Screening and verification of potential gene targets in esophageal carcinoma by bioinformatics analysis and immunohistochemistry
Introduction
Esophageal carcinoma (ESCA) is the 7th most common malignancy and the 6th leading cause of cancer related death globally. In 2018, about 500,000 patients were diagnosed with ESCA globally. China is one of the countries with a high incidence of ESCA. Notably, due to the atypical early symptoms of ESCA, a majority of patients are diagnosed with an advanced stage, often accompanied by distant or lymph node metastases, and the 5-year survival rate is less than 20% (1-4).
The two main histologic subtypes of ESCA are squamous cell carcinoma and adenocarcinoma. Despite biologic differences, treatment of ESCA did not vary based on histology, until recently. Multimodality treatment including systemic therapy, radiation, and surgery are required to achieve long-term survival. However, recurrence rates remain high and acute and chronic toxicity related to treatment remains significant. Chemotherapy and immune checkpoint inhibitors have modestly improved survival in metastatic disease (2,5). Hence, more efficacious and less toxic treatments remain a greatly unmet need for ESCA.
To date, the pathogenesis of ESCA has remained unclear. Therefore, the use of bioinformatics technology to compare ESCA patients with individuals without cancer, and to explore the early biomarkers and molecular pathways that drive the initiation and progression of ESCA, can contribute to further elucidating the pathogenesis of ESCA. This can potentially allow the design of personalized targeted drug therapy for ESCA patients and improve the overall survival rate and quality of life for these patients (6,7). In this study, we applied bioinformatics analysis technologies such as microarray data and high-throughput sequencing. Additionally, we used immunohistochemistry (IHC) to validate the differential expression of each gene in ESCA within patient samples at our hospital.
We present the following article in accordance with the REMARK reporting checklist (available at https://atm.amegroups.com/article/view/10.21037/atm-21-6589/rc).
Methods
Data download
The study was conducted in accordance with the Declaration of Helsinki (as revised in 2013). The dataset GSE20347 and Series Matrix File were downloaded from the Gene Expression Omnibus (GEO) of the National Center for Biotechnology Information (NCBI). The annotation platform was GPL571, with a total of 34 groups of transcriptome data, including a control group of normal adjacent esophageal tissue from patients (n=17) and a group of ESCA patients (n=17). The GSE17351 dataset and Series Matrix File were downloaded and the annotation platform was GPL570, with a total of 10 groups of transcriptome data, including a control group of adjacent normal esophageal mucosa from patients (n=5) and an ESCA patient group (n=5). The GSE45670 dataset and Series Matrix File were downloaded and the annotation platform was GPL570, with a total of 38 groups of transcriptome data, including a control group of normal esophageal epithelia from patients (n=10) and an ESCA patient group (n=28). A surrogate variable analysis (SVA) algorithm was used to correct the data between microarray and eliminate batch effect. Differently expressed genes (DEGs) between the 2 groups were calculated using the limma software package (https://bioconductor.org/packages/release/bioc/html/limma.html) with the criteria of |log2FC| >1 and P<0.05, to explore the differences in gene expression between ESCA patients and individuals without cancer. We downloaded ESCA data from The Cancer Genome Atlas (TCGA) for subsequent validation, with a total of 171 groups of transcriptome data, including a normal group (n=11) and diseased group (n=160). The survival of core genes was analyzed and the survival curve was drawn by R software.
Gene Ontology (GO) and Kyoto Encyclopedia of Genes and Genomes (KEGG) pathway analysis
To explore the biological functions and signaling pathways involved in pathogenesis, we performed annotation and visualization using the Metascape database (http://www.metascape.org) and conducted GO analysis and KEGG pathway analysis of specific genes. A minimum overlap ≥3 and P≤0.01 were considered statistically significant.
Least absolute shrinkage and selection operator (LASSO) regression and Boruta feature selection
We used the LASSO regression and the Boruta algorithm for feature selection of diagnostic markers. The LASSO algorithm uses the “glmnet” software package. Additionally, Boruta is a feature selection algorithm, which randomly shuffles the order of each real feature, evaluates the importance of each feature, and iteratively removes the features with low correlation to find the best variable. This study used the Boruta package for feature selection, and a total of 500 trees were constructed to further identify the diagnostic value of these biomarkers for diseases.
