Total nodule number as an independent prognostic factor in resected stage III non-small cell lung cancer: a deep learning-powered study
Introduction
Lung cancer is a leading cause of cancer-related death worldwide (1). As early detection of cancer is important for decreasing mortality, multiple randomized trials and guidelines recommend lung cancer screening using low-dose computed tomography (LDCT) for high-risk individuals (2-7). With the adoption of LDCT for lung cancer screening, the number of chest CT scans has increased dramatically each year (8). To address the repetitive and onerous task of dealing with images that are mostly normal, computer-aided detection/diagnosis (CAD), which could perform the task consistently and tirelessly, has become extremely appealing (9).
Since 2002, CAD, supported by machine learning techniques, has been utilized to detect pulmonary nodules (PNs) (10). Although standardized CAD systems have been shown to improve diagnostic accuracy, few have been implemented in actual clinical practice due to their high dependence on image processing and false positive rates (11,12). In recent years, deep learning-based AI algorithms using convolutional neural networks (CNNs) have attracted considerable attention in the area of machine learning. The key advantage that CNNs have over conventional CAD techniques is their ability to self-learn previously unknown features, maximizing classification accuracy with limited direct supervision (13). The use of CNNs has led to a significant reduction in false positives in PN detection, recognition, segmentation, and classification (14-19), thus laying the foundation for the extensive clinical application of deep learning-based AI algorithms. The first deep learning-based AI algorithm for PN detection approved by the United States Food and Drug Administration (FDA) was used to guarantee PN detection performance in this study. Compared with AI algorithms reported in proof-of-concept studies, its robustness and generalizability have been widely validated in multiple medical centers and proven valuable in enhancing imaging report standardization and improving clinical workflow (20-22).
The key issue in the management of incidental PNs detected on CT images is to differentiate between benign and malignant nodules. Radiological features, such as larger nodule size, upper lobe location, marginal spiculation, and faster growth rate are generally considered risk factors for malignancy (23-28). These principles mainly focus on the assessment of the largest or most suspicious nodule. However, although approximately 50% of the patients with detected PNs have multiple nodules (29), nodule multiplicity, which is a potential indicator for malignancy, is commonly overlooked. Only limited data concerning the relationship between TNN and lung cancer probability are available. In the Pan-Canadian Early Detection of Lung Cancer Study (PanCan) and the British Columbia Cancer Agency (BCCA) cancer screening trials, lower TNN was associated with an increased risk of lung cancer (23). However, another study analyzing patients from the Dutch-Belgian Lung Cancer Screening trial (NELSON) showed that the risk of lung cancer increased as the TNN rose from 1 to 4 but decreased in patients with 5 or more nodules (29).
The results of the abovementioned screening trials indicated that TNN was either negatively or not significantly associated with lung cancer probability, which might reflect a low incidence of multiple malignancies in the screening population (30). However, for patients with a high pretest probability of malignancy, it remains unknown whether TNN plays a role in (I) determining lung cancer probability with multiple pulmonary sites of involvement, (II) distinguishing multiple primary lung cancers (MPLC) from intrapulmonary metastasis (IPM), and (III) prognosis. This study aimed to calculate the TNN detected on preoperative CT images using a CNN-based AI algorithm and to deeply explore the relationship between AI-detected TNN and survival outcomes in patients with resectable stage I–III NSCLC. We report the following article in accordance with the Transparent Reporting of a multivariable prediction model for Individual Prognosis or Diagnosis (TRIPOD) statement checklist (available at https://atm.amegroups.com/article/view/10.21037/atm-21-3231/rc).
Methods
Patients
We retrospectively reviewed the medical records of patients pathologically diagnosed with stage I–III NSCLC [according to the 8th edition of the American Joint Committee on Cancer (AJCC) prognostic group] who had undergone surgical resection at the Department of Thoracic Surgery at the Peking University People’s Hospital from October 2005 to December 2018. Only patients who received a preoperative chest CT scan within 90 days prior to surgery at the institution were included. Patients were excluded if 1 or more of the following conditions were met: (I) had already received neoadjuvant therapy, (II) surgical margin was positive, (III) perioperative death occurred within 30 days, or (IV) the follow-up information was inadequate.
Routine follow-up after the surgical intervention included an outpatient department visit every 3 months for the first 2 years and at 6-month intervals thereafter. For patients who failed to present at the clinic, follow-up information was collected via telephone call. We diagnosed recurrence based on physical and imaging examinations and confirmed the diagnosis histologically when clinically feasible. Secondary primary lung cancer was differentiated from intrapulmonary metastases using either the Martini-Melamed criteria or a comprehensive histological assessment (31).
AI-powered PN detection
InferRead CT Lung (https://global.infervision.com/product/30.html), a widely used deep learning-based AI algorithm developed by InferVision (Beijing, China), was applied for PN detection in this study, and only the patient’s last chest CT scan before surgery was used. First, PNs were detected by the AI algorithm, and the TNN was calculated accordingly. Next, PNs were classified according to their lobar distribution (left lower lobe, left upper lobe, right lower lobe, right middle lobe, and right upper lobe), location [same lobe as the primary tumor (same-lobe), ipsilateral lobe different from the primary tumor (same-side), and contralateral lobe (other-side)], and type [solid nodule, mixed ground-glass nodule (m-GGN), pure ground-glass nodule (p-GGN), calcific nodule, and perifissural nodule]. In addition, solid and subsolid (m-GGN and p-GGN) nodules were categorized based on their size.
Statistical analysis
Continuous variables were presented as a median with an interquartile range (IQR) and were analyzed using Wilcoxon’s rank-sum test and one-way analysis of variance (ANOVA). Categorical variables were presented as frequencies and percentages. Survival curves were compared using the Kaplan-Meier method with a log-rank test, and Cox proportional hazards models were constructed to determine the independent prognostic factors.
