Analysis of cancer-promoting genes related to chemotherapy resistance in esophageal squamous cell carcinoma
Introduction
Esophageal cancer is one of the most common cancers worldwide, and with a 5-year survival rate less than 25%, is a leading cause of cancer-related deaths (1). Esophageal squamous cell carcinoma (ESCC), a malignancy of esophageal epithelial cells, accounts for around 90% of esophageal cancer cases (2). In clinical practice, surgery remains the best choice for esophageal cancer treatment (3). However, compared with surgery alone, chemotherapeutic approaches have demonstrated a survival advantage for patients undergoing either neoadjuvant chemotherapy or combined chemoradiotherapy treatment before surgery. Cisplatin and paclitaxel are two chemotherapeutic agents frequently used alone or in combination with surgery. Although the overall effect of chemotherapy is considerable and it has been widely used, many patients respond poorly to chemotherapy or eventually develop chemotherapy resistance. Chemoradiotherapy resistance (in particular, towards cisplatin and paclitaxel) and cancer relapse are among the most difficult issues in ESCC treatment (4,5). Thus, novel therapies developed with the aid of molecular biomarkers are urgently needed to prevent resistance of ESCC. Therefore, it is important to discover novel molecular biomarkers associated with chemotherapy resistance so as to predict the effectiveness of chemotherapy. There are some methods for screening tumor markers include radioimmunoassay, chemiluminescence immunoassay, enzyme-linked immunosorbent assay, immunosensor, proteomics, polymerase chain reaction (PCR), fluorescence in situ hybridization (FISH), single strand conformation polymorphism (SSCP), liquid biopsy and so on.
To discover useful and novel cancer-promoting genes related to chemotherapy resistance in ESCC, microRNA (miRNA) and messenger RNA (mRNA) expression patterns of the resistant ESCC strains can be probed. Theoretically, when a suspected miRNA and its potential targets reverse directions under chemotherapy stress, they are possibly involved in the process of resistance development. In other cancer types, miRNA expression has been shown to exert an impact on the response to chemotherapy (6-9), but few studies have reported on resistance in ESCC. Herein, we focus on the novel molecular biomarkers associated with chemotherapy resistance and the effectiveness of chemotherapy. Therefore, we aimed to seek associations between miRNA/mRNA expression and chemotherapy resistance in ESCC cell lines. In addition, we assessed the risk of potential oncogenes associated with chemotherapy resistance on survival in ESCC patients. Herein, we combined two datasets of the Gene Expression Omnibus (GEO) database and identified some novel and important cancer-promoting genes.
We present the following article in accordance with the STREGA reporting checklist (available at https://atm.amegroups.com/article/view/10.21037/atm-21-7032/rc).
Methods
Microarray data
We searched all gene expression data associated with chemotherapy resistance in ESCC using samples from the GEO database (http://www.ncbi.nlm.nih.gov/geo/). The inclusion criteria were as follows: (I) the samples were human ESCC cells or tissues; (II) mRNA or miRNA expression profiling was included; and (III) there were chemotherapy resistance samples as well as wild-type control.
There were two available arrays with chemotherapy-resistant ESCC samples: GSE86099 (gene expression signature of paclitaxel resistance in human ESCC cell lines) and GSE50224 (the expression profiling of miRNA from chemotherapy-resistant esophageal adenosquamous carcinoma cell line). The first dataset, GSE86099, used parental and paclitaxel resistant cell lines and studied mRNA transcription levels by microarray analysis to explore the key molecules for developing paclitaxel resistance. The second dataset, GSE50224, A global miRNA expression program for potential chemoresistance is described in detail, which identifies different classes of upregulated miRNAs in the generated chemoresistant cell lines. We only used the ESCC cell line (KYSE410) in this dataset. The study was conducted in accordance with the Declaration of Helsinki (as revised in 2013).