Analysis of immune cell infiltration
The cell-type identification by estimating relative subsets of RNA transcripts (CIBERSORT) method (https://cibersort.stanford.edu/) is widely used in the evaluation of immune cell types in the tumor microenvironment, which can perform deconvolution analysis of the expression matrix of immune cell subtypes based on the principle of support vector regression (SVR). It contains 547 biomarkers and distinguishes 22 human immune cell phenotypes, including T cell, B cell, plasma cell, and myeloid cell subsets. In this study, the CIBERSORT algorithm was used to analyze the data of ESCA patients from TCGA, infer the relative proportion of 22 kinds of immune infiltrating cells, and perform Spearman correlation analysis between gene expression and immune cell content.
Gene Set Variation Analysis (GSVA)
GSVA is a non-parametric and unsupervised method to evaluate the enrichment of transcriptome gene sets. It changes the gene level into the pathway level by comprehensively scoring the gene set of interest, thereby judging the biological function of samples. In this study, we downloaded gene sets from the Molecular signatures database (MSigDB; v7.0 version) and used the GSVA algorithm to score each gene set comprehensively, so as to evaluate the potential biological function changes of different samples.
Drug sensitivity analysis
Based on the largest pharmacogenomics database [Genomics of Drug Sensitivity in Cancer (GDSC), https://www.ca], we used the R software package “pRRophetic” to predict the chemosensitivity of each tumor sample. The IC50 of each specific chemotherapy drug was estimated by the regression method, and the GDSC training set was used for 10 cross-validations to test the regression and prediction accuracy. All parameters were set to default values, including the “combat” of removing batch effect and the average value of repeated gene expression.
Tumor mutational burden (TMB) and microsatellite instability (MSI) analysis
TMB is defined as the total amount of coding errors, base substitutions, insertions, or deletions of somatic genes detected per million bases. In this study, TMB was defined by calculating the mutation frequency and mutation/exon length of each sample and dividing the non-synonymous mutation sites by the total length of the protein coding region. The clinical features of MSI were obtained from TCGA database.
GeneMANIA analysis
The GeneMANIA (http://www.genemania.org) database is a flexible and user-friendly protein-protein interaction (PPI) network construction database, which is used to visualize the functional network between genes and analyze gene functions and interactions. The website can set up data sources of gene nodes and has many bioinformatics analysis methods, such as physical interaction, gene co-expression, gene co-location, gene enrichment analysis, and website prediction. In this study, the core gene network was generated by GeneMANIA to explore the possible mechanism of core genes in patient tumors.
Immunohistochemistry
Wax blocks were made from biopsy tissues of patients who came to our hospital for esophageal endoscopy. The thickness of paraffin section was 3 µm. The sections were baked for 2 h, dewaxing to water. Then, the sections were soaked in 3% H2O2 solution for 10 min, and blocked with fetal bovine serum for 30 min. Afterward, the sections were incubated with anti-CRIP2 (ab229110), anti-FOS (ab222699), and anti-HOXA10 (ab191470) antibodies at 4 °C overnight, then incubated with the corresponding secondary antibody at 37 °C for 1 h. Then, staining was visualized with 3,3-diaminobenzidine (DAB) for 4 min. Following distilled water washing, the sections were counterstained with hematoxylin dye for 5 min. The slices were dehydrated with ethanol, treated with xylene and sealed with neutral gum. Finally, photographs were taken with the microscope.
Statistical analysis
Statistical analysis was performed using R language (version 3.6; https://www.r-project.org/). All statistical tests were bilateral, and P<0.05 indicated statistical significance.
Results
The ESCA-related data sets GSE20347, GSE17351, and GSE45670 were downloaded from the GEO database, and a total of 82 groups of patient expression profile data were obtained, including a normal group of cancer free individuals (n=32) and diseased group of ESCA patients (n=50). The SVA algorithm was used to correct the microarray, and a principal component analysis (PCA) diagram showed the difference before and after correction. The results demonstrated that the batch effect between microarray was eliminated after SVA correction (Figure 1A,1B). We further used limma package to calculate the DEGs between the 2 groups, with the screening conditions of P<0.05 and |Log2FC| >1. Out of a total of 11,207 genes, a total of 430 DEGs were identified, including 210 upregulated genes and 220 downregulated genes (Figure 1C). We then conducted the pathway analysis of the DEGs, and the results showed that the DEGs were mainly enriched in pathways of the extracellular matrix, mitotic nuclear division, interleukin-17 (IL-17) signaling, glucosaminoglycan binding, and muscle system process (Figure 2A). The interaction among these genes is shown in Figure 2B.
