The methylation modification of m6A regulators contributes to the prognosis of ovarian cancer
Introduction
Ovarian cancer (OV) is the leading cause of death in gynecological cancer since patients with early-stage OV are asymptomatic, and 75% will have reached an advanced stage (stage III or IV) by the time they are diagnosed (1). Although age-standardized rates are stable or falling in most high-income countries, they are rising in many low- and middle-income countries (2). According to the American Cancer Society, it was estimated that there were approximately 295,414 new cases and 184,799 deaths attributed to OV worldwide in 2018 (3).
Post-transcriptional modification of RNA is an important means of post-transcriptional processing of RNA, and it is an essential mechanism for post-transcriptional regulation of RNA (4).
N6-methyladenosine (m6A) modification is the most prevalent internal methylation in mRNA, and it is deposited by m6A methyltransferases, removed by m6A demethylases (5). m6A modification play important role in gene expression regulation of basic cellular processes and multiple physiological functions, and among of them, the most important role is modulating the eukaryotic transcriptome to influence mRNA splicing, export, localization, translation, and stability (6,7). Aberrant m6A modification can cause cancer, but it is also have the potential to yield new therapies (8).
New research has found that methylated nucleosides, especially m6A modification, which determine the fate of mRNAs by the alternatives of cell differentiation and embryonic development, exist in the mRNA of Novikoff hepatoma cells (9,10). Moreover, evidence is emerging that m6A modification and its regulatory proteins also play critical roles in various cancers including urological tumors, clear cell renal cell carcinoma, glioma, gastric cancer, etc. In urological tumors, many m6A modification-related proteins are dysregulated and promote cancer development (11). In clear cell renal cell carcinoma, alterations of m6A RNA methylation regulators were found to be related to the clinicopathological characteristics and survival of patients (12), and m6A RNA methylation regulators are also critical regulators of glioma progression (13). Further, m6A modification was shown to influence the malignant phenotypes of gastric cancer by regulating the Wnt/PI3K-Akt signaling pathway (14).
In ovarian cancer, m6A regulators are promising as potential molecular therapeutic targets for ovarian cancer (15). For example, YTHDF1 can promote tumorigenesis of ovarian cancer cells by regulating the translation of eIF3C via an m6A-dependent way, thus affects the global protein translation in ovarian cancer (16). Moreover, Han et al. showed that WTAP was significantly increased in ovarian tissues, and the highly expression is associated with the regulation of the cell cycle and the target of MYC (17).
Although m6A modification has been reported to play an important role in tumors, the relationship between m6A RNA methylation modulator signatures and OV prognosis remains to be verified. To confirm the precise m6A regulation pattern in OV, we systematically analyzed the expression of m6A RNA methylation regulators and constructed a prognostic model based on The Cancer Genome Atlas (TCGA) and the Genotype-Tissue Expression (GTEx) databases.
We present the following article in accordance with the TRIPOD reporting checklist (available at https://atm.amegroups.com/article/view/10.21037/atm-21-6462/rc).
Methods
Patient information
The clinical data of OV patients were downloaded from TCGA database (https://portal.gdc.cancer.gov/). There are 379 OV patients with RNA-Seq profiles and clinical data in TCGA database. The RNA-Seq profiles of 379 OV tissues and 88 healthy ovarian tissues were extracted from the University of California Santa Cruz (UCSC) database (http://xena.ucsc.edu/). Gene Expression Omnibus (GEO) datasets, including GSE66957 and GSE63885, were downloaded from the GEO database (https://www.ncbi.nlm.nih.gov/geo/). The data from TCGA and GEO were downloaded on September 27, 2021. The study setting, eligibility criteria for participants, and treatments received were all obtained from TCGA. The study was conducted in accordance with the Declaration of Helsinki (as revised in 2013).
m6A RNA methylation modulator selection
A total of 13 genes are recognized as vital m6A methylation modulators, including METTL3, METTL14, WTAP, KIAA1429, RBM15, ZC3H13, YTHDC1, YTHDC2, YTHDF1, YTHDF2, HNRNPC, ALKBH5, and FTO (18).