In the stage III cohort, maximally selected log-rank statistics were used to determine the optimal nodule number cutoff value for predicting OS. Patients were then categorized into lower- and higher-nodule number groups according to the estimated cutoff value. Furthermore, a least absolute shrinkage and selection operator (LASSO)-based Cox regression model with cross-validation was used to select the most useful prognostic features among all categories of the AI-detected nodule numbers. Finally, survival tree analysis was conducted to generate a tree-based model for survival data using log-rank test statistics for recursive partitioning.
All the statistical analyses were executed using R version 4.0.0 for Windows (R Foundation for Statistical Computing, Vienna, Austria). All the statistical tests were 2-sided, and P values of 0.05 or less were considered statistically significant.
Ethical statement
The study was conducted in accordance with the Declaration of Helsinki (as revised in 2013). The study involving human participants was reviewed and approved by the Institutional Review Board of Peking University People’s Hospital (2020PHB385-01). Individual consent for this retrospective analysis was waived.
Results
Characteristics of participants and nodules
A total of 2,126 patients who underwent surgical resection for stage I–III NSCLC and had accessible preoperative chest CT scans were included in this study. The median follow-up time was 33 months (IQR, 21 to 48). The demographic and clinicopathological characteristics of the patients are summarized in Table 1.
Table 1
Variables | Value |
---|---|
Age (years) | |
Median [IQR] | 61 [54–68] |
Gender | |
Male | 998 (46.9%) |
Female | 1,128 (53.1%) |
Smoking history | |
No | 1,456 (68.5%) |
Yes | 670 (31.5%) |
Comorbidity | |
No | 850 (40.0%) |
Yes | 1,276 (60.0%) |
Surgical approach | |
VATS | 1,997 (93.9%) |
VATS converted to open | 61 (2.9%) |
Open | 68 (3.2%) |
Surgical procedure | |
Sublobar resection | 636 (29.9%) |
Lobectomy | 1,419 (66.8%) |
Sleeve lobectomy | 39 (1.8%) |
Pneumonectomy | 32 (1.5%) |
Histologic type | |
Adenocarcinoma | 1,780 (83.7%) |
Squamous cell carcinoma | 280 (13.2%) |
Others | 66 (3.1%) |
Pathologic T stage | |
T1 | 1,383 (65.1%) |
T2 | 579 (27.2%) |
T3 | 115 (5.4%) |
T4 | 49 (2.3%) |
Pathologic N stage | |
N0 | 1,765 (83.0%) |
N1 | 145 (6.8%) |
N2 | 216 (10.2%) |
AJCC stage (8th edition) | |
IA1 | 499 (23.5%) |
IA2 | 515 (24.2%) |
IA3 | 265 (12.5%) |
IB | 347 (16.3%) |
IIA | 53 (2.5%) |
IIB | 184 (8.7%) |
IIIA | 213 (10.0%) |
IIIB | 50 (2.3%) |
Complications | |
No | 2,038 (95.9%) |
Yes | 88 (4.1%) |
Adjuvant therapy | |
No | 1,294 (60.9%) |
Yes | 445 (20.9%) |
Unknown | 387 (18.2%) |
IQR, interquartile range; VATS, video-assisted thoracoscopic surgery; AJCC, American Joint Committee on Cancer.
The framework of the deep learning-powered PN detection algorithm and an example of 3-dimensional (3D) reconstruction of the AI-detected nodules are shown in Figure 1A,1B. A total of 33,410 PNs were detected in the 2,126 patients. The features of these AI-detected nodules are provided in Table 2. The distributions of AI-detected TNN, solid nodule number, and subsolid nodule number per person were all positively skewed, and the medians of these 3 factors were 12 (IQR, 7 to 20), 6 (IQR, 3 to 10), and 3 (IQR, 1 to 6), respectively (Figure 2A-2C).
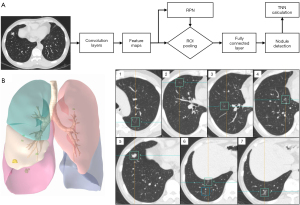
Table 2
Features | Value |
---|---|
Total nodule number, per person | |
Median [IQR] | 12 [7–20] |
Lobar distribution | |
Left lower lobe nodule | 6,630 (19.9%) |
Left upper lobe nodule | 7,934 (23.7%) |
Right lower lobe nodule | 6,631 (19.9%) |
Right middle lobe nodule | 2,680 (8.0%) |
Right upper lobe nodule | 9,535 (28.5%) |
Nodule location | |
Same-lobe nodule | 9,039 (27.0%) |
Same-side nodule | 9,114 (27.3%) |
Other-side nodule | 15,257 (45.7%) |
Nodule type | |
Solid nodule | 17,790 (53.2%) |
Mixed ground glass nodule | 1,616 (4.8%) |
Pure ground glass nodule | 10,276 (30.8%) |
Calcific nodule | 2,799 (8.4%) |
Perifissural nodule | 929 (2.8%) |
Solid nodule size | |
≤6 mm | 13,745 (77.2%) |
>6 mm & ≤8 mm | 1,487 (8.4%) |
>8 mm | 2,558 (14.4%) |
Mixed ground glass nodule size | |
≤6 mm | 273 (16.9%) |
>6 mm | 1,343 (83.1%) |
Pure ground glass nodule size | |
≤6 mm | 6,675 (65.0%) |
>6 mm | 3,601 (35.0%) |
AI, artificial intelligence; IQR, interquartile range.
