Effects of Mycobacterium tuberculosis lineages and regions of difference (RD) virulence gene variation on tuberculosis recurrence
Introduction
Against the backdrop of the COVID-19 pandemic, tuberculosis (TB) remains one of the leading causes of mortality throughout the world (1). The WHO End TB Strategy aims to reduce TB deaths by 95% and new TB cases by 90% from 2015 to 2035 (2). TB is the top disease killer worldwide due to a single infectious agent, which kills one person every 21 s on average. There were ∼10 million cases and ∼1.5 million attributed deaths in 2018 alone, among there were 866,000 of TB in China, ranking the country second among 30 countries with a high TB burden (3). The incidence rate in Xinjiang has been reported to be close to 1/10,000, which is far higher than that of 1/100,000 in other parts of China (4). For Xinjiang, a province with one of the highest TB burdens, it is of great urgency to advance the prevention and control of TB.
TB is a malignant infectious disease, which major caused by airborne pathogen Mycobacterium tuberculosis (M.tb). After inhalation, M.tb reaches the alveolar space and is bathed in alveolar lining fluid (ALF). The high relapse rate is the main reason why TB is difficult to cure. Over the course of its evolution, M.tb has gradually accumulated specific variations that can be divided into 7 lineages (L1 to L9) (5). Different lineages of M.tb manifest in a variety of distinctive clinical profiles in the population. L2 is associated with relapse, fever, and treatment failure (6-9), as well as a higher resistance than that of L1 or L3 (10). L3 can evade the body’s immune response due to its slower growth rate and reduced ability to induce pro-inflammatory factors, thereby maintaining its infectiousness in the population (11,12). L4 has a high capacity for reproduction and transmission, leading to its wide global distribution (13).
M.tb members evolved from a common ancestor through successive DNA deletions and insertions. Fifteen regions of difference (RD1–15) have been identified by genome alignment, which may lead to differences in pathogenicity. The pathogenicity of M.tb is also affected by virulence genes. There are currently more than 300 known virulence genes of M.tb, some of which are located in RD1–15. Mutations in these genes may influence the phenotype and pathogenicity of M.tb. One study showed that when mice were infected with H37Rv mutants (deletion of RD2), the number of bacteria in the lungs and the degree of lung injury were relatively low and survival time was increased, i.e., the deletion of RD2 changed the pathogenic ability of M.tb (14). Based on M.tb genomics, Meumann et al. (15) found that BacA and Rv2326c mutations might be associated with TB relapse. In strains clinically isolated from patients with relapsed TB, Witney et al. (16) discovered a total of 12 nonsynonymous single-nucleotide polymorphisms (SNPs), of which 2 were located on eccB3 and McE1B and related to the pathogenicity of M.tb. M.tb RD region virulence genes affect the pathogenicity of strains (17,18). The definition of “virulence” is still widely discussed and its defining parameters and conditions are unsettled. Here it means the ability of a pathogen to cause disease, overcome the host resistance mechanism via invasion and adhesion to host cells, and adapt to hostile environments, including immune response modulation (19). This heterogeneity that exists among M.tb strains has an impact on immunogenicity and virulence (20). Therefore, the study of virulence genes in RD regions can help to identify differences in pathogenicity between the different lineages.
This study collected 155 clinical strains from TB patients with the same genetic background and the same treatment conditions in Kashgar Prefecture, Xinjiang. Whole-genome sequencing (WGS) was conducted, and the virulence gene SNP in the RD region was analyzed. The correlation between M.tb lineage and TB recurrence and the virulence gene SNP of the RD region were analyzed to clarify the molecular mechanism of relapse after M.tb infection. For the first time, 3 virulence genes found in the RD region of M.tb were found to be associated with SNP recurrence. The variation of these loci is an important risk factor leading to recurrence. It is found that the variation of these three loci is more common in L2 pedigree and also exists in other pedigrees. In other words, patients infected with L2 are more likely to relapse. The virulence gene variation in RD region is an important factor of strong pathogenicity and easy recurrence after L2 infection. The results provide basic research data for the prevention and control of TB and the development of new treatment methods. We present the following article in accordance with the MDAR reporting checklist (available at https://atm.amegroups.com/article/view/10.21037/atm-21-6863/rc).