RNA isolation and quantitative real‑time PCR (qRT‑PCR)
For tissue RNA isolation, 1 mL AG RNAex Pro Reagent (Accurate Biotechnology Co.) was added to 50 mg of tissue and total RNA samples were extracted according to the manufacturer’s instructions. Purified RNA was quantified resort to NanoVue (GE Healthcare Life Sciences). cDNAs were synthesized from total RNAs by using RT reagent Kit (Takara Co., LTD, Japan) and ReverTra Ace qPCR RT Kit (Toyobo Co., LTD, Japan) (19).
qRT-PCR of GAPDH, KIAA1429, HNRNPC, YTHDC2, ZC3H13 and WTAP was performed with the SYBR qPCR Mix (Toyobo Co., LTD, Japan). 10 µL reaction system was adopted according to the manufacturer’s instructions and amplified for 40 cycles. The expression levels were normalized by GAPDH. Relative expression was calculated using the method of 2−∆∆Ct (20). Primer names and primer sequences are listed in the following tables (Table 1).
Table 1
Primer name | Primer sequence |
---|---|
GAPDH | Forward: CAACGTGTCAGTGGTGGACCTG |
GAPDH | Reverse: GTGTCGCTGTTGAAGTCAGAGGAG |
KIAA1429 | Forward: GGAATGGACACGTTTATTCGAG |
KIAA1429 | Reverse: GATAGAGCACAGGAGCATATGT |
ZC3H13 | Forward: GATCAGTTAAAGCGTGGAGAAC |
ZC3H13 | Reverse: CTCTCTGTCGTGTTCATATCGA |
WTAP | Forward: CTGACAAACGGACCAAGTAATG |
WTAP | Reverse: AAAGTCATCTTCGGTTGTGTTG |
YTHDC2 | Forward: GAGAATTGGGCTGTCGTTAAAG |
YTHDC2 | Reverse: TGAAGCAGGATGAAATCGTACT |
HNRNPC | Forward: CGTGTACCTCCTCCTCCTCCTATTG |
HNRNPC | Reverse: CCCCGCTGTCCACTCTTAGAATTG |
Bioinformatics analysis
A Gene Ontology (GO) functional enrichment analysis was performed to verify the function of the 13 m6A methylation modulators. Next, heatmap and violin plots were used to visualize the expression of the 13 regulators in 379 tumor tissues and 88 normal tissues. Spearman’s analysis was conducted to explore the correlations among these regulator genes, and a Kaplan-Meier (K-M) analysis was adopted to confirm the prognostic regulators. We performed a univariate Cox analysis to explore the prognostic role of m6A methylation regulators in OV patients. Then, a lasso regression analysis was used to construct the prognostic model. Five genes were identified as powerful prognostic factors. The risk score of each patient was calculated using the following formula: Risk score = 0.15987 × expression of KIAA1429 − 0.11282 × expression of HNRNPC + 0.069938 × expression of YTHDC2 + 0.109001 × expression of ZC3H13 + 0.123254 × expression of WTAP.
Statistical analysis
Unpaired t-tests were used to confirm the differential expression of the m6A RNA methylation modulators. The median risk score was set as the cut-off value to divide patients into high- or low-risk groups. The log-rank (Mantel-Cox) test was used for the survival analysis. Differences were considered statistically significant when the P value was <0.05.
Results
Confirmation of the function of m6A RNA methylation regulators
The level of m6A methylation is determined by the dynamic interplay between methyl transferases (‘writers’), binding proteins (‘readers’), and demethylases (‘erasers’). Writers of m6A include methyl transferase Like 3 (METTL3) (21), METTL14 (22,23), Wilms Tumor 1 Associated Protein (WTAP) (24), KIAA1429 (25), RNA Binding Motif Protein 15 (RBM15) (22), and ZC3H13 (26). Readers of m6A contain YTHDC1 (13,27), YTHDC2 (28), YTHDF1 (13), YTHDF2 (29), and HNRNPC (30). Erasers of m6A include ALKBH5 (31) and FTO (32).
We conducted a GO functional analysis to verify the function of the m6A RNA methylation regulators. Our results showed that the primary biological processes involved in these genes are RNA modification, methylation, metabolic process, and splicing. Moreover, these genes are involved in the formation of nuclear speck and methyltransferase complex (Figure 1A,1B, Table 2), and their molecular functions are catalytic activity and mRNA binding (Figure 1A, Table 2).