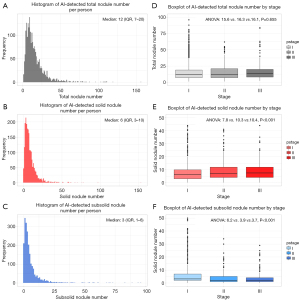
When considering discrepancies in nodule numbers among the different stages, we found that there was no statistically significant difference between the mean TNNs (one-way ANOVA P=0.655). However, the mean solid nodule numbers were significantly higher in participants with stage II and III, while the mean subsolid nodule numbers were higher in those with stage I (both P<0.001, Figure 2D-2F). Moreover, patients with late-stage cancer tended to have more solid nodules with greater size (Figure S1).
Survival analyses
We analyzed the survival of participants by stage according to the 8th edition of the AJCC prognostic group (Figure 3A,3B). The differences in both recurrence-free survival (RFS) and overall survival (OS) between any 2 stages were statistically significant (pairwise comparison P<0.001). Cox proportional hazards models were then built to determine the prognostic factors of the entire cohort (Table S1). The TNN was not an independent prognostic factor for either RFS (HR 1.006, 95% CI: 0.999 to 1.012, P=0.080) or OS (HR 1.002, 95% CI: 0.995 to 1.009, P=0.590) after adjusting for clinicopathological variables.
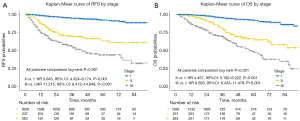
Subgroup analyses stratified by stage showed that the TNN was not significantly associated with survival for patients with stage I (RFS: HR 1.010, 95% CI: 0.998 to 1.022, P=0.102; OS: HR 1.003, 95% CI: 0.989 to 1.017, P=0.689) and stage II cancer (RFS: HR 1.000, 95% CI: 0.988 to 1.013, P=0.973; OS: HR 1.000, 95% CI: 0.989 to 1.012, P=0.965). However, in the stage III cohort, lower TNN was independently associated with improved survival in multivariate analyses (RFS: HR 1.012, 95% CI: 1.002 to 1.022, P=0.021; OS: HR 1.013, 95% CI: 1.002 to 1.025, P=0.021) (Tables 3,4).
Table 3
Variables | Univariate analysis | Multivariate analysis | |||||
---|---|---|---|---|---|---|---|
HR | 95% CI | P value | HR | 95% CI | P value | ||
Stage I (n=1,626, event =83) | |||||||
TNN (per 1 nodule increased) | 1.010 | 0.998–1.022 | 0.102 | 1.007 | 0.994–1.020 | 0.292 | |
Age (per 1 year increased) | 1.034 | 1.012–1.057 | 0.002* | 1.016 | 0.994–1.039 | 0.156 | |
Female gender | 0.650 | 0.422–0.999 | 0.050* | 1.088 | 0.613–1.933 | 0.773 | |
Positive smoking history | 2.038 | 1.319–3.149 | 0.001* | 1.155 | 0.632–2.111 | 0.639 | |
Comorbid conditions | 1.302 | 0.829–2.046 | 0.252 | ||||
Non-VATS approach | 3.280 | 1.483–7.256 | 0.003* | 1.814 | 0.805–4.087 | 0.151 | |
Non-sublobar resection | 1.861 | 1.087–3.186 | 0.024* | 1.027 | 0.585–1.803 | 0.926 | |
Non-adenocarcinoma | 3.459 | 2.155–5.553 | <0.001* | 2.217 | 1.271–3.866 | 0.005* | |
Postoperative complications | 0.901 | 0.284–2.862 | 0.860 | ||||
Adjuvant therapy | 2.309 | 1.325–4.025 | 0.003* | 1.409 | 0.780–2.545 | 0.256 | |
AJCC stage IA2 (8th edition) | 5.868 | 1.743–19.750 | 0.004* | 4.497 | 1.306–15.486 | 0.017* | |
AJCC stage IA3 (8th edition) | 11.566 | 3.448–38.790 | <0.001* | 7.719 | 2.202–27.065 | 0.001 | |
AJCC stage IB (8th edition) | 13.864 | 4.272–44.990 | <0.001* | 8.504 | 2.466–29.325 | <0.001* | |
Stage II (n=237, event =70) | |||||||
TNN (per 1 nodule increased) | 1.000 | 0.988–1.013 | 0.973 | 1.001 | 0.987–1.015 | 0.880 | |
Age (per 1 year increased) | 1.031 | 1.004–1.058 | 0.022* | 1.030 | 1.002–1.059 | 0.034* | |
Female sex | 1.504 | 0.924–2.449 | 0.100* | 1.588 | 0.966–2.611 | 0.068 | |
Positive smoking history | 0.866 | 0.541–1.387 | 0.549 | ||||
Comorbid conditions | 1.545 | 0.941–2.536 | 0.085* | 1.274 | 0.758–2.139 | 0.361 | |
Non-VATS approach | 1.393 | 0.820–2.367 | 0.220 | ||||
Non-sublobar resection | 0.871 | 0.273–2.776 | 0.816 | ||||
Non-adenocarcinoma | 0.785 | 0.487–1.267 | 0.322 | ||||
Postoperative complications | 0.420 | 0.058–3.023 | 0.389 | ||||
Adjuvant therapy | 1.038 | 0.637–1.693 | 0.881 | ||||
AJCC stage IIB (8th edition) | 0.837 | 0.484–1.445 | 0.523 | ||||
Stage III (n=263, event =119) | |||||||
TNN (per 1 nodule increased) | 1.015 | 1.005–1.024 | 0.003* | 1.012 | 1.002–1.022 | 0.021* | |
Age (per 1 year increased) | 1.022 | 1.004–1.041 | 0.019* | 1.019 | 1.000–1.039 | 0.051 | |
Female sex | 1.062 | 0.734–1.535 | 0.751 | ||||
Positive smoking history | 1.013 | 0.707–1.452 | 0.942 | ||||
Comorbid conditions | 0.862 | 0.600–1.238 | 0.421 | ||||
Non-VATS approach | 1.574 | 1.029–2.407 | 0.036* | 1.700 | 1.105–2.614 | 0.016* | |
Non-sublobar resection | 0.835 | 0.367–1.902 | 0.668 | ||||
Non-adenocarcinoma | 0.958 | 0.646–1.422 | 0.832 | ||||
Postoperative complications | 1.425 | 0.718–2.828 | 0.311 | ||||
Adjuvant therapy | 0.694 | 0.467–1.031 | 0.070* | 0.812 | 0.539–1.224 | 0.319 | |
AJCC stage IIIB (8th edition) | 1.421 | 0.912–2.215 | 0.121 |
*, statistical significance. RFS, recurrence-free survival; HR, hazard ratio; CI, confidence interval; TNN, total nodule number; VATS, video-assisted thoracoscopic surgery; AJCC, American Joint Committee on Cancer.