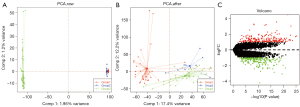
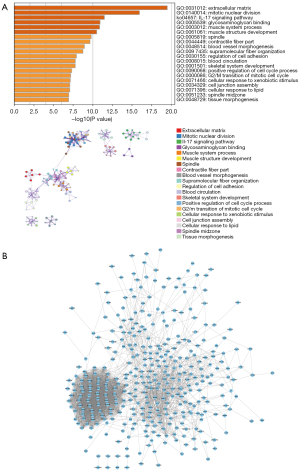
To further identify the core genes in the DEGs, we used LASSO regression and Boruta feature selection algorithm to screen the feature genes in ESCA. The results revealed 19 DEGs as the core genes of ESCA by LASSO regression (Figure 3A,3B, P<0.05). Boruta algorithm identified 93 DEGs as the core genes of ESCA (Figure 3C). After taking the intersection, a total of 15 core genes were screened, which included HOXB7, MYBL2, HOXA10, NEK2, TPX2, MARCKSL1, DDAH1, COL14A1, BLM, ITGA8, CENPM, PLA2G7, CRIP2, ISG15, and FOS. We then downloaded transcriptome data and corresponding clinical data of ESCA from TCGA public database, and further explored the core genes related to the prognosis of ESCA patients through Kaplan-Meier survival analysis. We found that CRIP2 (P=2.643e-02), FOS (P=4.837e-02), and HOXA10 (P=4.97e-02) significantly affected the prognosis of ESCA patients (Figure 4A-4C).
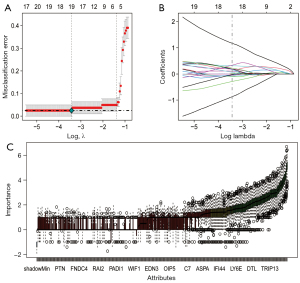
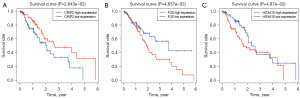
The tumor microenvironment is mainly composed of tumor-associated fibroblasts, immune cells, extracellular matrix, various growth factors, inflammatory factors, and tumor cells themselves. Factors inherent to the tumor microenvironment can significantly affect the diagnosis, survival outcome, and treatment sensitivity of tumors (3,5). We further explored the potential molecular mechanism of core genes affecting the progression of ESCA by analyzing the correlation between core genes and tumor immune infiltration in TCGA data sets. We found that 3 genes were strongly correlated with the content of immune cells (Figure 5A-5C). Next, we explored the correlation between the 3 genes and clinical symptoms of ESCA patients, and the results showed that CRIP2, FOS, and HOXA10 were all correlated with the stage of tumors (Figure 6A-6C). CRIP2 expression was not seen as often in stage IV patients.
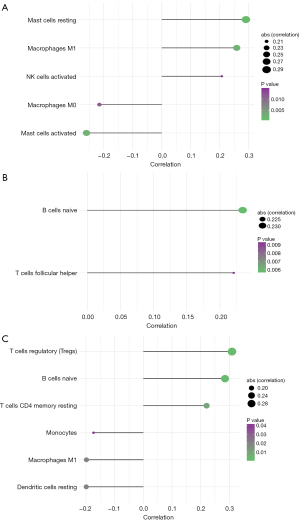
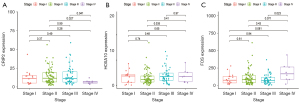
Based on the drug sensitivity data of GDSC database, we predicted the chemosensitivity of each tumor sample through R package “pRRophetic” to further explore the correlation between core genes and the sensitivity of common anti-cancer drugs. CRIP2 expression was correlated with the sensitivity of patients to dasatinib; FOS expression was correlated with the sensitivity of patients to erlotinib, and HOXA10 expression affected the sensitivity of patients to cisplatin, dasatinib, erlotinib, and gefitinib (Figure 7A-7C).