Differentially expressed genes (DEGs)
MRNA and miRNA expression profiles were analyzed for DEGs. Expression data were imported into the DEGseq package in R to compare expression differences between samples. The fold change and adjusted P value were obtained for each transcript. Based on the threshold of adjusted P<0.05 and fold change >2 or <0.5, the DEGs of each dataset were then acquired. If the screened results showed that the fold change was not significant, the fold change was widened to >1.5 or <0.6. Volcanic plots were produced for DEGs and differentially expressed (DE) transcripts using the ggplot2 package in R. In particular, we paid attention to DE mRNAs and miRNAs with changes in opposite directions.
Target prediction of the DE miRNAs and screening of the key oncogenes
The online tool miRWalk (http://mirwalk.umm.uni-heidelberg.de/) was used to predict the mRNAs potentially targeted by the DE miRNAs acquired from the GSE50224 dataset. The criterion of any target was the matching score =1. The intersection of all targets was obtained through a Venn diagram. Given all DE miRNAs were downregulated, we screened all the upregulated DE mRNAs from the GSE86099 dataset. The intersection of upregulated DE mRNAs and the targets of DE miRNAs represented the key oncogenes associated with chemotherapy resistance.
Correlation between drug sensitivity score and gene expression
The selected genes and their correlation with paclitaxel sensitivity were investigated. Drug response data [defined by the half-maximal inhibitory concentration (IC50) value] was downloaded from the Genomics of Drug Sensitivity in Cancer (GDSC) database. Gene expression was acquired from The Cancer Genome Atlas (TCGA) samples, and the sensitivity score was predicted using the pRRophetic package. We used ridge regression to estimate the IC50 of each sample based on risk and gene expression. Spearman correlation analysis of paclitaxel IC50 score and genes of interest was presented by a scatter plot for the 82 TCGA esophageal carcinoma (ESCA) samples.
Prognostic predictive signature
After screening the essential ESCC-associated genes involved in both chemotherapy resistance and survival, least absolute shrinkage and selection operator (LASSO) Cox regression was performed to identify relevant risk genes, using 10-fold cross-validation, and the prognostic predictive signature, using the R software package glmnet (v 4.1-1). Raw counts of RNA-sequencing data and corresponding clinical information were obtained from TCGA (https://portal.gdc.cancer.gov/). Kaplan-Meyer survival analysis and log-rank tests were also used to compare survival differences. The log-rank test and univariate Cox proportional risk regression were used to obtain P values with 95% confidence intervals and risk ratios.
Receiver operating characteristic (ROC) curve analysis was performed to compare the predictive accuracy of the risk score. All analytical methods and R packages are conducted using R software version 4.0.3 (R Statistical Computing Foundation, 2020). P<0.05 is considered statistically significant.
Gene Ontology (GO) enrichment analysis
We used the WEB-based Gene Set Analysis Toolkit to explore GO functional enrichment and Kyoto Encyclopedia of Genes and Genomes (KEGG) pathway enrichment of the key oncogenes. A directed acyclic graph (DAG) was then drawn based on the enriched GO terms. The overrepresentation analysis (ORA) enrichment method was used based on the H. sapiens organism. Among 35 unique Entrez Gene IDs, 34 were annotated to the selected functional categories and reference list, which were used for enrichment analysis. The Metascape gene-list analysis tool was used for analysis of enrichment in DisGeNET, which integrates information on human disease-associated genes, and the enriched transcription factor targets. Terms with a P<0.05, the minimum collection count 3 and enrichment factor (the ratio between observed count and accidental expected count) are greater than 1.5, and are grouped into clusters according to their membership similarity. P value is calculated based on cumulative hypergeometric distribution, and Q value is calculated by Benjamini-Hochberg program to illustrate multiple tests. When hierarchical clustering is performed on rich items, Kappa score is used as similarity measure, and subtrees with similarity >0.3 are considered as clusters. Select the most statistically significant term in a cluster to represent the cluster.
Protein-protein interaction (PPI) enrichment analysis
PPI enrichment analysis was carried out on the key oncogenes using the Search Tool for the Retrieval of Interacting Genes/Proteins (STRING) database (https://string-db.org/), and a PPI network was generated. Only physical interactions (physical score >0.4) in STRING were used.
