource/world-alzheimer-report-2018/
- Selkoe DJ. Toward a comprehensive theory for Alzheimer's disease. Hypothesis: Alzheimer's disease is caused by the cerebral accumulation and cytotoxicity of amyloid beta-protein. Ann N Y Acad Sci 2000;924:17-25. [Crossref] [PubMed]
- Atwood CS, Bowen RL. A Unified Hypothesis of Early- and Late-Onset Alzheimer's Disease Pathogenesis. J Alzheimers Dis 2015;47:33-47. [Crossref] [PubMed]
- Gouras GK, Tsai J, Naslund J, et al. Intraneuronal Abeta42 accumulation in human brain. Am J Pathol 2000;156:15-20. [Crossref] [PubMed]
- Pinto TCC, Machado L, Bulgacov TM, et al. Is the Montreal Cognitive Assessment (MoCA) screening superior to the Mini-Mental State Examination (MMSE) in the detection of mild cognitive impairment (MCI) and Alzheimer's Disease (AD) in the elderly? Int Psychogeriatr 2019;31:491-504. [Crossref] [PubMed]
- Hojjati SH, Ebrahimzadeh A, Babajani-Feremi A. Identification of the Early Stage of Alzheimer's Disease Using Structural MRI and Resting-State fMRI. Front Neurol 2019;10:904. [Crossref] [PubMed]
- Gaubert S, Raimondo F, Houot M, et al. EEG evidence of compensatory mechanisms in preclinical Alzheimer's disease. Brain 2019;142:2096-112. [Crossref] [PubMed]
- Yan Y, Somer E, Grau V. Classification of amyloid PET images using novel features for early diagnosis of Alzheimer's disease and mild cognitive impairment conversion. Nucl Med Commun 2019;40:242-8. [Crossref] [PubMed]
- Gupta Y, Lama RK, Kwon GR, et al. Prediction and Classification of Alzheimer's Disease Based on Combined Features From Apolipoprotein-E Genotype, Cerebrospinal Fluid, MR, and FDG-PET Imaging Biomarkers. Front Comput Neurosci 2019;13:72. [Crossref] [PubMed]
- Heneka MT, Carson MJ, El Khoury J, et al. Neuroinflammation in Alzheimer's disease. Lancet Neurol 2015;14:388-405. [Crossref] [PubMed]
- Zenaro E, Pietronigro E, Della Bianca V, et al. Neutrophils promote Alzheimer's disease-like pathology and cognitive decline via LFA-1 integrin. Nat Med 2015;21:880-6. [Crossref] [PubMed]
- Prokop S, Miller KR, Drost N, et al. Impact of peripheral myeloid cells on amyloid-β pathology in Alzheimer's disease-like mice. J Exp Med 2015;212:1811-8. [Crossref] [PubMed]
- Baruch K, Yoles E. Commentary: Chronic PD-1 Checkpoint Blockade Does Not Affect Cognition or Promote Tau Clearance in a Tauopathy Mouse Model. Front Aging Neurosci 2020;12:135. [Crossref] [PubMed]
- Merlini M, Kirabali T, Kulic L, et al. Extravascular CD3+ T Cells in Brains of Alzheimer Disease Patients Correlate with Tau but Not with Amyloid Pathology: An Immunohistochemical Study. Neurodegener Dis 2018;18:49-56. [Crossref] [PubMed]
- Ma G, Liu M, Du K, et al. Differential Expression of mRNAs in the Brain Tissues of Patients with Alzheimer's Disease Based on GEO Expression Profile and Its Clinical Significance. Biomed Res Int 2019;2019:8179145. [Crossref] [PubMed]
- Sood S, Gallagher IJ, Lunnon K, et al. A novel multi-tissue RNA diagnostic of healthy ageing relates to cognitive health status. Genome Biol 2015;16:185. [Crossref] [PubMed]
- Lewczuk P, Riederer P, O'Bryant SE, et al. Cerebrospinal fluid and blood biomarkers for neurodegenerative dementias: An update of the Consensus of the Task Force on Biological Markers in Psychiatry of the World Federation of Societies of Biological Psychiatry. World J Biol Psychiatry 2018;19:244-328. [Crossref] [PubMed]
- Goldsmith DR, Rapaport MH, Miller BJ. A meta-analysis of blood cytokine network alterations in psychiatric patients: comparisons between schizophrenia, bipolar disorder and depression. Mol Psychiatry 2016;21:1696-709. [Crossref] [PubMed]
- Moradi E, Marttinen M, Häkkinen T, et al. Supervised pathway analysis of blood gene expression profiles in Alzheimer's disease. Neurobiol Aging 2019;84:98-108. [Crossref] [PubMed]
- Voyle N, Keohane A, Newhouse S, et al. A Pathway Based Classification Method for Analyzing Gene Expression for Alzheimer's Disease Diagnosis. J Alzheimers Dis 2016;49:659-69. [Crossref] [PubMed]