Methods
Samples
Patients with TB treated in Kashgar Prefecture from January to December 2019 were diagnosed by etiology, drug sensitivity testing (rifampicin, isoniazid) and interferon gamma release assay (IGRA) clinical testing. A total of 155 patients with TB were enrolled, including 131 initial treatment patients and 24 relapsed patients, and clinical strains of M.tb were collected after sputum culture. The patients’ clinical data are shown in Table S1. This study has been carried out in accordance with the Declaration of Helsinki (as revised in 2013). The study was approved by the ethics committee of The First People’s Hospital of Kashgar (approval number: 2020, No. 55 and 2020, No. 58). Written informed consent was obtained from patients for the collection and use of the samples.
WGS
DNA extraction was performed using a magnetic bead extraction kit (MGIEasy, 1000006988, MGI Tech Co., Ltd., Shenzhen, China). A Qubit 3.0 Fluorometer (Q33216, Thermo Fisher Scientific, Waltham, MA, USA) was used for nucleic acid quantification. An MGIEasy FS DNA Library Prep Kit (MGIEasy, V1.0, 1000006988, MGI Tech Co., Ltd.) was used to construct the library. Agilent 2100 Bioanalyze (G2939AA, Agilent Technologies, Santa Clara, CA, USA) was used to detect the size of DNA fragments, and Qubit 3.0 was used to quantify the library. The WGS was performed on the MGISEQ-2000 platform (paired-ends, 100 bp; MGI Tech Co., Ltd.), with an average sequencing depth of 112×.
WGS data analysis and annotation
The quality of the raw reads was checked using FastQC version 0.11.8 toolkit (Babraham Bioinformatics, Cambridge, UK) followed by trimming of adapters, low-quality bases with a Phred quality score of less than 20, and fragments with a large fluctuation at the beginning of each sequence. Reads shorter than 30 bp were excluded from the downstream analysis, and the effective sequence length of reads was controlled at about 80 bp. The depth function of Samtools version 1.10 (21) was used to count the base coverage of the M.tb genome. Samples with a coverage of more than 95% were qualified for data sequencing. Reads were then mapped on to the reconstructed ancestral sequence of M.tb (22) using the Burrows-Wheeler Aligner Tool (BWA) version 0.7.17 (23). There is no reconstruction available for an ancestral M.tb chromosome; therefore, the chromosome coordinates and the annotation used was that of H37Rv. Duplicated reads were marked by the MarkDuplicates module of Picard version 1.119 (http://broadinstitute.github.io/picard/) and excluded, which of extra reads generated by polymerase chain reaction (PCR). Variant SNPs and insertion/deletions (in/dels) were called from each alignment file using Strelka2 version 2.9.10 (24). All SNPs were annotated using ANNOVAR version 2.1.1 (25), in accordance with the M.tb H37Rv reference annotation. The annotation consisted of the amino acid changes at the SNP site, the position information of the antigen peptide, and the gene name and Rv number.
Phylogenetic analysis
The complete genome data of 11 M.tb were obtained from the National Center for Biotechnology Information (NCBI). The dataset consisted of 13 complete genomes, available under accessions, including H37Rv (NC_000962.3, L4), HN-024 (AP018033.1, L1), 2242 (CP010335.1, L2), 2279 (CP010336.1, L2), 26105 (CP010340.1, L3), 22115 (CP010337.1, L4), UT307 (NZ_CP014617.1, L5), 25 (CP010334.1, L6), MAL010084 (KK338758.1, L6), 30 (CP010332.1, lineage animal), and BCG-26 (CP010331.1, lineage animal). Alignment of the other M.tb genomes to H37Rv was performed using the Nucmer functions of MUMmer version 3.1 (26). The output file generated by Nucmer after multiple sequence alignment was used to construct a phylogenetic tree in IQ-TREE version 1.6.12 (27) using the maximum-likelihood method. Ultrafast bootstrap (bb =1,000) approximation was used to assess branch supports. The output was visualized using Figtree version 1.4.4 (http://tree.bio.ed.ac.uk/software/figtree/). Finally, the specificity of the distribution of M.tb strains in the region was analyzed using a cluster analysis of clinical strains based on the locations of reference strains of different lineages.
