Analysis of the tumor immune environment identifies an immune gene set–based prognostic signature in non-small cell lung cancer
Introduction
Lung cancer is the leading cause of cancer-related morbidity and mortality, with non-small cell lung cancer (NSCLC) as the most prevalent form (1). Despite recent advances in lung cancer diagnosis and treatment, the survival time for patients with lung cancer is still short, and the majority of patients present with advanced disease (2). Predicting which patients are at high risk and investigating potential therapeutic targets are therefore necessary to promote the overall survival.
The immune system plays a key role in the monitoring and destruction of cancer cells; however, tumor cells can bypass this natural defense, and tolerance can form during disease progression (3). It has long been proposed that tumor immune environments are associated with tumor initiation and can influence therapeutic response (4). Tumor-reprogrammed immune cells are involved in both tumor-promoting and tumor-suppressing activities, which may predict clinical outcome (5). In this regard, immunotherapy focus on restoring the anti-tumor host immunity could promote occult cancerous cells destruction. Furthermore, patients who response to immunotherapeutic intervention would have better survival rates (6). Recently, immunotherapy has been recommended as the first-line therapy for patients with advanced lung cancer (7). However, the majority of patients with lung cancer do not benefit from this treatment, and so a deeper understanding of tumor immune environments is needed.
Gene expression profiling can reflect the interactions between tumor and immune cells and provide clues for finding predictive biomarkers and new targets (8). To this end, we calculated differentially expressed genes between tumor and normal tissues to uncover potential important players in tumor immune environments. An immune-related gene signature was then established to predict the prognosis of patients with lung cancer. Additionally, we described immune cell infiltration patterns in NSCLC. In this study, we aimed to provide the comprehensive description of immune characteristics of lung cancer, offering reference information for improving NSCLC treatments. We present the following article in accordance with the TRIPOD reporting checklist (available at https://dx.doi.org/10.21037/atm-21-6043).
Methods
Data acquisition and processing
The gene expression data of 497 lung adenocarcinoma tumor tissue samples and 54 normal lung tissue samples and the corresponding clinical information were acquired from The Cancer Genome Atlas (TCGA) database. A Wilcoxon test was used to analyze differentially expressed genes in the TCGA sample using the “limma” package in R (The R Foundation for Statistical Computing, Vienna, Austria). To identify differentially expressed genes in lung cancer, the cutoff threshold in TCGA was set as |log2 fold change (FC)| ≥1.0, and the false discovery rate (FDR) was set at <0.01. Immune-related genes and transcription factors (TFs) were extracted from the above-mentioned differentially expressed genes and analyzed for differential expression using the “limma” package. The corresponding clinical information of the patients with lung cancer was collected and used for the subsequent analyses. The external validation cohort consisted of the expression data and the comparative clinical data of 45 patients with squamous cell carcinoma, acquired from the Gene Expression Omnibus (GEO) database (GSE50081, n=181). The study was conducted in accordance with the Declaration of Helsinki (as revised in 2013).
Co‑expression network construction and functional enrichment analyses
A network of immune-related genes and TFs was created using Cytoscape v3.7.1 (Cytoscape Consortium, San Diego, CA, USA). Upregulated genes were represented by red circles, downregulated genes were represented by green ellipses, and TFs were represented by triangles. To identify the biological function of differentially expressed immune-related genes, we used the “clusterProfiler” package in R to perform Gene Ontology (GO) analyses. The functional enrichment of upregulated immune-related genes was represented by red bar graphs, and the functional enrichment of downregulated immune-related genes was represented by blue bar graphs.
Prognostic model construction
We used the “survival” package in R to perform a univariate Cox regression analysis for all differentially expressed immune-related genes and screened for significant candidate genes. Subsequently, based on the screened candidate genes, a prognostic model containing 12 immune-related genes was constructed, combining the effects of each gene. The coefficients of each gene were determined by multivariable Cox regression model. The risk score for each patient was determined using the following formula: risk score = Exp1 × Coe1 + Exp2 × Coe2 +……+ Expi × Coei.
