Establishment of a molecular risk model for the prognosis of cervical cancer based on microRNA expression
Introduction
Globally, the incidence of cervical cancer (CC) is highest among all tumors of the female reproductive system, with 342,000 deaths worldwide in 2020 (1). Despite the effectiveness of human papilloma virus (HPV) vaccines in reducing the incidence of CC, the proportion of people that have been vaccinated remains low (2-4). Even if early CC can be halted by surgery, metastatic CC cannot be cured (5), and there is no effective molecular marker to predict the prognosis of CC. Thus, it is crucial to identify new reliable biomarkers for the diagnosis and prognosis of CC.
The development of biological sequencing technology has brought about trillions of data resources, which are shared by researchers and are valuable to tumor research. The Cancer Genome Atlas (TCGA) is a landmark database jointly run by the National Cancer Institute and the National Human Genome Research Institute. It offers information on the genome, epigenome, and transcriptome profiles of patients that have or previously suffered from different types of cancer, including basic information about CC.
MicroRNAs (miRNAs) are short non-coding RNAs with a length of approximately 18–25 nucleotides, which are vital in the regulation of gene expression by interacting with target mRNAs, leading to subsequent translational repression (6). The variability of miRNA expression has been shown to be significantly associated with the risk of cancer development, disease progression, metastatic ability, and response to chemotherapy and radiotherapy in a variety of tumors (7,8). Some miRNAs may be oncogenes or tumor suppressor genes in different cancer cells (9); for example, miR-193a-5p is a tumor-suppressor in colorectal cancer but a tumor-promoter in pancreatic cancer (10,11). Targeting heterogeneous nuclear ribonucleoprotein K in hepatocellular carcinoma (HCC) cell lines, miR-1249-3p is an oncogene in the proliferation, migration and invasion of cells (12). Systematic studies of specific miRNAs have improved our understanding of tumorigenesis, clarified the pathogenesis of various malignant tumors, and enabled the identification of new biomarkers for treatment and prognosis prediction.
In this study, we constructed and verified a two-miRNA prognostic risk model based on miRNA-seq data of CC tissues from the National Cancer Center/National Clinical Research Center for Cancer/Cancer Hospital, Chinese Academy of Medical Sciences and Peking Union Medical College and TCGA database. This model can predict the survival risk of patients with CC. We then investigated the function of messenger RNAs (mRNAs), which are the predicted targets of miRNAs in our model. Finally, we carried out qPCR experiments in normal and tumor tissues to verify the reliability and effectiveness of different miRNA expressions in the model. This study aims to establish a molecular prognostic model of CC based on miRNAs, and optimize the personalized treatment of CC patients. In this article, we integrate our own miRNA-seq data and supplement the TCGA database. There were only two miRNAs in the prognostic risk model, and the functions of two miRNAs were explored.
We present the following article in accordance with the TRIPOD reporting checklist (available at https://atm.amegroups.com/article/view/10.21037/atm-21-6451/rc).
Methods
Data acquisition and processing
We selected human CC tissues and nearby normal cervical tissues from the specimen database of the Cancer Hospital (Chinese Academy of Medical Sciences and Peking Union Medical College). Four CC tissues without lymph node metastasis, four CC tissues with lymph node metastasis, and four adjacent normal cervical tissues were included for miRNA gene sequencing. The samples were obtained with the written informed consent of all patients, and the study was conducted in accordance with the Declaration of Helsinki (as revised in 2013). The study was approved by the Ethics Committee of the National Cancer Center/National Clinical Research Center for Cancer/Cancer Hospital, Chinese Academy of Medical Sciences and Peking Union Medical College (No. 20/207-2,403).
CC transcriptome expression and clinical data used in the study were obtained from TCGA (https://cancergenome.nih.gov/). The miRNA data (version 16.0 dated March 22, 2019) included 309 CC tissues and three normal cervical tissues. R software (version 3.6.1) and specific R packages (https://svn.r-project.org/R-dev-web/trunk/index.html) were used to process and analyze data. TCGA data did not require further ethical approval. All related regulations concerning access to TCGA data and protection of patient privacy were strictly followed.