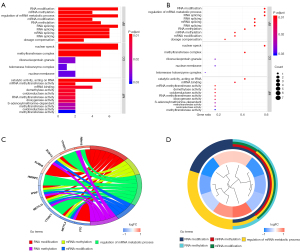
Table 2
Ontology | ID | Description | P value | Count | Gene ID |
---|---|---|---|---|---|
BP | GO:1903311 | Regulation of mRNA metabolic process | 6.82E-09 | 7 | HNRNPC, RBM15, METTL14, METTL3, WTAP, YTHDC1, YTHDF2 |
BP | GO:0009451 | RNA modification | 5.22E-10 | 7 | RBM15, METTL14, METTL3, ZC3H13, WTAP, FTO, ALKBH5 |
BP | GO:0008380 | RNA splicing | 1.72E-07 | 7 | HNRNPC, RBM15, METTL14, METTL3, ZC3H13, WTAP, YTHDC1 |
BP | GO:0001510 | RNA methylation | 4.46E-08 | 5 | RBM15, METTL14, METTL3, ZC3H13, WTAP |
BP | GO:0080009 | mRNA methylation | 6.82E-09 | 4 | METTL14, METTL3, ZC3H13, WTAP |
BP | GO:0016556 | mRNA modification | 3.72E-08 | 4 | METTL14, METTL3, ZC3H13, WTAP |
CC | GO:0016607 | Nuclear speck | 8.66E-09 | 7 | RBM15, METTL3, ZC3H13, WTAP, YTHDC1, FTO, ALKBH5 |
CC | GO:0034708 | Methyltransferase complex | 3.02E-08 | 5 | RBM15, METTL14, METTL3, ZC3H13, WTAP |
MF | GO:0140098 | Catalytic activity, acting on RNA | 8.92E-05 | 5 | METTL14, YTHDC2, METTL3, FTO, ALKBH5 |
MF | GO:0003729 | mRNA binding | 0.002685 | 4 | HNRNPC, RBM15, METTL14, METTL3 |
GO, Gene Ontology; BP, biological process; CC, cellular component; MF, molecular function.
The most significant biological processes in which m6A RNA methylation regulators are involved are RNA modification, regulation of mRNA metabolic process, and RNA splicing (Figure 1B). Among the genes related to m6A methylation, METTL3, METTL14, WTAP, and ZC3H13 participate in multiple RNA biological processes. However, YTHDF2, HNRNPC, YTHDC1, and FTO are only involved in RNA modification and the mRNA metabolic process (Figure 1C,1D).
Dysregulation of m6A RNA methylation regulators in OV tissues
There are 379 OV tissues with RNA-Seq in TCGA database and 88 normal ovarian tissues with RNA-Seq in the GTEx database. The expression of m6A RNA methylation regulators was detected in OV tissues and healthy ovarian tissues, and all were dysregulated in OV tissues compared with normal ovarian tissues (Figure 2A,2B, P<0.05). ZC3H13, ALKBH5, RBM15, YTHDF1, and YTHDF2 were upregulated in OV tissues, and the remainder were downregulated (Figure 2B, P<0.05).
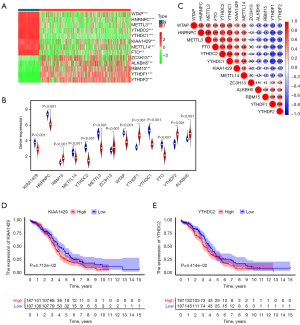
A co-expression analysis was performed to confirm if co-expression existed in the m6A RNA methylation regulators in OV tissues. The results showed the existence of positive and negative co-expressions. METTL3 (‘writers’) and HNRNPC proteins (‘readers’) have the most positive co-expression with an R value of 0.84, and YTHDF1 (‘readers’) and FTO (‘erasers’) have the strongest negative co-expression with an R value of −0.82 (Figure 2C).
Further, K-M analysis was applied to confirm the relationship between these genes’ expression and patients’ survival. Patients were divided into two groups according to the median value of the gene’s expression, and K-M curves were drawn to compare the OS time of the two patient groups. We found that a high expression of KIAA1429 and YTHDC2 predicted a poor prognosis in OV patients (P<0.05, Figure 2D,2E).
