Radiomics for predicting revised hematoma expansion with the inclusion of intraventricular hemorrhage growth in patients with supratentorial spontaneous intraparenchymal hematomas
Introduction
Spontaneous intracranial hemorrhage (sICH), accounting for 10–15% of all strokes, is the most devastating subtype of stroke, associated with high mortality, unfavorable outcome, and high treatment cost (1-3). Patients with sICH may continue to bleed after the initial hemorrhage, which is known as hematoma expansion (HE). HE, which occurs in about one-fifth of patients with sICH, is a well-established independent predictor of worse outcomes and early clinical deterioration (4,5). HE represents a plausible therapeutic target for anti-expansion treatment in many clinical trials due to the characteristic of being potentially preventable (6-8). So far, there is no consensus definition of HE in different trials (9-12). More recently, many research results have revealed that intraventricular hemorrhage (IVH) occurs in up to 50% of patients with sICH, which growth independently predicts poor outcome, and have recommended that it be incorporated into definitions of HE (13-16). Yogendrakumar et al. concluded that the revised definitions including new IVH development or expansion improve overall prediction of 90-day outcomes compared to a standard definition of HE (≥6 mL or ≥33%) (17). Recent trials failed to improve outcomes for ICH therapies, despite a reduction in HE (18-20). Therefore, extending the current HE definition to the revised hematoma expansion (RHE) definition with the inclusion of IVH growth may be conducive to anti-expansion treatment. Previous studies have validated that several noncontrast computed tomography (NCCT) markers such as black hole sign, bend sign, swirl sign, satellite sign, and island sign are effective predictors for HE (21-26). However, these qualitative predictors have relatively low accuracy and sensitivity (27), which may lead to missed diagnoses and misdiagnoses, affecting the achievement of precision medicine. As a noninvasive method for the objective assessment of lesion heterogeneity, radiomics is an emerging tool that extracts high-throughput quantitative features from medical images (28,29). By using machine learning (ML) methods such as logistic regression, random forest, support vector machines, and the Bayesian algorithm, radiomics analysis integrates quantitative imaging features and clinical findings. In conjunction with other clinical information, these quantitative features can support clinical decision making (29). Although radiomics analyses have been recently applied to predict HE (12,30-33), few studies have tried to predict RHE. In this study, we hypothesized that the nomogram model, which incorporates clinical-semantic and radiomics features, can be used to identify supratentorial sICH patients at high risk for RHE and can outperform the other 2 models alone as well as the BRAIN score model (9), serving as a promising clinical prediction model for HE.
We present the following article in accordance with the TRIPOD reporting checklist (available at https://dx.doi.org/10.21037/atm-21-6158).
Methods
All procedures performed in this study involving human participants were in accordance with the Declaration of Helsinki (as revised in 2013). The study was approved by Ethics Committee of Qilu Hospital of Shandong University (No. KYLL-2019014). Individual consent for this retrospective analysis was waived.
Patient selection and data collection
Figure 1 illustrates patient enrollment. The data of 1,790 patients with a primary diagnosis of sICH admitted to center 1 (Qilu Hospital of Shandong University, Qingdao) and center 2 (the Affiliated Hospital of Qingdao University) from January 2015 to December 2020 were retrospectively reviewed. Patients aged ≥18 years were eligible for our study if the following criteria were met: (I) Initial CT scan was completed within 24 h of ictus, and (II) follow-up CT scan was performed within 48 h after the baseline CT scan. The exclusion criteria were as follows: (I) secondary hemorrhage due to an external cause such as trauma, hemorrhagic transformation after acute ischemic stroke, tumor, aneurysm, cerebral aneurysm, or vascular malformation; (II) inadequate data or severe image artifacts; (III) surgical interventions before follow-up CT scan; (IV) subtentorial ICH; (V) multifocal ICH. Finally, 376 patients with sICH from center 1 and 91 patients with sICH from center 2 were included in the current study. Demographic information (age, gender), clinical data (baseline Glasgow Coma Scale (GCS), onset-to-baseline CT time), laboratory results (international normalized ratio (INR), glucose, D-dimer), medical history (intracerebral hemorrhage, ischemic stroke, antiplatelet use), and radiological data (ICH location, IVH, baseline hematoma volume, swirl sign, satellite sign) were recorded in a standardized data collection form.
Imaging acquisition
All NCCT scans were performed by scanners using a standard protocol, with details described in the Appendix 1.
Imaging analysis
RHE was defined as an increase in hematoma volume of >33% or >6 mL or any IVH at follow-up CT scan (17). Two neuroradiologists independently reviewed all images in a blinded manner. They assessed the NCCT markers on baseline CT scans according the guideline (34). Hematoma location was classified as deep (basal ganglia and thalamus) or lobar (34). Discrepancies were settled by joint discussion of the 2 readers and a third reader. Baseline and follow-up hematoma were semi-automatically detected and segmented by a computer-assisted software named “Dr. Wise Hemorrhagic Stroke Analyzer” with deep convolutional neural networks.