Screening for differential miRNA expression
We screened the differential expression of miRNA not only in TCGA database, but also in own miRNA-seq data, which is more persuasive and innovative. Leveraging the ‘edge’ R package in R software, we screened the differential expression of miRNAs in 12 miRNA-seq and TCGA database tissues. The screening criterion was log2(fold change) >1.5, and the cut-off for the false discovery rate (FDR) =0.05, there was statistical significance when P<0.05, in order to select the more significant differential miRNAs we referred to the literatures (13-15) and found that the difference was more significant when log2>1.5. With the help of the ‘ggplot2’ package in R software, two volcano maps of differentially-expressed miRNAs in the 12 miRNA-seq and TCGA database tissues were drawn. We compared the differentially-expressed miRNAs in the 12 miRNA-seq and the TCGA database tissues to distinguish the common differentially-expressed miRNAs.
Establishment and evaluation of a prognostic risk model
To predict CC prognosis, a risk score comprising two miRNAs was obtained by calculating the sum of each miRNA multiplied by its coefficient.
Assigned TCGA-CC datasets into training or testing sets (1:1 ratio) randomly. We used the miRNA model from the common differentially-expressed miRNAs to calculate the prognostic scores of the training and testing sets, as well as all CC patients. According to the median prognostic score in the training set, all patients were classified as high risk or low risk. We used Kaplan-Meier survival curves to verify survival differences among groups with high- and low-risk. To evaluate the predictive power of the prognostic model, time-dependent receiver operating characteristic (ROC) curve analysis was employed. To identify the value of the independent prognostic model, we subsequently analyzed the model and clinical parameters by univariate and multivariate Cox survival analysis, and the results were presented in the form of forest plots. We used the ‘survminer’, ‘caret’, ‘glmnet’, ‘survival’, and ‘survival ROC’ packages in R software during the process.
Predicting target genes in the prognostic risk model and establishment of the miRNA-mRNA co-expression network
Three databases (miRDB, miRTarBase, and TargetScan) were utilized for the prediction of target genes. To enhance the accuracy of the prediction, every gene classified as a target was placed in all three databases. We constructed Venn diagrams using the ‘VennDiagram’ package in R software. Next, the Pearson correlation test was performed on varied expressions of miRNAs and mRNAs in TCGA database. Finally, we obtained the miRNA/mRNA pairs with negative regulation and targeting.
Biological function studies of target genes
Cell culture and transfection
Human cervical epithelial cells (HCerEpiC) and human CC cell lines (ME180, HCC94) were cultured in a cell incubator with the following conditions: 5% CO2, high glucose Dulbecco’s modified eagle medium (DMEM) with 10% fetal bovine serum (FBS), 100 U/mL of penicillin, and 100 mg/mL of streptomycin. The cells were transfected in a six-well plate. We added miRNA-142-3p mimic and miR-100-3p inhibitor into the pre-transfection pores of a six-pore plate according to the related reagent specifications.
Colony formation assay
At 48 h post-transfection, the cells were digested with membrane protease, centrifuged, added with complete medium, and consecutively diluted to prepare a single-cell suspension. Approximately 500 cells were added to each well of a six-well plate; thus, the volume of the entire medium was increased to 2 mL. We seeded and assigned three pores for the transfected cells. The six-well plate was placed in an incubator with constant temperature for 2–3 weeks. The culture medium was replaced every 3 days until the cell clones could be perceived by the naked eye before terminating the culture. The cells were then fixed with 100% methanol for 20 min, stained with 0.1% crystal violet for 30 min, rinsed with phosphate buffered saline (PBS), dried, and photographed.
Migration and invasion assays
The cells were inoculated in the upper side of the transwell chamber (Corning, Corning, NY, USA). In the bottom side, 700 μL of medium with 10% FBS was applied. After 48 hours of incubation, the cells moved to the bottom side and were fixed in 50% methanol and 50% acetone for 15 min, and subsequently underwent crystal violet staining for 20 min. The cells were then counted under the microscope (OLMPUS, Japan). We randomly selected five fields in every sample to count number of migratory cells (magnification ×200).
