Development of a prognostic nomogram based on an eight-gene signature for esophageal squamous cell carcinoma by weighted gene co-expression network analysis (WGCNA)
Introduction
Esophageal cancer is the eighth most common cancer globally (1). Due to recurrence and metastasis, its 5-year survival rate is <20% (2). Esophageal squamous cell carcinoma (ESCC) is the main malignant subtype of esophageal cancer, accounting for over 90% of esophageal cancer cases (3). Despite advances in diagnosis and treatment techniques for ESCC, the 5-year survival rate is still very low (4). Current treatment methods include chemotherapy, radiation therapy, and surgery. There is still a lack of approved targeted therapy drugs for ESCC (5). The tumor node metastasis (TNM) staging system remains the gold standard for ESCC prognosis. Due to the heterogeneity of ESCC, the prognosis of patients in the same clinical stage varies (6). That is to say, relying on the TNM staging system to predict the prognosis of ESCC is often not accurate enough. Therefore, predictors that can accurately assess ESCC prognosis will be of great value for the individualized management of ESCC1.
With the development of high-throughput technologies such as microarray and RNA-seq, gene expression profiling has become a powerful tool for identifying prognostic biomarkers of ESCC (7-9). Furthermore, various differentially expressed genes (DEGs) and signaling pathways involved in the progression of ESCC have been identified (7-9). Nevertheless, the application of relevant research to clinical practice guidance is still very few. In this study, to obtain reliable results, we first used 2 independent datasets to build the 2 ESCC-related co-expression modules via weighted gene co-expression network analysis (WGCNA). By combining DEGs and genes in the ESCC-related modules, an 8-gene module was developed. Due to the heterogeneity and complexity of ESCC, multi-parameter markers are more accurate than a single marker for ESCC prognosis (10). Therefore, this study established a prognostic nomogram based on the 8-gene module and other factors. Furthermore, we explored the underlying mechanisms of the 8 genes during ESCC progression. Our findings may provide novel clues for the development of a promising prognostic tool for ESCC.
We present the following article in accordance with the TRIPOD reporting checklist (available at https://atm.amegroups.com/article/view/10.21037/atm-21-6935/rc).
Methods
Datasets
ESCC microarray and RNA-seq expression profiles were retrieved from the Gene Expression Omnibus (GEO) (https://www.ncbi.nlm.nih.gov/geo/) database (accession: GSE23400 and GSE130078) and The Cancer Genome Atlas (TCGA) database (https://portal.gdc.cancer.gov/). The GSE23400 dataset contained 53 ESCC samples and 53 matched normal samples (11). The GSE130078 dataset contained 23 ESCC samples and 23 corresponding normal samples (12). The study was conducted in accordance with the Declaration of Helsinki (as revised in 2013).
WGCNA
The GSE23400 and GSE130078 datasets were used for WGCNA, which was performed by the WGCNA package in R (13). To ensure a scale-free co-expression network, the soft threshold value β (the range was 0–30) was determined by the pickSoftThreshold function. The correlation coefficient matrix between genes (called an adjacency matrix) was constructed. Genes with similar expression patterns were assigned into a module. The dynamic cutting tree method was utilized to assign gene modules. Using topological overlap matrix (TOM), co-expression modules were constructed. The minimum number of genes in each gene module was set to 30. The correlation between gene significance (GS) and module significance (MS) was assessed.
Differential expression analysis
Differential expression analysis between ESCC and normal samples was performed using the GEO2R and DESq2 packages in R in the GSE23400 and GSE130078 datasets (14). The threshold of DEGs was set as adjusted P value <0.05 and log |fold change (FC)| >1. P values were corrected by Bonferroni’s method.
Functional enrichment analysis
Functional enrichment analysis was carried out via the Metascape online database (15). Metascape integrates multiple authoritative data resources such as Gene Ontology (GO), Kyoto Encyclopedia of Genes and Genomes (KEGG), UniProt, and DrugBank. It not only completes pathway enrichment and biological process annotation, but also performs gene-related protein network analysis and related information. Based on the integration of the above-mentioned database information, the rich biological pathways and protein complexes contained in the data are explained. The adjusted P value <0.05 was set as a significant result.
