Comparison of clinical scores for predicting stroke-associated pneumonia after intracerebral hemorrhage (ICH): potential tools for personalized care and clinical trials for ICH
Introduction
Spontaneous intracerebral hemorrhage (ICH) accounts for approximately 15–20% of all strokes and is one of the leading causes of mortality and morbidity worldwide (1,2). Despite advances being made in medical knowledge, treatment for ICH remains strictly supportive, with few evidence-based interventions currently available (3,4).
Stroke-associated pneumonia (SAP) is a common medical complication after stroke and has a significant impact on patient outcomes. Evidence has shown that SAP not only increases the length of stay (LOS) in hospital and medical costs, but is also an important risk factor for poststroke mortality and morbidity (5). In addition, pneumonia has been found to increase the risk of several non-pneumonia-related medical complications, such as deep vein thrombosis, gastrointestinal bleeding, and atrial fibrillation (6). In a previous study, SAP was also found to be more common in patients with ICH than in those with acute ischemic stroke (AIS) (6,7). These data point to the need for more aggressive SAP prophylaxis among patients with ICH.
Several clinical scores have been developed for predicting SAP after ICH, such as the Veterans Health Administration (VHA) risk score (8), intracerebral hemorrhage-associated pneumonia score (ICH-APS; both ICH-APS-A and ICH-ASP-B) (9), the integer-based pneumonia risk score (ISAN) (10), the ACCD4 (age ≥75 years =1; congestive heart failure =1; dysarthria =1; dysphagia =4) (11), and the Preventive Antibiotics in Stroke Study (PASS) score (12). In the current study, the ISAN (10), ACCD4 (11), and PASS (12) scores were used for ICH patients in the derivation cohort, while the ICH-APS-A (9) and ICH-APS-B (9) scores were used exclusively for the ICH validation cohort (Tables S1,S2). Although a few of these ICH risk models have been internally or externally validated, none are universally accepted or consistently used in routine clinical practice or clinical research. In addition, with many grading systems available, it is becoming increasingly difficult for clinicians and researchers to determine which risk model provides the optimal predictability and reliability in clinical practice and clinical trials. Therefore, we believe it is necessary to conduct a head-to-head comparison of these models in an independent cohort.
In this study, we aimed to systematically compare the discrimination and calibration of 5 clinical scores for in-hospital SAP after ICH following the TRIPOD (Transparent Reporting of a Multivariable Prediction Model for Individual Prognosis or Diagnosis) guideline (13,14). We present the following article in accordance with the Standards for Reporting Diagnostic Accuracy Studies (STARD) reporting checklist (available at https://atm.amegroups.com/article/view/10.21037/atm-21-4046/rc).
Methods
Validation cohort
This was a multicenter, prospective, observational cohort study, with the validation cohort being derived from the Beijing Registration of Intracerebral Hemorrhage (15). A total of 13 hospitals in the Beijing area participated. To be eligible, patients had to meet the following criteria: (I) age 18 years or older; (II) hospitalized with a primary diagnosis of spontaneous ICH and confirmed by brain computed tomography (CT) or magnetic resonance imaging (MRI); (III) direct admission to hospital from a physician’s clinic or emergency department; and (IV) written informed consent from patients or their legal representatives. The study was conducted in accordance with the Declaration of Helsinki (as revised in 2013). The study protocol was approved by the Institutional Review Board (IRB) of the Beijing Tiantan Hospital (No. KY2014-023-02), and informed consent was given by all patients.
Data collection and definition of variables
A standardized electronic case report form (eCRF) was used for data collection. Participating centers collected data and submitted it online to the coordinating center at Beijing Tiantan Hospital. For this study, the following candidate variables were analyzed: (I) demographics; (II) time from onset to hospitalization (hours); (III) stroke risk factors, including hypertension, diabetes mellitus, dyslipidemia, atrial fibrillation, history of stroke/transient ischemic attack (TIA), myocardial infarction, heart failure, and current smoking or alcohol consumption; (IV) prestroke modified Rankin Scale (mRS) score; (V) preadmission antithrombotic medications; (VI) admission stroke severity based on the National Institutes of Health Stroke Scale (NIHSS) score and the Glasgow Coma Scale (GCS) score; (VII) admission blood pressure (mmHg); (VIII) admission laboratory tests; (IX) neuroimaging variables, including hematoma volume (measured using the ABC/2 method) (16), hematoma location (supratentorial or infratentorial ICH), intraventricular extension (presence or absence), and subarachnoid extension (presence or absence); (X) etiology diagnosis (primary or secondary ICH); (XI) surgical treatment (craniotomy evacuation, minimally invasive surgical therapy, or brain ventricle puncture and drainage); (XII) withdrawal of medical care; and (XIII) LOS in hospital.
Diagnosis of SAP
In-hospital SAP was diagnosed by the treating physician according to the Center for Disease Control and Prevention’s criteria for hospital-acquired pneumonia (17,18). This included a basis of clinical and laboratory indices of respiratory tract infection (fever, cough, new purulent sputum, and auscultatory respiratory crackles) and was supported by typical chest X-ray findings. In this study, the occurrence of prestroke pneumonia was not considered.