BRAIN score
Based on 5 independent predictors including baseline ICH volume, recurrent ICH, anticoagulation with warfarin at onset, intraventricular extension, and number of hours to baseline CT from symptom onset, BRAIN scores were obtained for every patient according to the definition of Wang et al. (9). ICH volumes were obtained with the aforementioned software automatically. Being the most widely utilized prognostic model for HE based on NCCT images in recent clinical trials (9,35), the BRAIN score was chosen as the comparison since there is currently no recognized prediction score for RHE.
Feature extraction
The workflow of the radiomics analysis of hematoma is shown in Figure 2, which includes the steps of region of interest (ROI) segmentation, feature extraction, feature selection, model construction, model comparison, and evaluation.
We used the Deepwise multimodal research platform (https://keyan.deepwise.com, V1.6.2) to analyze the original DICOM format CT images of all cases.
Firstly, different image transformation methods were used to preprocess the original image, including high-pass filters, low-pass filters, wavelet filters, and Gaussian Laplacian filters with different λ parameters. Secondly, 3 types of radiomics features (first-order features, shape features, and texture features) were extracted from the original image and the preprocessed image, along with texture features consisting of Gray Level Co-occurrence Matrix (GLCM) features, Gray Level Run Length Matrix (GLRLM) features, Gray Level Size Zone Matrix (GLSZM) features, Gray Level Dependence Matrix (GLDM) features, and Neighboring Gray Tone Difference Matrix (NGTDM) features.
After feature extraction, each ROI extracted 2106 high-dimensional radiomics features, and the Z-score was used to standardize these features [Z = (X − mean)/SD].
Feature analysis
Firstly, we eliminated features with an intraclass correlation coefficient (ICC) value less than 0.8. We retained 1,492 features from the 2,106 radiomic features extracted from the segmented hematoma region of the initial CT image. Secondly, ANOVA was used to test the significance of a single feature between the hematoma-free and hematoma-enlarged group, and the statistically significant features were initially selected (P value <0.05). Then, the least absolute shrinkage and selection operator (LASSO) and logistic regression algorithms were used to select features and build ML models. The logistic regression machine learning was performed based on logistic regression analysis, searching the most fitting formula. Finally, 20 radiomics features with non-zero covariance became image predictors of HE. Details are presented in Table S1. In clinical and semantic feature analysis, univariate analysis was used to screen out features which had significant differences between groups (P<0.05).
Construction of multiple models
Firstly, we randomly divided the data set into a training set and a test set at a ratio of 8:2. In the training phase, in order to reduce the impact of the imbalance of positive and negative samples on the ML model, we first used the smote algorithm to resample the data and make the number of positive and negative samples consistent. In the training set, the model was trained after the data was divided by a 5-fold cross validation method. The performance of the model was tested on the test set.
In order to compare the prediction effects of clinical features, semantic features, radiomics features, and BRAIN scores on the classification of RHE and analyze their clinical value, we constructed 4 models, including: (I) BRAIN scores (BS); (II) clinical semantic model (CSM); (III) Radiomics model (RM); (IV) clinical semantic-radiomics model (CSRM), which contains significant clinical features, CT semantic features, and radiomics features.
We used logistic regression algorithms to train classification models. Five different ML algorithms, including the naïve Bayes (NB), random forest (RF), logistic regression (LR), support vector machine (SVM), and gradient boosting decision tree (GBDT) algorithms were used to construct models in the training cohort. These models were independently verified in the validation cohort. We used the output probability of the radiomics model as the Rad score, which can quantify the prediction probability of the radiomics model composed of high-dimensional features.
xk is one of the values of the radiomics features, ak is the coefficient of feature k.
In the CSRM, we combined clinical features, semantic features, and the Rad score.
Model evaluation
We used the area under the curve (AUC), accuracy, specificity, and sensitivity to evaluate the predictive performance of each model. Decision curves were drawn to display the relationship between the benefits and risks brought by different cut-off points in the different models. Cut off value was chosen as the biggest AUC value. Analysis of the calibration curves was performed for the calibration of the nomogram.
Statistical analysis
The statistical description of data was performed with R software (3.6.0) and Deepwise DxAI platform (https://dxonline.deepwise.com). ML algorithms and model evaluation were written in Python. Numerical variables were tested for normality. The independent sample t test was used for variables with a normal distribution, while the Wilcoxon rank sum test was used for variables with a non-normal distribution. Chi-square analysis or adjusted chi-square analysis were used for categorical variables. The DeLong test was used to compare the significance of the AUC difference of different models, and Spearman correlation was used to analyze the relationship between clinical features. In the bilateral significance test, P<0.05 was considered to be statistically significant.
Results
Patient characteristics
A total of 376 patients were enrolled in the study, including 236 males (62.8%) and 140 females (37.2%), with an average age of 59.9±13.3 years. Patients were assigned into 2 groups: a revised expander group (n=108) and a non-revised expander group (n=268). The patient characteristics in the training and test sets are summarized in Table 1.