The survival risk of key oncogenes in ESCC patients
We downloaded the survival data of ESCC patients along with the expression of key oncogenes from TCGA. There were 83 patients with clear gene expression data based on RNA sequencing (RNA-seq) and expressed as HTSeq-FPKM (Fragments Per Kilobase of transcript per Million mapped). The patients were divided into two groups for each oncogene (high expression and low expression) and the overall survival rate was analyzed through KM analysis by SPSS. The oncogenes with significant survival risk for ESCC patients were screened out to draw a KM survival curve.
Statistical analysis
Statistical analysis using R software (R: Language and Environment for Statistical Computing, R Core Team, R Foundation for Statistical Computing, Vienna, Austria, version 3.4.0). Examination time was compared by Student’s t-test after normality testing by Shapiro-Wilk test.
Results
ESCC-associated DEGs and chemotherapy-resistant DEGs
As shown in Figure 1A, there were 5,152 DE transcripts in the GSE86099 dataset associated with 2,942 DE mRNAs, including 2,675 upregulated and 267 downregulated genes (https://cdn.amegroups.cn/static/public/10.21037atm-21-7032-1.xlsx). When using a stricter screening criterion, there were 306 highly changed DEGs (Figure 1A). In the GSE50224 dataset, there were only 3 DE miRNAs (hsa-mir-18a-3p, hsa-mir-935, and hsa-mir-1226-3p) under the criteria of adjusted P<0.05 and fold change >2 or <0.5, and all were downregulated. Further, hsa-mir-99b-5p was also significantly decreased (adjusted P=0.0237, fold change =0.5899). Given there were very few screened DE miRNAs, we regarded the above 4 miRNAs as essential DE miRNAs, and their targets were collected. Theoretically, these upregulated mRNAs and the targets of downregulated miRNAs could eventually promote ESCC development.
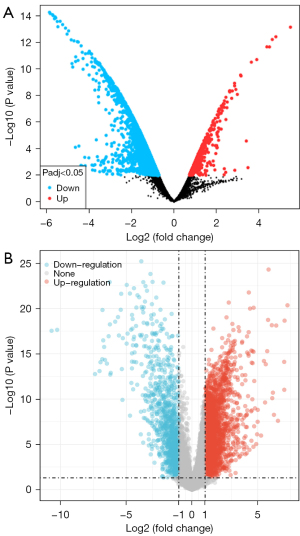
In addition, we used the TCGA-ESCA dataset to identify a total of 3,530 DEGs (2,404 upregulated and 1,126 downregulated) in the ESCA group versus normal control. The volcano plot of DEGs in TCGA-ESCA is shown in Figure 1B.
Upregulated targets of the DE miRNAs
Next, the targets of the above 4 miRNAs were acquired. In the miRWalk database, we found 4,143 targets of hsa-miR-935, 6,238 targets of hsa-miR-18a-3p, 6,041 targets of hsa-miR-1226-3p, and 1,960 targets of hsa-miR-99b-5p (Figure 2A). There were 173 common targets shared by the 4 miRNAs. The intersection of the two sets is presented in Figure 2B and include the miRNA targets of the 4 DE miRNAs in GSE50224 (all downregulated) and the 2,675 upregulated mRNAs in GSE86099. There were 35 common genes which could be important cancer-promoting genes related to chemotherapy resistance in ESCC. These 35 genes were: NEK1, KALRN, SCN4B, SATB1, IGF2, GJC1, SHANK2, EYA1, DOCK8, PTPRD, RBM23, CACNB2, GNB4, ZBED9, AKT2, NKAIN1, DLG2, GPR161, SCN1A, PDE4DIP, FAT2, ZBTB20, TCF4, STARD4, DCLK1, TIGIT, PPP1R9A, MRO, OSBPL1A, CIART, SLC39A14, CMKLR1, USH2A, HIF3A, and ZNF235.