Statistical analysis
For our statistical analysis, categorical variables are summarized as proportions, while continuous variables are summarized with mean and SD, or median and interquartile range (IQR). Chi-square or Fisher’s exact test was used to compare categorical variables, and a Mann-Whitney test or independent t-test was employed to compare continuous variables between groups. Through a systematic search, we identified 6 clinical scores which could be used to predict SAP after ICH. However, the VHA score (8) could not be validated in the study as we did not have information on “found down at symptom onset”. This left us with 5 clinical scores for our study: ICH-APS-A (9), ICH-ASP-B (9), ISAN (10), ACCD4 (11), and PASS (12). Discrimination was assessed by calculating the area under the receiver operating characteristic curve (AUROC) (13,14). Pairwise AUROC was compared with Delong’s method (19). Sensitivity, specificity, positive predictive value (PPV), and negative predictive value (NPV) were calculated at each risk model’s maximum Youden index. Calibration, on the other hand, was evaluated using a Hosmer-Lemeshow goodness-of-fit test, and observed versus predicted risk was plotted according to 10 deciles of the predicted risk. We were then able to calculate the Cox and Snell R2 and Nagelkerke R2 (13,14). As the Hosmer-Lemeshow test has been shown to be overly sensitive (20), we also used Pearson correlation coefficient to calculate the observed and predicted risk. All tests were 2-tailed, and statistical significance was determined at a P level of .05. Statistical analysis was conducted using SAS 9.1 (SAS Institute Inc., Cary, NC, USA), SPSS 26.0 (IBM Corp., Armonk, NY, USA), and MedCalc 12.3 (MedCalc Software Ltd., Ostend, Belgium) software.
Results
Patient characteristics
From December 2014 to September 2016, a total of 1964 patients were enrolled in the Beijing Registration of Intracerebral Hemorrhage. Their clinical characteristics are shown in Table 1. The mean age was 56.8±14.4 years, and 67.6% were male. The median time from onset to hospitalization was 4.0 h (IQR, 1.90–11.1 h), and the median NIHSS and GCS score on admission was 11 (IQR, 3–21) and 14 (IQR, 8–15), respectively. The median hematoma volume on CT was 15.8 cm3 (IQR, 6.0–38.6 cm3), while the median LOS was 16 days (IQR, 8–22 days). A total of 575 (29.2%) patients were diagnosed with in-hospital SAP after ICH. Compared to patients without in-hospital SAP, those with in-hospital SAP after ICH were older and had a higher proportion of dysphagia, dysarthria, hematoma intraventricular extension, and subarachnoid extension. Furthermore, they had received surgical treatment and had a higher NIHSS score (at admission), blood pressure, blood glucose level, and hematoma volume, as well as a longer LOS (Table 1).
Table 1
Variable | Overall (n=1,964) | Without SAP (n=1,389) | With SAP (n=575) | P value |
---|---|---|---|---|
Demographics | ||||
Age, years | 56.8±14.4 | 55.9±14.1 | 59.0±14.6 | <0.001 |
Gender (male), n (%) | 1,327 (67.6) | 925 (66.6) | 402 (69.9) | 0.16 |
Onset to hospital (h), median (IQR) | 4.0 (1.90–11.0) | 4.13 (1.98–12.8) | 3.45 (1.82–8.00) | 0.001 |
Risk factors, n (%) | ||||
Hypertension | 1,367 (69.6) | 955 (68.8) | 412 (71.7) | 0.22 |
Diabetes mellitus | 289 (14.7) | 205 (14.8) | 84 (14.6) | 1.00 |
Dyslipidemia | 184 (9.4) | 138 (9.9) | 46 (8.0) | 0.20 |
Atrial fibrillation | 30 (1.5) | 19 (1.4) | 11 (1.9) | 0.42 |