Construction of the prognostic model based on m6A RNA methylation regulators
A univariate Cox survival analysis was conducted to confirm the prognostic m6A RNA methylation regulators. We found five genes (KIAA1429, YTHDC2, ZC3H13, WTAP, and FTO) were highly expressed and were identified as high-risk factors for the poor prognosis of OV patients (Figure 3A).
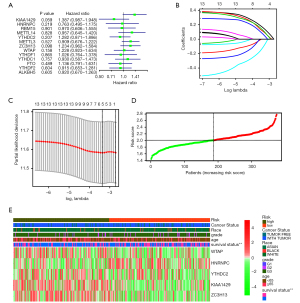
We also used a lasso regression algorithm to select genes and construct the prognostic model. According to the lasso regression analysis results, five genes (KIAA1429, YTHDC2, ZC3H13, WTAP, and FTO) were selected to construct the prognostic model for predicting the prognosis of OV patients (Figure 3B,3C, Table 3).
Table 3
Gene | HR | HR.95L | HR.95H | P value | Coef |
---|---|---|---|---|---|
KIAA1429 | 1.386533 | 0.986996 | 1.947802 | 0.059499 | 0.15987 |
HNRNPC | 0.762738 | 0.495315 | 1.174543 | 0.218851 | −0.11282 |
YTHDC2 | 1.281947 | 0.871357 | 1.886009 | 0.207342 | 0.069938 |
ZC3H13 | 1.234432 | 0.961737 | 1.584448 | 0.098201 | 0.109001 |
WTAP | 1.228301 | 0.923129 | 1.634359 | 0.158219 | 0.123254 |
m6A, N6-methyladenosine; HR, hazard rate.
A prognostic risk score formula was constructed according to the prognostic model. It was based on the linear combination of each gene’s expression multiplied by the coefficients from the results of the regression analysis as follows: Risk score = 0.15987 × expression of KIAA1429 − 0.11282 × expression of HNRNPC + 0.069938 × expression of YTHDC2 + 0.109001 × expression of ZC3H13 + 0.123254 × expression of WTAP. According to the risk score formula, each OV patient had a specific risk score value, and the patients were divided into two groups according to the median value of the risk score (Figure 3D).
Further, we compared the expression of the five m6A RNA methylation regulators in different clinicopathological parameters, including the two risk score groups. We found a significant difference between the high- and low-risk groups in OV patients’ survival status (P<0.05, Figure 3E).
The risk score was closely related to the prognosis of OV patients
A K-M analysis was conducted to validate the risk score derived from the m6A RNA methylation regulators. Patients with a high-risk score had a poorer prognosis than patients with a low-risk score (P<0.05, Figure 4A). Moreover, a three-dimensional heat map of survival time, risk score, and vital status showed more deaths among patients with a high-risk score (Figure 4B).
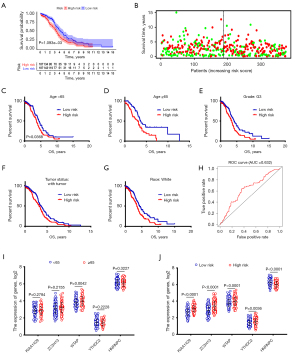
Next, we analyzed the effect of the risk score on the prognosis of patients with different clinicopathological parameters. As shown in the K-M plot curves, the risk score was a stable prognostic marker for OV patients of different ages (<65 or ≥65 years, Figure 4C,4D). Similarly, when we used the risk score to predict patients’ outcomes in other clinicopathological variables, we found that patients with a high-risk score who had grade 3 (G3) tumors, with tumor, and were White had a shorter survival time (Figure 4E-4G). ROC curve of the risk model derived from the five m6A RNA methylation regulators showed that the risk model has good accuracy and specificity. (Figure 4H). Further, we found that the m6A RNA methylation regulators were dysregulated in different stratified clinicopathological parameters. Results showed that WTAP was upregulated in the higher age group (P<0.05, Figure 4I). Additionally, all five m6A RNA methylation regulators showed significant differences between the low- and high-risk groups (P<0.05, Figure 4J). HNRNPC was downregulated in the high-risk group, whereas the other four genes were upregulated in the high-risk group (P<0.05, Figure 4J).
GEO datasets were used to verify the results from TCGA dataset and for further data mining. In dataset GSE66957, all five m6A RNA methylation regulators were downregulated in tumor tissues except ZC3H13, and the results are consistent with the results from TCGA dataset (Figures 2B,3E,5A). Moreover, the expression of m6A RNA methylation regulators were verified in clinical ovarian cancer tissues versus healthy ovarian tissues (Figure 5B).