Quantitative real-time polymerase chain reaction (qRT-PCR)
Total tissue and cellular RNA was extracted using the FastPure Cell/Tissue Total RNA Isolation Kit V2 (Vazyme, Nanjing, China). Reverse-transcription from RNAs into complementary DNAs (cDNAs) was performed using the miRNA First Strand cDNA Synthesis Kit (Vazyme, Nanjing, China). We assessed all miRNA levels using the RT SuperMix for qPCR (Vazyme, Nanjing, China). The small nuclear RNA U6 served as an internal reference. For each sample, three replicates were prepared, with average cycle threshold (Ct) values. The 2−ΔΔCt method was employed to calculate the relative expression of each miRNA.
Statistical analysis
All data were processed by SPSS 20.0 (IBM, USA), with continuous values presented as mean standard deviation. The t-test was used to examine the differences between two groups, and P<0.05 was considered as statistically significant.
Results
Workflow
Two miRNA databases were examined, including one piece from four CC tissues without lymph node metastasis, four CC tissues with lymph node metastasis, and four adjacent normal cervical tissues. The other piece was from 309 CC tissues and three adjacent normal cervical tissues, which was obtained from TCGA. We used these two pieces of data to develop a prognostic risk model (Figure 1). The clinical data of 255 patients with CC (also from TCGA) was used to develop a prognostic risk model.
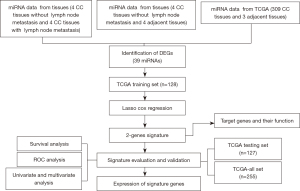
miRNA and clinicopathological data
We enrolled 255 patients with CC. These patients were randomly divided into training (n=128) and testing (n=127) sets. There was no significant difference in the clinical characteristics between the two groups (all P>0.05).
Screening for differentially-expressed miRNAs
In TCGA data, the miRNA data from CC tissues (n=309) and adjacent normal tissues (n=3) indicated 191 differentially-expressed miRNAs (Figure 2A,2B), and 158 mRNAs with different expression (Figure 2C,2D).
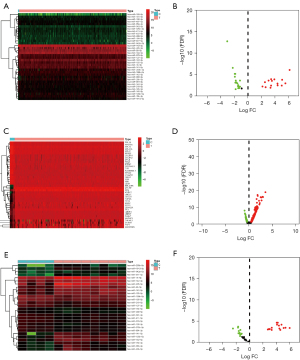
In 12 miRNA-seq tissues, the miRNA data from CC tissues (n=4) and adjacent normal tissues (n=4) suggested 258 differentially-expressed miRNAs, while the miRNA data from CC tissues (n=4) and CC tissues with lymph node metastasis (n=4) indicated 131 differentially-expressed miRNAs (Figure 2E,2F).
We compared the three pieces of data and distinguished 39 common differentially-expressed miRNAs.
Establishing and evaluating the prognostic risk models
The Venn diagram for each miRNA showed the number of target genes in each database, as well as the number of target genes typically displayed in any two or three databases (Figure 3A).
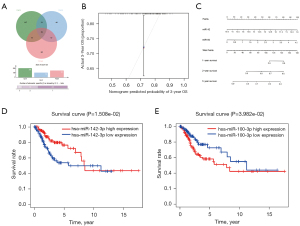
LASSO Cox regression analysis of the common differentially-expressed miRNAs resulted in a prognostic risk model based upon the expression of two miRNAs as follows:
A nomogram was built for intuitively and effectively analysis of the 2-miRNA risk score model 3-year survival were almost identical to the standard curve (Figure 3B,3C). Thus, one miRNA (hsa-miR-142-3p) was a protective factor in CC, and one miRNA (hsa-miR-100-3p) was a risk factor for CC (Figure 3D,3E).
Kaplan-Meier analysis of the training set showed that the 5-year survival rate was significantly lower in the high-risk group than in the low-risk group (P<0.01, Figure 4A). Similar results were obtained from the testing set (P<0.05, Figure 4B) and from all patients (P<0.001, Figure 4C). Therefore, the results indicated that the two-miRNA risk score can be used to predict the 5-year survival rate in patients with CCs. Moreover, the ROC curves of the training and testing sets, as well as all patients in both datasets combined were relatively smooth, with an area under the curve (AUC) greater than 0.7, which signifies that the prognostic performance of the model was receivable (Figures 4D-4F). Analyses of the training and testing sets, as well as all patients showed that mortality rate in the high-risk group was greater than that of the low-risk group (Figures 5A-5C).
