Systemic characterization of alternative splicing related to prognosis, immune infiltration, and drug sensitivity analysis in ovarian cancer
Introduction
Ovarian cancer is the seventh most common female cancer in the world, and epithelial ovarian cancer is the most dominant pathological subtype (1). Almost half of all patients with ovarian cancer are diagnosed over the age of 65, and this age group accounts for over 70% of deaths (2). Data from national databases, including the Surveillance, Epidemiology, and End Results (SEER) program, illustrate racial/ethnic disparities in risk and survival of epithelial ovarian cancer: compared with other races/ethnicity, white women have a higher incidence, while African American women have worse survival rates (3). In China, a rising occurrence and an improving survival trend could be seen in invasive epithelial ovarian carcinoma over the period from 1997 to 2006 (4). Usually, if ovarian cancer is found at an advanced stage, the overall 5-year survival rate is approximately 30–40% (5). Studies have shown that the alternative splicing (AS) events of some specific genes can affect the growth, invasion, and other aspects of ovarian cancer cells (6-9), and thereby promote or inhibit the occurrence of ovarian cancer. Although a large number of studies based on messenger ribonucleic acid (mRNA) or long noncoding ribonucleic acid (lncRNA) (10) have attempted to establish a prognostic model for ovarian serous cystadenocarcinoma (OV), a prognostic model based on AS provides more significant potential.
AS refers to a post-transcriptional processing of mRNA. Pre-mRNA can generate different types of mRNA spliceosomes through splicing at different sites, which markedly increases the diversity of proteins. About 95% of genes have been reported to undergo AS (11). AS not only plays a normal physiological role in the body (12), but also participates in the occurrence and progression of various malignant tumors (13-15). Increasing evidence suggests that AS is involved in carcinogenic processes, including proliferation, metastasis, hypoxia, apoptosis, drug resistance, and immune escape, thus influencing the prognosis of patients (15). For instance, some splicing factors, like RNA binding fox-1 homolog 2 (RBFox2), have been linked to epithelial-mesenchymal transition which related to invasion and metastasis in cancer (15). Moreover, the families of protein domains, which are often mutated in tumors, can be affected by some AS changes, thereby disrupting protein-protein interactions (PPIs) in cancer-related pathways (14). In addition, the selection of isotypes is regulated by a variety of factors, including signaling molecules, kinases, splicing factors (SFs), etc., which usually regulate AS events in a synergistic manner (15). AS events have been originally described as prognostic biomarkers for pancreatic cancer (16), colorectal cancer (17), thyroid cancer (18), and other tumors. However, few reports have focused on the likelihood of differentially-expressed AS events as a biomarker for forecasting the prognosis of OV (19). Therefore, we planned to construct a novel prognostic model based on AS events in OV and explore the relationship between AS events and AS-related molecules that included but not limited to SFs.
Human cancers are capable of utilizing aberrant AS to develop, grow, and progress into therapy-resistant tumors (20). Previous studies have shown that abnormal AS events can affect the outcome of platinum therapy for ovarian cancer. For example, osteopontin (OPN) is upregulated in several types of tumors and has been associated with chemoresistance, and the overexpression of its OPN-c isoform resulted in the increase of cisplatin sensitivity (21). Hence, comparing with other reports that focused on small-scale AS events, we intended to perform large-scale Genomics of Drug Sensitivity analysis to find some potential therapeutic targets which were concerned with platinum sensitivity. Recently, the significance of AS on tumor immunity has been gradually and extensively supported, and the independent oncogenic effects, which could be related to the suppressive immune microenvironment in cancers, have verified by further investigations of AS events (22,23). Moreover, an increasing number of studies have shown that the dysregulation of AS in tumors can affect the tumor microenvironment (24,25), but few studies have systematically revealed the correlation between AS events and OV cancer immunity. At present, several prognostic signatures derived from AS events have been identified in OV (19). However, to our knowledge, studies on immune-related AS events in OV are lacking. Therefore, we decided to perform immune cell infiltration analysis to explore the effect of AS on tumor microenvironment.
With the advancement of high throughput technology and the rapid development of genomics, The Cancer Genome Atlas (TCGA) project provides us with a powerful means to study multi-omics events. Based on the RNA sequencing (RNA-seq) data and clinical data provided by TCGA, this study first applied the SpliceSeq tool to carry out data mining on AS events in OV and constructed a prognostic model for OV patients. Secondly, the prognostic model was integrated with platinum sensitivity analysis to provide ideas for platinum-based drug therapy. Next, the prognostic SF-AS network was constructed using Cytoscape (26). In addition, we used a machine learning algorithm to classify OV patients into three subtypes and further evaluated the relationship between these subtypes and prognosis, immune cell infiltration, etc. Simultaneously, this study systematically revealed AS events in OV and provided a large number of new targets and ideas for cancer treatment. We present the following article in accordance with the REMARK reporting checklist (available at https://atm.amegroups.com/article/view/10.21037/atm-21-6422/rc).
Methods
Data curation process
The study was conducted in accordance with the Declaration of Helsinki (as revised in 2013).
We downloaded the RNA-seq data and corresponding clinical information of the OV cohort from TCGA data portal (https://portal.gdc.cancer.gov) (27). Next, we downloaded AS profiles related to TCGA patients from TCGASpliceSeq portal (https://bioinformatics.mdanderson.org/TCGASpliceSeq) (28). Spliceseq divided AS into seven types: Exon Skip (ES), Mutually Exclusive Exons (ME), Retained Intron (RI), Alternate Promoter (AP), Alternate Terminator (AT), Alternate Donor site (AD), and Alternate Acceptor site (AA), and quantitatively indicates the probability of occurrence of each AS event with the Percent Spliced In (PSI) value from 0 to 1 (29). To generate as reliable a set of AS events as possible, we implemented a series of stringent filters: percent with PSI value =75%; minimum PSI range =0; and minimum PSI standard deviation =0. For TCGA clinical data, patients with disease-free survival (DFS) >30 days were retained and integrated with the AS profiles (Table S1). Due to the existence of null PSI values of AS profiles, we used the multiple interpolation method provided by “mice” R package (30) to complete the null value. Finally, 318 ovarian cancer samples and 48,049 AS events were selected. The detailed design process of this study is shown in Figure 1.