Table 1
Variable | Training set (n=299) | Test set (n=77) | |||||
---|---|---|---|---|---|---|---|
No RHE (n=215) | RHE (n=84) | P value | No RHE (n=53) | RHE (n=24) | P value | ||
Age (years) | 58.479±12.931 | 62.048±13.812 | 0.036a | 63.679±12.643 | 56.750±14.411 | 0.036a | |
Male (%) | 139 (64.7) | 50 (59.5) | 0.409c | 31 (58.5) | 16 (66.7) | 0.496c | |
GCS | 13 (11, 15) | 10 (6.75, 13) | 0.001b | 13 (10, 14) | 12 (9, 12.25) | 0.041b | |
Onset to follow-up CT, h | 4.000 (2.000–13.000) |
2.500 (1.000–5.000) |
0.001b | 4.000 (2.500–12.000) |
1.650 (1.375–4.875) |
0.001b | |
Serum glucose, mmol/L | 6.670 (5.510–8.140) |
6.750 (6.115–9.000) |
0.088b | 6.480 (5.720–7.160) |
7.460 (5.820–9.310) |
0.192b | |
Platelet count, 109/L | 216.000 (181.250–258.500) |
217.500 (176.500–255.000) |
0.787b | 201.000 (163.750–240.250) |
229.500 (197.000–270.250) |
0.031b | |
INR | 1.060 (1.000–1.110) |
1.030 (0.965–1.105) |
0.179b | 1.065 (1.000–1.130) |
1.045 (1.018–1.062) |
0.218b | |
APTT, s | 29.400 (27.300–32.300) |
30.400 (27.500–33.700) |
0.186b | 30.450 (28.275–32.325) |
29.350 (28.025–30.825) |
0.380b | |
D-dimer, ug/mL | 0.330 (0.190–0.655) |
0.510 (0.310–0.965) |
0.005b | 0.470 (0.247–0.797) |
0.375 (0.180–1.163) |
0.667b | |
Baseline ICH volume, mL, n (%) | 12.577 (4.511–23.691) |
32.544 (9.764–49.477) |
0.000b | 18.369 (8.181–26.096) |
33.123 (24.125–55.783) |
0.002b | |
Prior cerebral hemorrhage, n (%), present | 24 (11.2) | 7 (8.3) | 0.471c | 7 (13.2) | 6 (25.0) | 0.342c | |
Prior ischemic stroke, n (%), present | 25 (11.6) | 9 (10.7) | 0.823c | 8 (15.1) | 4 (16.7) | 1.000c | |
Diabetes, n (%), present | 21 (9.8) | 7 (8.3) | 0.702c | 7 (13.2) | 5 (20.8) | 0.606c | |
Hypertension, n (%), present | 156 (72.6) | 57 (67.9) | 0.420c | 40 (75.5) | 17 (70.8) | 0.667c | |
Coronary artery disease, n (%), present | 20 (9.3) | 18 (21.4) | 0.005c | 9 (17.0) | 4 (16.7) | 1.000c | |
Alcohol consumption, n (%), present | 55 (25.6) | 12 (14.3) | 0.101c | 14 (26.4) | 3 (12.5) | 0.297c | |
Smoking, n (%), present | 47 (21.9) | 16 (19.0) | 0.757c | 14 (26.4) | 6 (25.0) | 0.783c | |
Previous anticoagulant therapy, n (%), present | 13 (6.0) | 5 (6.0) | 0.278c | 2 (3.8) | 2 (8.3) | 0.779c | |
Previous antiplatelet therapy, n (%), present | 24 (11.2) | 25 (29.8) | 0.000c | 11 (20.8) | 4 (16.7) | 0.913c | |
Hematoma location, n (%), deep | 169 (78.6) | 60 (71.4) | 0.188c | 38 (71.7) | 16 (66.7) | 0.655c | |
Black hole sign, n (%), present | 27 (12.6) | 23 (27.4) | 0.002c | 4 (7.5) | 5 (20.8) | 0.194c | |
Blend sign, n (%), present | 47 (21.9) | 29 (34.5) | 0.024c | 21 (39.6) | 11 (45.8) | 0.609c | |
Swirl sign, n (%), present | 69 (32.1) | 50 (59.5) | 0.000c | 16 (30.2) | 9 (39.1) | 0.446c | |
Island sign, n (%), present | 80 (37.2) | 48 (57.1) | 0.002c | 20 (37.7) | 18 (75.0) | 0.002c | |
Satellite sign, n (%), present | 51 (23.7) | 34 (40.5) | 0.004c | 17 (32.1) | 16 (66.7) | 0.004c | |
Midline shift, n (%), present | 160 (74.4) | 36 (42.9) | 0.000c | 34 (64.2) | 17 (70.8) | 0.566c | |
IVH, n (%), present | 45 (21.0) | 35 (41.7) | 0.000c | 37 (69.8) | 11 (45.8) | 0.044c |
a, two sample t test; b, Wilcoxon test; c, Chi-square test. RHE, revised hematoma expansion; GCS, Glasgow Coma Scale; INR, international normalized ratio; APTT, activated partial thromboplastin time; IVH, intraventricular hemorrhage.
BRAIN scores
The AUC of the training set was 0.7147, and the AUC of the test set was 0.6563 (Table 2). Comparing the model with the BRAIN score helps to better analyze the diagnostic significance of different models.