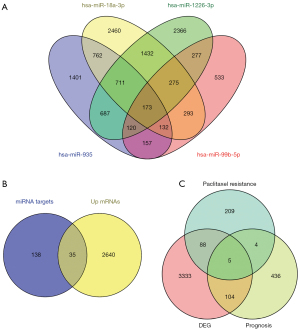
ESCC survival-associated genes and interaction analysis
There were 549 genes in the whole genome associated with the prognosis of ESCC, and together with the 306 essential genes associated with paclitaxel resistance and the 3,530 DEGs from TCGA, a Venn diagram was generated. The Venn diagram found 5 genes shared by the 3 sets: bone morphogenetic protein 1 (BMP1), dumbbell former 4 protein (DBF4), huntingtin-interacting protein 1 (HIP1), angiogenin (ANG), and MAP7 domain containing 2 (MAP7D2) (Figure 2C). These 5 genes were essential ESCC-associated genes involved in both chemotherapy resistance and survival.
Correlation between paclitaxel sensitivity prediction and 5 common genes
The estimated paclitaxel IC50 of the 82 TCGA-ESCA samples and the above 5 genes were paired for correlation analysis. Four genes showed significant association with the estimated IC50 of paclitaxel: BMP1, DBF4, ANG, and MAP7D2 (Figure 3A-3D).
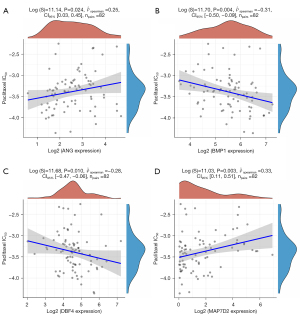
Prognostic performance of the signature
The prognostic performance of the risk-gene signature was showed (Figure 4A). Four risk factors (MP1, HIP1, ANG, and MAP7D2) were selected to construct a risk score using LASSO regression (Figure 4B), after dimensions were reduced (Figure 4C). Patients were divided into a high-risk group and low-risk group. KM survival analysis showed a significant difference in survival between the two groups (median survival period: 1.5 and 3.7 years, respectively; Figure 4D). This signature showed satisfactory performance in 1-, 2-, and 3-year survival prediction as shown by the area under the curve (AUC) of the ROC curve (Figure 4E). In particular, the AUC value for the 2-year survival evaluation was 0.856 (95% CI: 0.742−0.969).
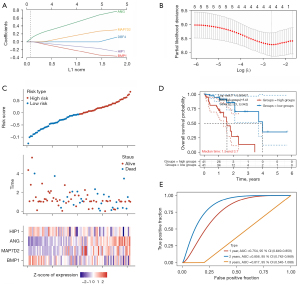
Enrichment analysis
Enrichment analysis was conducted based on the 35 cancer-promoting genes related to chemotherapy resistance as DE miRNA targets. Nine GO terms were significantly enriched, including AV node cell to bundle of His cell communication, cardiac muscle cell action potential involved in contraction, dendrite morphogenesis, cardiac muscle cell contraction, cardiac muscle cell action potential, positive regulation of dendrite development, regulation of membrane potential, post-synapse organization, and cell-cell signaling involved in cardiac conduction (Figure 5).
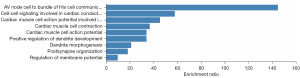
PPI network
A PPI network was generated using the STRING database. As shown in Figure 6, guanine nucleotide-binding protein subunit beta 4 (GNB4), calcium voltage-gated channel auxiliary subunit beta 2 (CACNB2), and sodium voltage-gated channel alpha subunit 1 (SCN1A) were located at key positions of the network. PPI analysis showed GNB4, CACNB2, and SCN1A were located at key positions of the network. Among potential risk genes, only the high expression of dedicator of cytokinesis 8 (DOCK8) was associated with poorer survival. GNB4, CACNB2, SCN1A and DOCK8 have different effects on the formation of tumor chemotherapy resistance microenvironment. These nodes merit more attention in future research of ESCC progression and treatment.