History of stroke/TIA | 309 (15.7) | 199 (14.3) | 110 (19.1) | 0.01 |
Myocardial infarction | 38 (1.9) | 25 (1.8) | 13 (2.3) | 0.47 |
Heart failure | 8 (0.4) | 5 (0.4) | 3 (0.5) | 0.70 |
Current smoker | 628 (32.0) | 421 (30.3) | 207 (36.0) | 0.02 |
Alcohol consumption | 716 (36.5) | 500 (36.0) | 216 (37.6) | 0.53 |
Preadmission anticoagulation, n (%) | 21 (1.1) | 13 (0.9) | 8 (1.4) | 0.46 |
Preadmission antiplatelet, n (%) | 277 (14.1) | 178 (12.8) | 99 (17.2) | 0.01 |
Preadmission statins, n (%) | 113 (5.8) | 72 (5.2) | 41 (7.1) | 0.11 |
Prestroke mRS score, median (IQR) | 0 (0–0) | 0 (0–0) | 0 (0–0) | 0.02 |
Admission NIHSS score, median [IQR] | 11 [3–21] | 8 [2–15] | 20 [12–27] | <0.001 |
Admission GCS score, median [IQR] | 14 [8–15] | 14 [12–15] | 9 [6–13] | <0.001 |
Admission dysphagia, n (%) | 666 (33.9) | 286 (20.6) | 380 (66.1) | <0.001 |
Admission dysarthria, n (%) | 918 (46.7) | 599 (43.1) | 319 (55.5) | <0.001 |
Admission SBP (mmHg), median [IQR] | 165 [147–186] | 161 [145–182] | 173 [150–191] | <0.001 |
Admission DBP (mmHg), median [IQR] | 96 [82–109] | 95 [81–108] | 98 [84–110] | 0.009 |
Admission WBC, 109/L, median (IQR) | 9.79 (7.35–13.0) | 9.14 (7.03–11.9) | 11.5 (8.30–14.7) | <0.001 |
Admission glucose (mmol/L), median (IQR) | 5.85 (4.89–7.39) | 5.54 (4.72–6.93) | 6.57 (5.53–8.14) | <0.001 |
Admission creatinine (μmol/L), median (IQR) | 63.4 (52.7–77.0) | 63.1 (52.9–76.5) | 63.8 (52.0–77.1) | 0.89 |
Hematoma location, n (%) | 0.17 | |||
Supratentorial ICH | 1,752 (89.2) | 1,248 (89.8) | 504 (87.7) | |
Infratentorial ICH | 212 (10.8) | 141 (10.2) | 71 (12.3) | |
Hematoma volume (cm3), median (IQR) | 15.8 (6.0–38.6) | 12.0 (5.0–28.1) | 30.0 (12.8–59.3) | <0.001 |
Intraventricular extension, n (%) | 655 (33.4) | 368 (26.5) | 287 (49.9) | <0.001 |
Subarachnoid extension, n (%) | 264 (13.4) | 152 (10.9) | 112 (19.9) | <0.001 |
Etiology diagnosis, n (%) | 0.61 | |||
Primary ICH | 1,785 (90.9) | 1,259 (90.6) | 526 (91.5) | |
Secondary ICH | 159 (8.1) | 117 (8.4) | 42 (7.3) | |
Primary IVH | 20 (1.0) | 13 (0.9) | 7 (1.2) | |
Withdrawal of medical care, n (%) | 139 (7.1) | 86 (6.2) | 53 (9.2) | 0.02 |
Surgical treatment, n (%) | 366 (18.6) | 159 (11.4) | 207 (36.0) | <0.001 |
Length of hospital stay, median [IQR] | 16 [8–22] | 15 [8–20] | 19 [8–29] | <0.001 |
SAP, stroke-associated pneumonia; IQR, interquartile range; NIHSS, National Institutes of Health Stroke Scale score; GCS, Glasgow Coma Scale; SBP, systolic blood pressure; DBP, diastolic blood pressure; WBC, white blood cell count; ICH, intracerebral hemorrhage; mRS, modified Rankin Scale; IVH, intraventricular hemorrhage; TIA, transient ischemic stroke.
LOS and in-hospital SAP after ICH
Previous studies have shown that predicting SAP with a clinical score is more effective for patients who survive 48–72 h after ICH. For this reason, we performed a sensitivity analysis by LOS. Patient characteristics stratified by LOS (≤72 vs. >72 h) are shown in Table 2. Compared to patients with an LOS >72 h, those with an LOS ≤72 h had a significantly higher NIHSS score (P<0.001) upon admission, a lower GCS score (P<0.001), and a larger hematoma volume (P<0.001). However, the proportion of in-hospital SAP between the 2 groups was not statistically significant (30.2% vs. 29.2%; P=0.75). It was also found that patients with an LOS ≤72 h had a significantly higher rate of in-hospital mortality (40.6% vs. 7.4%; P<0.001) and withdrawal of medical care (18.4% vs. 5.7%; P<0.001; Table 2).