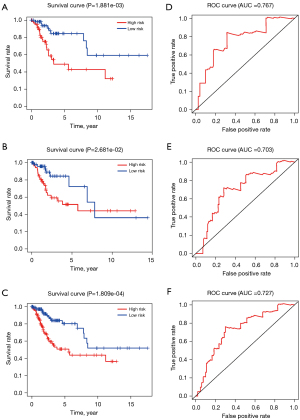
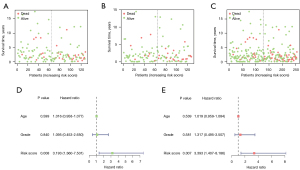
Univariate Cox regression analysis (Figure 5D) showed that the two-miRNA risk score was significantly correlated with prognosis (HR =3.193, P<0.05) in all ages and grades. Multivariate Cox regression analysis demonstrated that the two-miRNA risk score plays an independent role in prognosis (HR =3.393, P<0.05); none of the other examined variables were statistically significant (Figure 5E).
Identifying the miRNA-mRNA regulatory network
Databases including miRDB, miRTarBase, and TargetScan were used to predict the target genes of the two miRNAs in our model (Figure 6A,6B).
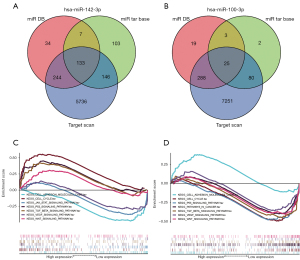
We obtained two miRNA/mRNA negatively regulated and targeted pair: HMGB1 id target for miR142-3p and DHCR24 is target for miRNA-100-3p.
Kyoto Encyclopedia of Genes and Genomes (KEGG) enrichment analysis
KEGG enrichment analysis was performed on the target mRNAs of the two miRNAs, which suggested that the enrichment of target genes mainly took place in the cell cycle, as well as the p53, transforming growth factor (TGF) beta, vascular endothelial growth factor (VEGF), and Wnt signaling pathways (Figure 6C,6D).
qPCR verification of results
The results of our model were verified by qPCR analysis in tissues and cells, which showed that miR-142-3p was significantly down-regulated in CC tissues (P<0.001) and ME180 cells (P<0.05). Also, miR-100-3p was found to be significantly up-regulated in CC tissues (P<0.001) and HCC94 cells (P<0.01) (Figure 7). The qPCR results were consistent with the database analysis. The primers sequences are shown in Table 1.
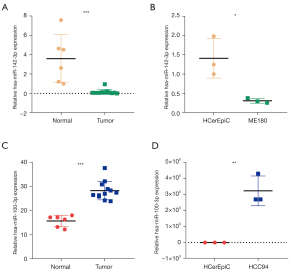
Table 1
Primer | Sequence, 5'‑3' |
---|---|
hsa-miR-142 | GCGCGTGTAGTGTTTCCTACTT |
hsa-miR-100 | GCGCGCAAGCTTGTATCTATA |
U6 | CTCGCTTCGGCAGCACAT |
hsa-miRNA-142-3p Mimic | UGUAGUGUUUCCUACUUUAUGGA |
UCCAUAAAGUAGGAAACACUACA | |
Mimic NC | UCACAACCUCCUAGAAAGAGUAGA |
UCUACUCUUUCUAGGAGGUUGUGA | |
hsa-miRNA-100-3p Inhibitor | CAUACCUAUAGAUACAAGCUUG |
Inhibitor NC | UCUACUCUUUCUAGGAGGUUGUGA |
hsa, homo sapiens; miR, microRNA; NC, negative control.
miR-142-3p and miR-100-3p regulated the proliferation, migration, and invasion of CC
The cellular behavior changes of CC influenced by miR-142-3p and miR-100-3p were explored through a series of functional experiments. In the colony formation assay, we found that the number of ME180 colonies in the mimic negative control (NC) group was greater than that of miR-142-3p mimic group (P<0.001; Figure 8A), and the number of HCC94 colonies in the inhibitor NC group was greater than that of miR-100-3p inhibitor group (P<0.001; Figure 8B). Thus, the up-regulated expression of miR-142-3p and low expression of miR-100-3p inhibited the formation of cell colonies. The primers sequences are shown in Table 1.