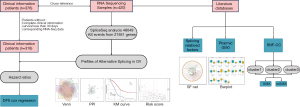
Survival analysis and construction of the prognostic models
For each AS event, patients were divided into two groups based on the median PSI value cut-off. To identity the relationship between AS events and DFS, we performed univariate Cox regression analysis for the seven types of AS events using the “survival” R package. A P value <0.05 was considered to indicate that an AS event was related to DFS. Next, we used the “STRINGdb” R package to draw the PPI network diagram of the seven kinds of 30×6+14 AS events (there are only 14 ME events) that were most significantly related to DFS. In order to eliminate any prognostic AS events that may not be independent factors, we further performed multivariate Cox regression analysis for the seven types of survival-related AS events (31). As a result, seven different types of independent prognostic AS events were combined to construct a final prognostic predictor model. To verify the prediction effect of these models, we first used the “survminer” R package to plot the Kaplan-Meier survival curves of seven diverse AS types as well as the combination of all types. The “plotROC” R package was then used to plot the receiver operating characteristic (ROC) curve to test the accuracy of the prognostic model of each AS type. Finally, we calculated the risk score of each OV patient according to the following formula:
Subsequently, we constructed a risk score model of prognostic AS events (32), and drew a heat map and a profile of the survival-death status combined with a risk score for each sample.
Platinum sensitivity analysis of prognostic factors
All of the AS prognostic factors that had been constructed in the prognostic model of ovarian cancer were selected for platinum sensitivity analysis. We obtained the drug sensitivity data from the CellMiner database (https://discover.nci.nih.gov/cellminer/home.do) established by the National Cancer Institute (NCI) (33). This database is based on the NCI-60 cell line, which is currently the most extensively used group of cancer cell samples for testing cancer drugs. According to previous studies, platinum-based drugs are used widely for the treatment of high-grade serous ovarian cancer (34). In order to analyse the platinum sensitivity of OV, we selected three platinum-based drugs (cisplatin, carboplatin, and oxaliplatin), which are clinically tested and Food and Drug Administration (FDA) approved.
Next, correlation analysis was performed on the RNA expression level of each AS event in the prognostic model and the sensitivity data of each drug (Spearman method, P<0.05). We visualized the correlation analysis results by drawing a total of 13 scatter plots. Finally, according to the expression level of the AS prognostic factors, boxplots of the difference in platinum sensitivity between two groups divided into high and low expression levels were drawn.
Construction of splicing correlation network
According to previous research, the regulatory mechanism of AS events involves the interaction of SF with its target RNA elements (35), while other proteins that do not directly bind RNA, such as kinases, transcription factors and histone-modifying enzymes, have also been shown to regulate AS (36-38). Consequently, MiasDB (http://47.88.84.236/Miasdb), a database of molecular interactions related to AS (39), was chosen to establish the network of the correlation between the PSI value of AS events and the expression of prognostic AS-related molecules. This database has collected 173 human AS events to conduct 938 human interactions between SFs, transcription factors, RNA elements, modified histones, and splicing-associated kinases, which are divided into SFs-RNA elements interactions (SF-RNA) and PPIs (39).
Firstly, Cox regression analysis was performed on AS-related molecules selected from MiasDB to screen the AS-related molecules associated with survival. Next, we separately correlated the expression level of these survival and AS-related molecules with the PSI value of all survival-related AS events (Spearman method, P<0.05). Furthermore, the AS correlation network graph was constructed using Cytoscape. Finally, we used the “clusterProfiler” R package for Gene Ontology (GO) enrichment to analyse the effects of these splicing network-related genes on biological processes (40).
Similar network fusion and consensus clustering (SNF-CC) for cancer subtypes
A modified clustering method, SNF-CC, which links similar network fusion (41) with consensus clustering (42), was applied to classify all OV patients. The “CancerSubtypes” R package, a software package for cancer classification, uses multi-omics data to identify cancer subtypes and provides a series of standard frameworks, including data preprocessing, feature selection, cancer subtype identification, result validation, and visualization (43). Based on the feature selection of Cox regression analysis, we selected all prognostic AS events to classify the molecular subtypes of ovarian cancer. To verify the results, we used the consensus heatmap and cumulative distribution function (CDF) to select a more appropriate number of clusters. Survival analysis based on the number of clustering candidates was also performed to select the number of clusters, and clusters with obvious survival differences were considered as the final OV subtypes. In addition, silhouette width, an effect indicator that signifies that the sample is well matched if the value is high, was used to measure the matching degree of the samples with the identified subtypes (43).
Gene Set Enrichment Analysis (GSEA) and single-sample GSEA (ssGSEA) for cancer subtypes
The intratumoral microenvironment is a complex that includes tumor and non-tumor cells, such as immune cells (44), and immune cells can regulate the occurrence and development of OV (45). By comparing with the IMMPORT database (https://www.immport.org/shared/home) (46), we found that some genes that were in the splicing correlation network, such as tumor protein, translationally-controlled 1 (TPT1), had immune functions. Therefore, we wanted to further analyze the relationship between the immune system and the identified three subtypes. First, we used the GSEA method to examine the differences in the biological pathway of the TPT1 gene in these three subtypes (47). Gene Ontology (GO) gene sets (C5) were selected as GSEA gene sets. Next, using the “GSVA” R package (48), the ssGSEA method was applied to score the OV subtypes for immune cell infiltration. SsGSEA is used to assess the enrichment score of a gene set in each single sample. It can obtain a numerical matrix, which contains the enrichment scores of diverse immune cells in all OV samples (49). The characteristic gene set of immune cells we selected was derived from the study of Charoentong et al. (50), which involved in 28 immune cell subsets, including the main cell types related to adaptive and innate immunity. Finally, in order to explore the influence of immune cells on prognosis in different types of samples, we evaluated the model using the consistency index (C-index); the higher the C-index value, the more significant the influence of a certain type of cell on the prognosis.