Analysis of SNP in RD region virulence genes
The virulence factor database (http://www.mgc.ac.cn/VFs/) contains various medically important bacterial pathogen virulence factors, which include 86 experimentally confirmed and 171 putative genes related to the virulence of M.tb. Nine of these are located in RD regions.
Statistical analysis
The statistical analysis was performed using SPSS 19.0 software (SPSS Inc., Chicago, IL, USA). A chi-squared test was used to compare rates between groups. Binary logistic regression analysis was used for qualitative variables, one-way analysis of variance was used to compare means between samples, and Pearson correlation analysis was used to determine correlations between continuous variables. A P value of <0.05 was considered statistically significant. GraphPad Prism 5.0 (GraphPad Software, Inc., San Diego, CA, USA) was used to draw the forest map and histogram.
Results
Correlation analysis between M.tb lineage and relapse
The RD region gene variation of 155 M.tb clinical strains was analyzed. A total of 164,111 SNPs were detected, including 98,035 nonsynonymous SNPs (Figure 1A). A phylogenetic tree of 155 M.tb clinical strains was constructed based on RD region SNPs. The M.tb in Kashgar Prefecture was composed of 3 lineages: L2 (East-Asian) accounted for 45.81% (71/155), L3 (African-American) accounted for 32.90% (51/155), and L4 (Euro-American) accounted for 21.29% (33/155; Figure 1B). Among patients with relapse, L2 infection (70.83%, 17/24) was significantly higher than non-L2 infection (29.17%, 7/24; P<0.05; Figure 1C). L2 was significantly correlated with relapse only [odds ratio (OR) =3.505; 95% confidence interval (CI): 1.341–9.158; P=0.011; Figure 1D-1F].
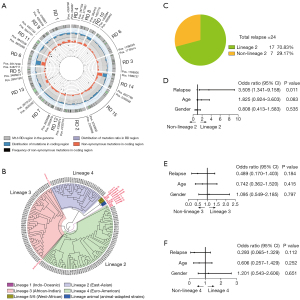
Variation of virulence genes in the RD region of M.tb clinical strains between different lineages
A total of 240 nonsynonymous SNPs were detected in 9 virulence genes (eccCb1, PE35, esxB, esxA, eccD1, espK, and P1cA-C) in the RD region of the M.tb clinical strains (Figure 2A,2B). A chi-squared test was used to compare the variation of virulence genes in the RD region between lineages, and the results showed that there were 12 nonsynonymous SNPs with significant differences between lineages, which were located in 5 genes (esxB, eccD1, espK, p1cC, and p1cA; Table 1). The mutation frequencies of g.2627618 in the p1cC gene, g.2630740 in the p1cA gene, and g.4357804 and g.4359653 in the espK gene in the L2 lineage were significantly higher than those in the L3 and L4 lineages (P<0.05). The mutation frequencies of g.2627382 in the p1cC gene, g.4355319 in the eccD1 gene, and g.4358392 in the espK gene in the L3 lineage were significantly higher than those in the L2 and L4 lineages (P<0.05). The mutation frequencies of g.2631226 in the p1cA gene, g.4352383 in the esxB gene, and g.4355141 in the eccD1 gene in the L3 lineage were significantly higher than those in the L2 and L4 lineages (P<0.05). g.2627618 and g.2630740 were the lineage-specific SNPs of the L2 lineage, g.2627382 was the lineage-specific SNP of the L3 lineage, and g.2631226 and g.4355141 were the lineage-specific SNPs of the L4 lineage (Figure 2C).