According to the median value of the risk score, the patients with lung cancer were classified into high-risk and low-risk groups. Kaplan-Meier analysis was used to construct a survival curve. Then, a logrank test was applied to compare the overall survival (OS) of the 2 subgroups. Subsequently, a receiver operating characteristic (ROC) curve was drawn to evaluate the performance of the prognostic model using the “survivalROC” package in R. The correlation between the immune-related genes that constituted the prognostic model and various clinical factors were analyzed using the “beeswarm” package in R.
Quantitative real-time polymerase chain reaction (qRT-PCR)
Total RNA was extracted using TRIZOL (Invitrogen Inc., USA), and subjected to qRT-PCR using the following primers: VIPR1 (TCATCCGAATCCTGCTTCAGA; AGGCGAACATGATGTAGTGTACT), S100A16 (ATGTCAGACTGCTACACGGAG; GTTCTTGACCAGGCTGTACTTAG), GAPDH (GGCTCATGACCACAGTCCAT; GACGGACACATTGGGGGTAG). Gene expression was normalized to the level of GAPDH within each sample using the relative ∆∆Ct method.
Unsupervised clustering and analysis of immune cell-related genes
The abundance of tumor infiltrating immune cells was calculated using the CIBERSORT website (https://cibersort.stanford.edu/). Based on the abundance of immune cell infiltration provided by CIBERSORT, we used the “ConsensusClusterPlus” package in R to perform unsupervised clustering of the patients with lung cancer. We then visualized programmed cell death protein 1 (PD-1) infiltration between the 2 cluster subgroups with the “ggplot2” and “ggpubr” packages in R.
Statistical analysis
We used Kaplan-Meier analysis to construct survival curves using the “survival” and “survminer” packages in R and a log rank test to evaluate the significance of differences between the 2 subgroups. Wilcoxon signed-rank tests were performed to explore quantitative variables. Significance was defined as P<0.05. All statistical analyses were performed using R version 4.0.3.
Results
Differentially expressed immune genes and TFs in lung cancer
We used data from the TCGA dataset to calculate differentially expressed immune genes between tumor and normal tissues. A total of 489 differentially expressed genes were identified, in which 321 genes were upregulated in tumor tissues (Figure 1A,1B). Differentially expressed TFs were then selected (Figure 1C,1D), and a network of TFs and genes was constructed (Figure 1E). Among TFs, regulatory molecules enhancer of zeste homolog 2 (EZH2) (9) and transcription factor 21 (TCF21) (10) are involved in the immunosuppressive microenvironment, and chromobox homolog (CBX7) (11) is associated with lung carcinogenesis. GO analysis was carried out to explore the potential functions of these differentially expressed genes (Figure 2). For upregulated genes in tumors, immunoglobulin complex, humoral immune response, complement activation, and lymphocyte-mediated immunity were the most populated subcategories, indicating that the immune system plays a key role in tumor progress. For downregulated genes, signaling receptor activator activity, receptor ligand activity, and cell chemotaxis were the most abundant activities, indicating that homeostasis-related terms are disrupted in the tumor microenvironment and potentially robust immunity may be suppressed. Our enrichment analysis results were consistent with pathological changes in lung cancer.
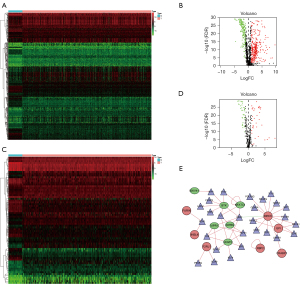
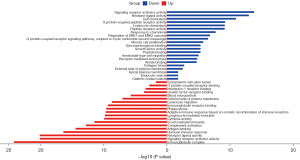
Immune signature for lung cancer prognosis
Nineteen significant prognostic immune genes were identified using a univariate Cox regression analysis with P<0.05 (Figure 3). Related genes then underwent a Cox proportional hazards regression to generate the best gene model. Finally, a gene model with 12 immune genes (Figure S1) was created. We calculated the risk score for each patient according to the coefficient value of the 12 genes. Subsequently, the patients were divided into high-risk and low-risk groups based on the median risk score. A longer survival time was found in the low-risk patients when compared with that of the high-risk patients (Figure 4A), and the risk score distribution between the 2 groups was shown in Figure 4B. To further evaluate the prognostic accuracy of the risk scores, time-dependent ROC curves were plotted (Figure 4C), yielding an area under the curve (AUC) of 0.733. To investigate the stability of our model, we assessed the prognostic value of the immune signature in the GEO database. The patients in the GEO cohort were similarly divided into a high-risk group (n=90) and a low-risk group (n=91). The survival of the patients in the low-risk group was significantly better than that of the patients in the high-risk group (P<0.001; Figure S2). Furthermore, we validated the representative genes expression in 8 matched pairs of primary lung tumors and the adjacent normal samples. Consistent with the previous findings, the protective gene vasoactive intestinal peptide receptor 1 (VIPR1) showed decreased expression level in tumors compared with adjacent normal samples, while the risky gene S100A16 expression was up-regulated in tumors (Figure S3).