Table 2
Machine learning | Training | Test | Validation | |||||||||||
---|---|---|---|---|---|---|---|---|---|---|---|---|---|---|
ACC | SEN | SPE | AUC | ACC | SEN | SPE | AUC | ACC | SEN | SPE | AUC | |||
BS | 0.6622 | 0.7738 | 0.5708 | 0.7147 | 0.6486 | 0.7917 | 0.5370 | 0.6563 | 0.6374 | 0.7714 | 0.4921 | 0.6437 | ||
CSM | 0.7391 | 0.8095 | 0.6652 | 0.8065 | 0.6892 | 0.8333 | 0.5849 | 0.7054 | 0.6703 | 0.8000 | 0.5323 | 0.6864 | ||
RM | 0.8696 | 0.8810 | 0.8304 | 0.9158 | 0.7568 | 0.8750 | 0.6731 | 0.8124 | 0.7582 | 0.8286 | 0.6557 | 0.7843 | ||
CSRM | 0.9130 | 0.8929 | 0.8879 | 0.9429 | 0.8243 | 0.8148 | 0.9167 | 0.8447 | 0.8132 | 0.8571 | 0.7333 | 0.8287 |
BS, BRAIN score; CSM, clinical-semantic model; RM, radiomics model; CSRM, clinical-semantic radiomics model.
Radiomics models based on different ML algorithms
We tested 5 classic ML algorithms to screen out the best ML classifiers and found that the LR algorithm had the best performance on the training set (AUC =0.9158) and test set (AUC =0.8124). The results are shown in Table 3.
Table 3
Model | Training | Test | |||||||
---|---|---|---|---|---|---|---|---|---|
ACC | SEN | SPE | AUC | ACC | SEN | SPE | AUC | ||
SVM | 0.8361 | 0.8452 | 0.7885 | 0.8545 | 0.7403 | 0.7500 | 0.6724 | 0.8012 | |
RF | 0.7559 | 0.7381 | 0.6949 | 0.8084 | 0.7013 | 0.7083 | 0.6271 | 0.7300 | |
GBDT | 0.7826 | 0.6905 | 0.7333 | 0.8204 | 0.6883 | 0.6667 | 0.6167 | 0.7240 | |
LR | 0.8696 | 0.8810 | 0.8304 | 0.9158 | 0.7568 | 0.8750 | 0.6731 | 0.8124 | |
NB | 0.7190 | 0.6429 | 0.6598 | 0.7328 | 0.6494 | 0.3333 | 0.6176 | 0.6349 |
ACC, accuracy; SEN, sensitivity; SPE, specificity; AUC, area under the curve; SVM, support vector machine; RF, random forest; GBDT, gradient boosting decision tree; LR, logistic regression; NB, naïve Bayes.
Analysis of clinical and semantic features
After univariate analysis of the clinical features and semantic features with significant differences between the groups, 7 clinical factors were finally selected. The results are shown in Table 4.
Table 4
Variable | Univariate analysis | |
---|---|---|
Odds ratio (95% CI) | P value | |
Rad score | 12.492 (5.741–28.685) | <0.001 |
CT time from ICH onset, h | 0.346 (0.203–0.581) | <0.001 |
Satellite sign, present | 2.614 (1.551–4.422) | <0.001 |
Swirl sign, present | 2.388 (1.432–4.009) | <0.001 |
D-dimer, ug/mL | 0.983 (0.899–1.042) | 0.0062 |
Baseline midline shift, present | 3.398 (2.016–5.777) | <0.001 |
Serum glucose, mmol/L | 1.049 (0.95–1.158) | 0.00328 |
Baseline ICH volume, mL | 2.204 (1.626–3.025) | <0.001 |
ICH, intracerebral hemorrhage; CI, confidence interval.
We constructed a classification model (CSM) based on clinical and semantic features. Its AUC on the training set was 0.81 and the AUC on the test set was 0.71 (Table 2).
Comparison between models
We compared the performances of all models on the training set and test set (Table 2), and tested their differences in AUC. On the training, test, and validation sets, the AUC of the CSRM was significantly higher than the RM, CSM, and BS (P<0.05). The AUC of the RM was higher than the CSM and BS (P<0.05). Among them, the CSRM had the best performance while the BS had the worst performance.
In order to intuitively quantify the probability of each factor in predicting the expansion of cerebral hematoma with the CSRM, we converted the value of each factor into a score, and calculated the total score of all risk factors to obtain the corresponding prediction probability of HE, as shown in Figure 3. At the same time, we carried out model correction, and the results showed that the sample classification correctness of the CSRM was excellent.
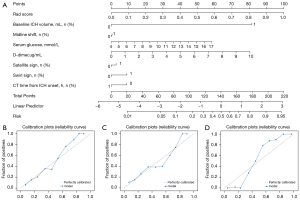
Figure 4 shows the receiver operating characteristic curves (ROC curves) of the 4 models in the training set, test set, and validation set. The results showed that the AUC of the CSRM and RM were larger.
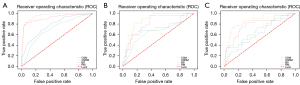
Figure 5 shows the decision curve analysis (DCA) curves of the 4 models on the training set and the test set. The clinical net benefits of the CSRM were always significantly higher than those of the RM, CSM, and BS on the training (Figure 5A), test (Figure 5B), and validation (Figure 5C) sets.