Construction of a prognostic risk score
Gene expression data and clinical information were obtained from TCGA database. Univariate Cox regression analysis was utilized to screen survival-related genes (P<0.05). The results were visualized by the forest package in R. Using multivariate Cox regression analysis, a prognostic model was built and the risk score was calculated according to the expression level of each gene and its regression coefficient. The Akaike information criterion (AIC) was calculated to assess the model. All ESCC samples from TCGA database were divided into high- and low-risk score groups. Kaplan-Meier survival analysis was then performed using the survival package in R. The prediction efficacy of the model was assessed by construction of a time-dependent receiver operating characteristic (ROC) curve utilizing the survivalROC package in R.
Nomogram
Based on the Cox proportional hazards regression model, a nomogram was constructed by integrating gender, age, stage, and the risk score through the rms package in R. The Bootstrap self-sampling method was utilized to verify the prediction effect of the model, which was assessed by the C-index.
mRNA-lncRNA co-expression network
The correlation between mRNAs and lncRNAs was analyzed based on the disease-related co-expression gene modules. Then, the mRNA-lncRNA co-expression network was visualized using Cytoscape software (version 3.7.2) (16). Functional enrichment analysis of the co-expressed mRNAs was achieved using the Metascape database.
Statistical analysis
All analyses were performed with R version 4.0.2 (https://www.r-project.org/) and the corresponding packages. OS was assessed with the Kaplan-Meier method and log-rank test for variance analysis. P value less than 0.05 was considered statistically significant.
Results
Identification of ESCC-related co-expression modules
In this study, 2 datasets were utilized for WGCNA. In the GSE23400 dataset, to ensure the network was scale-free, the optimal soft threshold β was determined as 6 (Figure 1A). Highly similar genes were assigned to a module. Finally, a total of 13 modules were determined by dynamic cutting tree (Figure 1B). A total of 400 genes were randomly selected for the heatmap. As shown in Figure 1C, 1 module was independent from the others. Among the 13 modules, the brown module was significantly correlated with ESCC (r=0.74 and P=4e−10), which was considered as a disease-related module (Figure 1D). In Figure 1E, the genes in the brown module were highly related to ESCC (r=0.82 and P<1e−200). Furthermore, we performed WGCNA in the GSE130078 dataset. The optimal soft threshold β was set to 20 (Figure 2A). Following module assignment by dynamic cutting tree, 13 modules were constructed (Figure 2B). In Figure 2C, the heatmap depicted that 1 module was independent from the others based on the 400 randomly selected genes. The yellow module had the highest correlation with ESCC (Figure 2D; r=0.9 and P=3e−17). In the module, the genes had a highly positive relationship with ESCC (Figure 2E; r=0.87 and P<1e−200).
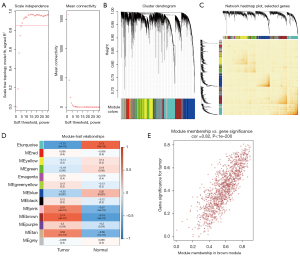
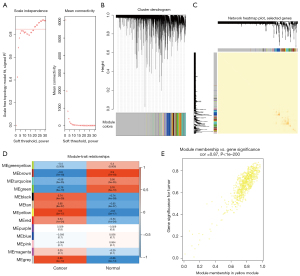
DEGs in the ESCC-related co-expression modules
The genes in the ESCC-related “brown” module obtained from the GSE23400 dataset were intersected with the genes in the ESCC-related “yellow” module from the GSE130078 dataset. These overlapped genes were considered as ESCC-related genes. With the threshold of adjusted P value <0.05 and log |FC| >1, 222 DEGs were screened between ESCC and normal samples in the GSE23400 dataset (table available at https://cdn.amegroups.cn/static/public/atm-21-6935-1.xlsx). Furthermore, 5,661 DEGs were identified for ESCC in the GSE130078 dataset (table available at https://cdn.amegroups.cn/static/public/atm-21-6935-2.xlsx). Then, these ESCC-related genes were overlapped with DEGs from the 2 datasets. Finally, 3 ESCC-related DEGs (DUXAP10, WDR72, and FST) were identified, which could be critical genes for ESCC (Figure 3A). To probe the underlying biological functions and pathways of the genes in the 2 ESCC-related co-expression modules, functional enrichment analysis was carried out using the Metascape database. In Figure 3B,3C, genes in the ESCC-related co-expression module from the GSE23400 dataset were mainly involved in mitochondrial gene expression, non-coding RNA (ncRNA) metabolic process, and chromosome segregation. Genes in the module from the GSE130078 dataset were mainly involved in extracellular matrix organization, collagen formation, NABA matrisome associated, skeletal system development, PID integrin 1 pathway, blood vessel development, collagen fibril organization, and regulation of cell adhesion (Figure 3D,3E).