Survival risk of DOCK8 in ESCC patients
Finally, we analyzed the influence of 35 key oncogenes on survival using TCGA data of 82 ESCC cases (https://cdn.amegroups.cn/static/public/10.21037atm-21-7032-2.xlsx). Among these potentially risk genes, we found only DOCK8 had a correlation with overall survival, and high expression of DOCK8 also implied poorer survival time (Figure 7). The median survival of the low-DOCK8 group was 1,361 days (95% CI: 754–1,968 days), while that of the high-DOCK8 group was 650 days (95% CI: 499–801 days) (logrank P=0.02). There were no correlations between the 4 miRNAs and survival in ESCC patients. Taken together, DOCK8 should be considered a preferred gene for detecting the underlying mechanisms of chemotherapy resistance. Among 35 key oncogenes, only 1 factor (DOCK8) was found to be a survival risk gene, implying that the influence and deep network of oncogenes under chemotherapy stress is more complicated.
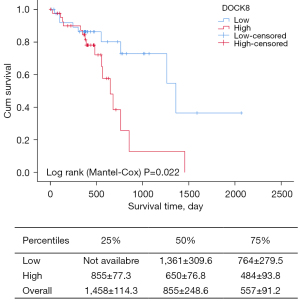
Discussion
The mRNAs and miRNAs associated with ESCC development have been widely studied through high-throughput approaches, and known ESCC-related miRNAs include miR-196a-5p, miR-1-3p, miRNA-134, miR-135b-5p, miR-15b-5p, miR-195-5p, miR-124, miRNA-1290, miRNA-20b-5p, miR-885-5p, miR-140-3p, miR-708, miR-639, miR-596, miRNA-506, and miR-338-5p (10-16). In addition, hundreds of ESCC-driving mRNAs have been reported. However, the key genes associated with chemotherapy-resistance in ESCC are not fully understood. Several published works have demonstrated that this resistance could be affected by both mRNAs, including taurine upregulated 1 (TUG1), zinc finger protein x-linked (ZFX), and nuclear factor-erythroid factor 2-related factor 2 (Nrf2), and miRNAs, such as miR-130a-3p, miR-148a-3p, miR-125a-5p, and miR-224 (17-21). Herein, we discovered that hsa-miR-18a-3p, hsa-miR-935, hsa-miR-1226-3p, and hsa-miR-99b-5p were downregulated in resistant ESCC cells, and for the first time, that some novel targets, including DOCK8, GNB4, CACNB2, and SCN1A, were possibly highly involved in ESCC chemotherapy resistance. To the best of our knowledge, these results, particularly of the key mRNAs, have not been reported in previous ESCC studies. Although there appear to be discrepancies between our results and the original article of some data (e.g., GSE50224), this was mainly due to the use of different grouping methods. For example, we combined all chemotherapy-resistant esophageal cancer cells into the resistant group. SCN1A is a neuronal sodium-channel gene and is associated with different nervous-system related disorders, as shown in the enrichment results in DisGeNET (22,23). Currently, this is the first study reporting that SCN1A may be an important ESCC driver.
In prognosis analysis using the 4-risk factor signature (BMP1, HIP1, ANG, and MAP7D2), we observed a good performance, especially in 2-year survival prediction. BMP1 is capable of inducing formation of cartilage in vivo and is associated with osteogenesis imperfecta and high bone mass osteogenesis imperfecta. Among its related pathways are activation of cyclic adenosine monophosphate (cAMP)-dependent protein kinase A (PKA) and adipogenesis. A previous study on the immune genes associated with prognosis in esophageal carcinoma also reported that BMP1 was a risk gene in esophageal carcinoma (24). HIP1 is a membrane-associated protein that functions in clathrin-mediated endocytosis and protein trafficking within the cell. Recently, it was reported that abnormally high HIP1 expression was associated with metastatic behaviors and poor prognosis in ESCC (25). ANG is a member of the ribonuclease (RNase) A superfamily and is associated with amyotrophic lateral sclerosis and amyotrophic lateral sclerosis. Among its related pathways are cell junction organization and pathways of neurodegeneration. One study reported, consistent with our results, that all-trans retinoic acid (ATRA) inhibited angiogenesis and metastasis of ESCC by suppressing the ANG-Tie2 pathway (26). MAP7D2 is a relatively novel risk gene in carcinoma. An integrated bioinformatics analysis showed that MAP7D2 was a prognostic biomarker of gastric cancer (27), and it may contribute to the progression of ESCC through a similar mechanism.