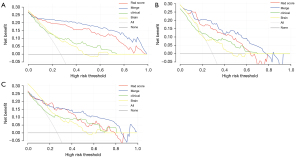
Discussion
In this retrospective study, we constructed a CSRM to discriminate early RHE after supratentorial sICH. This combined model can be completed in the ultra early phase of sICH and is only based on NCCT without contrast injection, which provides a fast and auxiliary method to identify patients with a high risk of RHE. Besides, with relatively lower examination cost and technical requirements, NCCT is widely used in almost every emergency room.
In the current study, several factors including midline shift, NCCT time from sICH onset, baseline ICH volume, D-dimer, serum glucose, swirl sign, and satellite sign were independently associated with RHE, which were pathologically plausible and constituted the CSM. Previous studies have indicated that midline shift was a predictor for poor outcome and HE in ICH patients (36,37), which is consistent with the findings of our study. The risk of RHE was associated with a short NCCT time from sICH onset, which may be due to further hematoma enlargement occurring within the first few hours after onset (38,39). The baseline hematoma volume was a potential predictor of HE, though with a contradictory effect. Our study result was consistent with most studies, in that the larger the volume, the higher the risk of HE (40). However, this is contrary to a small number of studies with a diametrically opposite conclusion (12). A larger baseline ICH may reflect multiple bleeding points or perilesional hemorrhage (9). D-dimer, as a marker of fibrin turnover reflecting an impairment in the coagulation and fibrinolysis pathways, may lead to early HE and may be related to intraventricular extension (41,42). Elevated serum glucose levels impair brain microvascular endothelial function and accelerate the destruction of the blood-brain barrier, which is likely to cause HE (43). Swirl sign, as a manifestation of heterogeneous density, constitutes more immature bleed areas leading to subsequent HE. This sign has been confirmed as an independent predictor of HE in previous studies (44), which is consistent with our results. Satellite signs have been explained as multifocal active bleeding from peripheral arterioles or reperfusion injury resulting from perihematomal edema, and were proven to be reliable predictors for HE and RHE (44). Our CSM demonstrated suboptimal discrimination performance, with AUCs of 0.81 in the training set and 0.71 in the test set.
This result indicates that it is not good enough to predict RHE based on extra-hematoma and visual assessment information alone. Therefore, quantitative predictors hidden in hematoma beyond vision have been sought.
In our study, the RM demonstrated better discrimination performance and higher sensitivity than the CSM in all 3 sets. By only requiring the delineation of the relatively well-defined boundaries of the hematoma rather than complex NCCT signs, the requirement for clinician experience is significantly reduced. An important aspect of constructing an NCCT radiomics-based model for prediction is eliminating unstable features caused by observer difference (45). After screening by the ICC value, ANOVA test, LASSO, and LR algorithms, only 20 of the 2,106 radiomics features associated with RHE of sICH were identified in this study. These 20 features contained the 3 types of aforementioned features as follows: first-order features, shape features, and texture features, which described the heterogeneity and shape of the hematoma associated with active bleeding and multifocal hemorrhage (46). The ability of only histogram-based texture features for quantifying hematoma heterogeneity in the prediction of HE has been previously verified (31). Subsequently, several studies based on R-score evaluated the risk of HE with AUCs of 0.69–0.89 (12,47), lower than our result of 0.92. Another study showed that the optimal accuracy (ACC) of RM in predicting HE was 0.88, which was slightly higher than our result of 0.87, but the sample size of 251 was significantly smaller than our study (32).
Furthermore, we compared the ability of 5 classic ML algorithms, LR, SVM, RF, GBDT, and NB, in constructing the RM to discriminate RHE after supratentorial sICH. As the tree-based ensemble model appeared to be overfitted on the training set, the regularized LR algorithm achieves a more robust performance in this study and the result is consistent with a previous study (48).
By adding the Rad score to the CSM, we developed the CSRM, which showed significantly better discriminating efficacy for RHE in sICH patients compared with the CSM in the training and test set (all P<0.05), indicating the statistical contribution of the R-score to the construction of the merged model. Additionally, the CSRM obtained more clinical net benefits in the prediction of RHE with almost all of the range of threshold probabilities compared with the CSM and RM. Compared with other research, more intra-hematoma and extra-hematoma characteristics are considered in our model, for more accurately predicting RHE. These results indicate that the combined application of intra-hematoma and extra-hematoma characteristics is expected to guide clinical practice and improve precision medicine. Compared with the test set, the results did not show a big change in the validation set, indicating that our model has good generalization performance.
The predictive ability of the BRAIN score for HE, which was derived from a prospective large sample study based on simple routinely available variables, has been proven (9), but has not been validated in RHE. In our study, the discrimination efficacy of the BS for RHE was only acceptable with an optimal AUC of 0.71 and an ACC of 0.66 in the training set and an AUC of 0.66 and ACC of 0.65 in the test set, which was significantly lower than the RM and CSRM (P<0.05). Furthermore, DCA showed its limitation in clinical practice. Although at this stage it is speculative and part of future studies, the proposed ML classifier may provide promising complementary results. The biggest drawback of the BS was the lack of NCCT markers describing the hematoma density, which might contribute to the relatively lower discernment. In this study, the top 5 predictors with first-order feature and second-order texture features in the RM are all linking to hematoma heterogeneity. Hence, the CSRM can be used as a supportive tool to augment conventional multidimensional scoring systems and to improve prognostic decisions for clinicians to achieve precision medicine.