Table 4
Variables | Univariate analysis | Multivariate analysis | |||||
---|---|---|---|---|---|---|---|
HR | 95% CI | P value | HR | 95% CI | P value | ||
Stage I (n=1,626, event =80) | |||||||
TNN (per 1 nodule increased) | 1.003 | 0.989–1.017 | 0.689 | 0.995 | 0.978–1.012 | 0.572 | |
Age (per 1 year increased) | 1.080 | 1.054–1.106 | <0.001* | 1.062 | 1.035–1.090 | <0.001* | |
Female gender | 0.381 | 0.241–0.603 | <0.001* | 0.722 | 0.403–1.295 | 0.274 | |
Positive smoking history | 2.634 | 1.697–4.090 | <0.001* | 1.259 | 0.705–2.250 | 0.436 | |
Comorbid conditions | 1.883 | 1.152–3.077 | 0.012* | 1.182 | 0.713–1.962 | 0.517 | |
Non-VATS approach | 3.415 | 1.656–7.043 | <0.001* | 2.163 | 1.028–4.553 | 0.042* | |
Non-sublobar resection | 1.232 | 0.737–2.059 | 0.427 | ||||
Non-adenocarcinoma | 3.921 | 2.472–6.220 | <0.001* | 1.990 | 1.173–3.375 | 0.011* | |
Postoperative complications | 1.155 | 0.418–3.191 | 0.782 | ||||
Adjuvant therapy | 1.044 | 0.531–2.052 | 0.900 | ||||
AJCC stage IA2 (8th edition) | 9.332 | 2.199–39.600 | 0.002* | 5.510 | 1.285–23.633 | 0.022* | |
AJCC stage IA3 (8th edition) | 14.513 | 3.389–62.150 | <0.001* | 6.554 | 1.494–28.743 | 0.013* | |
AJCC stage IB (8th edition) | 14.918 | 3.575–62.240 | <0.001* | 6.839 | 1.606–29.127 | 0.009* | |
Stage II (n=237, event =61) | |||||||
TNN (per 1 nodule increased) | 1.000 | 0.989–1.012 | 0.965 | 1.001 | 0.988–1.013 | 0.934 | |
Age (per 1 year increased) | 1.044 | 1.015–1.074 | 0.003* | 1.044 | 1.015–1.074 | 0.003* | |
Female sex | 1.275 | 0.737–2.209 | 0.385 | ||||
Positive smoking history | 1.138 | 0.678–1.909 | 0.625 | ||||
Comorbid conditions | 1.394 | 0.831–2.339 | 0.208 | ||||
Non-VATS approach | 1.327 | 0.763–2.309 | 0.317 | ||||
Non-sublobar resection | 0.601 | 0.187–1.929 | 0.392 | ||||
Non-adenocarcinoma | 1.105 | 0.668–1.827 | 0.699 | ||||
Postoperative complications | 0.496 | 0.069–3.587 | 0.488 | ||||
Adjuvant therapy | 0.723 | 0.436–1.201 | 0.210 | ||||
AJCC stage IIB (8th edition) | 0.700 | 0.395–1.240 | 0.222 | ||||
Stage III (n=263, event =108) | |||||||
TNN (per 1 nodule increased) | 1.018 | 1.008–1.029 | <0.001* | 1.013 | 1.002–1.025 | 0.021* | |
Age (per 1 year increased) | 1.035 | 1.015–1.056 | <0.001* | 1.036 | 1.014–1.058 | <0.001* | |
Female sex | 0.645 | 0.428–0.972 | 0.036* | 1.054 | 0.568–1.955 | 0.868 | |
Positive smoking history | 1.716 | 1.168–2.521 | 0.006* | 1.443 | 0.792–2.631 | 0.231 | |
Comorbid conditions | 0.826 | 0.565–1.209 | 0.325 | ||||
Non-VATS approach | 2.340 | 1.556–3.517 | <0.001* | 2.480 | 1.541–3.990 | <0.001* | |
Non-sublobar resection | 0.724 | 0.293–1.789 | 0.483 | ||||
Non-adenocarcinoma | 1.614 | 1.093–2.384 | 0.016* | 0.933 | 0.567–1.535 | 0.784 | |
Postoperative complications | 1.380 | 0.690–2.761 | 0.362 | ||||
Adjuvant therapy | 0.458 | 0.309–0.679 | <0.001* | 0.560 | 0.394–0.913 | 0.017* | |
AJCC stage IIIB (8th edition) | 1.841 | 1.176–2.882 | 0.008* | 1.338 | 0.823–2.175 | 0.241 |
*, statistical significance. OS, overall survival; HR, hazard ratio; CI, confidence interval; TNN, total nodule number; VATS, video-assisted thoracoscopic surgery; AJCC, American Joint Committee on Cancer.