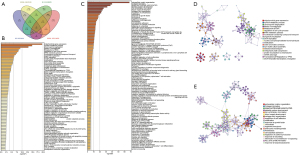
A nomogram based on an 8-gene prognostic model for ESCC
After univariate Cox regression analysis, 55 survival-related genes were identified for ESCC through TCGA database (Table 1). MAGEA6, MAGEA3, LINC01524, CFAP53, IGF2, GNGT1, FCGR3A, and FCGR2A were used to construct a prognostic model for ESCC following multivariate Cox regression analysis (Table 2). The risk score was calculated based on coefficients and their expression levels. Among them, in Figure 4A, MAGEA6 [hazard ratio (HR): 0.270, 95% confidence interval (CI): 0.087–0.850, P=0.026], CFAP53 (HR: 0.080, 95% CI: 0.014–0.460, P=0.004), GNGT1 (HR: 0.340, 95% CI: 0.150–0.920, P=0.009), and FCGR3A (HR: 0.370, 95% CI: 0.150–0.920, P=0.033) were protective factors for ESCC. Also, LINC01524 (HR: 2.7e+06, 95% CI: 518.649–0.850, P<0.01), IGF2 (HR: 2.000, 95% CI: 1.205–3.400, P=0.008), and FCGR2A (HR: 3.400, 95% CI: 1.287–9.200, P=0.014) were risk factors for ESCC. All ESCC patients were divided into high and low risk groups in line with the median value of the risk score. Kaplan-Meier survival analysis results demonstrated that patients with a high-risk score usually had a poorer overall survival (OS) time than those with a low-risk score (Figure 4B; P=1.78e−05). An ROC curve was generated to validate the prediction performance for the prognosis of ESCC. The area under the curve (AUC) was 0.961, suggesting that the risk score was highly sensitive and accurate for prognostic prediction (Figure 4C). Furthermore, 4 prognostic factors (gender, age, stage, and risk score) were used to establish a nomogram for OS prediction. As shown in Figure 4D, the predictive ability of the nomogram was accurate for the OS of ESCC patients.
Table 1
Gene | HR | z | P value |
---|---|---|---|
MAGEA6 | 0.632622 | −3.06737 | 0.00216 |
MAGEA3 | 0.64959 | −3.04259 | 0.002346 |
LINC02154 | 1.57539 | 3.030769 | 0.002439 |
AMIGO2 | 2.050024 | 3.015008 | 0.00257 |
LUCAT1 | 3.411003 | 2.91137 | 0.003598 |
TREML2 | 13.93435 | 2.894222 | 0.003801 |
LINC02081 | 2.495196 | 2.836985 | 0.004554 |
LINC01524 | 9571.259 | 2.829145 | 0.004667 |
CFAP53 | 0.286082 | −2.78916 | 0.005284 |
IGF2 | 1.681858 | 2.771445 | 0.005581 |
GNGT1 | 0.425633 | −2.77041 | 0.005599 |
HAS2-AS1 | 2.432934 | 2.74082 | 0.006129 |
SLC44A5 | 0.511921 | −2.70496 | 0.006831 |
IFITM3 | 1.839623 | 2.681378 | 0.007332 |
FCGR3A | 1.722055 | 2.644744 | 0.008175 |
FCGR2A | 1.802957 | 2.623342 | 0.008707 |
FCER1G | 1.80513 | 2.608749 | 0.009087 |
IFITM1 | 1.556451 | 2.582223 | 0.009817 |
MSC | 1.548024 | 2.531968 | 0.011342 |
LINC00898 | 0.018806 | −2.52327 | 0.011627 |