- Bock JB, Matern HT, Peden AA, et al. A genomic perspective on membrane compartment organization. Nature 2001;409:839-41. [Crossref] [PubMed]
- Somsel Rodman J, Wandinger-Ness A. Rab GTPases coordinate endocytosis. J Cell Sci 2000;113:183-92. [Crossref] [PubMed]
- Horak M, Holubova K, Nepovimova E, et al. The pharmacology of tacrine at N-methyl-d-aspartate receptors. Prog Neuropsychopharmacol Biol Psychiatry 2017;75:54-62. [Crossref] [PubMed]
- Wang R, Reddy PH. Role of Glutamate and NMDA Receptors in Alzheimer's Disease. J Alzheimers Dis 2017;57:1041-8. [Crossref] [PubMed]
- Hallschmid M. Intranasal Insulin for Alzheimer's Disease. CNS Drugs 2021;35:21-37. [Crossref] [PubMed]
- Man HY, Lin JW, Ju WH, et al. Regulation of AMPA receptor-mediated synaptic transmission by clathrin-dependent receptor internalization. Neuron 2000;25:649-62. [Crossref] [PubMed]
- Baskys A, Bayazitov I, Zhu E, et al. Rab-mediated endocytosis: linking neurodegeneration, neuroprotection, and synaptic plasticity? Ann N Y Acad Sci 2007;1122:313-29. [Crossref] [PubMed]
- Wang WW, Han R, He HJ, et al. Delineating the Role of Mitophagy Inducers for Alzheimer Disease Patients. Aging Dis 2021;12:852-67. [Crossref] [PubMed]
- Kerr JS, Adriaanse BA, Greig NH, et al. Mitophagy and Alzheimer's Disease: Cellular and Molecular Mechanisms. Trends Neurosci 2017;40:151-66. [Crossref] [PubMed]
- Yin F, Sancheti H, Patil I, et al. Energy metabolism and inflammation in brain aging and Alzheimer's disease. Free Radic Biol Med 2016;100:108-22. [Crossref] [PubMed]
- Ryu MS, Zhang D, Protchenko O, et al. PCBP1 and NCOA4 regulate erythroid iron storage and heme biosynthesis. J Clin Invest 2017;127:1786-97. [Crossref] [PubMed]
- Garcia-Esparcia P, Sideris-Lampretsas G, Hernandez-Ortega K, et al. Altered mechanisms of protein synthesis in frontal cortex in Alzheimer disease and a mouse model. Am J Neurodegener Dis 2017;6:15-25. [PubMed]
- Wong YC, Ysselstein D, Krainc D. Mitochondria-lysosome contacts regulate mitochondrial fission via RAB7 GTP hydrolysis. Nature 2018;554:382-6. [Crossref] [PubMed]
- Lunnon K, Ibrahim Z, Proitsi P, et al. Mitochondrial dysfunction and immune activation are detectable in early Alzheimer's disease blood. J Alzheimers Dis 2012;30:685-710. [Crossref] [PubMed]
- Tao Y, Han Y, Yu L, et al. The Predicted Key Molecules, Functions, and Pathways That Bridge Mild Cognitive Impairment (MCI) and Alzheimer's Disease (AD). Front Neurol 2020;11:233. [Crossref] [PubMed]
- Di Paolo G, Kim TW. Linking lipids to Alzheimer's disease: cholesterol and beyond. Nat Rev Neurosci 2011;12:284-96. Erratum in: Nat Rev Neurosci 2011;12:484. [Crossref] [PubMed]
- Pilliod J, Desjardins A, Pernègre C, et al. Clearance of intracellular tau protein from neuronal cells via VAMP8-induced secretion. J Biol Chem 2020;295:17827-41. [Crossref] [PubMed]
- Marlatt M, Lee HG, Perry G, et al. Sources and mechanisms of cytoplasmic oxidative damage in Alzheimer's disease. Acta Neurobiol Exp (Wars) 2004;64:81-7. [PubMed]
- Olsen I, Singhrao SK. Inflammasome Involvement in Alzheimer's Disease. J Alzheimers Dis 2016;54:45-53. [Crossref] [PubMed]
- Johnson P, Ruffell B. CD44 and its role in inflammation and inflammatory diseases. Inflamm Allergy Drug Targets 2009;8:208-20. [Crossref] [PubMed]
- Tan ZS, Beiser AS, Vasan RS, et al. Inflammatory markers and the risk of Alzheimer disease: the Framingham Study. Neurology 2007;68:1902-8. [Crossref] [PubMed]
- Uberti D, Cenini G, Bonini SA, et al. Increased CD44 gene expression in lymphocytes derived from Alzheimer disease patients. Neurodegener Dis 2010;7:143-7. [Crossref] [PubMed]
- Pinner E, Gruper Y, Ben Zimra M, et al. CD44 Splice Variants as Potential Players in Alzheimer's Disease Pathology. J Alzheimers Dis 2017;58:1137-49. [Crossref] [PubMed]
- Moreno-Rodriguez M, Perez SE, Nadeem M, et al. Frontal cortex chitinase and pentraxin neuroinflammatory alterations during the progression of Alzheimer's disease. J Neuroinflammation 2020;17:58. [Crossref] [PubMed]