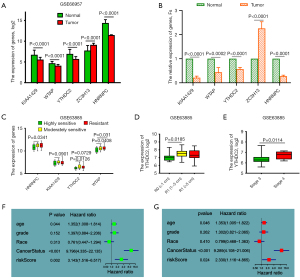
Interestingly, in the stratified analysis in dataset GSE63885, we found that four of the genes in our prognostic model were expressed differently in the stratified clinicopathological parameters. In the stratification analysis of chemotherapy resistance, HNRNPC, KIAA1429, YTHDC2, and WTAP were upregulated in the moderately sensitive and resistant groups (Figure 5C). In the stratification analysis of tumor size and stage, YTHDC2 was more highly expressed in larger tumor sizes and stage 4, implying that YTHDC2 may be a key m6A methylation gene in OV patients although this will require further verification (Figure 5D,5E).
Prognostic factors for OV patients
Univariate and multivariate Cox survival analyses were conducted to investigate the prognostic factors for OV patients. The univariate Cox survival analysis revealed that age, cancer status, and risk score were high-risk factors for the poor prognosis of OV patients (P<0.05, Figure 5F, Table 4). Moreover, they were all independent risk factors affecting the prognosis of OV patients (Figure 5G, Table 4).
Table 4
Clinical feature | Univariate analysis | Multivariate analysis | |||||||
---|---|---|---|---|---|---|---|---|---|
HR | HR.95L | HR.95H | P value | HR | HR.95L | HR.95H | P value | ||
Age | 1.352072 | 1.007566 | 1.81437 | 0.04441 | 1.353225 | 1.005279 | 1.821601 | 0.046077 | |
Grade | 1.396651 | 0.884412 | 2.205573 | 0.15184 | 1.302327 | 0.821149 | 2.065467 | 0.261623 | |
Race | 0.760836 | 0.447244 | 1.294305 | 0.313301 | 0.798667 | 0.468054 | 1.362808 | 0.409609 | |
Cancer status | 9.795922 | 4.335205 | 22.13507 | 4.10E-08 | 9.289214 | 4.107879 | 21.00585 | 8.61E-08 | |
Risk score | 3.143275 | 1.516093 | 6.516869 | 0.00208 | 2.3302 | 1.116067 | 4.865149 | 0.024301 |
HR, hazard rate.
Discussion
OV is one of the most lethal tumors for women, with a 5-year survival rate below 45% (2). It is difficult to diagnose OV in the early stages because the patient is generally asymptomatic. Therefore, more than half of patients are in an advanced stage (stage 3 or 4) by the time of diagnosis (1). Moreover, the treatments for OV are limited, and the death rates are higher than the incidence rates because of the resistance to radiotherapy and chemotherapy, especially in advanced stages (33). Thus, new, effective therapies urgently need to be identified.
m6A, as the most abundant post-transcriptional modification of RNA, is reportedly associated with different metabolic processes in various RNAs (5,10). Recently, several studies have revealed that m6A is involved in the development of many cancers (34,35). In this study, we analyzed the expression of the genes involved in modifying m6A in OV and found that all are dysregulated in OV tissues compared with normal ovarian tissues (Figures 2A,2B,5A). Moreover, positive and negative co-expression were found among the genes related to m6A methylation modification (Figure 2C).
KIAA1429, one of the writers of m6A, has been reported to facilitate the migration and invasion of hepatocellular carcinoma by inhibiting ID2 via upregulation of the m6A modification of ID2 mRNA (36). Additionally, YTHDC2, the reader of m6A, is dysregulated in many kinds of cancers and is implicated in the metastasis of colon tumors (37), the growth of tumor cells (38), and the susceptibility of pancreatic cancer (39). However, there is a lack of research on KIAA1429 and YTHDC2 in OV. In our research, we identified for the first time that a high expression of KIAA1429 and YTHDC2 is associated with the poor prognosis of OV patients (Figure 2D,2E).