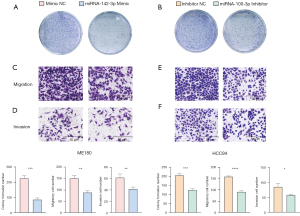
Transwell assays (with or without the Matrigel-coated membrane) indicated less active migration and invasion in the miR-142-3p mimic group compared to the mimic NC group (P<0.01 and P<0.01, respectively; Figure 8C,8D), and less active migration and invasion in the miR-100-3p inhibitor group than in the inhibitor NC group (P<0.0001 and P<0.05, respectively; Figure 8E,8F). These results indicated that miR-142-3p played an inhibiting role and miR-100-3p was a promoting factor in CC cell migration and invasion.
Discussion
Our study identified differentially-expressed miRNAs in CC by screening 12 miRNA-seq and TCGA tissues using bioinformatics technology. We subsequently constructed a prognostic risk model, the two-miRNA risk score, by combining expression data with the clinical parameters of patients. The model performed well in predicting prognosis in the training and testing sets, as well as in both sets together. ROC curve analysis of the 5-year overall survival rate suggested that the AUC value of each dataset was greater than 0.7. More importantly, multivariate Cox regression analysis revealed that the two-miRNA risk score is significantly and independently correlated with prognosis. Our analysis predicted cancer-related target genes and their pathways: pathways in cancer, T cell receptor signaling pathway, and the Wnt signaling pathway. These pathways have recognized functions in the development and progression of CC. Therefore, the results suggested that the two-miRNA risk score can potentially be used for predicting molecular pathogenesis, clinical progression, and prognosis of CC patients. Clinical studies are required to confirm whether the two-miRNA risk score is helpful for the diagnosis and personalized treatments in the early stage of CC.
One of the two miRNAs in our prognostic model—miR-100-3p—was correlated with a higher risk; that is, high expression was correlated with poor OS. Consistent with TCGA data, miR-100-3p expression was higher in CC cancerous cells than normal cells. Interestingly, miR-100-3p has inconsistent roles in different cancer types. Figueroa-González et al. (16) reported that miR-100-3p was over-expressed in head and neck cancer samples. A previous study reported that miR-100-3p is a tumor suppressor miRNA and directly targets BMPR2, thereby regulating the extracellular signal regulated kinase (ERK)/protein kinase B (AKT) and BCL2-associated X (Bax)/B-cell lymphoma-2 (Bcl2)-Caspase3 signaling pathways in gastric cancer (17). However, no previous reports on the role of miR-100-3p in CC could be found.
Our TCGA analysis indicated that miR-142-3p was associated with reduced risk. Previous studies found that miR-142-3p functioned as a tumor suppressor in CC and other tumors. Tang et al. (18) showed that miR-142-3p was down-regulated in CC cell lines compared with normal cervical epithelium cells. Deng et al. (19) reported that miR-142-3p can directly affect the expression Frizzled7 Receptor (FZD7), and thus, is an inhibitor of proliferation and invasion of CC cells. Metformin was found to upregulate miR-142-3p expression in CC in vitro and disrupts the MALAT1/miR-142-3p sponge, resulting in the combination of miR-142-3p and HMGA2, which subsequently inhibits CC invasion and migration (20). In breast cancer, miR-142-3p might serve as a tumor suppressor by targeting oncogenic HMGA2 (21). The expression of miR-142-3p is down-regulated in pancreatic cancer tissues and cell lines, and miR-142-3p inhibits the proliferation, migration, and invasion of pancreatic cancer cells by targeting NUCKS1 (22).