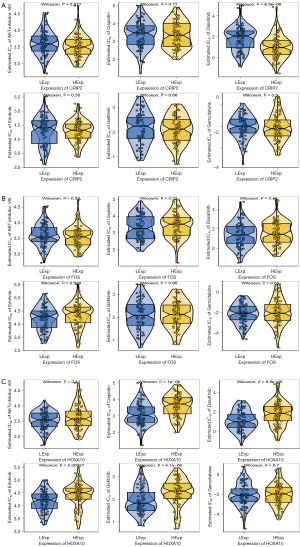
We used the cBioportal database (https://www.cbioportal.org/) to investigate the mutation of core genes in ESCA, and selected the data set as TCGA-PanCancer data set. The results showed that a total of 56 patients (31%) had the above core gene mutations, and the mutation rates of the 3 genes were the following: CRIP2 (8%), FOS (10%), and HOXA10 (17%) (Figure 8). Moreover, HOXA10 expression was correlated with tumor mutation load, suggesting that the mechanism of HOXA10 affecting tumor drug-resistance might be related to mutation load, and HOXA10 was also related to MSI (Figure 9A-9C). Next, we investigated the specific signaling pathways involved in the 3 core genes to explore the potential molecular mechanisms of the core genes in the progression of ESCA. The GSVA results showed that high expression of CRIP2, FOS, and HOXA10 participated in ANDROGEN_RESPONSE and BILE_ACID_METABOLISM, suggesting that core genes could influence the progression of ESCA by regulating tumor metabolism (Figure 10A-10C). The interaction mechanism network among the 3 core genes is shown in Figure 11.

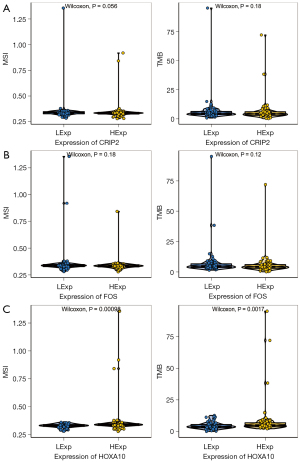
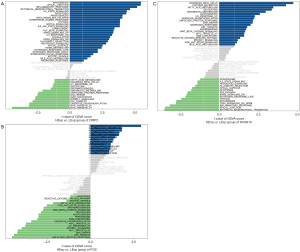
The average optical density values of the 3 core genes in the control group and the observation group were calculated and compared. The results are shown in Table 1 and Figure 12. There were significant differences in the expressions of CRIP2, FOS, and HOXA10 between the control group of cancer-free individuals and the ESCA patient group (P<0.05).
Table 1
Groups | CRIP2 | FOS | HOXA10 |
---|---|---|---|
Control group | 0.290±0.132 | 0.650±0.178 | 0.260±0.095 |
Observation group | 0.390±0.136 | 0.523±0.208 | 0.215±0.070 |
t | 3.036 | 2.782 | 2.793 |
P value | 0.002 | 0.003 | 0.003 |
SD, standard deviation.
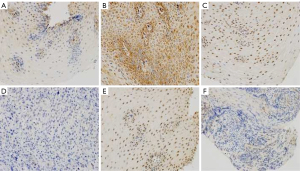
Discussion
Better biomarkers for the management of ESCA are needed. In this study, widely used bioinformatics analysis technologies such as microarray data and high-throughput sequencing were used to identify potential novel biomarkers for ESCA. We found that CRIP2, FOS, and HOXA10 were significantly associated with the prognosis of ESCA patients with high CRIP2 expression associated with better overall survival (OS), high FOS expression associated with worse OS, and high HOXA10 expression associated with worse OS.
Cysteine-rich intestinal protein 2 (CRIP2) (8) is a member of LIM domain protein family and located on chromosome 14q32.3. It can be detected in the heart (9,10), mammary gland (11), skin (12), skeletal muscle, esophagus (8), prostate, and small intestine, but its expression is usually deficient in many tumors. The CRIP2 gene is related to growth and development and can interact with the nuclear factor (NF)-κB/p65 pathway to suppress the DNA binding ability of angiogenic cytokines; it has been shown to inhibit the occurrence and angiogenesis of nasopharyngeal carcinoma and ESCA (13). Similarly, this study demonstrated that the expression of CRIP2 in ESCA tissues was significantly lower than that in normal tissues (P<0.05), indicating that the expression of CRIP2 was negatively correlated with the occurrence of ESCA. Additionally, the correlation between the content of ESCA immune infiltrating cells and the expression of CRIP2 was analyzed using TCGA data set. It was found that the ESCA tissues with low expression of CRIP2 had a high degree of immune cell infiltration, suggesting that as a target of immunotherapy, CRIP2 expression may be associated with therapeutic effects. Low expression CRIP2 was also correlated with more advanced stage at diagnosis and worse grade of tumors. The drug sensitivity test confirmed that CRIP2 expression was related to the sensitivity of patients to dasatinib. In this study, 8% of ESCA patients had CRIP2 gene mutation. Therefore, it is expected that targeting CRIP2 could prevent the further progression of ESCA if we can carry out gene detection for high-risk groups, screen patients with low CRIP2 expression, and implement targeted drug therapy.