DOCK8 is a member of the DOCK family of guanine nucleotide exchange factors, which interact with Rho GTPases and are components of intracellular signaling networks. In patients with human papillomavirus (HPV)-positive head and neck squamous cell carcinoma, DOCK8 can serve as a prognostic biomarker and is related to immune infiltration (28). In 2008, a Japanese study reported that DOCK8 was an upregulated gene involved in radiosensitivity of ESCC (29). The specific mechanism of DOCK8 towards the stress of chemotherapeutic drugs is largely unknown. Notably, mutations of DOCK8 are associated with immunodeficiency (30,31). Expression levels of DOCK8 were reduced in 87% of primary lung cancers in comparison with normal lung tissue (32). These results appeared to be the exact opposite of our findings. In hepatocellular carcinoma, DOCK8 is a downstream member of signal transducer and activator of transcription 3 (STAT3) and can play a role as a guanine-nucleotide exchanging factor for Rac Family Small GTPase 1 (RAC1), which drives mesenchymal-type movement (33). Moreover, DOCK family proteins are involved in the regulation of migration, morphology, adhesion, and cell growth (32), suggesting that they may enhance the migration ability of ESCC cells under the stress of chemotherapy. Given the increased expression of DOCK8 in resistant ESCC cells and its association with poorer survival, we believe that its molecular action mechanism in ESCC merits urgent further exploration. GNB4 is also a hub gene in ovarian cancer and Helicobacter pylori-induced gastric cancer (34,35), but its detailed mechanism in tumor development is largely unknown. The association between CACNB2 and squamous cell carcinoma (SCC) was reported by another miRNA study regarding head and neck SCC (36), which found that the tumor suppressor miR-31-5p targeted CACNB2 and affected prognosis.
The authors of dataset GSE50224 published 2 similar articles regarding the 4 downregulated miRNAs, with consistent results (37,38), and another study reported that miR-1226-3p could sensitize ESCC towards cisplatin in 50% of cell lines (19). However, other supporting evidence is limited. Therefore, miR-18a-3p, hsa-miR-935, and hsa-miR-99b-5p in ESCC is worthy of more attention.
Some upregulated mRNAs seemed contradictory to previous studies. For example, NK6 Homeobox 1 (NKX61) has been reported as a suppressor of metastasis in colorectal cancer (39). This inconsistency can be explained by the fact that the role of NKX61 in ESCC and colorectal cancer may differ. Further, we cannot confirm that the upregulated targets were a driver of chemotherapy resistance as this may have been a concomitant phenomenon, albeit with a high probability of being a driving factor.
Radiotherapy combined with cisplatin and taxol chemotherapy can improve the immune function of patients with esophageal cancer. In the training and validation cohort, the down-regulation of NS1-BP is related to chemoradiotherapy resistance and short disease-specific survival (DSS). Both synchronous radiotherapy and pure radiotherapy are appropriate. Improving the immune function of patients can promote progress, increase T lymphocyte, inhibitory T-cells, helper T cells and Th/Ts levels of patients, lower the Ts level and lower the tumor index. The level of SCC-Ag and CYFRA21-1 should be kept at the same time, and chemotherapy should be performed simultaneously.
This study had some limitations. First, of the 35 key oncogenes, only 1 factor (DOCK8) was found to be a survival risk gene, implying that the influence and deep network of oncogenes under chemotherapy stress is more complicated. In addition, we did not perform verification of the expression of DE mRNA and miRNA, the protein expression of the corresponding mRNAs, or their matched targeting relationships. We have hardly analyzed lncRNA and ceRNA network which will be carried out in the next research work. The functions of the key genes have not been verified by experiments. This step is exceedingly necessary for deeper exploration. Finally, the number of ESCC cases in TCGA was limited, and the phenotype dimension was not sufficient. Therefore, patients with different stages and treatments were pooled together for analysis. This may have led to the negative results of most candidate oncogenes.