Our ROC curve analysis showed that the AUC values of our model in the training and testing sets, and in the two datasets were all between 0.7 and 0.8. It is worth noting that the univariate and multivariate Cox regression analyses in our study indicated a significant and independent correlation between the two-miRNA risk score and OS (HR =3.393, P<0.05).
There were some limitations in this study that should be noted. Firstly, we only used data from one single database to assign into the training and testing sets. Ideally, another set of data should be used for external testing. Secondly, the sample size for qPCR verification of the model was small; therefore, it is essential to recruit more CC patients and carry out a long-term follow-up study to confirm the results of this paper. In addition, the functions and detailed mechanisms of the two microRNAs clarified here require further studies.
Conclusions
We used 12 miRNA-seq and TCGA tissues to build a prognostic model for CC according to the expression of two miRNAs. ROC curve analysis suggested that the model had sound performance. Our qPCR experiment compared the expression of two microRNA in tissues and cell lines, and the actual results were identical to the model predictions. In this study, we constructed a two-miRNA risk score model with predictive potential, which will provide a new strategy for the individualized diagnosis and treatment of CC.
Acknowledgments
Funding: This work was financially supported by the National Natural Science Foundation (No. 81802747 and No. 81972450).
Footnote
Provenance and Peer Review: This article was commissioned by the Guest Editor (Danbo Wang) for the series “New Progress and Challenge in Gynecological Cancer” published in Annals of Translational Medicine. The article has undergone external peer review.
Reporting Checklist: The authors have completed the TRIPOD reporting checklist. Available at https://atm.amegroups.com/article/view/10.21037/atm-21-6451/rc
Data Sharing Statement: Available at https://atm.amegroups.com/article/view/10.21037/atm-21-6451/dss
Conflicts of Interest: All authors have completed the ICMJE uniform disclosure form (available at https://atm.amegroups.com/article/view/10.21037/atm-21-6451/coif). The series “New Progress and Challenge in Gynecological Cancer” was commissioned by the editorial office without any funding or sponsorship. JA reports funding from National Natural Science Foundation (No. 81802747). LW reports funding from National Natural Science Foundation (No. 81972450). The authors have no other conflicts of interest to declare.
Ethical Statement: The authors are accountable for all aspects of the work in ensuring that questions related to the accuracy or integrity of any part of the work are appropriately investigated and resolved. The samples were obtained with the written informed consent of all patients, and the study was conducted in accordance with the Declaration of Helsinki (as revised in 2013). The study was approved by the Ethics Committee of the National Cancer Center/National Clinical Research Center for Cancer/Cancer Hospital, Chinese Academy of Medical Sciences and Peking Union Medical College (20/207-2,403).
Open Access Statement: This is an Open Access article distributed in accordance with the Creative Commons Attribution-NonCommercial-NoDerivs 4.0 International License (CC BY-NC-ND 4.0), which permits the non-commercial replication and distribution of the article with the strict proviso that no changes or edits are made and the original work is properly cited (including links to both the formal publication through the relevant DOI and the license). See: https://creativecommons.org/licenses/by-nc-nd/4.0/.