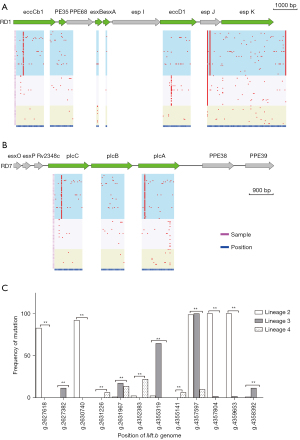
Table 1
Number | Gene | Rv | Position | Reference | Variant | Annotation | P value |
---|---|---|---|---|---|---|---|
1 | esxB | Rv3874 | 4352383 | G | C | ESX-1 secretion | <0.01 |
2 | eccD1 | Rv3877 | 4355319 | C | G | ESX-2 secretion | <0.01 |
3 | eccD1 | Rv3877 | 4355141 | A | C | ESX-3 secretion | <0.05 |
4 | espK | Rv3879c | 4357597 | C | G | ESX-4 secretion | <0.01 |
5 | espK | Rv3879c | 4357804 | T | G | ESX-5 secretion | <0.01 |
6 | espK | Rv3879c | 4359653 | C | T | ESX-6 secretion | <0.01 |
7 | espK | Rv3879c | 4358392 | G | C | ESX-7 secretion | <0.05 |
8 | pIcC | Rv2349c | 2627618 | C | A | Phospholipase C | <0.01 |
9 | pIcC | Rv2349c | 2627382 | C | T | Phospholipase C | <0.01 |
10 | pIcA | Rv2351c | 2630740 | T | C | Phospholipase C | <0.01 |
11 | pIcA | Rv2351c | 2631226 | C | A | Phospholipase C | <0.05 |
12 | pIcA | Rv2351c | 2631967 | G | A | Phospholipase C | <0.05 |
SNP, single-nucleotide polymorphism.
RD region virulence gene variation is associated with relapse
There were 421 SNPs in 9 virulence genes (eccCb1, PE35, esxB, esxA, eccD1, espK, and P1cA-C) in RD region of 155 M.tb clinical strains, among which 240 were nonsynonymous SNPs. The correlation between mutation and relapse was analyzed using binary logistic regression analysis of 240 nonsynonymous SNPs (table available at https://cdn.amegroups.cn/static/public/atm-21-6863-1.xlsx). The results showed that relapse of the g.4357804 (T→G), g.4359653 (C→T), and g.2627618 (C→A) mutations was significantly higher than that of the wild type (P<0.05; Figure 3A). The g.4357804 (OR =4.278; 95% CI: 1.594–11.481; P=0.004), g.4359653 (OR =3.356; 95% CI: 1.303–8.644; P=0.012), and g.2627618 (OR =2.676; 95% CI: 1.101–6.502; P=0.030) mutations were risk factors for relapse (Figure 3B). The mutation frequencies of these 3 SNPs were significantly higher in L2 than those in non-L2 (P<0.01; Figure 3C). The above results suggested that L2 patients were more likely to relapse due to the mutation of virulence genes in the RD region.
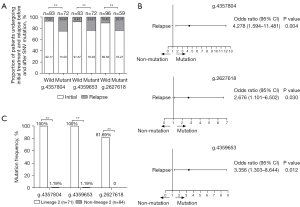
Effect of RD region virulence gene SNP mutation on protein structure and function
The three-dimensional structure of the protein was predicted by Phyre2 software (28), and the effect of amino acid mutation on protein function was analyzed. The results showed that the SNPs significantly correlated with the relapse of TB patients were located in the espK gene [g.4357804 (T→G), g.4359653 (C→T); Figure 4A] and the p1cC gene [g.2627618 (C→A); Figure 4B], and that the 3 SNP mutations all led to changes in amino acids (Table 2). Remote homology detection was used for 3D modeling in Phyre2. The 3D model map with the highest coverage was selected, which showed the positions of SNPs in the protein structure. As the espK encoded protein model map did not cover g.4357804, its position in the protein structure could not be displayed. These results suggested that SNP mutations may affect protein function.