Exploratory analyses in the stage III cohort
To further evaluate the prognostic effect of the AI-detected TNN, we used maximally selected log-rank statistics to categorize patients into lower- and higher-TNN groups. The optimal cutoff value of 8 was selected (Figure S2). Participants with a lower TNN (≤8) had significantly improved OS (log-rank P<0.001, Figure 4A) compared with those with a higher TNN (>8). Lower TNN was also an independent favorable predictor for OS in multivariate analyses (HR 2.348, 95% CI: 1.351 to 4.082, P=0.002).
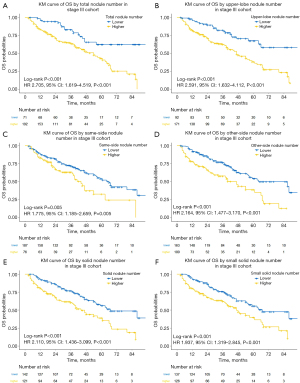
To assess which of the components were associated with survival, we classified AI-detected nodules into different categories. When analyzed as continuous variables, the numbers of upper-lobe nodule (HR 1.028, 95% CI: 1.008 to 1.049, P=0.006), same-side nodule (HR 1.032, 95% CI: 1.001 to 1.064, P=0.046), other-side nodule (HR 1.020, 95% CI: 1.001 to 1.039, P=0.040), solid nodule (HR 1.020, 95% CI: 1.004 to 1.036, P=0.012), and even solid nodule at small size (≤6 mm) (HR 1.027, 95% CI: 1.007 to 1.047, P=0.008) were independently associated with OS in multivariate analyses. However, none of the numbers of the middle/lower-lobe nodule (HR 1.016, 95% CI: 0.994 to 1.039, P=0.153), same-lobe nodule (HR 1.021, 95% CI: 0.986 to 1.056, P=0.246), m-GGN (HR 1.104, 95% CI: 0.885 to 1.376, P=0.381), p-GGN (HR 1.015, 95% CI: 0.976 to 1.056, P=0.462), calcific nodule (HR 1.021, 95% CI: 0.975 to 1.068, P=0.384), or perifissural nodule (HR 1.007, 95% CI: 0.792 to 1.279, P=0.957) were significantly associated with survival. The 5 independent prognostic nodule numbers were then set as binary variables according to their optimal cutoff values. Similarly, participants with lower nodule numbers had significantly improved OS compared with those with higher nodule numbers (Figure 4B-4F).
Finally, to evaluate which of the components contributed most to prognosis, a LASSO-based Cox regression model incorporating both clinicopathological features and all categories of AI-detected nodule numbers (as continuous variables) was built (Figure S3). The resulting 7 features with a nonzero coefficient were as follows: age (0.021), smoking history (0.106), surgical approach (0.669), adjuvant therapy status (−0.389), IIIA/IIIB classification (0.095), upper-lobe nodule number (0.014), and small (≤6 mm) solid nodule number (0.008). The number of upper-lobe nodules and the number of solid nodules of a small size were the individual features that contributed most to the model and correlated best with OS among all categories of AI-detected nodule numbers.
Survival tree analyses
A tree-based model incorporating AI-detected TNNs and the 8th edition of AJCC prognostic groups was constructed based on the best determination of OS for the entire cohort (Figure 5A). We found that the discrimination of survival curves for sub-stages was unsatisfactory with the current staging system in our study, especially in the sub-stages of IA2 to IB (IA2 vs. IA3: log-rank P=0.177; IA3 vs. IB: log-rank P=0.778) and IIA to IIB (log-rank P=0.236). Moreover, in the stage III cohort, rather than using the traditional IIIA and IIIB classifications, the model grouped OS according to AI-detected TNNs (lower vs. higher: log-rank P<0.001) since it showed a more effective determination of survival rates. The Kaplan-Meier curves of OS from the tree-based grouping scheme are shown in Figure 5B.
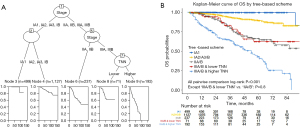
Treatment failure analyses
To evaluate the potential relationship between AI-detected TNNs and tumor recurrence patterns, we further divided the stage III cohort into 2 groups depending on their first disease progression site. Among all 263 participants in the stage III group, 60 had local recurrence, 40 had distant metastasis, and 19 had progressive cancer without a specified pattern. Participants with localized recurrence had a lower AI-detected TNN (median: 14; IQR, 7.75 to 18.25) compared with the distant metastasis group (median: 17; IQR, 10.75 to 23.25). However, the difference between these 2 groups was not statistically significant (Wilcoxon rank-sum P=0.077, Figure S4).
Discussion
The widespread application of AI algorithms in PN detection is reshaping our knowledge on this topic. The number of patients with tens or even hundreds of PNs is rapidly increasing. However, the interpretation of these lesions and their impact on surgical decision-making remain complicated and underrepresented. As the number of nodules grows, accurate diagnosis for every single nodule becomes onerous and statistically challenging. As an alternative, we hypothesized that TNN measured by a deep-learning algorithm may serve as a surrogate indicator of the probability of malignancy and metastasis in locally advanced NSCLC. This hypothesis was preliminarily supported by our results, which showed that the TNN is an independent prognostic factor in stage III lung cancer.