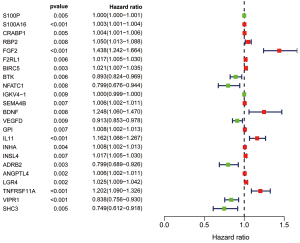
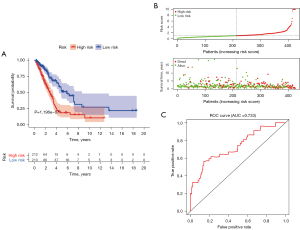
Association with clinicopathologic factors
A univariate Cox regression analysis was conducted using the risk score and various clinical characteristics. The analysis showed that clinical stage, degree of tissue involvement (T), lymphatic involvement (N), tumor metastasis (M), and risk score were correlated with the OS of patients (Figure 5A). A subsequent multivariate Cox regression analysis showed that only the risk score and the stage were associated with the OS of patients with lung cancer (P<0.05; Figure 5B), proving the accuracy of the risk score in predicting survival outcomes. In addition, we assessed the relationship between the genes in our model and clinicopathologic factors. We found that genes related to poor survival, such as S100A16 and angiopoietin-like 4 (ANGPTL4), were significantly associated with T, N, and stage, and that the expression of higher-risk genes was correlated with more malignant lung cancer. In contrast, increased expression of the protective gene VIPR1 was correlated with a decreased degree of malignancy (N; Figure 6).
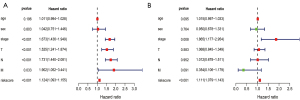
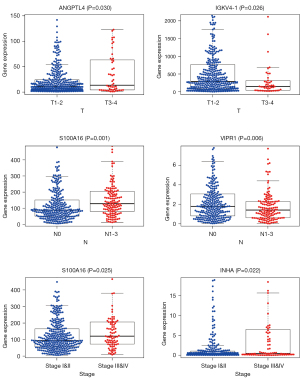
Immune cell infiltration patterns
To illustrate the immune cell infiltration patterns in tumors, we used a gene expression-based approach to estimate the abundances of specific cell types. As shown in Figure 7A, most immune cell populations exhibited a positive correlation without any anticorrelation, indicating the reliability of the gene sets. Unsupervised clustering showed that the tumor samples were predominantly separated into 2 clusters; more memory B cells, mast cells, and activated dendritic cells were found in cluster A, while more CD8 T cells, Treg T cells, resting natural killer (NK) cells, and M0 macrophages (an intermediate macrophages with differentiative ability) were found in cluster B (Figure 7B). The patients in cluster A had better OS compared with that of the patients in cluster B (Figure 7C) and lower PD-1 expression (Figure 7D).
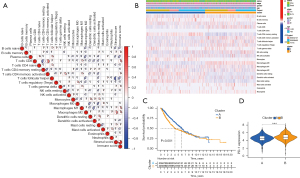
Discussion
The immune environment plays a critical role in tumor initiation and therapeutic response (4). Lung cancer typically harbors extensive genomic alterations and is responsive to immunotherapy (12). Immune cells influence patient survival and the effectiveness of treatments. Previous studies have shown that levels of tumor-infiltrating lymphocytes are significantly correlated with prognosis in NSCLC (13). The neutrophil-to-lymphocyte ratio has also been recognized as an indicator of poor prognosis (14). Furthermore, research has found that lung cancer exhibits increased inflammatory gene transcripts and that circulating T cell subpopulations can predict immune responses (15). Traditional methods to assess immune cell infiltration mainly rely on immunohistochemistry, but the number of cell types and the characteristics of each immune cell are limited from section staining, confined by the quantity and quality of antibodies. The gene expression profile of bulk tumors provides more information in this regard. The immune environment, including immune cells and proteins expressed by immune-related genes, has a significant impact on predicting the clinical outcomes of cancers (16). Furthermore, gene expression profiling of lung cancer offers useful information for cancer diagnosis, prognosis as well as new therapy development (17). Research has shown that more than 3,000 protein-coding genes change in NSCLC after tumor resection, especially genes in pathways associated with the innate immune response suppression (18). In this study, we collected differentially expressed immune genes between tumor and normal tissues and constructed a prediction model based on prognostic genes. In our model, the patients in the high-risk group had a poor prognosis with shorter survival time.