Conclusions
In conclusion, we combined miRNA and mRNA changes and found 35 key oncogenes involved in chemotherapy resistance in ESCC, as well as the corresponding enrichment and regulatory network. The novel candidates in this study merit further investigation and may provide a deeper understanding of the molecular mechanisms in ESCC treatment failure.
Acknowledgments
Funding: This study was sponsored by Clinical Medicine Plus Technology Project from Shanghai Jiaotong University School of Medicine and Shanghai Key Laboratory of Nucleic Acid Chemistry & Nanomedicine (No. 2021ZYA008).
Footnote
Reporting Checklist: The authors have completed the STREGA reporting checklist. Available at https://atm.amegroups.com/article/view/10.21037/atm-21-7032/rc
Conflicts of Interest: All authors have completed the ICMJE uniform disclosure form (available at https://atm.amegroups.com/article/view/10.21037/atm-21-7032/coif). The authors have no conflicts of interest to declare.
Ethical Statement: The authors are accountable for all aspects of the work in ensuring that questions related to the accuracy or integrity of any part of the work are appropriately investigated and resolved. The study was conducted in accordance with the Declaration of Helsinki (as revised in 2013).
Open Access Statement: This is an Open Access article distributed in accordance with the Creative Commons Attribution-NonCommercial-NoDerivs 4.0 International License (CC BY-NC-ND 4.0), which permits the non-commercial replication and distribution of the article with the strict proviso that no changes or edits are made and the original work is properly cited (including links to both the formal publication through the relevant DOI and the license). See: https://creativecommons.org/licenses/by-nc-nd/4.0/.
References
- Then EO, Lopez M, Saleem S, et al. Esophageal Cancer: An Updated Surveillance Epidemiology and End Results Database Analysis. World J Oncol 2020;11:55-64. [Crossref] [PubMed]
- Zeng RJ, Zheng CW, Gu JE, et al. RAC1 inhibition reverses cisplatin resistance in esophageal squamous cell carcinoma and induces downregulation of glycolytic enzymes. Mol Oncol 2019;13:2010-30. [Crossref] [PubMed]
- Allum WH, Bonavina L, Cassivi SD, et al. Surgical treatments for esophageal cancers. Ann N Y Acad Sci 2014;1325:242-68. [Crossref] [PubMed]
- Zhou C, Zhang L, Xu P. Growth inhibition and chemo-radiosensitization of esophageal squamous cell carcinoma by survivin-shRNA lentivirus transfection. Oncol Lett 2018;16:4813-20. [Crossref] [PubMed]
- Ou Y, Ma L, Ma L, et al. Overexpression of cyclin B1 antagonizes chemotherapeutic-induced apoptosis through PTEN/Akt pathway in human esophageal squamous cell carcinoma cells. Cancer Biol Ther 2013;14:45-55. [Crossref] [PubMed]
- To KK, Tong CW, Wu M, et al. MicroRNAs in the prognosis and therapy of colorectal cancer: From bench to bedside. World J Gastroenterol 2018;24:2949-73. [Crossref] [PubMed]
- Feng Y, Zhong M, Zeng S, et al. Exosome-derived miRNAs as predictive biomarkers for diffuse large B-cell lymphoma chemotherapy resistance. Epigenomics 2019;11:35-51. [Crossref] [PubMed]
- Jayaraj R, Nayagam SG, Kar A, et al. Clinical Theragnostic Relationship between Drug-Resistance Specific miRNA Expressions, Chemotherapeutic Resistance, and Sensitivity in Breast Cancer: A Systematic Review and Meta-Analysis. Cells 2019;8:1250. [Crossref] [PubMed]