The accurate measurement of TNNs is highly challenging. First, the definition of PN varies among radiologists and surgeons due to their different purposes: some may only report guideline-mandated PNs in order not to provoke panic in patients, while others may report all detected PNs for more accurate surgical planning. Unfortunately, both standards are rather subjective and have poor replicability. Second, the accuracy and robustness of a single radiologist or surgeon are limited. The sensitivity of PN detection by a single radiologist is around 77%, though this can be increased to 90% with a concurrent radiologist’s help (32). However, such a method is time-consuming and remains subject to human error.
The emergence of a deep learning-based AI algorithm ensures the objectiveness and robustness of PN detection and, consequently, the measurement of TNNs. Mature algorithms have reached a diagnostic sensitivity of 85–100% (33-35). The best-performing deep learning algorithm is the LUNA16 challenge, which is based on the Lung Image Database Consortium and Image Database Resource Initiative (LIDC-IDRI) dataset and has exhibited an excellent sensitivity of over 95% with a less than 1.0 false positive per scan (36). The algorithm (InferRead CT Lung, InferVision) in this study was trained using over 350,000 chest CTs labeled by radiologists (20). In real-world applications, the performance of this model has reached an area under the curve (AUC) of 0.89 in PN detection and can significantly improve the performance of radiologists (20-22). Our result showed the median TNN to be 12 per patient, much higher than the median of 2 per patient reported in the malignant cohort of the NELSON study (29). Such a difference may, on the one hand, be due to differences in CT radiation dosage, or on the other hand, may reflect differences in diagnostic preference and consistency between AI and human radiologists.
From a clinical standpoint, our results suggested that the TNN may be a simplified representation of the tumor burden in stage III NSCLC. In contrast to the results of the NELSON study, which showed that a higher nodule count favored a benign diagnosis (29), our study focused on more advanced NSCLC patients instead of a high-risk screening population. Past evidence vaguely showed that with confirmed histology, extensive nodal or systemic metastases are substantial evidence that multiple PNs indicate IPM (37), suggesting that a high TNN may relate to a higher pretest probability of IPM. Our results further supported this speculation by revealing the improved survival rates of the lower TNN group compared to the higher TNN group, which existed when analyzing the TNN as either a continuous or a binary variable, and thus strengthened our hypothesis.
It is worth noting that the factor that most impacted survival was the number of solid nodules, not the number of GGNs. For the GGN components, the International Association for the Study of Lung Cancer (IASLC) guidelines suggest that the prognosis with multifocal GGNs be similar to that of a single minimally invasive adenocarcinoma (MIA) or adenocarcinoma in situ (AIS) (38), while others have indicated that there are metastatic GGNs on a molecular level (39). In our study, concurrent multiple GGNs in all 3 stages did not increase HR, indicating that concurrent multiple GGNs in invasive lung cancer possess the same biological behavior as in multifocal GGN cases. For solid components, most of the nodules were ≤6 mm and radiologically benign, with a round shape, no spiculation, and no lobulation. However, the growth of a few unresected nodules suggested their malignancy (Figure S5). These results showed that diagnosis using traditional radiological characteristics for multiple PNs in stage III NSCLC patients is not that reliable. Treatment failure pattern analysis showed that a higher TNN was related to distant metastasis (without statistical significance due to small sample size), indicating that TNN was not only an indicator of IPM, but also a visual representation of the systematic tumor burden.
From a surgeon’s perspective, the impact of PNs on surgical planning is substantial. Convincing a patient to accept unresected GGNs after surgery is difficult even with the guidelines’ support. A sublobar resection of a GGN may turn into a lobectomy due to multiple GGNs being detected by AI, while a lobectomy may also be changed to a sublobar resection due to bilateral nodules being clinically diagnosed as a separate primary lung cancer. However, no prior research has shown the validity of such an approach. Our study provided the first proof of concept that the TNN, determined by deep learning algorithms, should be considered a mandatory test before surgical planning. It would be reasonable for surgeons to be more aggressive in the resection of solid nodules instead of GGNs. Moreover, neoadjuvant therapy should be considered for stage III patients with a higher TNN for better PN evaluation since empirical diagnosis may not be reliable.
Some may argue that positron emission tomography-computed tomography (PET-CT) is a valid method for differentiating MPLC and IPM before surgery. However, the partial-volume effect of PET-CT prevents it from achieving optimum diagnostic performance for solid nodules of less than 8 mm, which represented 85.6% of the solid nodules in our study (26,40,41). Moreover, PET-CT is relatively expensive for most underdeveloped countries and not affordable for every patient.
As a retrospective study, our results need validation before clinical application. However, no public databases currently provide sufficient data. Therefore, prospective validation is necessary yet time-consuming. The AI algorithm requires optimization to further reduce the false positive rate, and perivascular nodule detection still needs improvement. Technological developments for the alignment of pre- and postoperative PNs on chest CT are urgently needed. The goals of future research are to analyze the growth speed and pathology findings of the unresected PNs and investigate the biological nature of the TNN, especially in the stage III NSCLC cohort. To our knowledge, this study was the first to identify that TNN measured by a deep learning algorithm is an independent prognostic factor in stage III lung cancer. Our results suggested a potentially critical clinical application of AI as a mandatory examination for surgical decision-making. The current cutoff point of the TNN is still preliminary but shows great potential and provides motivation for future validation.
Acknowledgments
We would like to thank Yuqing Huang, Xianjun Min, and Guotian Pei from the Beijing Haidian Hospital for sharing their thoughts on this work. We would like to thank Yutong Wang from the University of Michigan for his help in polishing our paper.
Funding: This work was supported by the National Natural Science Foundation of China (82002983, XC).