KIAA1324L | 0.427248 | −2.44412 | 0.014521 |
RPL29P19 | 1.60387 | 2.443574 | 0.014543 |
SLC2A3 | 1.885667 | 2.435088 | 0.014888 |
GAS1 | 1.462505 | 2.402565 | 0.016281 |
C3AR1 | 2.049546 | 2.379985 | 0.017313 |
SPP1 | 1.313026 | 2.365654 | 0.017998 |
SERPINH1 | 2.181163 | 2.354909 | 0.018527 |
DENND2D | 0.332112 | −2.33765 | 0.019405 |
CTSL | 1.718827 | 2.319918 | 0.020345 |
LY96 | 1.904947 | 2.294088 | 0.021785 |
APBA2 | 1.929652 | 2.292849 | 0.021857 |
C1R | 1.625295 | 2.28929 | 0.022063 |
IFITM2 | 1.776594 | 2.277153 | 0.022777 |
HOXC8 | 2.075444 | 2.274953 | 0.022909 |
POPDC3 | 1.532657 | 2.259542 | 0.02385 |
MAGEA11 | 0.595434 | −2.25558 | 0.024097 |
APLN | 1.751567 | 2.236769 | 0.025301 |
STC2 | 1.705247 | 2.185285 | 0.028868 |
MIR4435-2HG | 2.654231 | 2.166308 | 0.030288 |
HAS2 | 1.608846 | 2.165967 | 0.030314 |
MNDA | 1.79297 | 2.119068 | 0.034085 |
PARVB | 2.112533 | 2.115255 | 0.034408 |
G0S2 | 1.502597 | 2.112957 | 0.034604 |
CSF3 | 0.579595 | −2.08523 | 0.037048 |
TWIST2 | 1.729333 | 2.084705 | 0.037096 |
TNFRSF11B | 0.301497 | −2.08378 | 0.03718 |
FAM225A | 538.3035 | 2.071588 | 0.038304 |
HOOK1 | 0.565932 | −2.07044 | 0.038411 |
TIMP1 | 1.623557 | 2.046971 | 0.040661 |
HSPD1P6 | 141.4708 | 2.03818 | 0.041532 |
FCGR1A | 2.772473 | 2.030437 | 0.042312 |
HK3 | 2.14885 | 1.9925 | 0.046316 |
ACAN | 4.27515 | 1.978141 | 0.047913 |
OSM | 2.206695 | 1.978062 | 0.047922 |
PDLIM7 | 1.62091 | 1.969755 | 0.048866 |
P values less than 0.01 were considered significant. ESCC, esophageal squamous cell carcinoma; HR, hazard ratio; z, the value of the hypothesis test statistic for the regression coefficients.
Table 2
Gene | Exp (coef) |
---|---|
MAGEA6 | 0.271936 |
MAGEA3 | 2.102895 |
LINC01524 | 2737999 |
CFAP53 | 0.080066 |
IGF2 | 2.014585 |
GNGT1 | 0.338051 |
FCGR3A | 0.372708 |
FCGR2A | 3.446703 |
ESCC, esophageal squamous cell carcinoma; Exp (coef), weighting factor for gene expression.
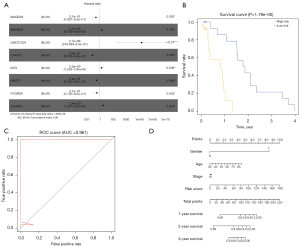
Identification of 8 prognostic factors for ESCC
We further performed Kaplan-Meier survival analysis for CFAP53 (Figure 5A), FCGR2A (Figure 5B), FCGR3A (Figure 5C), GNGT1 (Figure 5D), IGF2 (Figure 5E), LINC01524 (Figure 5F), MAGEA3 (Figure 5G), and MAGEA6 (Figure 5H). The results showed that ESCC patients with low CFAP53 (P=1.04e−02), GNGT1 (P=2.059e−02), MAGEA3 (P=1.144e−02), and MAGEA6 (P=3.648e−02) expression had a shorter OS time than those with high expression. Also, high FCGR2A (P=1.001e−01), FCGR3A (P=3.816e−02), IGF2 (P=1.211e−01), and LINC01524 (P=3.139e−02) expression indicated poorer OS compared to low expression.