One strength of our study is that the AUC and ACC of the RM and CSRM in the external validation set were similar to the test set, which means our models have stable predictive performance for RHE and can be further extended to other hospitals. Another strength of this study is the incorporation of the classical scoring system (BS) and the comparison of its prediction ability for RHE in supratentorial sICH patients with radiomics-related models (RM and CSRM). Since our quantitative feature analysis outperformed classical scoring systems, the application of the proposed ML approach may be of value for future randomized clinical trials. Challenges and opportunities to optimize clinical research and randomized trials in ICH are ongoing (49). The ML approach may simplify trial procedures by performing an imaging-based prediction of RHE. Furthermore, this approach may be applied in telemedicine and the remote prediction of RHE in poor regions lacking medical resources. Taken together, the proposed method integrates the advantages from quantitative radiomic and clinical semantic features and ML algorithms and relates the employed predictors to well-known imaging and clinical characteristics.
Several limitations deserve comment in this study. First, it was a double-center retrospective study with a relatively small sample size. Its reliability in other external cohorts needs to be validated with prospective, multi-center, large sample studies. Additionally, infratentorial hematomas were excluded. Therefore, the CSRM cannot be applied to patients with brainstem or cerebellar hemorrhage. Furthermore, perihematomal data such as edema was not included, which may limit the use of the CSRM and RM.
Conclusions
The addition of the Rad score to clinical-semantic factors can significantly improve the discriminating efficacy for RHE in sICH patients, and outperforms the classical BRAIN scoring system. These results support the potential of the CSRM to augment conventional image analysis, aid selection of those at risk of RHE, and simplify trial procedures. In the very near future, the CSRM may be incorporated into therapeutic trials once the challenges around predictive accuracy and variability of radiomics features have been settled.
Acknowledgments
Funding: This research was supported by Qingdao science and technology demonstration and guidance project (grant numbers 20-3-4-37-nsh) and the Scientific Research Foundation of Qilu Hospital of Shandong University (Qingdao) (QDKY2020QN04).
Footnote
Reporting Checklist: The authors have completed the TRIPOD reporting checklist. Available at https://dx.doi.org/10.21037/atm-21-6158
Data Sharing Statement: Available at https://dx.doi.org/10.21037/atm-21-6158
Conflicts of Interest: All authors have completed the ICMJE uniform disclosure form (available at https://dx.doi.org/10.21037/atm-21-6158). HD, CH, JX, KL, HW, and KH are from Deepwise AI Lab, Beijing Deepwise & League of PHD Technology Co., Ltd. The other authors have no conflicts of interest to declare.
Ethical Statement: The authors are accountable for all aspects of the work in ensuring that questions related to the accuracy or integrity of any part of the work are appropriately investigated and resolved. All procedures performed in this study involving human participants were in accordance with the Declaration of Helsinki (as revised in 2013). The study was approved by Ethics Committee of Qilu Hospital of Shandong University (No. KYLL-2019014). Individual consent for this retrospective analysis was waived.
Open Access Statement: This is an Open Access article distributed in accordance with the Creative Commons Attribution-NonCommercial-NoDerivs 4.0 International License (CC BY-NC-ND 4.0), which permits the non-commercial replication and distribution of the article with the strict proviso that no changes or edits are made and the original work is properly cited (including links to both the formal publication through the relevant DOI and the license). See: https://creativecommons.org/licenses/by-nc-nd/4.0/.
References
- van Asch CJ, Luitse MJ, Rinkel GJ, et al. Incidence, case fatality, and functional outcome of intracerebral haemorrhage over time, according to age, sex, and ethnic origin: a systematic review and meta-analysis. Lancet Neurol 2010;9:167-76. [Crossref] [PubMed]
- Keep RF, Hua Y, Xi G. Intracerebral haemorrhage: mechanisms of injury and therapeutic targets. Lancet Neurol 2012;11:720-31. [Crossref] [PubMed]
- Qureshi AI, Mendelow AD, Hanley DF. Intracerebral haemorrhage. Lancet 2009;373:1632-44. [Crossref] [PubMed]