According to previous studies, the genes used in our risk prediction model are tightly associated with lung cancer prognosis. Some of the risk genes in our model participate in lung cancer progression. Higher S100A16 messenger RNA (mRNA) expression in lung cancer is significantly correlated with poor survival (19). Retinol binding protein 2 (RBP2) performs an oncogenic function in lung tumorigenesis and progression (20) and induces epithelial-mesenchymal transition (21). Fibroblast growth factor 2 (FGF2) promotes lung cancer cell proliferation (22), and some clinical trials have evaluated the efficacy of its inhibitors on lung cancer treatment (23). In addition, interleukin 11 (IL11) acts on leukocytes to induce pro-tumorigenic and pro-metastatic neutrophils (24). In contrast, some of the tumor suppressor genes of our model, such as VIPR1, have been shown to have protective effects (25). Research has shown that VIPR1 significantly inhibits the growth, migration, and invasion of lung cancer cells (26). Moreover, VIPR1 expression is decreased in metastatic lung cancer compared with that in non-metastatic lung cancer (27).
In our study, we described the immune cell infiltration patterns in lung cancer and classified patients into 2 groups based on immune cell population. The patients in cluster B had worse OS with more M0 macrophages and Treg cells. It has been reported that M0 macrophages are associated with decreased survival (28). Treg cells, as the most notable immunosuppressive cells, can help cancer cells escape from immunological surveillance, and therapies based on Treg blockade have benefited a group of patients with lung cancer (29). The patients in cluster A exhibited more infiltration of B cells, dendritic cells, and mast cells. Consistent with previous studies, the high infiltration of B cells, dendritic cells, and mast cells indicated a favorable prognosis (30). Furthermore, the expression of PD-1 was higher in cluster B. PD-1 is an inhibitory checkpoint molecule which can inhibit T cell activation. Immune checkpoint blockade therapy targeting PD-1 has yielded promising clinical responses in patients with lung cancer (31). Therefore, we propose that the patients in cluster B would be more likely to benefit from immunotherapy.
In recent years, increasing attention has been paid to tumor immune microenvironments, and immune prognostic signatures have been constructed for various cancer types. For NSCLC, a gene model consisting of 30 immune-related genes has been shown to have a strong association with recurrence and clinical stage (32). Immune-associated risk score prognostic models have also been established (33), with an AUC of 0.702 and 0.723, respectively. Our prognostic model showed a similar true positive rate compared with that of previous studies. In addition to an immune gene signature, we established a connection between genes and TFs and the related pathway. We also selected representative genes of different immune cells to identify immune cell infiltration patterns and pave a path to better targeting of prognosis-related immune cell subsets. Our retrospective analysis provides comprehensive information about lung cancer immune environments, although further studies are required to confirm our findings.
Conclusions
In summary, we constructed a risk score prognostic model based on 12 immune-related genes. The survival analysis revealed that a high-risk score was significantly associated with poor survival outcomes. In addition, we described the immune cell infiltration patterns and found that high infiltration of B cells, dendritic cells, and mast cells indicated a favorable prognosis.
Acknowledgments
Funding: None.
Footnote
Reporting Checklist: The authors have completed the TRIPOD reporting checklist. Available at https://dx.doi.org/10.21037/atm-21-6043
Conflicts of Interest: All authors have completed the ICMJE uniform disclosure form (available at https://dx.doi.org/10.21037/atm-21-6043). The authors have no conflicts of interest to declare.