Statistical analysis
Spearman’s correlational analysis was performed to explore the relationships among the variables. Wilcoxon Signed Rank Test was used to conduct paired independent sample t-tests. Statistical analyses were performed and visualized using R version 4.1.0.
Results
An overview of AS events in TCGA ovarian cancer samples
Based on RNA-Seq data obtained from 318 OV patients, we established an integrated AS events profiling. As shown in Figure 2A, the seven AS types are AA, AD, AP, AT, ES, ME, and RI. As a result, this AS events profile included 48,049 AS events and its related 21,841 genes. Specifically, we obtained 4,006 AA type AS events comprising 2,777 genes, 3,497 AD types AS events comprising 2,389 genes, 9,689 AP type AS events comprising 3,901 genes, 8,453 AT type AS events comprising 3,691 genes, 19,251 ES type AS events comprising 6,931 genes, 207 ME type AS events comprising 201 genes, and 2,946 RI type AS events comprising 1,951 genes (Figure 2B). It turned out that ES was the largest number of AS event types, and a single gene could correspond to multiple AS types. We used an UpSet diagram (51) to visualize the interactions between these AS events (Figure 2C). Finally, A Venn plot between the seven groups showed that there were three single genes corresponding to seven AS types (Figure 2D).
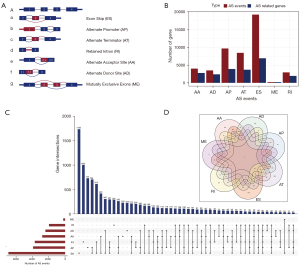
Construction of a prognostic model for ovarian cancer based on AS events
To explore the prognosis of AS events in OV patients, we first used univariate Cox regression analysis to evaluate the impact of all AS events on prognosis. We obtained a total of 2,206 survival-related AS events (P<0.05), and the hazard ratio (HR) value was used to evaluate which of these were poor prognostic factors (HR >1) or favourable prognostic factors (HR <1), as shown in Figure 3A,3B. Forest maps were drawn for the top 20 AS events that were significantly related to survival for the seven different AS types (except for ME, which only had 14), as shown in Figure 3C-3I. Next, 194 (30×6+14) AS events significantly associated with survival from the seven different AS types were selected to construct a PPI network (only showing the names of proteins with interactions greater than 5). The PPI network revealed the interactions between AS events (Figure 3J), in which it could be seen that RPS27A, MYC, etc., were most closely related to other AS events.
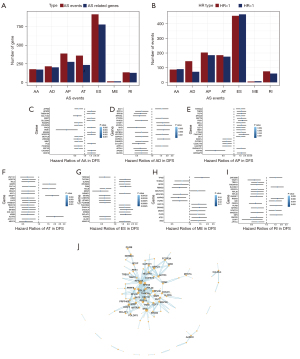
In order to obtain the independent prognostic factors for OV patients, for each type of AS event, we selected approximately the top 20 AS events that were markedly associated with survival for multivariate Cox regression analysis (19) (factors that could clearly distinguish risk were also included in the model construction, except for ME, which only had 14) (P<0.05). We found that the AA type contained eight independent prognostic factors, the AD type contained six independent prognostic factors, the AP type contained 10 independent prognostic factors, the AT type contained 10 independent prognostic factors, the ES type contained 16 independent prognostic factors, the ME type contained three independent prognostic factors, and the RI type contained five independent prognostic factors. Finally, a total of 58 independent prognostic factors were involved in the construction of ovarian cancer prognosis model based on AS events (Table S2).
Kaplan-Meier survival analysis was performed to verify the ability of the prognostic model constructed by different types of AS events to forecast the survival ability of ovarian cancer patients (Figure 4A-4H). Prognostic models for all types of AS events could significantly divide patients into high- and low-risk groups (P<0.05), and the area under curve (AUC) value of the ROC curve remained above 0.6 (except for ME, 0.59) (Figure 4I). In particular, the AUC of the final prognostic model was 0.75, indicating a high accuracy. Subsequently, according to the risk score formula, we constructed a risk score model to further verify the prognostic value. The results showed that the risk score chart and the survival-death status chart of patients ranked by the risk value of each sample indicated that the prognostic model could clearly divide patients into the high- and low-risk groups (Figure 4J-4L).
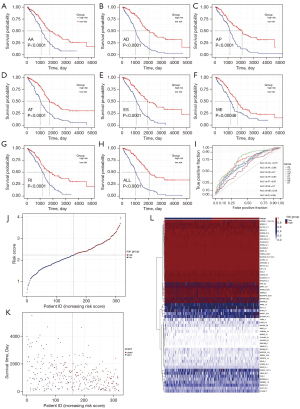
Platinum sensitivity analysis of prognostic factors
The CellMiner database provided us with a method for the Genomics of Drug Sensitivity (GDS) analysis. We screened and retained the data of three platinum-based GI50 drugs (Z-score standardized) that were approved by clinical trials and the FDA. GI50, the half maximal inhibitory concentration (IC50) revised by the NCI, represents the concentration of the drug when half the cancer cells undergo apoptosis (33). The CellMiner database also provided us with the expression levels of 22,217 genes (33). A total of 58 AS events were selected in the OV prognostic model and compared with the gene set provided by CellMiner, and 56 genes corresponding to AS events were retained.