Footnote
Reporting Checklist: Available at https://atm.amegroups.com/article/view/10.21037/atm-21-3231/rc
Data Sharing Statement: Available at https://atm.amegroups.com/article/view/10.21037/atm-21-3231/dss
Peer Review File: Available at https://atm.amegroups.com/article/view/10.21037/atm-21-3231/prf
Conflicts of Interest: All authors have completed the ICMJE uniform disclosure form (available at https://atm.amegroups.com/article/view/10.21037/atm-21-3231/coif). XC reports funding from the National Natural Science Foundation of China (82002983). DW, JS, and WT were employed by the company Beijing Infervision Technology Co., Ltd. The other authors have no conflicts of interest to declare.
Ethical Statement: The authors are accountable for all aspects of the work in ensuring that questions related to the accuracy or integrity of any part of the work are appropriately investigated and resolved. The study was conducted in accordance with the Declaration of Helsinki (as revised in 2013). The study involving human participants was reviewed and approved by the Institutional Review Board of Peking University People’s Hospital (2020PHB385-01). Individual consent for this de-identified retrospective analysis was waived.
Open Access Statement: This is an Open Access article distributed in accordance with the Creative Commons Attribution-NonCommercial-NoDerivs 4.0 International License (CC BY-NC-ND 4.0), which permits the non-commercial replication and distribution of the article with the strict proviso that no changes or edits are made and the original work is properly cited (including links to both the formal publication through the relevant DOI and the license). See: https://creativecommons.org/licenses/by-nc-nd/4.0/.
References
- Bray F, Ferlay J, Soerjomataram I, et al. Global cancer statistics 2018: GLOBOCAN estimates of incidence and mortality worldwide for 36 cancers in 185 countries. CA Cancer J Clin 2018;68:394-424. [Crossref] [PubMed]
- National Lung Screening Trial Research Team. Reduced lung-cancer mortality with low-dose computed tomographic screening. N Engl J Med 2011;365:395-409. [Crossref] [PubMed]
- Detterbeck FC, Mazzone PJ, Naidich DP, et al. Screening for lung cancer: Diagnosis and management of lung cancer, 3rd ed: American College of Chest Physicians evidence-based clinical practice guidelines. Chest 2013;143:e78S-92S.
- Ruparel M, Quaife SL, Navani N, et al. Pulmonary nodules and CT screening: the past, present and future. Thorax 2016;71:367-75. [Crossref] [PubMed]
- Smith RA, Andrews KS, Brooks D, et al. Cancer screening in the United States, 2017: A review of current American Cancer Society guidelines and current issues in cancer screening. CA Cancer J Clin 2017;67:100-21. [Crossref] [PubMed]
- Wood DE, Kazerooni EA, Baum SL, et al. Lung Cancer Screening, Version 3.2018, NCCN Clinical Practice Guidelines in Oncology. J Natl Compr Canc Netw 2018;16:412-41. [Crossref] [PubMed]
- de Koning HJ, van der Aalst CM, de Jong PA, et al. Reduced Lung-Cancer Mortality with Volume CT Screening in a Randomized Trial. N Engl J Med 2020;382:503-13. [Crossref] [PubMed]
- Gould MK, Tang T, Liu IL, et al. Recent Trends in the Identification of Incidental Pulmonary Nodules. Am J Respir Crit Care Med 2015;192:1208-14. [Crossref] [PubMed]
- Erickson BJ, Korfiatis P, Akkus Z, et al. Machine Learning for Medical Imaging. Radiographics 2017;37:505-15. [Crossref] [PubMed]
- Armato SG 3rd, Altman MB, La Rivière PJ. Automated detection of lung nodules in CT scans: effect of image reconstruction algorithm. Med Phys 2003;30:461-72. [Crossref] [PubMed]
- Hwang EJ, Park CM. Clinical Implementation of Deep Learning in Thoracic Radiology: Potential Applications and Challenges. Korean J Radiol 2020;21:511-25. [Crossref] [PubMed]
- Ather S, Kadir T, Gleeson F. Artificial intelligence and radiomics in pulmonary nodule management: current status and future applications. Clin Radiol 2020;75:13-9. [Crossref] [PubMed]
- Murphy A, Skalski M, Gaillard F. The utilisation of convolutional neural networks in detecting pulmonary nodules: a review. Br J Radiol 2018;91:20180028. [Crossref] [PubMed]
- Hua KL, Hsu CH, Hidayati SC, et al. Computer-aided classification of lung nodules on computed tomography images via deep learning technique. Onco Targets Ther 2015;8:2015-22. [PubMed]
- Cheng JZ, Ni D, Chou YH, et al. Computer-Aided Diagnosis with Deep Learning Architecture: Applications to Breast Lesions in US Images and Pulmonary Nodules in CT Scans. Sci Rep 2016;6:24454. [Crossref] [PubMed]
- Li W, Cao P, Zhao D, et al. Pulmonary Nodule Classification with Deep Convolutional Neural Networks on Computed Tomography Images. Comput Math Methods Med 2016;2016:6215085. [Crossref] [PubMed]
- Liu S, Xie Y, Jirapatnakul A, et al. Pulmonary nodule classification in lung cancer screening with three-dimensional convolutional neural networks. J Med Imaging (Bellingham) 2017;4:041308. [Crossref] [PubMed]
- da Silva GLF, Valente TLA, Silva AC, et al. Convolutional neural network-based PSO for lung nodule false positive reduction on CT images. Comput Methods Programs Biomed 2018;162:109-18. [Crossref] [PubMed]
- Xie Y, Xia Y, Zhang J, et al. Knowledge-based Collaborative Deep Learning for Benign-Malignant Lung Nodule Classification on Chest CT. IEEE Trans Med Imaging 2019;38:991-1004. [Crossref] [PubMed]
- Wang Y, Yan F, Lu X, et al. IILS: Intelligent imaging layout system for automatic imaging report standardization and intra-interdisciplinary clinical workflow optimization. EBioMedicine 2019;44:162-81. [Crossref] [PubMed]
- Liu K, Li Q, Ma J, et al. Evaluating a Fully Automated Pulmonary Nodule Detection Approach and Its Impact on Radiologist Performance. Radiology: Artificial Intelligence 2019;1. [Crossref] [PubMed]
- Yang F, Fan J, Tian Z, et al. Population-based research of pulmonary subsolid nodule CT screening and artificial intelligence application. Chin J Thorac Cardiovasc Surg 2020;36:145-50.