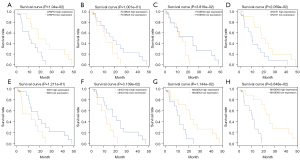
Construction of an mRNA-lncRNA co-expression network for ESCC
Based on the 8 prognostic signatures, a mRNA-lncRNA co-expression network was constructed for ESCC (Figure 6A). Co-expressed RNAs of the 8 prognostic RNAs were enriched in various biological processes and signaling pathways such as extracellular matrix organization, collagen formation, NABA matrisome associated, skeletal system development, and blood vessel development (Figure 6B). Pathway enrichment network diagram results revealed that the functional network of these RNAs was complex and diverse (Figure 6C).
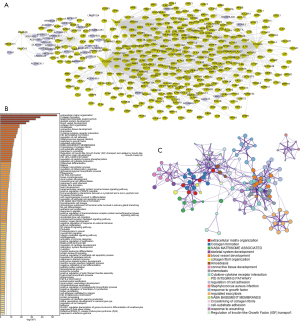
Discussion
As the main histological subtype of esophageal cancer, ESCC is a highly aggressive malignant tumor. A variety of environmental factors contribute to ESCC, such as smoking, drinking, and chemical exposure. Genomic studies have confirmed that changes in gene expression in ESCC mediate the biological behavior of tumor cells (17). Despite in-depth studies on its molecular mechanisms, the clinical outcomes of ESCC patients are still unsatisfactory. Thus, in this study, we constructed a robust prognostic nomogram based on the 8-gene signature, age, gender, and stage. This model exhibited good performance for prognostic prediction of ESCC. Hence, our study may provide novel clues for the early detection and treatment of ESCC.
WGCNA has been widely applied to explore ESCC-related modules. For instance, TPX2, CDK1, and CEP55 hub genes related to relapse-free survival have been identified in ESCC by WGCNA (18). In this study, we constructed 2 ESCC-related co-expression modules from 2 GEO datasets. Functional enrichment analysis results demonstrated that genes in the 2 co-expression modules were significantly involved in ESCC-related pathways such as mitochondrial gene expression (17), ncRNA metabolic process (19), and chromosome segregation (20), which confirmed the clinical significance of the 2 modules for ESCC. Based on univariate and multivariate Cox regression analyses, an 8-gene model was built for ESCC. TNM staging is the main tool used to guide therapeutic strategies for ESCC as a prognostic indicator. However, due to heterogeneity at the molecular level, the clinical outcome of patients is different. Our findings proposed that the 8-gene signature could accurately predict the prognosis of ESCC patients, the risk scores have the ability to discriminate high-risk patients, who have worse survival than low-risk patients. ROC confirmed its good performance for the prognostic prediction of ESCC. In a previous study, an immune-related nomogram was shown to provide more accurate prognostic prediction for patients with operable ESCC, as a supplement to TNM staging (21). In this study, by integrating the risk score and other factors, the nomogram could more accurately predict the OS of patients with ESCC.
Our survival analysis revealed that ESCC patients with low CFAP53, GNGT1, MAGEA3, and MAGEA6 expression had a shorter OS time than those with high expression. Moreover, high FCGR2A, FCGR3A, IGF2, and LINC01524 expression indicated poorer OS than low expression. Thus, these 8 genes are considered as potential prognostic markers for ESCC. In previous study, CFAP53 has been detected in the bronchial epithelium (22), and is highly expressed in the sputum of asthmatics. FCGR2A gene polymorphism is related to the prognosis and treatment response of a variety of cancer types. For example, the FCGR3A-158 gene polymorphism may predict the efficacy of trastuzumab for early ERBB2/HER2-positive breast cancer patients (23). What this study suggests to us is that 8 gene signatures in the prognostic models may be targets for therapy. The FCGR2A rs1801274 variant is associated with a high risk of gastric cancer in the Chinese population (24). MiR-139-3p is a candidate serum biomarker for predicting the prognosis of ESCC. Previous study showed that FCGR2A could be mediated by miR-139-3p at the post-transcriptional level (25). GNGT1 can predict the response to platinum-based chemotherapy drugs (26). IGF2 could maintain the stem cell characteristics of ESCC cells (27), and the prognostic potential of IGF2 in ESCC has been confirmed (28). IGF2 may promote ESCC cell migration and invasion (29). High expression of IGF2 can enhance the chemoresistance of ESCC (30). In comparison to mRNAs, lncRNAs possess higher tissue specificity, which is easier to detect (31). Thus, lncRNAs are also a key marker for ESCC diagnosis and prognosis. Only one study has demonstrated that LINC01524 is up-regulated in Helicobacter pylori-positive gastric cancer tissues compared to Helicobacter pylori-negative tissues (32). MAGEA3 is an independent prognostic factor for ESCC patients (33). Its expression is induced by decitabine, thereby enhancing the recognition of ESCC by T cells (34). The roles of the 8 genes in ESCC require in-depth exploration.