Multiple dysregulated gene signatures from patients’ tumor tissues can provide better accuracy in predicting cancer prognosis than one specific gene and enable more effective and individualized treatment plans (40,41). In this study, we found that all of the genes related to m6A methylation were dysregulated in OV patients. Therefore, we were interested in knowing whether multigene signatures of m6A methylation could provide a more accurate prognosis prediction for OV patients. A Cox survival analysis and lasso regression analysis were used to construct the prognostic model for predicting OV patients’ prognosis (Figure 3B,3C). Finally, a risk score formula was constructed, where the risk score = 0.15987 × expression of KIAA1429 − 0.11282 × expression of HNRNPC + 0.069938 × expression of YTHDC2 + 0.109001 × expression of ZC3H13 + 0.123254 × expression of WTAP.
Moreover, K-M survival curves and a three-dimensional plot including patients’ risk score, survival time, and survival status were used to verify the accuracy and specificity of the prognostic model we constructed (Figure 4A-4G). There was a greater number of deaths in the high-risk score group, and multivariate Cox survival analysis revealed that the risk score acted as an independent risk factor in the prognosis of OV patients (Figure 5G, Table 4). The analysis from the GEO datasets was consistent with the results from TCGA dataset (Figures 2A,5A). Of the identified genes, YTHDC2 was dysregulated in OV tissues and demonstrated a higher expression in larger tumor sizes and stage 4. This implies that YTHDC2 may be a key m6A methylation gene in OV, although this finding will require further verification (Figure 5D,5E). The accuracy and specificity of the model constructed in this study will be improved by a larger sample of normal ovarian tissues, and the specific molecular mechanism of m6A needs to be further studied.
In the future, m6A modification could be used as diagnostic or prognostic targets for ovarian cancer. The upstream regulators of m6A modification, or their downstream targets, will provide new ideas and targets for the treatment of ovarian cancer. In general, the prospect of m6A modification research is helpful for the diagnosis, treatment and prognosis of ovarian cancer.
Conclusions
Collectively, this is the first study to combine TCGA and GTEx databases to explore the effect of m6A methylation genes on the prognosis of OV patients, which remedies the shortcoming of the small sample size of normal ovarian tissues in TCGA database. Moreover, we report for the first time that YTHDC2 (readers of m6A) and KIAA1429 (writers of m6A) are associated with the prognosis of OV patients. The prognostic model we have constructed could be a valuable clinical tool to indicate the prognosis for OV patients, and further research on the genes in our prognostic model will provide a future direction for the theoretical research and clinical treatment of OV.
Acknowledgments
Funding: None.
Footnote
Reporting Checklist: The authors have completed the TRIPOD reporting checklist. Available at https://atm.amegroups.com/article/view/10.21037/atm-21-6462/rc
Conflicts of Interest: All authors have completed the ICMJE uniform disclosure form (available at https://atm.amegroups.com/article/view/10.21037/atm-21-6462/coif). The authors have no conflicts of interest to declare.
Ethical Statement: The authors are accountable for all aspects of the work in ensuring that questions related to the accuracy or integrity of any part of the work are appropriately investigated and resolved. The study was conducted in accordance with the Declaration of Helsinki (as revised in 2013).
Open Access Statement: This is an Open Access article distributed in accordance with the Creative Commons Attribution-NonCommercial-NoDerivs 4.0 International License (CC BY-NC-ND 4.0), which permits the non-commercial replication and distribution of the article with the strict proviso that no changes or edits are made and the original work is properly cited (including links to both the formal publication through the relevant DOI and the license). See: https://creativecommons.org/licenses/by-nc-nd/4.0/.