Table 2
SNV | Gene | Function | Corresponding amino acid changes before and after mutation | ||||
---|---|---|---|---|---|---|---|
Amino acid | Acidity and basicity | Hydrophilic and hydrophobic | Electrical | Polarity/non-polarity | |||
g.4357804 | espK (Rv3879c) | ESX-1 secretion | Before: glycine | Neutral | Hydrophilic | 0 | Non-polarity |
After: cysteine | Neutral | Hydrophobic | 0 | Polarity | |||
g.4359653 | espK (Rv3879c) | ESX-1 secretion | Before: glutamic acid | Acidity | Hydrophilic | – | Polarity |
After: alanine | Neutral | Hydrophobic | 0 | Non-polarity | |||
g.2627618 | pIcC (Rv2349c) | Phospholipase C synthesis | Before: aspartic acid | Acidity | Hydrophilic | – | Polarity |
After: asparagine | Neutral | Hydrophilic | 0 | Polarity |
SNP, single-nucleotide polymorphism; SNV, single-nucleotide variant.
Discussion
The prevalence of TB may be worsened by the current COVID-19 pandemic, exacerbating the global health crisis and undermining TB prevention and control strategies. WGS of within-host M.tb diversity may provide new insights into the complex underlying molecular mechanisms of TB incidence and drug resistance. The clinical manifestations of TB patients are variable. In addition to individual differences between patients, this variability is related to the M.tb lineage and the genomic variation of the infection (29,30). In a study of M.tb lineage distribution in Xinjiang, Chen et al. (31) confirmed that the recurrence rate of patients infected with L2 (73.1%) was significantly higher than that of patients with other lineage infections (32.5%; P<0.01) (6,7). This is consistent with the results of the current study. Numerous genotype-specific mutations in the genes of functional categories such as intermediate metabolism and respiration, cell wall and cell processes, lipid metabolism, regulatory proteins, information pathways and virulence, detoxification, and adaptation could be responsible for the differential presentation of infection seen in different lineages (32-34). The virulence gene of M.tb affects the biological function and immunogenicity of M.tb (35).
In this study, WGS of 155 clinical strains of M.tb in Kashgar Prefecture was conducted. The results revealed several new genetic variations that had not been reported before, and the frequency of these SNPs in different lineages was significant. Among them, the 3 newly discovered SNPs in the virulence genes of the RD region had a high mutation frequency in L2 and were significantly related to patient recurrence. These SNPs belong to espK (2 SNPs) related to the secretion system and are p1cC gene (1 SNP) highly related to encoding phospholipase C. espK is a secretion-related protein that affects the migration of M.tb between cells and is necessary for inhibiting the inflammation and immune response of macrophages (36,37). p1cC plays an important role in the process of persistent M.tb infection (38-40). It is speculated that these 3 SNPs may have changed the immunogenicity of the L2 M.tb strain, making it difficult for the body to clear. This may be one of the reasons for the wide distribution of the L2 lineage in this region and its easy recurrence. In addition, the Rv3879c mutation increases the hydrophobicity of L2, and its higher hydrophobicity might have contributed to increased transmission through aerosolization. The p1cA/B/C gene in the L2 Beijing strain is more conserved (39,41). In our study, the 4 SNP mutation frequencies on the p1cA and p1cC genes were significantly different between L2 and non-L2, which may result from the adaptive evolution of this lineage in the local population. In brief, we find that patients infected with L2 are more prone to relapse, and RD region virulence gene variation is an important factor for the strong pathogenicity and easy relapse after infection associated with L2.
To date, within-host microevolving SNPs have been used to distinguish between reinfection and relapse or between acquired and transmitted drug resistance, as well as numerically to calculate the SNP distance and mutation rates in transmission studies, but their type, location, function, and frequency pattern have not been systematically studied. To improve our understanding of the role of mutations in the successful adaptation of M.tb to the changing environment in the host, future research should investigate the drivers of within-host genomic diversity and the impact of different mutations on phenotype, disease progression, diagnosis, and transmission. Learning from the experience of COVID-19’s prevention and control, we believe that the prevention of TB is mainly based on community prevention and control, such as full publicity, universal vaccination, timely isolation of patients, accurate and timely treatment to prevent drug resistance and relapse, otherwise, M.tb will continue to evolve along with the increase of transmission, just like COVID-19.