An mRNA-lncRNA co-expression network was built based on the 8 genes for ESCC. These co-expressed genes are involved in a variety of biological functions. For example, the extracellular matrix participates in the adhesion and metastasis of ESCC cells (35), which could be mediated by these co-expressed genes. Collagen is a component of the extracellular matrix, and is closely related to tumor growth as well as epithelial-mesenchymal transition (36). Blood vessel development as a key prognostic factor was distinctly enriched by these genes (37). Combining previous research, the 8 genes may participate in the progression of ESCC through complex interactions. However, there are still some limitations in this study. Firstly, the 8-gene signature should be verified in an independent dataset. Secondly, more clinical features should be integrated into our nomogram model. Thirdly, the specific functional mechanism of the 8-gene signature and 3 ESCC-related DEGs (DUXAP10, WDR72, and FST) (Figure 3A) in ESCC needs further study. Fourth, the relationship between risk levels and disease treatment response remains to be explored in treatment-group samples.
In this study, in the GSE23400 and GSE130078 datasets, WGCNA was carried out, and the co-expression gene modules related to ESCC were determined. Then, the genes in these modules were analyzed by Metascape, revealing that these genes might play important roles in ESCC. Combining the genes in these modules and DEGs, we identified 8 survival-related genes in TCGA database. The Cox regression model composed of these 8 genes demonstrated good performance in predicting prognosis. At the same time, the mRNA-lncRNA co-expression network was analyzed, indicating that these 8 genes exhibited complex interaction relationships. In summary, the 8 genes found by the analysis of multiple datasets can be used as ESCC biomarkers to provide certain theoretical support for ESCC research.
Conclusions
Taken together, WGCNA identified ESCC-related co-expression modules. A robust 8-gene signature could accurately predict the prognosis of ESCC patients. Furthermore, a prognostic nomogram based on risk score, age, gender, and stage was constructed for ESCC, which may be beneficial for early diagnosis and treatment. In future studies, the 8 genes will be verified in more clinical trials.
Acknowledgments
Funding: None.
Footnote
Reporting Checklist: The authors have completed the TRIPOD reporting checklist. Available at https://atm.amegroups.com/article/view/10.21037/atm-21-6935/rc
Conflicts of Interest: All authors have completed the ICMJE uniform disclosure form (available at https://atm.amegroups.com/article/view/10.21037/atm-21-6935/coif). The authors have no conflicts of interest to declare.
Ethical Statement: The authors are accountable for all aspects of the work in ensuring that questions related to the accuracy or integrity of any part of the work are appropriately investigated and resolved. The study was conducted in accordance with the Declaration of Helsinki (as revised in 2013).
Open Access Statement: This is an Open Access article distributed in accordance with the Creative Commons Attribution-NonCommercial-NoDerivs 4.0 International License (CC BY-NC-ND 4.0), which permits the non-commercial replication and distribution of the article with the strict proviso that no changes or edits are made and the original work is properly cited (including links to both the formal publication through the relevant DOI and the license). See: https://creativecommons.org/licenses/by-nc-nd/4.0/.