Table 2
Variable | Overall (n=1,964) | LOS ≤72 h (n=212) | LOS >72 h (n=1,752) | P value |
---|---|---|---|---|
Demographics | ||||
Age (years) | 56.8±14.4 | 57.1±13.3 | 56.7±14.4 | 0.73 |
Gender (male), n (%) | 1,327 (67.6) | 145 (68.4) | 1,182 (67.5) | 0.82 |
Onset to hospital (h), median (IQR) | 4.0 (1.90–11.0) | 4.01 (1.92–12.5) | 3.28 (1.98–5.96) | <0.001 |
Risk factors, n (%) | ||||
Hypertension | 1,367 (69.6) | 152 (71.7) | 1,215 (69.3) | 0.53 |
Diabetes mellitus | 289 (14.7) | 34 (16.0) | 255 (14.6) | 0.53 |
Dyslipidemia | 184 (9.4) | 22 (10.4) | 162 (9.2) | 0.62 |
Atrial fibrillation | 30 (1.5) | 4 (1.9) | 26 (1.5) | 0.41 |
History of stroke/TIA | 309 (15.7) | 27 (12.7) | 282 (16.1) | 0.23 |
Myocardial infarction | 38 (1.9) | 5 (2.4) | 33 (1.9) | 0.60 |
Heart failure | 8 (0.4) | 2 (0.9) | 6 (0.3) | 0.21 |
Current smoker | 628 (32.0) | 60 (28.3) | 568 (32.4) | 0.24 |
Alcohol consumption | 716 (36.5) | 70 (33.0) | 646 (36.9) | 0.29 |
Preadmission anticoagulation, n (%) | 21 (1.1) | 2 (0.9) | 19 (1.1) | 1.00 |
Preadmission antiplatelet, n (%) | 277 (14.1) | 29 (13.7) | 248 (14.2) | 0.92 |
Prestroke mRS score, median [IQR] | 0 [0–0] | 0 [0–0] | 0 [0–0] | 0.38 |
Admission NIHSS score, median [IQR] | 11 [3–21] | 26 [12–33] | 10 [3–18] | <0.001 |
Admission GCS score, median [IQR] | 14 [8–15] | 6 [3–13] | 14 [10–15] | <0.001 |
Admission dysphagia, n (%) | 666 (33.9) | 104 (49.1) | 562 (32.1) | <0.001 |
Admission dysarthria, n (%) | 918 (46.7) | 128 (60.4) | 790 (45.1) | <0.001 |
Admission SBP (mmHg), median [IQR] | 165 [147–186] | 173 [150–200] | 164 [146–185] | <0.001 |
Admission DBP (mmHg), median [IQR] | 96 [82–109] | 97 [85–110] | 95 [82–102] | 0.15 |
Admission WBC, 109/L, median (IQR) | 9.79 (7.35–13.0) | 12.4 (9.31–17.3) | 9.49 (7.20–12.4) | <0.001 |
Admission glucose (mmol/L), median (IQR) | 5.85 (4.89–7.39) | 8.84 (6.84–11.6) | 7.18 (6.02–8.88) | <0.001 |
Admission creatinine (μmol/L), median (IQR) | 63.4 (52.7–77.0) | 64.9 (53.6–83.0) | 63.1 (52.6–76.2) | 0.17 |
Hematoma location, n (%) | <0.001 | |||
Supratentorial ICH | 1,752 (89.2) | 172 (81.1) | 1,580 (90.2) | |
Infratentorial ICH | 212 (10.8) | 40 (18.9) | 172 (9.8) | |
Hematoma volume (cm3), median (IQR) | 15.8 (6.0–38.6) | 40.9 (12.0–78.1) | 15.0 (5.8–34.8) | <0.001 |
Intraventricular extension, n (%) | 655 (33.4) | 79 (37.3) | 576 (32.9) | 0.21 |
Subarachnoid extension, n (%) | 264 (13.4) | 55 (25.9) | 209 (11.9) | <0.001 |
Etiology diagnosis, n (%) | 0.02 | |||
Primary ICH | 1,785 (90.9) | 204 (96.2) | 1,581 (90.2) | |
Secondary ICH | 159 (8.1) | 7 (3.3) | 152 (8.7) | |
Primary IVH | 20 (1.0) | 1 (0.5) | 19 (1.1) | |
Withdrawal of medical care, n (%) | 139 (7.1) | 39 (18.4) | 100 (5.7) | <0.001 |
Surgical treatment, n (%) | 366 (18.6) | 18 (8.5) | 348 (19.9) | <0.001 |
In-hospital SAP, n (%) | 575 (29.3) | 64 (30.2) | 511 (29.2) | 0.75 |
In-hospital mortality, n (%) | 216 (11.0) | 86 (40.6) | 130 (7.4) | <0.001 |
IQR, interquartile range; NIHSS, National Institutes of Health Stroke Scale score; GCS, Glasgow Coma Scale; SBP, systolic blood pressure; DBP, diastolic blood pressure; WBC, white blood cell count; ICH, intracerebral hemorrhage; mRS, modified Rankin Scale; TIA, transient ischemic stroke; IVH, intraventricular hemorrhage; LOS, length of stay; SAP, stroke-associated pneumonia.
Comparison of model discrimination for in-hospital SAP
Figure 1 shows the discrimination of the 5 clinical scores regarding in-hospital SAP after ICH. The AUROC of the 5 scores ranged from 0.732 to 0.800 (Table 3). The sensitivity, specificity, PPV, NPV, and maximum Youden index for predicting in-hospital SAP after ICH are shown in Table 3. We found that the ICH-SAP-B had the maximum value in the Youden index. In the comparison of scores, the ICH-APS-B (0.800; 95% CI: 0.780–0.820; P<0.001) also showed a statistically better discrimination than did the other risk models for in-hospital SAP after ICH (all P<0.001). However, all risk models showed a much better discrimination in patients with an LOS >72 h (Table 3). Among these patients with an LOS >72 h, the ICH-APS-B (0.827; 95% CI: 0.806–0.848; P<0.001) still showed statistically better discrimination for in-hospital SAP after ICH than did the other risk models (all P<0.001).