The FOS gene is located on chromosome 14q21-31, consisting of FOS, FOSB, FOS1, and FOS2, which is a regulator of cell growth, proliferation, differentiation, transformation, and apoptosis (14). It is related to the plasticity of neurons and can affect the formation of cell memory by participating in the regulation of neuropeptides (15). In the pathological state, the FOS gene is related to the occurrence and development of a variety of malignant tumors (16-18) such as ovarian cancer (19), as well as brain diseases (20,21). In this study, the high expression of FOS in ESCA was confirmed by bioinformatics methods, and the above conclusion was further verified by IHC. The expression of FOS in ESCA tissues was higher than that in normal tissues (P<0.05). In TCGA data set analysis, we found that ESCA tissues with high expression of FOS had a high degree of immune cell infiltration, suggesting that FOS expression along with treatment with immunotherapy may obtain better therapeutic effects. Additionally, the high expression of FOS was associated with more advanced tumor stage and worse tumor grade, as well as lower sensitivity to erlotinib. In this study, 10% of ESCA patients had a FOS gene mutation.
The HOX gene, also known as homeobox gene, is a transcription regulatory gene that regulates the expression of target genes. It can participate in the process of body development, cell metabolism, apoptosis, and autophagy, thus playing an important role in the pathogenesis of tumors (22). Accumulating studies have shown that the HOX gene is closely related to the proliferation and metastasis of various malignant tumors (23-25), such as lung cancer (26), ovarian cancer (27), breast cancer (28), pancreatic cancer (29), as well as others. The HOXA10 gene is one of the important members of HOX gene family, which possesses the common properties of HOX gene family and promotes the proliferation, metastasis, and invasion of tumor cells. Its high expression is significantly correlated with poor tumor prognosis (22). The expression of HOXA10 in ESCA tissues was higher than that in normal tissues (P<0.05). In TCGA data set analysis, we found that ESCA tissues with high HOXA10 expression had a high degree of immune cell infiltration, suggesting that HOXA10 expression and immunotherapy may obtain better therapeutic effects. High expression of the HOXA10 gene was also correlated with more advanced stage and worse tumor grade. The drug sensitivity test confirmed that the expression of HOXA10 included the sensitivity of patients to cisplatin, dasatinib, erlotinib, and gefitinib. In this study, 17% of ESCA patients had HOXA10 gene mutation. A correlation was identified between HOXA10 and tumor mutation load, suggesting that the mechanism of HOXA10 affecting tumor drug-resistance might be related to mutation load, and HOXA10 was also related to MSI. This study confirmed that CRIP2, FOS, and HOXA10 may promote the progression of ESCA by participating in the regulation of tumor metabolism, were associated with patients’ clinical symptoms and sensitivity to chemotherapy drugs. However, the specific mechanism remained unclear, and further research is warranted.
This study has some limitations. Firstly, when we selected platforms and microarray data sets from GEO public database, some targets might have been missed due to inherent limitations of the database used. Secondly, the sample size of patients in this study was relatively small, leading to a wide confidence interval for the observed associations. Independent validation is another cohort is warranted. There are several limitations, yet this study still has significant clinical implications. Although numerous researches have explored the prognosis of ESCA, this study investigate that CRIP2, FOS, and HOXA10 may be prognostic targets for ESCA and was validated using immunohistochemistry for the first time. In addition, the analysis of TMB and immune infiltration on ESCA provides some implications for the personalized treatment of ESCA patients.
Conclusions
In this study, the DEGs between ESCA patients and samples from cancer free individuals were efficiently screened by bioinformatics analysis, and 3 core DEGs of ESCA patients were found. Through functional enrichment analysis and PPI network analysis, we detected some enrichment pathways and core genes that might be involved in the pathogenesis of ESCA, including CRIP2, FOS, and HOXA10. Furthermore, IHC confirmed that their expressions in ESCA were closely correlated with advanced stage and possibly survival. Further validation in a larger sample size and experiments to elucidate the underlying molecular function in the pathogenesis of ESCA are warranted. The 3 core genes may serve as potential biomarkers to guide prognosis and treatment of ESCA.