Acknowledgments
Funding: This work was supported by the Natural Science Foundation of Xinjiang Uygur Autonomous Region (No. 2020D01C013) and the Special Project for the Construction of the Autonomous Region’s Innovative Environment (Talents, Bases) [Tianshan Cedar Project (No. 2019XS22)].
Footnote
Reporting Checklist: The authors have completed the MDAR reporting checklist. Available at https://atm.amegroups.com/article/view/10.21037/atm-21-6863/rc
Data Sharing Statement: Available at https://atm.amegroups.com/article/view/10.21037/atm-21-6863/dss
Conflicts of Interest: All authors have completed the ICMJE uniform disclosure form (available at https://atm.amegroups.com/article/view/10.21037/atm-21-6863/coif). CH reports that this work was supported by the Natural Science Foundation of Xinjiang Uygur Autonomous Region (No. 2020D01C013) and the Special Project for the Construction of the Autonomous Region’s Innovative Environment (Talents, Bases) [Tianshan Cedar Project (No. 2019XS22)]; HP reports this work was supported by the Natural Science Foundation of Xinjiang Uygur Autonomous Region (No. 2020D01C013); XC, AK, JR, WL, JX, and AX report that this work was supported by the Special Project for the Construction of the Autonomous Region’s Innovative Environment (Talents, Bases) [Tianshan Cedar Project (No. 2019XS22)]. The other authors have no conflicts of interest to declare.
Ethical Statement: The authors are accountable for all aspects of the work in ensuring that questions related to the accuracy or integrity of any part of the work are appropriately investigated and resolved. The study was conducted in accordance with the Declaration of Helsinki (as revised in 2013). The study was approved by the ethics committee of The First People’s Hospital of Kashgar (approval number: 2020, No. 55 and 2020, No. 58). Written informed consent was obtained from patients for the collection and use of the samples.
Open Access Statement: This is an Open Access article distributed in accordance with the Creative Commons Attribution-NonCommercial-NoDerivs 4.0 International License (CC BY-NC-ND 4.0), which permits the non-commercial replication and distribution of the article with the strict proviso that no changes or edits are made and the original work is properly cited (including links to both the formal publication through the relevant DOI and the license). See: https://creativecommons.org/licenses/by-nc-nd/4.0/.
References
- Sands P. HIV, tuberculosis, and malaria: how can the impact of COVID-19 be minimised? Lancet Glob Health 2020;8:e1102-3. [Crossref] [PubMed]
- Global Tuberculosis Report 2013. World Health Organization, 2013.
- Global Tuberculosis Report 2019. World Health Organization, 2019.
- Wang L, Cheng S, Chen M, et al. The fifth China national epidemiology of tuberculosis survey 2010. Chinese Journal of Antituberculosis 2012;34:485-508.