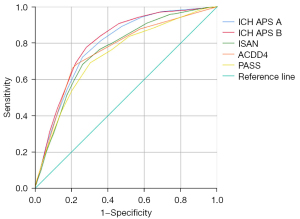
Table 3
Variable | AUROC | 95% CI | Δ AUROC* | P value& | Youden Index | Cutoff | Sensitivity | Specificity | PPV | NPV |
---|---|---|---|---|---|---|---|---|---|---|
Overall cohort (N=1,964) | ||||||||||
ICH-APS-B (2014) | 0.800 | 0.780–0.820 | Reference | 0.495 | 6 | 0.777 | 0.718 | 0.533 | 0.886 | |
ICH-APS-A (2014) | 0.787 | 0.766–0.808 | 0.013 | <0.001 | 0.462 | 6 | 0.724 | 0.739 | 0.534 | 0.866 |
ISAN (2015) | 0.755 | 0.732–0.777 | 0.045 | <0.001 | 0.421 | 8 | 0.683 | 0.737 | 0.519 | 0.849 |
ACDD4 (2017) | 0.755 | 0.735–0.774 | 0.045 | <0.001 | 0.456 | 3 | 0.662 | 0.794 | 0.571 | 0.850 |
PASS (2018) | 0.732 | 0.708–0.756 | 0.068 | <0.001 | 0.388 | 6 | 0.692 | 0.696 | 0.485 | 0.845 |
LOS ≤72 h (N=288) | ||||||||||
ICH-APS-B (2014) | 0.639 | 0.574–0.703 | Reference | 0.265 | 6 | 0.830 | 0.435 | 0.392 | 0.853 | |
ICH-APS-A (2014) | 0.637 | 0.573–0.702 | 0.002 | 0.92 | 0.272 | 6 | 0.773 | 0.500 | 0.405 | 0.833 |
ISAN (2015) | 0.599 | 0.533–0.665 | 0.040 | 0.09 | 0.214 | 9 | 0.784 | 0.430 | 0.377 | 0.819 |
ACDD4 (2017) | 0.648 | 0.580–0.716 | 0.009 | 0.73 | 0.319 | 2 | 0.704 | 0.615 | 0.446 | 0.826 |
PASS (2018) | 0.627 | 0.556–0.697 | 0.012 | 0,70 | 0.249 | 7 | 0.534 | 0.715 | 0.452 | 0.777 |
LOS >72 h (N=1,676) | ||||||||||
ICH-APS-B (2014) | 0.827 | 0.806–0.848 | Reference | 0.533 | 6 | 0.768 | 0.765 | 0.573 | 0.890 | |
ICH-APS-A (2014) | 0.811 | 0.789–0.832 | 0.016 | <0.001 | 0.493 | 6 | 0.714 | 0.779 | 0.570 | 0.869 |
ISAN (2015) | 0.780 | 0.756–0.804 | 0.047 | <0.001 | 0.455 | 8 | 0.661 | 0.793 | 0.568 | 0.851 |
ACDD4 (2017) | 0.773 | 0.747–0.798 | 0.055 | <0.001 | 0.482 | 1 | 0.679 | 0.802 | 0.585 | 0.859 |
PASS (2018) | 0.750 | 0.724–0.775 | 0.077 | <0.001 | 0.421 | 6 | 0.702 | 0.718 | 0.505 | 0.855 |
*, Δ AUROC denotes the difference in AUROC between the ICH-SAP-B and the other scores regarding SAP after ICH; &, P value of comparing paired AUROC with Delong’s method. ICH, intracerebral hemorrhage; AUROC, area under the receiver operating characteristic curve; PPV, positive predictive value; NPV, negative predictive value; PASS, Preventive Antibiotics in Stroke Study Score; LOS, length of stay; ISAN, integer-based pneumonia risk score; ACCD4, an 8-point pneumonia prediction scale (age ≥75 years =1; congestive heart failure =1; dysarthria =1; dysphagia =4); ICH-APS, intracerebral hemorrhage–associated pneumonia score.
Comparison of model calibration for in-hospital SAP
We plotted the predicted and observed risk according to 10 deciles of the predicted risk of in-hospital SAP after ICH (Figure S1). The results of the Hosmer-Lemeshow test are shown in Table 4. In the overall cohort, the Hosmer-Lemeshow test showed that the ICH-APS-B had a significance level greater than .05, indicating that the observed values were not statistically different from the expected values. The ICH-SAP-B had the largest Cox and Snell R2. Similar results were found for patients with an LOS >72 h. As the Hosmer-Lemeshow test has been shown to be overly sensitive to trivial deviations from the ideal fit, Pearson correlation coefficient was also used to calculate the predicted and observed risk. All the correlation coefficients of the 5 models were greater than 0.90 (all P<0.001), and the ICH-SAP-B had the largest Pearson correlation coefficient.