Next, correlation analysis was conducted on the RNA expression levels of these 56 genes and the GI50 data of three platinum-based drugs, and 13 sets of results were obtained (Spearman, P<0.05). All 13 groups of results were selected to draw the scatter plots (Figure 5A), and according to the gene expression level, box plots were used to draw the differences between the high and low expression groups (Figure 5B). For example, the expression level of melanoma antigen family member D2 (MAGED2) was significantly negatively correlated with the GI50 value of oxaliplatin (R=0.301, P<0.05), indicating that with the increase of MAGED2 expression, the half inhibitory concentration of oxaliplatin decreased, which might improve the effectiveness of treatment. On the other hand, the expression level of lymphocyte activating 3 (LAG3) was positively correlated with the GI50 value of cisplatin (R=0.300, P<0.05), indicating that with the increase of the expression level of LAG3, the half inhibitory concentration of cisplatin increased, possibly leading to platinum resistance.
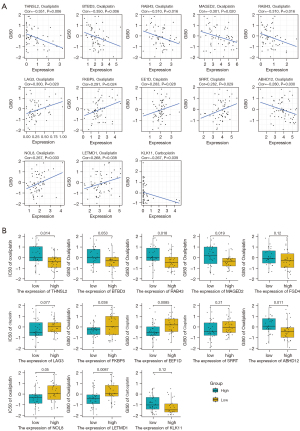
Construction of the splicing correlation network
In order to identify the biological factors involved in the regulation of survival-related AS events in OV, we performed survival analysis of AS-related molecules provided by MiasDB, and correlation analysis was performed between the expression levels of AS-related molecules and the PSI value of all survival-related AS events (Spearman, P<0.05). Cytoscape was then used to build AS correlation network (Figure 6A). The result showed that 10 AS-related molecules (squares) were associated with OV prognosis and were significantly associated with the PSI value of 89 AS events (circles). Specifically, four AS-related molecules presented a poor prognosis (turquoise squares), and six AS-related molecules presented a favourable prognosis (green squares). The red line indicated that the expression of AS-related molecules was positively correlated with AS events, while the black line indicated that AS-related molecules were negatively correlated with AS events. For example, the AS-related molecules Poly(U) binding splicing factor 60 (PUF60) and RAN binding protein 9 (RNABP9) were associated with survival (Figure 6B,6C). The PSI value of PHD finger protein 19 (PHF19) was positively correlated with the expression level of DExH-box helicase 30 (DHX30), while the PSI value of dishevelled binding antagonist of beta catenin 3 (DACT3) was negatively correlated with the expression level of the SF neuro-oncological ventral antigen 2 (NOVA2) (Figure 6D,6E).
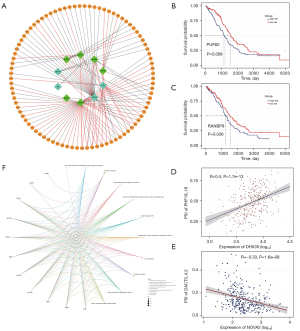
We performed GO enrichment analysis based on all AS-related molecules and AS events in the AS interaction network. The biological process showed that these genes were mainly related to the process of viral gene expression, transcription, and nuclear-transcribed mRNA metabolism. Finally, the network diagram of the relationship between the enrichment pathway of biological processes and related genes was presented in detail (Figure 6F).
SNF-CC divided OV samples into three subtypes
We performed cancer subtypes analysis using the “CancerSubtypes” R package. The package’s built-in Cox model enabled us to filter out critical survival-related AS events and then identify cancer subtypes based on the characteristic AS events. We used SNF-CC method to build a clustering model (k=2–5), and the performance of this clustering model was evaluated by consensus heatmap, survival curve, and silhouette width (Figure 7A-7D). The results showed that when all OV patients were divided into two groups, three groups, and four groups, the silhouette width exhibited sufficient robustness, but began to be unstable in five groups. Moreover, when the samples were divided into three groups, the survival ability of patients showed the most significant differences (P=9.54e-07), while the model of the two groups could not distinguish the survival ability of patients (P=0.362). Therefore, we chose three clusters as the final cancer subtypes model: Cluster1 consisted of 167 patients, Cluster2 consisted of 62 patients, and Cluster3 consisted of 89 patients.
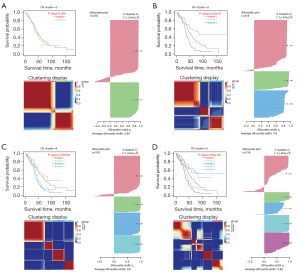
Association of identified subtypes with immune gene expression and immune cell infiltration
We validated the association between immune system processes and these three subtypes based on the samples of OV subtypes. We found that the immune gene TPT1 was differentially expressed between the three groups. In order to explore the differences in functional annotations and signalling pathways between different clusters, we selected TPT1 to conduct GSEA analysis (Figure 8A-8C). As a result, the enrichment abundances and signalling pathways of TPT1 were different between these three groups.
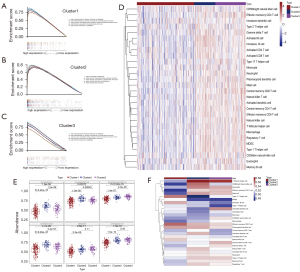
To explore whether there were differences in immune cell infiltration between the three clusters, we performed ssGSEA analysis using the “GSVA” R package and used a heatmap to visualize the ssGSEA results of 28 immune cells (Figure 8D,8E). Significant differences were observed in immune cell infiltration between these three clusters. Most of the immune cells had the lowest enrichment score in Cluster1, while that of Cluster2 was highest, and the enrichment score in Cluster3 was slightly lower (although some of them were higher than Cluster2 or Cluster1). We also found that Cluster2 exhibited a higher abundance of anti-tumor immune cell types, including activated B cell, immature B cell, activated CD8 T cell, etc. Meanwhile, pro-tumor immune cells, such as mast cells, immature dendritic cells, CD56bright natural killer cells, and regulatory T cells, were significantly abundant in Cluster3. To some extent, this could explain why samples in Cluster2 had the lowest risk and samples in Cluster3 had the highest risk in the survival analysis of OV subtypes.