Ethical Statement: The authors are accountable for all aspects of the work in ensuring that questions related to the accuracy or integrity of any part of the work are appropriately investigated and resolved. The study was conducted in accordance with the Declaration of Helsinki (as revised in 2013).
Open Access Statement: This is an Open Access article distributed in accordance with the Creative Commons Attribution-NonCommercial-NoDerivs 4.0 International License (CC BY-NC-ND 4.0), which permits the non-commercial replication and distribution of the article with the strict proviso that no changes or edits are made and the original work is properly cited (including links to both the formal publication through the relevant DOI and the license). See: https://creativecommons.org/licenses/by-nc-nd/4.0/.
References
- Bray F, Ferlay J, Soerjomataram I, et al. Global cancer statistics 2018: GLOBOCAN estimates of incidence and mortality worldwide for 36 cancers in 185 countries. CA Cancer J Clin 2018;68:394-424. [Crossref] [PubMed]
- Temel JS, Greer JA, Muzikansky A, et al. Early palliative care for patients with metastatic non-small-cell lung cancer. N Engl J Med 2010;363:733-42. [Crossref] [PubMed]
- Bodor JN, Boumber Y, Borghaei H. Biomarkers for immune checkpoint inhibition in non-small cell lung cancer (NSCLC). Cancer 2020;126:260-70. [Crossref] [PubMed]
- Binnewies M, Roberts EW, Kersten K, et al. Understanding the tumor immune microenvironment (TIME) for effective therapy. Nat Med 2018;24:541-50. [Crossref] [PubMed]
- Altorki NK, Markowitz GJ, Gao D, et al. The lung microenvironment: an important regulator of tumour growth and metastasis. Nat Rev Cancer 2019;19:9-31. [Crossref] [PubMed]
- Saab S, Zalzale H, Rahal Z, et al. Insights Into Lung Cancer Immune-Based Biology, Prevention, and Treatment. Front Immunol 2020;11:159. [Crossref] [PubMed]
- Reck M, Rodríguez-Abreu D, Robinson AG, et al. Updated Analysis of KEYNOTE-024: Pembrolizumab Versus Platinum-Based Chemotherapy for Advanced Non-Small-Cell Lung Cancer With PD-L1 Tumor Proportion Score of 50% or Greater. J Clin Oncol 2019;37:537-46. [Crossref] [PubMed]
- Gibellini L, De Biasi S, Porta C, et al. Single-Cell Approaches to Profile the Response to Immune Checkpoint Inhibitors. Front Immunol 2020;11:490. [Crossref] [PubMed]
- Zhao Y, Wang XX, Wu W, et al. EZH2 regulates PD-L1 expression via HIF-1α in non-small cell lung cancer cells. Biochem Biophys Res Commun 2019;517:201-9. [Crossref] [PubMed]
- Wu H, Zhou J, Zeng C, et al. Curcumin increases exosomal TCF21 thus suppressing exosome-induced lung cancer. Oncotarget 2016;7:87081-90. [Crossref] [PubMed]
- Forzati F, Federico A, Pallante P, et al. Tumor suppressor activity of CBX7 in lung carcinogenesis. Cell Cycle 2012;11:1888-91. [Crossref] [PubMed]
- Guo X, Zhang Y, Zheng L, et al. Global characterization of T cells in non-small-cell lung cancer by single-cell sequencing. Nat Med 2018;24:978-85. [Crossref] [PubMed]
- Zeng DQ, Yu YF, Ou QY, et al. Prognostic and predictive value of tumor-infiltrating lymphocytes for clinical therapeutic research in patients with non-small cell lung cancer. Oncotarget 2016;7:13765-81. [Crossref] [PubMed]
- Nakaya A, Kurata T, Yoshioka H, et al. Neutrophil-to-lymphocyte ratio as an early marker of outcomes in patients with advanced non-small-cell lung cancer treated with nivolumab. Int J Clin Oncol 2018;23:634-40. [Crossref] [PubMed]