Table 4
International ICH models | Goodness-of-fit test regarding SAP after ICH | ||
---|---|---|---|
P value | Cox and Snell R2 | Nagelkerke R2 | |
Overall cohort (N=1,964) | |||
ICH-APS-B (2014) | 0.08 | 0.210 | 0.299 |
ICH-APS-A (2014) | <0.05 | 0.194 | 0.277 |
ISAN (2015) | <0.05 | 0.155 | 0.221 |
ACDD4 (2017) | <0.05 | 0.178 | 0.253 |
PASS (2018) | <0.05 | 0.128 | 0.183 |
LOS ≤72 h (N=288) | |||
ICH-APS-B (2014) | 0.05 | 0.055 | 0.078 |
ICH-APS-A (2014) | 0.09 | 0.053 | 0.074 |
ISAN (2015) | 0.04 | 0.030 | 0.043 |
ACDD4 (2017) | 0.30 | 0.072 | 0.102 |
PASS (2018) | 0.37 | 0.036 | 0.050 |
LOS >72 h (N=1,676) | |||
ICH-APS-B (2014) | 0.10 | 0.251 | 0.358 |
ICH-APS-A (2014) | <0.05 | 0.228 | 0.326 |
ISAN (2015) | <0.05 | 0.190 | 0.271 |
ACDD4 (2017) | 0.09 | 0.202 | 0.288 |
PASS (2018) | <0.05 | 0.149 | 0.212 |
ICH-APS, intracerebral hemorrhage–associated pneumonia score; ISAN, integer-based pneumonia risk score; ACCD4, an 8-point pneumonia prediction scale (age ≥75 years =1; congestive heart failure =1; dysarthria =1; dysphagia =4); PASS, Preventive Antibiotics in Stroke Study Score; LOS, length of stay.
Discussion
In this study, we systematically compared the discrimination and calibration of 5 clinical scores regarding in-hospital SAP after ICH. The AUROC ranged from 0.732 to 0.800 in the overall cohort. Through a comparative analysis, we found the ICH-APS-B showed statistically better discrimination than did the other risk models in terms of in-hospital SAP after ICH. Furthermore, all risk models showed much better discrimination among patients with an LOS >72 h. The ICH-APS-B still showed statistically better discrimination than did the other risk models for in-hospital SAP after ICH in patients with an LOS >72 h. The Hosmer-Lemeshow test also revealed that the ICH-SAP-B had the largest Cox and Snell R2 among patients with an LOS >72 h.
Previous studies have shown that clinical scores for predicting SAP performed better in sensitivity analyses for patient survival beyond 48–72 h after ICH (9,10). We found the potential reasons for this to be worth investigating. To this end, we compared the baseline characteristics of patients with an LOS ≤72 h and those with an LOS >72 h and found that patients with a shorter LOS had a significantly more severe neurological deficit on admission as indicated by a higher NIHSS score, lower GCS score, and larger hematoma volume. However, these factors did not correspond to an increased risk of in-hospital SAP after ICH. The rates of in-hospital SAP between the 2 groups were not statistically different. Furthermore, we found that patients with a shorter LOS had a significantly higher proportion of in-hospital mortality and withdrawal of medical care (Table 2). For our study, limited data were available about the time course of SAP after stroke. However, previous studies have reported a 3-day (IQR, 2–5) median time from onset to diagnosis of SAP after ICH), a 4-day median for AIS (IQR, 2–7), and a 5-day median for subarachnoid hemorrhage (SAH; IQR, 3–7) (21). Therefore, based on these data, we conjectured that the contradiction between neurological severity and risk of in-hospital SAP after ICH in patients with an LOS ≤72 h might be due to the patient dying or leaving hospital before the pneumonia occurred. For this patient subgroup of in-hospital pneumonia after ICH, this might be a potential reason for the lower sensitivity of these clinical scores. Despite advances in medical knowledge, treatment for ICH remains strictly supportive, with few evidence-based interventions currently available (3,4). Evidence has shown that SAP not only increases LOS and medical costs, but is also an important risk factor for poststroke mortality and morbidity. In addition, a previous study has shown the rate of SAP to be higher among patients with ICH than those with AIS (6). These data suggest that compared to patients with AIS, patients with ICH require a more aggressive SAP prophylaxis. Several clinical scores have been developed for predicting SAP after ICH. Although some risk models have been internally or externally validated, none of them have been universally accepted or consistently used in routine clinical practice and clinical research. In this study’s large ICH cohort (n=1,964), the ICH-APS-B showed a statistically better discrimination than did the other risk models regarding in-hospital SAP after ICH. It also displayed the largest Cox and Snell R2 through the Hosmer-Lemeshow goodness-of-fit test, which was used to predict in-hospital SAP after ICH. Although these results are promising, caution needs to be taken when interpreting the results, as the cohorts used to develop these clinical scores were different. In other words, the ISAN, ACCD4, and PASS scores were only used for ICH patients in the derivation cohort, while the ICH-APS-A and ICH-APS-B scores were used exclusively for the ICH validation cohort. Furthermore, the baseline characteristics between these 2 study groups were different, and there might be complex genetic, social, and economic factors, as well as regional management philosophies and preferences, that are difficult to account for when risk models are developed or applied to a distinct population. Therefore, these clinical scores need to be further validated by a study with a larger sample size.