References
- Jayde V, Boughton M. The diagnostic journey of ovarian cancer: a review of the literature and suggestions for practice. Contemp Nurse 2012;41:5-17. [Crossref] [PubMed]
- Webb PM, Jordan SJ. Epidemiology of epithelial ovarian cancer. Best Pract Res Clin Obstet Gynaecol 2017;41:3-14. [Crossref] [PubMed]
- Bray F, Ferlay J, Soerjomataram I, et al. Global cancer statistics 2018: GLOBOCAN estimates of incidence and mortality worldwide for 36 cancers in 185 countries. CA Cancer J Clin 2018;68:394-424. [Crossref] [PubMed]
- Nachtergaele S, He C. The emerging biology of RNA post-transcriptional modifications. RNA Biol 2017;14:156-63. [Crossref] [PubMed]
- Fu Y, Dominissini D, Rechavi G, et al. Gene expression regulation mediated through reversible m6A RNA methylation. Nat Rev Genet 2014;15:293-306. [Crossref] [PubMed]
- Xiao W, Adhikari S, Dahal U, et al. Nuclear m(6)A Reader YTHDC1 Regulates mRNA Splicing. Mol Cell 2016;61:507-19. [Crossref] [PubMed]
- Wang Y, Li Y, Toth JI, et al. N6-methyladenosine modification destabilizes developmental regulators in embryonic stem cells. Nat Cell Biol 2014;16:191-8. [Crossref] [PubMed]
- Zhang S. Mechanism of N6-methyladenosine modification and its emerging role in cancer. Pharmacol Ther 2018;189:173-83. [Crossref] [PubMed]
- Desrosiers R, Friderici K, Rottman F. Identification of methylated nucleosides in messenger RNA from Novikoff hepatoma cells. Proc Natl Acad Sci U S A 1974;71:3971-5. [Crossref] [PubMed]
- Zhao BS, Roundtree IA, He C. Post-transcriptional gene regulation by mRNA modifications. Nat Rev Mol Cell Biol 2017;18:31-42. [Crossref] [PubMed]
- Lobo J, Barros-Silva D, Henrique R, et al. The Emerging Role of Epitranscriptomics in Cancer: Focus on Urological Tumors. Genes (Basel) 2018;9:552. [Crossref] [PubMed]
- Zhou J, Wang J, Hong B, et al. Gene signatures and prognostic values of m6A regulators in clear cell renal cell carcinoma - a retrospective study using TCGA database. Aging (Albany NY) 2019;11:1633-47. [Crossref] [PubMed]
- Chai RC, Wu F, Wang QX, et al. m6A RNA methylation regulators contribute to malignant progression and have clinical prognostic impact in gliomas. Aging (Albany NY) 2019;11:1204-25. [Crossref] [PubMed]
- Zhang C, Zhang M, Ge S, et al. Reduced m6A modification predicts malignant phenotypes and augmented Wnt/PI3K-Akt signaling in gastric cancer. Cancer Med 2019;8:4766-81. [Crossref] [PubMed]
- Guo J, Zheng J, Zhang H, et al. RNA m6A methylation regulators in ovarian cancer. Cancer Cell Int 2021;21:609. [Crossref] [PubMed]
- Liu T, Wei Q, Jin J, et al. The m6A reader YTHDF1 promotes ovarian cancer progression via augmenting EIF3C translation. Nucleic Acids Res 2020;48:3816-31. [Crossref] [PubMed]
- Han X, Liu J, Cheng G, et al. Gene Signatures and Prognostic Values of m6A RNA Methylation Regulators in Ovarian Cancer. Cancer Control 2020;27:1073274820960460. [Crossref] [PubMed]
- Zaccara S, Ries RJ, Jaffrey SR. Reading, writing and erasing mRNA methylation. Nat Rev Mol Cell Biol 2019;20:608-24. [Crossref] [PubMed]
- Shan P, Yang F, Qi H, et al. Alteration of MDM2 by the Small Molecule YF438 Exerts Antitumor Effects in Triple-Negative Breast Cancer. Cancer Res 2021;81:4027-40. [Crossref] [PubMed]
- Zhu W, Niu J, He M, et al. SNORD89 promotes stemness phenotype of ovarian cancer cells by regulating Notch1-c-Myc pathway. J Transl Med 2019;17:259. [Crossref] [PubMed]
- Bokar JA, Shambaugh ME, Polayes D, et al. Purification and cDNA cloning of the AdoMet-binding subunit of the human mRNA (N6-adenosine)-methyltransferase. RNA 1997;3:1233-47. [PubMed]
- Schwartz S, Mumbach MR, Jovanovic M, et al. Perturbation of m6A writers reveals two distinct classes of mRNA methylation at internal and 5' sites. Cell Rep 2014;8:284-96. [Crossref] [PubMed]