Combined with the results of GSEA, the distinctions of the enrichment of immune-related pathways in different subtypes might be due to the disparities of the different immune cell infiltration in tumors. Finally, to explore the effect of each immune cell on prognosis in different clusters of samples, we evaluated the model using the conformance index (C-index) of univariate Cox regression (Figure 8F). The results showed that the T follicular helper cell had the greatest effect on the prognosis of Cluster2 and Cluster3, and the effector memory CD8 T cell had the greatest effect on the prognosis of Cluster1.
Discussion
With advances in high-throughput technology and the development of next-generation sequencing technologies, it is possible to combine multi-omics databases and use bioinformatics methods to investigate the mechanism of AS behind cancer (49). In order to make all-around and in-depth analysis based on AS events in OV, we focused on constructing an innovative prognostic model to gain more precise survival prediction and combining prognostic AS events with immune infiltration and drug sensitivity analysis to search for more potential therapeutic targets. By analyzing TCGA database, we identified AS events in OV and constructed a prognostic model based on AS events. The AS profiles we compiled included 48,049 AS events and its related 21,841 genes. Interestingly, ES events accounted for the main component of these seven types, because ES events could be easily verified by polymerase chain reaction (PCR). We systematically explained the relationship between AS events and DFS in 318 patients with OV and established prognostic models for each AS type using multivariate Cox regression. After comparing the AUC values of each AS type, we found that ES and AD had better prediction effects (AUC =0.70) than the other five prognostic models, and the final prognostic model, which combined the seven AS types, had the most effective prediction effect (AUC =0.75). Some genes of the prognostic AS events, which were constructed in the final ovarian cancer prognostic model, have been confirmed to play significant roles in the occurrence of OV in previous studies. For example, high kallikrein related peptidase 11 (KLK11) mRNA expression is markedly related to prolonged overall survival and DFS (52). Vascular endothelial growth factor A (VEGFA) is an angiogenic factor, and circular absent, small or homeotic 2-like protein (circASH2L) binding to VEGFA plays a key role in the regulation of tumor genesis, angiogenesis, and lymphangiogenesis in ovarian cancer cells (53). Meanwhile, VEGFA can activate OV stem cells through miR-128-2 (MicroRNA 128-2) methylation and B lymphoma Mo-MLV insertion region 1 homolog (Bmi1) up-regulation driven by Src-DNMT3A (54). LAG3 acts synergistically with programmed cell death 1 (PD1) to regulate T cell conduction and inhibit the anti-tumor immune process (55). These previous studies are consistent with the results of the prognostic impact in our analysis, and they could not only indicate that the model has a good accuracy but also provide certain clues for other potential therapeutic targets and biomarkers that have not been studied.
Based on AS factors in the OV prognostic model, the CellMiner database was used for platinum sensitivity analysis. Previous studies have shown that the expression of the melanoma antigen (MAGE) protein family is dysregulated in a variety of cancers, and its abnormal expression is often associated with poor prognosis (56,57). In our analysis, the expression level of MAGED2 was significantly negatively correlated with the GI50 value of oxaliplatin, indicating that with the increased expression level of MAGED2, the half inhibitory concentration of oxaliplatin decreased and the therapeutic effect might be improved. On the other hand, LAG3, a cancer immunotherapy target (58), was significantly expressed positively correlated with the GI50 value of cisplatin, indicating that with the increased expression level of LAG3, the half inhibitory concentration of cisplatin increased, possibly leading to the development of platinum resistance. Therefore, this research can provide some ideas for the platinum-based drug treatment of OV.
Our analysis also emphasized the potential contribution of SF. We not only studied the regulation of SF on AS events but also focused on the effects of AS-related molecules, such as kinases, transcription factors, and histone-modifying enzymes, on AS (36-38). In the splicing correlation network, there are some interactions between AS-related molecules and AS events, which have potential value. In particular, there is a significant negative correlation between the expression level of NOVA2 and the PSI value of DACT3. NOVA2 is a variable SF, and the over expression of NOVA2 in ovarian cancer may be related to tumor angiogenesis (59). DACT3 is a negative regulator of Wnt/β-catenin signal transduction, and Wnt signaling plays a vital role in epithelial-mesenchymal transition, drug resistance, and prognosis of ovarian cancer stem cells (60,61).
Next, we used the SNF-CC method to classify OV samples, and the classification results showed that there were significant differences between the survival of these three subtypes’ samples. Relevant studies have shown that the changes of AS are not only limited to tumor tissues but also exist in the tumor microenvironment, and the changes of tumor-related splicing events are regulated by SF that are differentially expressed in tumor tissues (62). Therefore, we hoped to further explore the tumor microenvironment differences in the three subtypes of ovarian cancer. After performing GSEA analysis of the immune-related gene TPT1, we analyzed the immune cell infiltration of these three OV subtypes. These results in accordance with the survival analysis results of cancer classification showed that most of the enrichment abundance of anti-tumor immune cells, such as activated B cell, immature B cell, activated CD8 T cell in Cluster2, was the highest, and most of the enrichment abundance of pro-tumor immune cells, such as mast cell, immature dendritic cell, CD56bright natural killer cells, and regulatory T cell in Cluster3, was the highest. In conclusion, the overall survival effect of Cluster2 was superior to that of Cluster3, while Cluster1 was intermediate.
Our study focused on the effect of AS on the occurrence and progression of tumor, and this process have been confirmed by a large number of previous reports. AS of mRNA not only participates in a variety of normal physiological activities, but also plays an important role in cancer (13). Previous studies have found that AS events in certain genes can promote or inhibit the development of ovarian cancer (6-9). For example, abnormal splicing of breast-cancer susceptibility gene 1 (BRCA1) and breast-cancer susceptibility gene 2 (BRCA2) may be associated with the pathogenesis of ovarian cancer (6). Recepteur d’origine nantais (RON) and its AS variants participate in multiple tumor biological processes, including cell proliferation, adhesion, movement, and apoptosis. Overexpression of RON and the emergence of RON AS variants have been detected in ovarian cancer, and the up-regulation of these variants are significantly correlated with the expression of SF (7). Two variants of cell division cycle 42 (CDC42), CDC42-v1 and CDC42-v2, can be produced by AS. CDC42-v2 has been observed to exert inhibitory effects on the growth and invasion of ovarian tumor cells, whereas CDC42-v1 has not shown these effects (9). In conclusion, these reports provide a theoretical basis for studying the regulatory mechanism of AS in ovarian cancer.