In 2 recent large, randomized trials [STROKE-INF (22) and PASS (23)], preventive antibiotic therapy did not improve functional outcomes after stroke. These trials selected patients based on symptoms and signs, with a “one-size-fits-all” prevention strategy being developed for the average person. This approach gave less consideration to the differences in SAP risk between individuals. In this way, it was inevitable that they would include patients with an unbalanced, too-high, or too-low risk of developing SAP in their clinical trials. In our study, we found the clinical scores we validated could be used to stratify patients by risk of SAP after stroke, meaning they could aid the testing of prophylactic antibiotics in different risk stratifications. Clinical trials conducted in this way would allow one to more accurately clarify which prophylactic antibiotics will work better for different SAP stratifications. However, whether preventive antibiotic therapy in a high-risk subgroup of SAP patients can improve functional outcomes after ICH requires further investigation.
It is also important to note that our study has certain limitations. First, like all observational studies, we cannot rule out the possibility that additional baseline variables (unmeasured confounders), such as use of angiotensin-converting enzyme inhibitors (24), acid-suppressive medications (25), oral hygiene (26), and biomarkers for stroke-induced immunodepression (27,28), might have some impact on the development of in-hospital SAP after ICH. Second, we could not include all elements required for all risk models. For example, we did not have information about the “found down at symptom onset”, and the VHA score (8) could not be validated. Third, our study included only hospitalized patients, and patients who died in the emergency room or who were treated in outpatient clinics were not included. Furthermore, like most registries, our registry required informed consent, and selection bias was therefore inevitable (29). Lastly, the validation cohorts originated from an Asian population, and thus the ICH models need to be further validated in different populations.
Conclusions
In summary, among the 5 models for predicting SAP after ICH, the ICH-APS-B showed the best predictive performance. This indicates that the model can be applied in personalized care and clinical trials of SAP after ICH.
Acknowledgments
Funding: This study was sponsored by Beijing Municipal Science & Technology Commission (Z131107002213009), the Nova Program of Beijing Science and Technology Commission (2008B30), the National Natural Science Foundation of China (81471208 and 81641162), the Beijing High-Level Healthy Human Resource Project (014-3-033), and the Shandong Province Key Innovation Project (2019JZZY020901).
Footnote
Reporting Checklist: The authors have completed the STARD reporting checklist. Available at https://atm.amegroups.com/article/view/10.21037/atm-21-4046/rc
Data Sharing Statement: Available at https://atm.amegroups.com/article/view/10.21037/atm-21-4046/dss
Peer Review File: Available at https://atm.amegroups.com/article/view/10.21037/atm-21-4046/prf
Conflicts of Interest: All authors have completed the ICMJE uniform disclosure form (available at https://atm.amegroups.com/article/view/10.21037/atm-21-4046/coif). The authors have no conflicts of interest to declare.
Ethical Statement: The authors are accountable for all aspects of the work in ensuring that questions related to the accuracy or integrity of any part of the work are appropriately investigated and resolved. The study was conducted in accordance with the Declaration of Helsinki (as revised in 2013). The study protocol was approved by the Institutional Review Board (IRB) of the Beijing Tiantan Hospital (No. KY2014-023-02). Informed consent was given by all patients involved.
Open Access Statement: This is an Open Access article distributed in accordance with the Creative Commons Attribution-NonCommercial-NoDerivs 4.0 International License (CC BY-NC-ND 4.0), which permits the non-commercial replication and distribution of the article with the strict proviso that no changes or edits are made and the original work is properly cited (including links to both the formal publication through the relevant DOI and the license). See: https://creativecommons.org/licenses/by-nc-nd/4.0/.
References
- Virani SS, Alonso A, Benjamin EJ, et al. Heart Disease and Stroke Statistics-2020 Update: A Report From the American Heart Association. Circulation 2020;141:e139-596. [Crossref] [PubMed]
- Wang W, Jiang B, Sun H, et al. Prevalence, Incidence, and Mortality of Stroke in China: Results from a Nationwide Population-Based Survey of 480 687 Adults. Circulation 2017;135:759-71. [Crossref] [PubMed]
- Hemphill JC 3rd, Greenberg SM, Anderson CS, et al. Guidelines for the Management of Spontaneous Intracerebral Hemorrhage: A Guideline for Healthcare Professionals From the American Heart Association/American Stroke Association. Stroke 2015;46:2032-60. [Crossref] [PubMed]