- Ngabonziza JCS, Loiseau C, Marceau M, et al. A sister lineage of the Mycobacterium tuberculosis complex discovered in the African Great Lakes region. Nat Commun 2020;11:2917. [Crossref] [PubMed]
- Sun YJ, Lee AS, Wong SY, et al. Association of Mycobacterium tuberculosis Beijing genotype with tuberculosis relapse in Singapore. Epidemiol Infect 2006;134:329-32. [Crossref] [PubMed]
- Huyen MN, Buu TN, Tiemersma E, et al. Tuberculosis relapse in Vietnam is significantly associated with Mycobacterium tuberculosis Beijing genotype infections. J Infect Dis 2013;207:1516-24. [Crossref] [PubMed]
- Parwati I, Alisjahbana B, Apriani L, et al. Mycobacterium tuberculosis Beijing genotype is an independent risk factor for tuberculosis treatment failure in Indonesia. J Infect Dis 2010;201:553-7. [Crossref] [PubMed]
- van Crevel R, Nelwan RH, de Lenne W, et al. Mycobacterium tuberculosis Beijing genotype strains associated with febrile response to treatment. Emerg Infect Dis 2001;7:880-3. [Crossref] [PubMed]
- Singh J, Sankar MM, Kumar P, et al. Genetic diversity and drug susceptibility profile of Mycobacterium tuberculosis isolated from different regions of India. J Infect 2015;71:207-19. [Crossref] [PubMed]
- Newton SM, Smith RJ, Wilkinson KA, et al. A deletion defining a common Asian lineage of Mycobacterium tuberculosis associates with immune subversion. Proc Natl Acad Sci U S A 2006;103:15594-8. [Crossref] [PubMed]
- Tanveer M, Hasan Z, Kanji A, et al. Reduced TNF-alpha and IFN-gamma responses to Central Asian strain 1 and Beijing isolates of Mycobacterium tuberculosis in comparison with H37Rv strain. Trans R Soc Trop Med Hyg 2009;103:581-7. [Crossref] [PubMed]
- European Concerted Action on New Generation Genetic Markers and Techniques for the Epidemiology and Control of Tuberculosis. Beijing/W genotype Mycobacterium tuberculosis and drug resistance. Emerg Infect Dis 2006;12:736-43. [Crossref] [PubMed]
- Kozak RA, Alexander DC, Liao R, et al. Region of difference 2 contributes to virulence of Mycobacterium tuberculosis. Infect Immun 2011;79:59-66. [Crossref] [PubMed]
- Meumann EM, Globan M, Fyfe JAM, et al. Genome sequence comparisons of serial multi-drug-resistant Mycobacterium tuberculosis isolates over 21 years of infection in a single patient. Microb Genom 2015;1:e000037. [Crossref] [PubMed]
- Witney AA, Bateson AL, Jindani A, et al. Use of whole-genome sequencing to distinguish relapse from reinfection in a completed tuberculosis clinical trial. BMC Med 2017;15:71. [Crossref] [PubMed]
- Lewis KN, Liao R, Guinn KM, et al. Deletion of RD1 from Mycobacterium tuberculosis mimics bacille Calmette-Guérin attenuation. J Infect Dis 2003;187:117-23. [Crossref] [PubMed]
- Ru H, Liu X, Lin C, et al. The Impact of Genome Region of Difference 4 (RD4) on Mycobacterial Virulence and BCG Efficacy. Front Cell Infect Microbiol 2017;7:239. [Crossref] [PubMed]
- Mikheecheva NE, Zaychikova MV, Melerzanov AV, et al. A Nonsynonymous SNP Catalog of Mycobacterium tuberculosis Virulence Genes and Its Use for Detecting New Potentially Virulent Sublineages. Genome Biol Evol 2017;9:887-99. [Crossref] [PubMed]
- Baena A, Cabarcas F, Alvarez-Eraso KLF, et al. Differential determinants of virulence in two Mycobacterium tuberculosis Colombian clinical isolates of the LAM09 family. Virulence 2019;10:695-710. [Crossref] [PubMed]
- Li H. A statistical framework for SNP calling, mutation discovery, association mapping and population genetical parameter estimation from sequencing data. Bioinformatics 2011;27:2987-93. [Crossref] [PubMed]
- Comas I, Coscolla M, Luo T, et al. Out-of-Africa migration and Neolithic coexpansion of Mycobacterium tuberculosis with modern humans. Nat Genet 2013;45:1176-82. [Crossref] [PubMed]