To our knowledge, this study is the first to use the CellMiner database in combination with the AS prognostic model to explore platinum sensitivity in ovarian cancer. Furthermore, it is also the first to use the SNF-CC method to classify ovarian cancer samples and explore immune cell infiltration differences in OV subtypes. These findings have enriched our understanding of the AS events in ovarian cancer and provided substantive candidate biomarkers and potential targets for the treatment of ovarian cancer.
Although our study provides some new ideas for the AS prognostic model of ovarian cancer, there were some limitations and room for improvement. Firstly, the establishment and verification of the model was only limited to the TCGA-OV expression profiles and the TCGASpliceSeq AS profiles. However, independent OV data sets should also be used for verification and extension. Secondly, due to the scarcity of normal samples in the TCGASpliceSeq database, differences between ovarian cancer tumor samples and normal samples were not analyzed. Furthermore, because our study was based on single-omics, the different clinicopathological features between populations at different risk and subtypes may be due to inherent differences in other factors, such as deoxyribonucleic acid (DNA) methylation, which might have influenced our results.
Conclusions
In conclusion, our study identified significant AS events in OV and constructed an effective model to predict patient survival outcomes. OV subtypes based on survival-related AS events suggested that the tumor microenvironment, especially immune cell infiltration, had an obvious influence on the prognosis of OV. In addition, the combination of platinum sensitivity analysis and the construction of splicing-related factor networks provided various valuable potential therapeutic targets for the study of OV. Therefore, this in-depth analysis based on AS events can provide some new perspectives for the development of treatment strategies for OV.
Acknowledgments
We would like to thank the academic editor and reviewers for their important contributions, which improved the quality of this article.
Funding: This research was funded by the Fundamental Research Funds for the Central Universities [grant number: DUT19RC(3)076], the National Natural Science Foundation of China (grant numbers: 82103056 and 81600370), and the China Postdoctoral Science Foundation (grant number: 2018M640270).
Footnote
Provenance and Peer Review: This article was commissioned by the Guest Editor (Danbo Wang) for the series “New Progress and Challenge in Gynecological Cancer” published in Annals of Translational Medicine. The article has undergone external peer review.
Reporting Checklist: The authors have completed the REMARK reporting checklist. Available at https://atm.amegroups.com/article/view/10.21037/atm-21-6422/rc
Conflicts of Interest: All authors have completed the ICMJE uniform disclosure form (available at https://atm.amegroups.com/article/view/10.21037/atm-21-6422/coif). The series “New Progress and Challenge in Gynecological Cancer” was commissioned by the editorial office without any funding or sponsorship. The authors have no other conflicts of interest to declare.
Ethical Statement: The authors are accountable for all aspects of the work in ensuring that questions related to the accuracy or integrity of any part of the work are appropriately investigated and resolved. The study was conducted in accordance with the Declaration of Helsinki (as revised in 2013).
Open Access Statement: This is an Open Access article distributed in accordance with the Creative Commons Attribution-NonCommercial-NoDerivs 4.0 International License (CC BY-NC-ND 4.0), which permits the non-commercial replication and distribution of the article with the strict proviso that no changes or edits are made and the original work is properly cited (including links to both the formal publication through the relevant DOI and the license). See: https://creativecommons.org/licenses/by-nc-nd/4.0/.
References
- Reid BM, Permuth JB, Sellers TA. Epidemiology of ovarian cancer: a review. Cancer Biol Med 2017;14:9-32. [Crossref] [PubMed]
- Oberaigner W, Minicozzi P, Bielska-Lasota M, et al. Survival for ovarian cancer in Europe: the across-country variation did not shrink in the past decade. Acta Oncol 2012;51:441-53. [Crossref] [PubMed]
- Stenzel AE, Buas MF, Moysich KB. Survival disparities among racial/ethnic groups of women with ovarian cancer: An update on data from the Surveillance, Epidemiology and End Results (SEER) registry. Cancer Epidemiol 2019;62:101580. [Crossref] [PubMed]