References
- Sung H, Ferlay J, Siegel RL, et al. Global Cancer Statistics 2020: GLOBOCAN Estimates of Incidence and Mortality Worldwide for 36 Cancers in 185 Countries. CA Cancer J Clin 2021;71:209-49. [Crossref] [PubMed]
- Cancer Genome Atlas Research Network. Integrated genomic and molecular characterization of cervical cancer. Nature 2017;543:378-84. [Crossref] [PubMed]
- Guo F, Cofie LE, Berenson AB. Cervical Cancer Incidence in Young U.S. Females After Human Papillomavirus Vaccine Introduction. Am J Prev Med 2018;55:197-204. [Crossref] [PubMed]
- Muñoz N, Kjaer SK, Sigurdsson K, et al. Impact of human papillomavirus (HPV)-6/11/16/18 vaccine on all HPV-associated genital diseases in young women. J Natl Cancer Inst 2010;102:325-39. [Crossref] [PubMed]
- Uyar D, Rader J. Genomics of cervical cancer and the role of human papillomavirus pathobiology. Clin Chem 2014;60:144-6. [Crossref] [PubMed]
- Bartel DP. MicroRNAs: target recognition and regulatory functions. Cell 2009;136:215-33. [Crossref] [PubMed]
- Si W, Shen J, Zheng H, et al. The role and mechanisms of action of microRNAs in cancer drug resistance. Clin Epigenetics 2019;11:25. [Crossref] [PubMed]
- Kim J, Yao F, Xiao Z, et al. MicroRNAs and metastasis: small RNAs play big roles. Cancer Metastasis Rev 2018;37:5-15. [Crossref] [PubMed]
- Hatziapostolou M, Polytarchou C, Iliopoulos D. miRNAs link metabolic reprogramming to oncogenesis. Trends Endocrinol Metab 2013;24:361-73. [Crossref] [PubMed]
- Li M, Wu P, Yang Z, et al. miR-193a-5p promotes pancreatic cancer cell metastasis through SRSF6-mediated alternative splicing of OGDHL and ECM1. Am J Cancer Res 2020;10:38-59. [PubMed]
- Zhang P, Ji DB, Han HB, et al. Downregulation of miR-193a-5p correlates with lymph node metastasis and poor prognosis in colorectal cancer. World J Gastroenterol 2014;20:12241-8. [Crossref] [PubMed]
- Shu H, Hu J, Deng H. miR-1249-3p accelerates the malignancy phenotype of hepatocellular carcinoma by directly targeting HNRNPK. Mol Genet Genomic Med 2019;7:e00867. [Crossref] [PubMed]
- Saini J, Bandyopadhyay B, Pandey AD, et al. High-Throughput RNA Sequencing Analysis of Plasma Samples Reveals Circulating microRNA Signatures with Biomarker Potential in Dengue Disease Progression. mSystems 2020;5:00724-20. [Crossref] [PubMed]
- Schmelter C, Fomo KN, Perumal N, et al. Regulation of the HTRA2 Protease Activity by an Inhibitory Antibody-Derived Peptide Ligand and the Influence on HTRA2-Specific Protein Interaction Networks in Retinal Tissues. Biomedicines 2021;9:1013. [Crossref] [PubMed]
- Li T, Wang T, Yan L, et al. Identification of potential novel biomarkers for abdominal aortic aneurysm based on comprehensive analysis of circRNA-miRNA-mRNA networks. Exp Ther Med 2021;22:1468. [Crossref] [PubMed]
- Figueroa-González G, Carrillo-Hernández JF, Perez-Rodriguez I, et al. Negative Regulation of Serine Threonine Kinase 11 (STK11) through miR-100 in Head and Neck Cancer. Genes (Basel) 2020;11:1058. [Crossref] [PubMed]
- Peng CW, Yue LX, Zhou YQ, et al. miR-100-3p inhibits cell proliferation and induces apoptosis in human gastric cancer through targeting to BMPR2. Cancer Cell Int 2019;19:354. [Crossref] [PubMed]
- Tang T, Wong HK, Gu W, et al. MicroRNA-182 plays an onco-miRNA role in cervical cancer. Gynecol Oncol 2013;129:199-208. [Crossref] [PubMed]
- Deng B, Zhang Y, Zhang S, et al. MicroRNA-142-3p inhibits cell proliferation and invasion of cervical cancer cells by targeting FZD7. Tumour Biol 2015;36:8065-73. [Crossref] [PubMed]
- Xia C, Liang S, He Z, et al. Metformin, a first-line drug for type 2 diabetes mellitus, disrupts the MALAT1/miR-142-3p sponge to decrease invasion and migration in cervical cancer cells. Eur J Pharmacol 2018;830:59-67. [Crossref] [PubMed]
- Mansoori B, Duijf PHG, Mohammadi A, et al. MiR-142-3p targets HMGA2 and suppresses breast cancer malignancy. Life Sci 2021;276:119431. [Crossref] [PubMed]
- Zhu K, Zhang Z, Zhang H, et al. MiR-142-3p targeting NUCKS1 inhibits proliferation and invasion of pancreatic cancer cells. Artif Cells Nanomed Biotechnol 2020;48:415-24. [Crossref] [PubMed]
(English Language Editor: A. Kassem)