Acknowledgments
We would like to acknowledge the reviewers for their helpful comments on this paper. The authors also appreciate the academic support from the AME Esophageal Cancer Collaborative Group.
Funding: None.
Footnote
Reporting Checklist: The authors have completed the REMARK reporting checklist. Available at https://atm.amegroups.com/article/view/10.21037/atm-21-6589/rc
Conflicts of Interest: All authors have completed the ICMJE uniform disclosure form (available at https://atm.amegroups.com/article/view/10.21037/atm-21-6589/coif). FD reports research funding to the institution from Astrazeneca, BMS, Merck, Taiho and consulting fees from Astrazenca. RT reports receiving consulting fees for participating on cholangiocarcinoma advisory boards from Helsinn, QED Therapeutics, and The Lynx Group, outside this work; she serves on Huntsman Cancer Institute’s Data Safety Monitoring board that oversees institutional investigator initiated trials; she serves as a co-chair of the International Cholangiocarcinoma Research Network’s Radiation Oncology Working Group and on the NCCN Hodgkin Lymphoma panel; and her travel costs to the cholangiocarcinoma advisory boards and the Cholangiocarcinoma Summit have been covered by Helsinn, QED Therapeutics, and The Lynx Group all outside this work. The other authors have no conflicts of interest to declare.
Ethical Statement: The authors are accountable for all aspects of the work in ensuring that questions related to the accuracy or integrity of any part of the work are appropriately investigated and resolved. The study was conducted in accordance with the Declaration of Helsinki (as revised in 2013).
Open Access Statement: This is an Open Access article distributed in accordance with the Creative Commons Attribution-NonCommercial-NoDerivs 4.0 International License (CC BY-NC-ND 4.0), which permits the non-commercial replication and distribution of the article with the strict proviso that no changes or edits are made and the original work is properly cited (including links to both the formal publication through the relevant DOI and the license). See: https://creativecommons.org/licenses/by-nc-nd/4.0/.
References
- Lao-Sirieix P, Fitzgerald RC. Screening for oesophageal cancer. Nat Rev Clin Oncol 2012;9:278-87. [Crossref] [PubMed]
- Paul S, Altorki N. Outcomes in the management of esophageal cancer. J Surg Oncol 2014;110:599-610. [Crossref] [PubMed]
- Twarock S, Reichert C, Bach K, et al. Inhibition of the hyaluronan matrix enhances metabolic anticancer therapy by dichloroacetate in vitro and in vivo. Br J Pharmacol 2019;176:4474-90. [Crossref] [PubMed]
- Lagergren J, Smyth E, Cunningham D, et al. Oesophageal cancer. Lancet 2017;390:2383-96. [Crossref] [PubMed]
- Smyth EC, Lagergren J, Fitzgerald RC, et al. Oesophageal cancer. Nat Rev Dis Primers 2017;3:17048. [Crossref] [PubMed]
- Qin HD, Liao XY, Chen YB, et al. Genomic Characterization of Esophageal Squamous Cell Carcinoma Reveals Critical Genes Underlying Tumorigenesis and Poor Prognosis. Am J Hum Genet 2016;98:709-27. [Crossref] [PubMed]
- Cancer Genome Atlas Research Network. Integrated genomic characterization of oesophageal carcinoma. Nature 2017;541:169-75. [Crossref] [PubMed]
- Lo PH, Ko JM, Yu ZY, et al. The LIM domain protein, CRIP2, promotes apoptosis in esophageal squamous cell carcinoma. Cancer Lett 2012;316:39-45. [Crossref] [PubMed]
- Wei TC, Lin HY, Lu CC, et al. Expression of Crip2, a LIM-domain-only protein, in the mouse cardiovascular system under physiological and pathological conditions. Gene Expr Patterns 2011;11:384-94. [Crossref] [PubMed]