- Wong KH, Mang OW, Au KH, et al. Incidence, mortality, and survival trends of ovarian cancer in Hong Kong, 1997 to 2006: a population-based study. Hong Kong Med J 2012;18:466-74. [PubMed]
- Enroth S, Berggrund M, Lycke M, et al. High throughput proteomics identifies a high-accuracy 11 plasma protein biomarker signature for ovarian cancer. Commun Biol 2019;2:221. [Crossref] [PubMed]
- Sanz DJ, Acedo A, Infante M, et al. A high proportion of DNA variants of BRCA1 and BRCA2 is associated with aberrant splicing in breast/ovarian cancer patients. Clin Cancer Res 2010;16:1957-67. [Crossref] [PubMed]
- Mayer S, Hirschfeld M, Jaeger M, et al. RON alternative splicing regulation in primary ovarian cancer. Oncol Rep 2015;34:423-30. [Crossref] [PubMed]
- Fischer DC, Noack K, Runnebaum IB, et al. Expression of splicing factors in human ovarian cancer. Oncol Rep 2004;11:1085-90. [Crossref] [PubMed]
- Tien JF, Mazloomian A, Cheng SG, et al. CDK12 regulates alternative last exon mRNA splicing and promotes breast cancer cell invasion. Nucleic Acids Res 2017;45:6698-716. [Crossref] [PubMed]
- Li N, Zhan X. Identification of clinical trait-related lncRNA and mRNA biomarkers with weighted gene co-expression network analysis as useful tool for personalized medicine in ovarian cancer. EPMA J 2019;10:273-90. [Crossref] [PubMed]
- Nilsen TW, Graveley BR. Expansion of the eukaryotic proteome by alternative splicing. Nature 2010;463:457-63. [Crossref] [PubMed]
- Traunmüller L, Gomez AM, Nguyen TM, et al. Control of neuronal synapse specification by a highly dedicated alternative splicing program. Science 2016;352:982-6. [Crossref] [PubMed]
- Lee SC, Abdel-Wahab O. Therapeutic targeting of splicing in cancer. Nat Med 2016;22:976-86. [Crossref] [PubMed]
- Climente-González H, Porta-Pardo E, Godzik A, et al. The Functional Impact of Alternative Splicing in Cancer. Cell Rep 2017;20:2215-26. [Crossref] [PubMed]
- Oltean S, Bates DO. Hallmarks of alternative splicing in cancer. Oncogene 2014;33:5311-8. [Crossref] [PubMed]
- Yu M, Hong W, Ruan S, et al. Genome-Wide Profiling of Prognostic Alternative Splicing Pattern in Pancreatic Cancer. Front Oncol 2019;9:773. [Crossref] [PubMed]
- Xiong Y, Deng Y, Wang K, et al. Profiles of alternative splicing in colorectal cancer and their clinical significance: A study based on large-scale sequencing data. EBioMedicine 2018;36:183-95. [Crossref] [PubMed]
- Lin P, He RQ, Huang ZG, et al. Role of global aberrant alternative splicing events in papillary thyroid cancer prognosis. Aging (Albany NY) 2019;11:2082-97. [Crossref] [PubMed]
- Zhu J, Chen Z, Yong L. Systematic profiling of alternative splicing signature reveals prognostic predictor for ovarian cancer. Gynecol Oncol 2018;148:368-74. [Crossref] [PubMed]
- Farina AR, Cappabianca L, Sebastiano M, et al. Hypoxia-induced alternative splicing: the 11th Hallmark of Cancer. J Exp Clin Cancer Res 2020;39:110. [Crossref] [PubMed]
- Brum MCM, Guimaraes IDS, Ferreira LB, et al. Osteopontin-c isoform inhibition modulates ovarian cancer cell cisplatin resistance, viability and plasticity. Oncol Rep 2021;45:652-64. [Crossref] [PubMed]
- Frankiw L, Baltimore D, Li G. Alternative mRNA splicing in cancer immunotherapy. Nat Rev Immunol 2019;19:675-87. [Crossref] [PubMed]
- Li ZX, Zheng ZQ, Wei ZH, et al. Comprehensive characterization of the alternative splicing landscape in head and neck squamous cell carcinoma reveals novel events associated with tumorigenesis and the immune microenvironment. Theranostics 2019;9:7648-65. [Crossref] [PubMed]
- Luo Z, Wang Q, Lau WB, et al. Tumor microenvironment: The culprit for ovarian cancer metastasis? Cancer Lett 2016;377:174-82. [Crossref] [PubMed]
- Kim EK, Yoon SO, Jung WY, et al. Implications of NOVA1 suppression within the microenvironment of gastric cancer: association with immune cell dysregulation. Gastric Cancer 2017;20:438-47. [Crossref] [PubMed]
- Shannon P, Markiel A, Ozier O, et al. Cytoscape: a software environment for integrated models of biomolecular interaction networks. Genome Res 2003;13:2498-504. [Crossref] [PubMed]
- Wang Z, Jensen MA, Zenklusen JC. A Practical Guide to The Cancer Genome Atlas (TCGA). Methods Mol Biol 2016;1418:111-41. [Crossref] [PubMed]
- Ryan M, Wong WC, Brown R, et al. TCGASpliceSeq a compendium of alternative mRNA splicing in cancer. Nucleic Acids Res 2016;44:D1018-22. [Crossref] [PubMed]
- Schafer S, Miao K, Benson CC, et al. Alternative Splicing Signatures in RNA-seq Data: Percent Spliced in (PSI). Curr Protoc Hum Genet 2015;87:11.16.1-11.16.14.