- Steiner T, Al-Shahi Salman R, Beer R, et al. European Stroke Organisation (ESO) guidelines for the management of spontaneous intracerebral hemorrhage. Int J Stroke 2014;9:840-55. [Crossref] [PubMed]
- Teh WH, Smith CJ, Barlas RS, et al. Impact of stroke-associated pneumonia on mortality, length of hospitalization, and functional outcome. Acta Neurol Scand 2018;138:293-300. [Crossref] [PubMed]
- Ji R, Wang D, Shen H, et al. Interrelationship among common medical complications after acute stroke: pneumonia plays an important role. Stroke 2013;44:3436-44. [Crossref] [PubMed]
- Kwan J, Pickering RM, Kunkel D, et al. Impact of stroke-associated infection on long-term survival: a cohort study. J Neurol Neurosurg Psychiatry 2013;84:297-304. [Crossref] [PubMed]
- Chumbler NR, Williams LS, Wells CK, et al. Derivation and validation of a clinical system for predicting pneumonia in acute stroke. Neuroepidemiology 2010;34:193-9. [Crossref] [PubMed]
- Ji R, Shen H, Pan Y, et al. Risk score to predict hospital-acquired pneumonia after spontaneous intracerebral hemorrhage. Stroke 2014;45:2620-8. [Crossref] [PubMed]
- Smith CJ, Bray BD, Hoffman A, et al. Can a novel clinical risk score improve pneumonia prediction in acute stroke care? A UK multicenter cohort study. J Am Heart Assoc 2015;4:e001307. [Crossref] [PubMed]
- Kumar S, Marchina S, Massaro J, et al. ACDD4 score: A simple tool for assessing risk of pneumonia after stroke. J Neurol Sci 2017;372:399-402. [Crossref] [PubMed]
- Westendorp WF, Vermeij JD, Hilkens NA, et al. Development and internal validation of a prediction rule for post-stroke infection and post-stroke pneumonia in acute stroke patients. Eur Stroke J 2018;3:136-44. [Crossref] [PubMed]
- Moons KG, Altman DG, Reitsma JB, et al. Transparent Reporting of a multivariable prediction model for Individual Prognosis or Diagnosis (TRIPOD): explanation and elaboration. Ann Intern Med 2015;162:W1-73. [Crossref] [PubMed]
- Collins GS, Reitsma JB, Altman DG, et al. Transparent reporting of a multivariable prediction model for individual prognosis or diagnosis (TRIPOD): the TRIPOD statement. BMJ 2015;350:g7594. [Crossref] [PubMed]
- Ji R, Wang W, Liu X, et al. Head-to-head comparison of prognostic models of spontaneous intracerebral hemorrhage: tools for personalized care and clinical trial in ICH. Neurol Res 2022;44:146-55. [Crossref] [PubMed]
- Kothari RU, Brott T, Broderick JP, et al. The ABCs of measuring intracerebral hemorrhage volumes. Stroke 1996;27:1304-5. [Crossref] [PubMed]
- Garner JS, Jarvis WR, Emori TG, et al. CDC definitions for nosocomial infections, 1988. Am J Infect Control 1988;16:128-40. [Crossref] [PubMed]
- Smith CJ, Kishore AK, Vail A, et al. Diagnosis of Stroke-Associated Pneumonia: Recommendations From the Pneumonia in Stroke Consensus Group. Stroke 2015;46:2335-40. [Crossref] [PubMed]
- DeLong ER, DeLong DM, Clarke-Pearson DL. Comparing the areas under two or more correlated receiver operating characteristic curves: a nonparametric approach. Biometrics 1988;44:837-45. [Crossref] [PubMed]
- Kramer AA, Zimmerman JE. Assessing the calibration of mortality benchmarks in critical care: The Hosmer-Lemeshow test revisited. Crit Care Med 2007;35:2052-6. [Crossref] [PubMed]
- Liu X, Wang L, Li G, et al. Characteristics of Stroke Associated Pneumonia by Stroke Subtypes: Incidence, Time Course, Potential Risk and Microbiological Pathogen. Int J Cerebrovasc Dis Stroke 2021;4:129.
- Kalra L, Irshad S, Hodsoll J, et al. Prophylactic antibiotics after acute stroke for reducing pneumonia in patients with dysphagia (STROKE-INF): a prospective, cluster-randomised, open-label, masked endpoint, controlled clinical trial. Lancet 2015;386:1835-44. [Crossref] [PubMed]
- Westendorp WF, Vermeij JD, Zock E, et al. The Preventive Antibiotics in Stroke Study (PASS): a pragmatic randomised open-label masked endpoint clinical trial. Lancet 2015;385:1519-26. [Crossref] [PubMed]
- Caldeira D, Alarcão J, Vaz-Carneiro A, et al. Risk of pneumonia associated with use of angiotensin converting enzyme inhibitors and angiotensin receptor blockers: systematic review and meta-analysis. BMJ 2012;345:e4260. [Crossref] [PubMed]
- Marchina S, Doros G, Modak J, et al. Acid-suppressive medications and risk of pneumonia in acute stroke patients: A systematic review and meta-analysis. J Neurol Sci 2019;400:122-8. [Crossref] [PubMed]
- Wagner C, Marchina S, Deveau JA, et al. Risk of Stroke-Associated Pneumonia and Oral Hygiene. Cerebrovasc Dis 2016;41:35-9. [Crossref] [PubMed]
- Mengel A, Ulm L, Hotter B, et al. Biomarkers of immune capacity, infection and inflammation are associated with poor outcome and mortality after stroke - the PREDICT study. BMC Neurol 2019;19:148. [Crossref] [PubMed]
- Nam KW, Kim TJ, Lee JS, et al. High Neutrophil-to-Lymphocyte Ratio Predicts Stroke-Associated Pneumonia. Stroke 2018;49:1886-92. [Crossref] [PubMed]
- Tu JV, Willison DJ, Silver FL, et al. Impracticability of informed consent in the Registry of the Canadian Stroke Network. N Engl J Med 2004;350:1414-21. [Crossref] [PubMed]