- Delcourt C, Huang Y, Arima H, et al. Hematoma growth and outcomes in intracerebral hemorrhage: the INTERACT1 study. Neurology 2012;79:314-9. [Crossref] [PubMed]
- Dowlatshahi D, Demchuk AM, Flaherty ML, et al. Defining hematoma expansion in intracerebral hemorrhage: relationship with patient outcomes. Neurology 2011;76:1238-44. [Crossref] [PubMed]
- Sprigg N, Flaherty K, Appleton JP, et al. Tranexamic acid for hyperacute primary IntraCerebral Haemorrhage (TICH-2): an international randomised, placebo-controlled, phase 3 superiority trial. Lancet 2018;391:2107-15. [Crossref] [PubMed]
- Steiner T, Bösel J. Options to restrict hematoma expansion after spontaneous intracerebral hemorrhage. Stroke 2010;41:402-9. [Crossref] [PubMed]
- Qureshi AI, Palesch YY, Barsan WG, et al. Intensive Blood-Pressure Lowering in Patients with Acute Cerebral Hemorrhage. N Engl J Med 2016;375:1033-43. [Crossref] [PubMed]
- Wang X, Arima H, Al-Shahi Salman R, et al. Clinical prediction algorithm (BRAIN) to determine risk of hematoma growth in acute intracerebral hemorrhage. Stroke 2015;46:376-81. [Crossref] [PubMed]
- Morotti A, Dowlatshahi D, Boulouis G, et al. Predicting Intracerebral Hemorrhage Expansion With Noncontrast Computed Tomography: The BAT Score. Stroke 2018;49:1163-9. [Crossref] [PubMed]
- Miyahara M, Noda R, Yamaguchi S, et al. New Prediction Score for Hematoma Expansion and Neurological Deterioration after Spontaneous Intracerebral Hemorrhage: A Hospital-Based Retrospective Cohort Study. J Stroke Cerebrovasc Dis 2018;27:2543-50. [Crossref] [PubMed]
- Ma C, Zhang Y, Niyazi T, et al. Radiomics for predicting hematoma expansion in patients with hypertensive intraparenchymal hematomas. Eur J Radiol 2019;115:10-5. [Crossref] [PubMed]
- Yogendrakumar V, Ramsay T, Fergusson D, et al. New and expanding ventricular hemorrhage predicts poor outcome in acute intracerebral hemorrhage. Neurology 2019;93:e879-88. [Crossref] [PubMed]
- Li Q, Li R, Zhao LB, et al. Intraventricular Hemorrhage Growth: Definition, Prevalence and Association with Hematoma Expansion and Prognosis. Neurocrit Care 2020;33:732-9. [Crossref] [PubMed]
- Moullaali TJ, Sato S, Wang X, et al. Prognostic significance of delayed intraventricular haemorrhage in the INTERACT studies. J Neurol Neurosurg Psychiatry 2017;88:19-24. [Crossref] [PubMed]
- Steiner T, Diringer MN, Schneider D, et al. Dynamics of intraventricular hemorrhage in patients with spontaneous intracerebral hemorrhage: risk factors, clinical impact, and effect of hemostatic therapy with recombinant activated factor VII. Neurosurgery 2006;59:767-73; discussion 773-4. [Crossref] [PubMed]
- Yogendrakumar V, Ramsay T, Fergusson DA, et al. Redefining Hematoma Expansion With the Inclusion of Intraventricular Hemorrhage Growth. Stroke 2020;51:1120-7. [Crossref] [PubMed]
- Mayer SA, Brun NC, Begtrup K, et al. Efficacy and safety of recombinant activated factor VII for acute intracerebral hemorrhage. N Engl J Med 2008;358:2127-37. [Crossref] [PubMed]
- Qureshi AI, Foster LD, Lobanova I, et al. Intensive Blood Pressure Lowering in Patients with Moderate to Severe Grade Acute Cerebral Hemorrhage: Post Hoc Analysis of Antihypertensive Treatment of Acute Cerebral Hemorrhage (ATACH)-2 Trial. Cerebrovasc Dis 2020;49:244-52. [Crossref] [PubMed]
- Baharoglu MI, Cordonnier C, Al-Shahi Salman R, et al. Platelet transfusion versus standard care after acute stroke due to spontaneous cerebral haemorrhage associated with antiplatelet therapy (PATCH): a randomised, open-label, phase 3 trial. Lancet 2016;387:2605-13. [Crossref] [PubMed]
- Ng D, Churilov L, Mitchell P, et al. The CT Swirl Sign Is Associated with Hematoma Expansion in Intracerebral Hemorrhage. AJNR Am J Neuroradiol 2018;39:232-7. [Crossref] [PubMed]
- Li Q, Zhang G, Huang YJ, et al. Blend Sign on Computed Tomography: Novel and Reliable Predictor for Early Hematoma Growth in Patients With Intracerebral Hemorrhage. Stroke 2015;46:2119-23. [Crossref] [PubMed]
- Li Q, Zhang G, Xiong X, et al. Black Hole Sign: Novel Imaging Marker That Predicts Hematoma Growth in Patients With Intracerebral Hemorrhage. Stroke 2016;47:1777-81. [Crossref] [PubMed]
- Yu Z, Zheng J, Ali H, et al. Significance of satellite sign and spot sign in predicting hematoma expansion in spontaneous intracerebral hemorrhage. Clin Neurol Neurosurg 2017;162:67-71. [Crossref] [PubMed]
- Li Q, Liu QJ, Yang WS, et al. Island Sign: An Imaging Predictor for Early Hematoma Expansion and Poor Outcome in Patients With Intracerebral Hemorrhage. Stroke 2017;48:3019-25. [Crossref] [PubMed]
- Yang WS, Zhang SQ, Shen YQ, et al. Noncontrast Computed Tomography Markers as Predictors of Revised Hematoma Expansion in Acute Intracerebral Hemorrhage. J Am Heart Assoc 2021;10:e018248. [Crossref] [PubMed]