- Li H, Durbin R. Fast and accurate short read alignment with Burrows-Wheeler transform. Bioinformatics 2009;25:1754-60. [Crossref] [PubMed]
- Kim S, Scheffler K, Halpern AL, et al. Strelka2: fast and accurate calling of germline and somatic variants. Nat Methods 2018;15:591-4. [Crossref] [PubMed]
- Wang K, Li M, Hakonarson H. ANNOVAR: functional annotation of genetic variants from high-throughput sequencing data. Nucleic Acids Res 2010;38:e164. [Crossref] [PubMed]
- Kurtz S, Phillippy A, Delcher AL, et al. Versatile and open software for comparing large genomes. Genome Biol 2004;5:R12. [Crossref] [PubMed]
- Nguyen LT, Schmidt HA, von Haeseler A, et al. IQ-TREE: a fast and effective stochastic algorithm for estimating maximum-likelihood phylogenies. Mol Biol Evol 2015;32:268-74. [Crossref] [PubMed]
- Kelley LA, Mezulis S, Yates CM, et al. The Phyre2 web portal for protein modeling, prediction and analysis. Nat Protoc 2015;10:845-58. [Crossref] [PubMed]
- Thuong NT, Tram TT, Dinh TD, et al. MARCO variants are associated with phagocytosis, pulmonary tuberculosis susceptibility and Beijing lineage. Genes Immun 2016;17:419-25. [Crossref] [PubMed]
- Malik AN, Godfrey-Faussett P. Effects of genetic variability of Mycobacterium tuberculosis strains on the presentation of disease. Lancet Infect Dis 2005;5:174-83. [Crossref] [PubMed]
- Chen H, He L, Huang H, et al. Mycobacterium tuberculosis Lineage Distribution in Xinjiang and Gansu Provinces, China. Sci Rep 2017;7:1068. [Crossref] [PubMed]
- Tram TTB, Nhung HN, Vijay S, et al. Virulence of Mycobacterium tuberculosis Clinical Isolates Is Associated With Sputum Pre-treatment Bacterial Load, Lineage, Survival in Macrophages, and Cytokine Response. Front Cell Infect Microbiol 2018;8:417. [Crossref] [PubMed]
- Wong KC, Leong WM, Law HK, et al. Molecular characterization of clinical isolates of Mycobacterium tuberculosis and their association with phenotypic virulence in human macrophages. Clin Vaccine Immunol 2007;14:1279-84. [Crossref] [PubMed]
- Theus SA, Cave MD, Eisenach KD. Intracellular macrophage growth rates and cytokine profiles of Mycobacterium tuberculosis strains with different transmission dynamics. J Infect Dis 2005;191:453-60. [Crossref] [PubMed]
- Kroesen VM, Madacki J, Frigui W, et al. Mycobacterial virulence: impact on immunogenicity and vaccine research. F1000Res 2019;8:eF1000 Faculty Rev-2025.
- Jia X, Yang L, Dong M, et al. The Bioinformatics Analysis of Comparative Genomics of Mycobacterium tuberculosis Complex (MTBC) Provides Insight into Dissimilarities between Intraspecific Groups Differing in Host Association, Virulence, and Epitope Diversity. Front Cell Infect Microbiol 2017;7:88. [Crossref] [PubMed]
- Forrellad MA, Klepp LI, Gioffré A, et al. Virulence factors of the Mycobacterium tuberculosis complex. Virulence 2013;4:3-66. [Crossref] [PubMed]
- Vera-Cabrera L, Hernández-Vera MA, Welsh O, et al. Phospholipase region of Mycobacterium tuberculosis is a preferential locus for IS6110 transposition. J Clin Microbiol 2001;39:3499-504. [Crossref] [PubMed]
- Goudarzi H, Mirsamadi E, Farnia P, et al. Phospholipase C in Beijing strains of Mycobacterium tuberculosis. Iran J Microbiol 2010;2:194-7. [PubMed]
- Raynaud C, Guilhot C, Rauzier J, et al. Phospholipases C are involved in the virulence of Mycobacterium tuberculosis. Mol Microbiol 2002;45:203-17. [Crossref] [PubMed]
- Zhang YY. Large sequence polymorphisms of Mycobacterium tuberculosis from Plateau and their relations with drug resistance[D]. Qinghai University, 2019.
(English Language Editor: C. Gourlay)