- van Buuren S, Groothuis-Oudshoorn K. mice: Multivariate Imputation by Chained Equations in R. Journal of Statistical Software 2011;45:1-67. [Crossref]
- Chen QF, Li W, Wu P, et al. Alternative splicing events are prognostic in hepatocellular carcinoma. Aging (Albany NY) 2019;11:4720-35. [Crossref] [PubMed]
- Li B, Cui Y, Diehn M, et al. Development and Validation of an Individualized Immune Prognostic Signature in Early-Stage Nonsquamous Non-Small Cell Lung Cancer. JAMA Oncol 2017;3:1529-37. [Crossref] [PubMed]
- Reinhold WC, Sunshine M, Liu H, et al. CellMiner: a web-based suite of genomic and pharmacologic tools to explore transcript and drug patterns in the NCI-60 cell line set. Cancer Res 2012;72:3499-511. [Crossref] [PubMed]
- van Zyl B, Tang D, Bowden NA. Biomarkers of platinum resistance in ovarian cancer: what can we use to improve treatment. Endocr Relat Cancer 2018;25:R303-18. [Crossref] [PubMed]
- Luco RF, Allo M, Schor IE, et al. Epigenetics in alternative pre-mRNA splicing. Cell 2011;144:16-26. [Crossref] [PubMed]
- Gómez Acuña LI, Fiszbein A, Alló M, et al. Connections between chromatin signatures and splicing. Wiley Interdiscip Rev RNA 2013;4:77-91. [Crossref] [PubMed]
- Shin C, Manley JL. Cell signalling and the control of pre-mRNA splicing. Nat Rev Mol Cell Biol 2004;5:727-38. [Crossref] [PubMed]
- Martinez NM, Lynch KW. Control of alternative splicing in immune responses: many regulators, many predictions, much still to learn. Immunol Rev 2013;253:216-36. [Crossref] [PubMed]
- Xing Y, Zhao X, Yu T, et al. MiasDB: A Database of Molecular Interactions Associated with Alternative Splicing of Human Pre-mRNAs. PLoS One 2016;11:e0155443. [Crossref] [PubMed]
- Yu G, Wang LG, Han Y, et al. clusterProfiler: an R package for comparing biological themes among gene clusters. OMICS 2012;16:284-7. [Crossref] [PubMed]
- Wang B, Mezlini AM, Demir F, et al. Similarity network fusion for aggregating data types on a genomic scale. Nat Methods 2014;11:333-7. [Crossref] [PubMed]
- Monti S, Tamayo P, Mesirov J, et al. Consensus Clustering: A Resampling-Based Method for Class Discovery and Visualization of Gene Expression Microarray Data. Machine Learning 2003;52:91-118. [Crossref]
- Xu T, Le TD, Liu L, et al. CancerSubtypes: an R/Bioconductor package for molecular cancer subtype identification, validation and visualization. Bioinformatics 2017;33:3131-3. [Crossref] [PubMed]
- Golebiewska A, Bougnaud S, Stieber D, et al. Side population in human glioblastoma is non-tumorigenic and characterizes brain endothelial cells. Brain 2013;136:1462-75. [Crossref] [PubMed]
- Shen S, Wang G, Zhang R, et al. Development and validation of an immune gene-set based Prognostic signature in ovarian cancer. EBioMedicine 2019;40:318-26. [Crossref] [PubMed]
- Bhattacharya S, Andorf S, Gomes L, et al. ImmPort: disseminating data to the public for the future of immunology. Immunol Res 2014;58:234-9. [Crossref] [PubMed]
- Subramanian A, Tamayo P, Mootha VK, et al. Gene set enrichment analysis: a knowledge-based approach for interpreting genome-wide expression profiles. Proc Natl Acad Sci U S A 2005;102:15545-50. [Crossref] [PubMed]
- Hänzelmann S, Castelo R, Guinney J. GSVA: gene set variation analysis for microarray and RNA-seq data. BMC Bioinformatics 2013;14:7. [Crossref] [PubMed]
- Zhao L, Zhang J, Liu Z, et al. Comprehensive Characterization of Alternative mRNA Splicing Events in Glioblastoma: Implications for Prognosis, Molecular Subtypes, and Immune Microenvironment Remodeling. Front Oncol 2021;10:555632. [Crossref] [PubMed]
- Charoentong P, Finotello F, Angelova M, et al. Pan-cancer Immunogenomic Analyses Reveal Genotype-Immunophenotype Relationships and Predictors of Response to Checkpoint Blockade. Cell Rep 2017;18:248-62. [Crossref] [PubMed]
- Lex A, Gehlenborg N, Strobelt H, et al. UpSet: Visualization of Intersecting Sets. IEEE Trans Vis Comput Graph 2014;20:1983-92. [Crossref] [PubMed]
- Geng X, Liu Y, Dreyer T, et al. Elevated tumor tissue protein expression levels of kallikrein-related peptidases KLK10 and KLK11 are associated with a better prognosis in advanced high-grade serous ovarian cancer patients. Am J Cancer Res 2018;8:1856-64. [PubMed]
- Chen J, Li X, Yang L, et al. CircASH2L Promotes Ovarian Cancer Tumorigenesis, Angiogenesis, and Lymphangiogenesis by Regulating the miR-665/VEGFA Axis as a Competing Endogenous RNA. Front Cell Dev Biol 2020;8:595585. [Crossref] [PubMed]
- Jang K, Kim M, Gilbert CA, et al. VEGFA activates an epigenetic pathway upregulating ovarian cancer-initiating cells. EMBO Mol Med 2017;9:304-18. [Crossref] [PubMed]
- Huang RY, Eppolito C, Lele S, et al. LAG3 and PD1 co-inhibitory molecules collaborate to limit CD8+ T cell signaling and dampen antitumor immunity in a murine ovarian cancer model. Oncotarget 2015;6:27359-77. [Crossref] [PubMed]
- Vansteenkiste JF, Cho BC, Vanakesa T, et al. Efficacy of the MAGE-A3 cancer immunotherapeutic as adjuvant therapy in patients with resected MAGE-A3-positive non-small-cell lung cancer (MAGRIT): a randomised, double-blind, placebo-controlled, phase 3 trial. Lancet Oncol 2016;17:822-35. [Crossref] [PubMed]
- Li R, Gong J, Xiao C, et al. A comprehensive analysis of the MAGE family as prognostic and diagnostic markers for hepatocellular carcinoma. Genomics 2020;112:5101-14. [Crossref] [PubMed]
- Andrews LP, Marciscano AE, Drake CG, et al. LAG3 (CD223) as a cancer immunotherapy target. Immunol Rev 2017;276:80-96. [Crossref] [PubMed]
- Angiolini F, Belloni E, Giordano M, et al. A novel L1CAM isoform with angiogenic activity generated by NOVA2-mediated alternative splicing. Elife 2019;8:44305. [Crossref] [PubMed]
- Teeuwssen M, Fodde R. Wnt Signaling in Ovarian Cancer Stemness, EMT, and Therapy Resistance. J Clin Med 2019;8:1658. [Crossref] [PubMed]
- Jiang X, Tan J, Li J, et al. DACT3 is an epigenetic regulator of Wnt/beta-catenin signaling in colorectal cancer and is a therapeutic target of histone modifications. Cancer Cell 2008;13:529-41. [Crossref] [PubMed]
- Brosseau JP, Lucier JF, Nwilati H, et al. Tumor microenvironment-associated modifications of alternative splicing. RNA 2014;20:189-201. [Crossref] [PubMed]
(English Language Editor: A. Kassem)