- Morotti A, Boulouis G, Romero JM, et al. Blood pressure reduction and noncontrast CT markers of intracerebral hemorrhage expansion. Neurology 2017;89:548-54. [Crossref] [PubMed]
- Mayerhoefer ME, Materka A, Langs G, et al. Introduction to Radiomics. J Nucl Med 2020;61:488-95. [Crossref] [PubMed]
- Gillies RJ, Kinahan PE, Hricak H. Radiomics: Images Are More than Pictures, They Are Data. Radiology 2016;278:563-77. [Crossref] [PubMed]
- Zhan C, Chen Q, Zhang M, et al. Radiomics for intracerebral hemorrhage: are all small hematomas benign? Br J Radiol 2021;94:20201047. [Crossref] [PubMed]
- Shen Q, Shan Y, Hu Z, et al. Quantitative parameters of CT texture analysis as potential markersfor early prediction of spontaneous intracranial hemorrhage enlargement. Eur Radiol 2018;28:4389-96. [Crossref] [PubMed]
- Xie H, Ma S, Wang X, et al. Noncontrast computer tomography-based radiomics model for predicting intracerebral hemorrhage expansion: preliminary findings and comparison with conventional radiological model. Eur Radiol 2020;30:87-98. [Crossref] [PubMed]
- Chen Q, Zhu D, Liu J, et al. Clinical-radiomics Nomogram for Risk Estimation of Early Hematoma Expansion after Acute Intracerebral Hemorrhage. Acad Radiol 2021;28:307-17. [Crossref] [PubMed]
- Morotti A, Boulouis G, Dowlatshahi D, et al. Standards for Detecting, Interpreting, and Reporting Noncontrast Computed Tomographic Markers of Intracerebral Hemorrhage Expansion. Ann Neurol 2019;86:480-92. [Crossref] [PubMed]
- Huynh TJ, Aviv RI, Dowlatshahi D, et al. Validation of the 9-Point and 24-Point Hematoma Expansion Prediction Scores and Derivation of the PREDICT A/B Scores. Stroke 2015;46:3105-10. [Crossref] [PubMed]
- Yang WS, Li Q, Li R, et al. Defining the Optimal Midline Shift Threshold to Predict Poor Outcome in Patients with Supratentorial Spontaneous Intracerebral Hemorrhage. Neurocrit Care 2018;28:314-21. [Crossref] [PubMed]
- Tang SC, Huang SJ, Jeng JS, et al. Third ventricle midline shift due to spontaneous supratentorial intracerebral hemorrhage evaluated by transcranial color-coded sonography. J Ultrasound Med 2006;25:203-9. [Crossref] [PubMed]
- Hemphill JC 3rd, Greenberg SM, Anderson CS, et al. Guidelines for the Management of Spontaneous Intracerebral Hemorrhage: A Guideline for Healthcare Professionals From the American Heart Association/American Stroke Association. Stroke 2015;46:2032-60. [Crossref] [PubMed]
- Davis SM, Broderick J, Hennerici M, et al. Hematoma growth is a determinant of mortality and poor outcome after intracerebral hemorrhage. Neurology 2006;66:1175-81. [Crossref] [PubMed]
- Brouwers HB, Chang Y, Falcone GJ, et al. Predicting hematoma expansion after primary intracerebral hemorrhage. JAMA Neurol 2014;71:158-64. [Crossref] [PubMed]
- Fujii Y, Takeuchi S, Harada A, et al. Hemostatic activation in spontaneous intracerebral hemorrhage. Stroke 2001;32:883-90. [Crossref] [PubMed]
- Delgado P, Alvarez-Sabín J, Abilleira S, et al. Plasma d-dimer predicts poor outcome after acute intracerebral hemorrhage. Neurology 2006;67:94-8. [Crossref] [PubMed]
- Allen CL, Bayraktutan U. Antioxidants attenuate hyperglycaemia-mediated brain endothelial cell dysfunction and blood-brain barrier hyperpermeability. Diabetes Obes Metab 2009;11:480-90. [Crossref] [PubMed]
- Yu Z, Zheng J, He M, et al. Accuracy of swirl sign for predicting hematoma enlargement in intracerebral hemorrhage: a meta-analysis. J Neurol Sci 2019;399:155-60. [Crossref] [PubMed]
- Xu W, Ding Z, Shan Y, et al. A Nomogram Model of Radiomics and Satellite Sign Number as Imaging Predictor for Intracranial Hematoma Expansion. Front Neurosci 2020;14:491. [Crossref] [PubMed]
- Boulouis G, Morotti A, Brouwers HB, et al. Association Between Hypodensities Detected by Computed Tomography and Hematoma Expansion in Patients With Intracerebral Hemorrhage. JAMA Neurol 2016;73:961-8. [Crossref] [PubMed]
- Pszczolkowski S, Manzano-Patrón JP, Law ZK, et al. Quantitative CT radiomics-based models for prediction of haematoma expansion and poor functional outcome in primary intracerebral haemorrhage. Eur Radiol 2021;31:7945-59. [Crossref] [PubMed]
- Song Z, Guo D, Tang Z, et al. Noncontrast Computed Tomography-Based Radiomics Analysis in Discriminating Early Hematoma Expansion after Spontaneous Intracerebral Hemorrhage. Korean J Radiol 2021;22:415-24. [Crossref] [PubMed]
- Hemorrhagic Stroke Academia Industry (HEADS) Roundtable Participants. Second HEADS Roundtable Participants. Recommendations for Clinical Trials in ICH: The Second Hemorrhagic Stroke Academia Industry Roundtable. Stroke 2020;51:1333-8. [Crossref] [PubMed]