References
- Siegel RL, Miller KD, Jemal A. Cancer statistics, 2019. CA Cancer J Clin 2019;69:7-34. [Crossref] [PubMed]
- Hu X, Wu D, He X, et al. circGSK3β promotes metastasis in esophageal squamous cell carcinoma by augmenting β-catenin signaling. Mol Cancer 2019;18:160. [Crossref] [PubMed]
- Sánchez-Danés A, Blanpain C. Deciphering the cells of origin of squamous cell carcinomas. Nat Rev Cancer 2018;18:549-61. [Crossref] [PubMed]
- Reichenbach ZW, Murray MG, Saxena R, et al. Clinical and translational advances in esophageal squamous cell carcinoma. Adv Cancer Res 2019;144:95-135. [Crossref] [PubMed]
- Zhao Y, Zhu J, Shi B, et al. The transcription factor LEF1 promotes tumorigenicity and activates the TGF-β signaling pathway in esophageal squamous cell carcinoma. J Exp Clin Cancer Res 2019;38:304. [Crossref] [PubMed]
- Mao Y, Fu Z, Zhang Y, et al. A seven-lncRNA signature predicts overall survival in esophageal squamous cell carcinoma. Sci Rep 2018;8:8823. [Crossref] [PubMed]
- Du X, Xu Q, Pan D, et al. HIC-5 in cancer-associated fibroblasts contributes to esophageal squamous cell carcinoma progression. Cell Death Dis 2019;10:873. [Crossref] [PubMed]
- Ishibashi O, Akagi I, Ogawa Y, et al. MiR-141-3p is upregulated in esophageal squamous cell carcinoma and targets pleckstrin homology domain leucine-rich repeat protein phosphatase-2, a negative regulator of the PI3K/AKT pathway. Biochem Biophys Res Commun 2018;501:507-13. [Crossref] [PubMed]
- Shen L, Xia M, Deng X, et al. A lectin-based glycomic approach identifies FUT8 as a driver of radioresistance in oesophageal squamous cell carcinoma. Cell Oncol (Dordr) 2020;43:695-707. [Crossref] [PubMed]
- Tang X, Xu P, Wang B, et al. Identification of a Specific Gene Module for Predicting Prognosis in Glioblastoma Patients. Front Oncol 2019;9:812. [Crossref] [PubMed]
- Su H, Hu N, Yang HH, et al. Global gene expression profiling and validation in esophageal squamous cell carcinoma and its association with clinical phenotypes. Clin Cancer Res 2011;17:2955-66. [Crossref] [PubMed]
- You BH, Yoon JH, Kang H, et al. HERES, a lncRNA that regulates canonical and noncanonical Wnt signaling pathways via interaction with EZH2. Proc Natl Acad Sci U S A 2019;116:24620-9. [Crossref] [PubMed]
- Langfelder P, Horvath S. WGCNA: an R package for weighted correlation network analysis. BMC Bioinformatics 2008;9:559. [Crossref] [PubMed]
- Di Lena P, Sala C, Prodi A, et al. Missing value estimation methods for DNA methylation data. Bioinformatics 2019;35:3786-93. [Crossref] [PubMed]
- Zhou Y, Zhou B, Pache L, et al. Metascape provides a biologist-oriented resource for the analysis of systems-level datasets. Nat Commun 2019;10:1523. [Crossref] [PubMed]
- Doncheva NT, Morris JH, Gorodkin J, et al. Cytoscape StringApp: Network Analysis and Visualization of Proteomics Data. J Proteome Res 2019;18:623-32. [Crossref] [PubMed]
- Lin CS, Huang YY, Pan SC, et al. Involvement of increased p53 expression in the decrease of mitochondrial DNA copy number and increase of SUVmax of FDG-PET scan in esophageal squamous cell carcinoma. Mitochondrion 2019;47:54-63. [Crossref] [PubMed]
- Dong Z, Zhang H, Zhan T, et al. Integrated analysis of differentially expressed genes in esophageal squamous cell carcinoma using bioinformatics. Neoplasma 2018;65:523-31. [Crossref] [PubMed]
- Song Y, Li L, Ou Y, et al. Identification of genomic alterations in oesophageal squamous cell cancer. Nature 2014;509:91-5. [Crossref] [PubMed]
- Moghanibashi M, Rastgar Jazii F, Soheili ZS, et al. Esophageal cancer alters the expression of nuclear pore complex binding protein Hsc70 and eIF5A-1. Funct Integr Genomics 2013;13:253-60. [Crossref] [PubMed]