- Kim JD, Kim HJ, Koun S, et al. Zebrafish Crip2 plays a critical role in atrioventricular valve development by downregulating the expression of ECM genes in the endocardial cushion. Mol Cells 2014;37:406-11. [Crossref] [PubMed]
- Shi W, Bruce J, Lee M, et al. MiR-449a promotes breast cancer progression by targeting CRIP2. Oncotarget 2016;7:18906-18. [Crossref] [PubMed]
- Zhou L, Wang Y, Zhou M, et al. HOXA9 inhibits HIF-1α-mediated glycolysis through interacting with CRIP2 to repress cutaneous squamous cell carcinoma development. Nat Commun 2018;9:1480. [Crossref] [PubMed]
- Cheung AK, Ko JM, Lung HL, et al. Cysteine-rich intestinal protein 2 (CRIP2) acts as a repressor of NF-kappaB-mediated proangiogenic cytokine transcription to suppress tumorigenesis and angiogenesis. Proc Natl Acad Sci U S A 2011;108:8390-5. [Crossref] [PubMed]
- Gallo FT, Katche C, Morici JF, et al. Immediate Early Genes, Memory and Psychiatric Disorders: Focus on c-Fos, Egr1 and Arc. Front Behav Neurosci 2018;12:79. [Crossref] [PubMed]
- Rodríguez-Berdini L, Caputto BL. Lipid Metabolism in Neurons: A Brief Story of a Novel c-Fos-Dependent Mechanism for the Regulation of Their Synthesis. Front Cell Neurosci 2019;13:198. [Crossref] [PubMed]
- Bakiri L, Hamacher R, Graña O, et al. Liver carcinogenesis by FOS-dependent inflammation and cholesterol dysregulation. J Exp Med 2017;214:1387-409. [Crossref] [PubMed]
- Ding Y, Hao K, Li Z, et al. c-Fos separation from Lamin A/C by GDF15 promotes colon cancer invasion and metastasis in inflammatory microenvironment. J Cell Physiol 2020;235:4407-21. [Crossref] [PubMed]
- Liu R, Zhang T, Zhu G, et al. Regulation of mutant TERT by BRAF V600E/MAP kinase pathway through FOS/GABP in human cancer. Nat Commun 2018;9:579. [Crossref] [PubMed]
- Choi Y, Rosewell KL, Brännström M, et al. FOS, a Critical Downstream Mediator of PGR and EGF Signaling Necessary for Ovulatory Prostaglandins in the Human Ovary. J Clin Endocrinol Metab 2018;103:4241-52. [Crossref] [PubMed]
- Zhou J, Wang M, Deng D. c-Fos/microRNA-18a feedback loop modulates the tumor growth via HMBOX1 in human gliomas. Biomed Pharmacother 2018;107:1705-11. [Crossref] [PubMed]
- Huang J, Liu F, Wang B, et al. Central and Peripheral Changes in FOS Expression in Schizophrenia Based on Genome-Wide Gene Expression. Front Genet 2019;10:232. [Crossref] [PubMed]
- Mallo M. Reassessing the Role of Hox Genes during Vertebrate Development and Evolution. Trends Genet 2018;34:209-17. [Crossref] [PubMed]
- Shah N, Sukumar S. The Hox genes and their roles in oncogenesis. Nat Rev Cancer 2010;10:361-71. [Crossref] [PubMed]
- Bhatlekar S, Fields JZ, Boman BM. HOX genes and their role in the development of human cancers. J Mol Med (Berl) 2014;92:811-23. [Crossref] [PubMed]
- Paço A, Aparecida de Bessa Garcia S, Leitão Castro J, et al. Roles of the HOX Proteins in Cancer Invasion and Metastasis. Cancers (Basel) 2020;13:10. [Crossref] [PubMed]
- Li L, Wang Y, Song G, et al. HOX cluster-embedded antisense long non-coding RNAs in lung cancer. Cancer Lett 2019;450:14-21. [Crossref] [PubMed]
- Idaikkadar P, Morgan R, Michael A. HOX Genes in High Grade Ovarian Cancer. Cancers (Basel) 2019;11:1107. [Crossref] [PubMed]
- de Bessa Garcia SA, Araújo M, Pereira T, et al. HOX genes function in Breast Cancer development. Biochim Biophys Acta Rev Cancer 2020;1873:188358. [Crossref] [PubMed]
- Kuo TL, Cheng KH, Chen LT, et al. Deciphering The Potential Role of Hox Genes in Pancreatic Cancer. Cancers (Basel) 2019;11:734. [Crossref] [PubMed]
(English Language Editor: J. Jones)