- Manjarrez-Orduño N, Menard LC, Kansal S, et al. Circulating T Cell Subpopulations Correlate With Immune Responses at the Tumor Site and Clinical Response to PD1 Inhibition in Non-Small Cell Lung Cancer. Front Immunol 2018;9:1613. [Crossref] [PubMed]
- Lu Y, Zhou X, Liu Z, et al. Assessment for Risk Status of Colorectal Cancer Patients: A Novel Prediction Model Based on Immune-Related Genes. DNA Cell Biol 2020;39:958-64. [Crossref] [PubMed]
- Wang L, Luo X, Cheng C, et al. A gene expression-based immune signature for lung adenocarcinoma prognosis. Cancer Immunol Immunother 2020;69:1881-90. [Crossref] [PubMed]
- Kossenkov AV, Vachani A, Chang C, et al. Resection of non-small cell lung cancers reverses tumor-induced gene expression changes in the peripheral immune system. Clin Cancer Res 2011;17:5867-77. [Crossref] [PubMed]
- Kobayashi M, Nagashio R, Saito K, et al. Prognostic significance of S100A16 subcellular localization in lung adenocarcinoma. Hum Pathol 2018;74:148-55. [Crossref] [PubMed]
- Teng YC, Lee CF, Li YS, et al. Histone demethylase RBP2 promotes lung tumorigenesis and cancer metastasis. Cancer Res 2013;73:4711-21. [Crossref] [PubMed]
- Wang S, Wang Y, Wu H, et al. RBP2 induces epithelial-mesenchymal transition in non-small cell lung cancer. PLoS One 2013;8:e84735. [Crossref] [PubMed]
- Hamamoto J, Yasuda H, Nonaka Y, et al. The FGF2 aptamer inhibits the growth of FGF2-FGFR pathway driven lung cancer cells. Biochem Biophys Res Commun 2018;503:1330-4. [Crossref] [PubMed]
- Jones RL, Ratain MJ, O'Dwyer PJ, et al. Phase II randomised discontinuation trial of brivanib in patients with advanced solid tumours. Eur J Cancer 2019;120:132-9. [Crossref] [PubMed]
- Janiszewska M, Tabassum DP, Castaño Z, et al. Subclonal cooperation drives metastasis by modulating local and systemic immune microenvironments. Nat Cell Biol 2019;21:879-88. [Crossref] [PubMed]
- Mlakar V, Strazisar M, Sok M, et al. Oligonucleotide DNA microarray profiling of lung adenocarcinoma revealed significant downregulation and deletions of vasoactive intestinal peptide receptor 1. Cancer Invest 2010;28:487-94. [Crossref] [PubMed]
- Zhao L, Yu Z, Zhao B. Mechanism of VIPR1 gene regulating human lung adenocarcinoma H1299 cells. Med Oncol 2019;36:91. [Crossref] [PubMed]
- Sun L, Zhang Z, Yao Y, et al. Analysis of expression differences of immune genes in non-small cell lung cancer based on TCGA and ImmPort data sets and the application of a prognostic model. Ann Transl Med 2020;8:550. [Crossref] [PubMed]
- Liu X, Wu S, Yang Y, et al. The prognostic landscape of tumor-infiltrating immune cell and immunomodulators in lung cancer. Biomed Pharmacother 2017;95:55-61. [Crossref] [PubMed]
- Xie M, Wei J, Xu J. Inducers, Attractors and Modulators of CD4+ Treg Cells in Non-Small-Cell Lung Cancer. Front Immunol 2020;11:676. [Crossref] [PubMed]
- Zuo S, Wei M, Wang S, et al. Pan-Cancer Analysis of Immune Cell Infiltration Identifies a Prognostic Immune-Cell Characteristic Score (ICCS) in Lung Adenocarcinoma. Front Immunol 2020;11:1218. [Crossref] [PubMed]
- Liu X, Cho WC. Precision medicine in immune checkpoint blockade therapy for non-small cell lung cancer. Clin Transl Med 2017;6:7. [Crossref] [PubMed]
- Song Q, Shang J, Yang Z, et al. Identification of an immune signature predicting prognosis risk of patients in lung adenocarcinoma. J Transl Med 2019;17:70. [Crossref] [PubMed]
- Wang Z, Chen X. Establishment and validation of an immune-associated signature in lung adenocarcinoma. Int Immunopharmacol 2020;88:106867. [Crossref] [PubMed]
(English Language Editor: C. Gourlay)