- Schöller E, Weichmann F, Treiber T, et al. Interactions, localization, and phosphorylation of the m6A generating METTL3-METTL14-WTAP complex. RNA 2018;24:499-512. [Crossref] [PubMed]
- Ping XL, Sun BF, Wang L, et al. Mammalian WTAP is a regulatory subunit of the RNA N6-methyladenosine methyltransferase. Cell Res 2014;24:177-89. [Crossref] [PubMed]
- Agarwala SD, Blitzblau HG, Hochwagen A, et al. RNA methylation by the MIS complex regulates a cell fate decision in yeast. PLoS Genet 2012;8:e1002732. [Crossref] [PubMed]
- Patil DP, Chen CK, Pickering BF, et al. m(6)A RNA methylation promotes XIST-mediated transcriptional repression. Nature 2016;537:369-73. [Crossref] [PubMed]
- Nettersheim D, Berger D, Jostes S, et al. N6-Methyladenosine detected in RNA of testicular germ cell tumors is controlled by METTL3, ALKBH5, YTHDC1/F1/F2, and HNRNPC as writers, erasers, and readers. Andrology 2019;7:498-506. [PubMed]
- Wojtas M N., Pandey R R., Mendel M, et al. Regulation of m(6)A Transcripts by the 3'-->5' RNA Helicase YTHDC2 Is Essential for a Successful Meiotic Program in the Mammalian Germline. Mol Cell 2017;68:374-387.e12. [Crossref] [PubMed]
- Wu R, Yao Y, Jiang Q, et al. Epigallocatechin gallate targets FTO and inhibits adipogenesis in an mRNA m6A-YTHDF2-dependent manner. Int J Obes (Lond) 2018;42:1378-88. [Crossref] [PubMed]
- Fischl H, Neve J, Wang Z, et al. hnRNPC regulates cancer-specific alternative cleavage and polyadenylation profiles. Nucleic Acids Res 2019;47:7580-91. [Crossref] [PubMed]
- Tang C, Klukovich R, Peng H, et al. ALKBH5-dependent m6A demethylation controls splicing and stability of long 3'-UTR mRNAs in male germ cells. Proc Natl Acad Sci U S A 2018;115:E325-33. [Crossref] [PubMed]
- Ding C, Zou Q, Ding J, et al. Increased N6-methyladenosine causes infertility is associated with FTO expression. J Cell Physiol 2018;233:7055-66. [Crossref] [PubMed]
- Alkema NG, Wisman GB, van der Zee AG, et al. Studying platinum sensitivity and resistance in high-grade serous ovarian cancer: Different models for different questions. Drug Resist Updat 2016;24:55-69. [Crossref] [PubMed]
- Chen M, Wei L, Law CT, et al. RNA N6-methyladenosine methyltransferase-like 3 promotes liver cancer progression through YTHDF2-dependent posttranscriptional silencing of SOCS2. Hepatology 2018;67:2254-70. [Crossref] [PubMed]
- Liu X, Liu L, Dong Z, et al. Expression patterns and prognostic value of m6A-related genes in colorectal cancer. Am J Transl Res 2019;11:3972-91. [PubMed]
- Cheng X, Li M, Rao X, et al. KIAA1429 regulates the migration and invasion of hepatocellular carcinoma by altering m6A modification of ID2 mRNA. Onco Targets Ther 2019;12:3421-8. [Crossref] [PubMed]
- Tanabe A, Tanikawa K, Tsunetomi M, et al. RNA helicase YTHDC2 promotes cancer metastasis via the enhancement of the efficiency by which HIF-1α mRNA is translated. Cancer Lett 2016;376:34-42. [Crossref] [PubMed]
- Tanabe A, Konno J, Tanikawa K, et al. Transcriptional machinery of TNF-α-inducible YTH domain containing 2 (YTHDC2) gene. Gene 2014;535:24-32. [Crossref] [PubMed]
- Fanale D, Iovanna JL, Calvo EL, et al. Germline copy number variation in the YTHDC2 gene: does it have a role in finding a novel potential molecular target involved in pancreatic adenocarcinoma susceptibility? Expert Opin Ther Targets 2014;18:841-50. [Crossref] [PubMed]
- Zuo S, Dai G, Ren X. Identification of a 6-gene signature predicting prognosis for colorectal cancer. Cancer Cell Int 2019;19:6. [Crossref] [PubMed]
- Sun LL, Wu JY, Wu ZY, et al. A three-gene signature and clinical outcome in esophageal squamous cell carcinoma. Int J Cancer 2015;136:E569-77. [Crossref] [PubMed]
(English Language Editor: D. Fitzgerald)