- McWilliams A, Tammemagi MC, Mayo JR, et al. Probability of cancer in pulmonary nodules detected on first screening CT. N Engl J Med 2013;369:910-9. [Crossref] [PubMed]
- Horeweg N, van Rosmalen J, Heuvelmans MA, et al. Lung cancer probability in patients with CT-detected pulmonary nodules: a prespecified analysis of data from the NELSON trial of low-dose CT screening. Lancet Oncol 2014;15:1332-41. [Crossref] [PubMed]
- Walter JE, Heuvelmans MA, de Jong PA, et al. Occurrence and lung cancer probability of new solid nodules at incidence screening with low-dose CT: analysis of data from the randomised, controlled NELSON trial. Lancet Oncol 2016;17:907-16. [Crossref] [PubMed]
- Gould MK, Donington J, Lynch WR, et al. Evaluation of individuals with pulmonary nodules: when is it lung cancer? Diagnosis and management of lung cancer, 3rd ed: American College of Chest Physicians evidence-based clinical practice guidelines. Chest 2013;143:e93S-e120S.
- Callister ME, Baldwin DR, Akram AR, et al. British Thoracic Society guidelines for the investigation and management of pulmonary nodules. Thorax 2015;70:ii1-ii54. [Crossref] [PubMed]
- MacMahon H, Naidich DP, Goo JM, et al. Guidelines for Management of Incidental Pulmonary Nodules Detected on CT Images: From the Fleischner Society 2017. Radiology 2017;284:228-43. [Crossref] [PubMed]
- Heuvelmans MA, Walter JE, Peters RB, et al. Relationship between nodule count and lung cancer probability in baseline CT lung cancer screening: The NELSON study. Lung Cancer 2017;113:45-50. [Crossref] [PubMed]
- Walter JE, Heuvelmans MA, de Bock GH, et al. Relationship between the number of new nodules and lung cancer probability in incidence screening rounds of CT lung cancer screening: The NELSON study. Lung Cancer 2018;125:103-8. [Crossref] [PubMed]
- Girard N, Deshpande C, Lau C, et al. Comprehensive histologic assessment helps to differentiate multiple lung primary nonsmall cell carcinomas from metastases. Am J Surg Pathol 2009;33:1752-64. [Crossref] [PubMed]
- Nair A, Screaton NJ, Holemans JA, et al. The impact of trained radiographers as concurrent readers on performance and reading time of experienced radiologists in the UK Lung Cancer Screening (UKLS) trial. Eur Radiol 2018;28:226-34. [Crossref] [PubMed]
- Setio AA, Ciompi F, Litjens G, et al. Pulmonary Nodule Detection in CT Images: False Positive Reduction Using Multi-View Convolutional Networks. IEEE Trans Med Imaging 2016;35:1160-9. [Crossref] [PubMed]
- Dou Q, Chen H, Yu L, et al. Multilevel Contextual 3-D CNNs for False Positive Reduction in Pulmonary Nodule Detection. IEEE Trans Biomed Eng 2017;64:1558-67. [Crossref] [PubMed]
- Tajbakhsh N, Suzuki K. Comparing two classes of end-to-end machine-learning models in lung nodule detection and classification: MTANNs vs. CNNs. Pattern Recognition 2017;63:476-486. [Crossref]
- Setio AAA, Traverso A, de Bel T, et al. Validation, comparison, and combination of algorithms for automatic detection of pulmonary nodules in computed tomography images: The LUNA16 challenge. Med Image Anal 2017;42:1-13. [Crossref] [PubMed]
- Detterbeck FC, Nicholson AG, Franklin WA, et al. The IASLC Lung Cancer Staging Project: Summary of Proposals for Revisions of the Classification of Lung Cancers with Multiple Pulmonary Sites of Involvement in the Forthcoming Eighth Edition of the TNM Classification. J Thorac Oncol 2016;11:639-50.
- Detterbeck FC, Marom EM, Arenberg DA, et al. The IASLC Lung Cancer Staging Project: Background Data and Proposals for the Application of TNM Staging Rules to Lung Cancer Presenting as Multiple Nodules with Ground Glass or Lepidic Features or a Pneumonic Type of Involvement in the Forthcoming Eighth Edition of the TNM Classification. J Thorac Oncol 2016;11:666-80.
- Li R, Li X, Xue R, et al. Early metastasis detected in patients with multifocal pulmonary ground-glass opacities (GGOs). Thorax 2018;73:290-2. [Crossref] [PubMed]
- Soret M, Bacharach SL, Buvat I. Partial-volume effect in PET tumor imaging. J Nucl Med 2007;48:932-45. [Crossref] [PubMed]
- Groheux D, Quere G, Blanc E, et al. FDG PET-CT for solitary pulmonary nodule and lung cancer: Literature review. Diagn Interv Imaging 2016;97:1003-17. [Crossref] [PubMed]
(English Language Editors: L. Roberts and J. Jones)