- Duan J, Xie Y, Qu L, et al. A nomogram-based immunoprofile predicts overall survival for previously untreated patients with esophageal squamous cell carcinoma after esophagectomy. J Immunother Cancer 2018;6:100. [Crossref] [PubMed]
- Qin L, Gibson PG, Simpson JL, et al. Dysregulation of sputum columnar epithelial cells and products in distinct asthma phenotypes. Clin Exp Allergy 2019;49:1418-28. [Crossref] [PubMed]
- Gavin PG, Song N, Kim SR, et al. Association of Polymorphisms in FCGR2A and FCGR3A With Degree of Trastuzumab Benefit in the Adjuvant Treatment of ERBB2/HER2-Positive Breast Cancer: Analysis of the NSABP B-31 Trial. JAMA Oncol 2017;3:335-41. [Crossref] [PubMed]
- Xia HZ, Du WD, Wu Q, et al. E-selectin rs5361 and FCGR2A rs1801274 variants were associated with increased risk of gastric cancer in a Chinese population. Mol Carcinog 2012;51:597-607. [Crossref] [PubMed]
- Wang Y, Fang Q, Tian L, et al. Expression and Regulatory Network Analysis of MiR-139-3p, a New Potential Serum Biomarker for Esophageal Squamous Cell Carcinoma Based on Bioinformatics Analysis. Technol Cancer Res Treat 2020;19:1533033820920967. [Crossref] [PubMed]
- Mucaki EJ, Zhao JZL, Lizotte DJ, et al. Predicting responses to platin chemotherapy agents with biochemically-inspired machine learning. Signal Transduct Target Ther 2019;4:1. [Crossref] [PubMed]
- Xu WW, Li B, Zhao JF, et al. IGF2 induces CD133 expression in esophageal cancer cells to promote cancer stemness. Cancer Lett 2018;425:88-100. [Crossref] [PubMed]
- Murata A, Baba Y, Watanabe M, et al. IGF2 DMR0 methylation, loss of imprinting, and patient prognosis in esophageal squamous cell carcinoma. Ann Surg Oncol 2014;21:1166-74. [Crossref] [PubMed]
- Zheng DN, Zhang CJ, Sun GP. Long non-coding RNA MNX1-AS1 promotes migration and invasion of esophageal squamous cell carcinoma by upregulating IGF2. Eur Rev Med Pharmacol Sci 2019;23:6179-85. [PubMed]
- Li B, Xu WW, Guan XY, et al. Competitive Binding Between Id1 and E2F1 to Cdc20 Regulates E2F1 Degradation and Thymidylate Synthase Expression to Promote Esophageal Cancer Chemoresistance. Clin Cancer Res 2016;22:1243-55. [Crossref] [PubMed]
- Yang B, Shen J, Xu L, et al. Genome-Wide Identification of a Novel Eight-lncRNA Signature to Improve Prognostic Prediction in Head and Neck Squamous Cell Carcinoma. Front Oncol 2019;9:898. [Crossref] [PubMed]
- Chu A, Liu J, Yuan Y, et al. Comprehensive Analysis of Aberrantly Expressed ceRNA network in gastric cancer with and without H.pylori infection. J Cancer 2019;10:853-63. [Crossref] [PubMed]
- Liu S, Chen H, Ge X, et al. MAGEA3 serves as an independent indicator for predicting the prognosis of ESCC. Panminerva Med 2021;63:382-3. [Crossref] [PubMed]
- Shi X, Chen X, Fang B, et al. Decitabine enhances tumor recognition by T cells through upregulating the MAGE-A3 expression in esophageal carcinoma. Biomed Pharmacother 2019;112:108632. [Crossref] [PubMed]
- Xiao J, Yang W, Xu B, et al. Expression of fibronectin in esophageal squamous cell carcinoma and its role in migration. BMC Cancer 2018;18:976. [Crossref] [PubMed]
- Li J, Wang X, Zheng K, et al. The clinical significance of collagen family gene expression in esophageal squamous cell carcinoma. PeerJ 2019;7:e7705. [Crossref] [PubMed]
- Tachezy M, Tiebel AK, Gebauer F, et al. Prognostic impact of perineural, blood and lymph vessel invasion for esophageal cancer. Histol Histopathol 2014;29:1467-75. [PubMed]
(English Language Editor: C. Betlazar-Maseh)