- Shen M, Dong C, Ruan X, et al. Chemotherapy-Induced Extracellular Vesicle miRNAs Promote Breast Cancer Stemness by Targeting ONECUT2. Cancer Res 2019;79:3608-21. [Crossref] [PubMed]
- Sang C, Chao C, Wang M, et al. Identification and validation of hub microRNAs dysregulated in esophageal squamous cell carcinoma. Aging (Albany NY) 2020;12:9807-24. [Crossref] [PubMed]
- Li CY, Zhang WW, Xiang JL, et al. Identification of microRNAs as novel biomarkers for esophageal squamous cell carcinoma: a study based on The Cancer Genome Atlas (TCGA) and bioinformatics. Chin Med J (Engl) 2019;132:2213-22. [Crossref] [PubMed]
- Yang H, Su H, Hu N, et al. Integrated analysis of genome-wide miRNAs and targeted gene expression in esophageal squamous cell carcinoma (ESCC) and relation to prognosis. BMC Cancer 2020;20:388. [Crossref] [PubMed]
- Sun H, Wang L, Zhao Q, et al. Diagnostic and prognostic value of serum miRNA-1290 in human esophageal squamous cell carcinoma. Cancer Biomark 2019;25:381-7. [Crossref] [PubMed]
- Yu J, Chen S, Niu Y, et al. Functional Significance and Therapeutic Potential of miRNA-20b-5p in Esophageal Squamous Cell Carcinoma. Mol Ther Nucleic Acids 2020;21:315-31. [Crossref] [PubMed]
- Li SP, Su HX, Zhao D, et al. Plasma miRNA-506 as a Prognostic Biomarker for Esophageal Squamous Cell Carcinoma. Med Sci Monit 2016;22:2195-201. [Crossref] [PubMed]
- Cui D, Zhu Y, Yan D, et al. Dual inhibition of cMET and EGFR by microRNA-338-5p suppresses metastasis of esophageal squamous cell carcinoma. Carcinogenesis 2021;42:995-1007. [Crossref] [PubMed]
- Jiang L, Wang W, Li G, et al. High TUG1 expression is associated with chemotherapy resistance and poor prognosis in esophageal squamous cell carcinoma. Cancer Chemother Pharmacol 2016;78:333-9. [Crossref] [PubMed]
- Wu J, Zhou Y, Wang T, et al. ZFX promotes tumorigenesis and confers chemotherapy resistance in esophageal squamous cell carcinoma. Clin Res Hepatol Gastroenterol 2021;45:101586. [Crossref] [PubMed]
- Lindner K, Eichelmann AK, Matuszcak C, et al. Complex Epigenetic Regulation of Chemotherapy Resistance and Biohlogy in Esophageal Squamous Cell Carcinoma via MicroRNAs. Int J Mol Sci 2018;19:499. [Crossref] [PubMed]
- Chang ZW, Jia YX, Zhang WJ, et al. LncRNA-TUSC7/miR-224 affected chemotherapy resistance of esophageal squamous cell carcinoma by competitively regulating DESC1. J Exp Clin Cancer Res 2018;37:56. [Crossref] [PubMed]
- Zhang Z, Xiong R, Li C, et al. LncRNA TUG1 promotes cisplatin resistance in esophageal squamous cell carcinoma cells by regulating Nrf2. Acta Biochim Biophys Sin (Shanghai) 2019;51:826-33. [Crossref] [PubMed]
- Weale ME, Depondt C, Macdonald SJ, et al. Selection and evaluation of tagging SNPs in the neuronal-sodium-channel gene SCN1A: implications for linkage-disequilibrium gene mapping. Am J Hum Genet 2003;73:551-65. [Crossref] [PubMed]
- Das A, Zhu B, Xie Y, et al. Interneuron Dysfunction in a New Mouse Model of SCN1A GEFS. eNeuro 2021;8. ENEURO. [Crossref] [PubMed]
- Fei Z, Xie R, Chen Z, et al. Establishment of a Novel Risk Score System of Immune Genes Associated With Prognosis in Esophageal Carcinoma. Front Oncol 2021;11:625271. [Crossref] [PubMed]
- Sun Y, Zhou Y, Xia J, et al. Abnormally high HIP1 expression is associated with metastatic behaviors and poor prognosis in ESCC. Oncol Lett 2021;21:79. [Crossref] [PubMed]
- Li N, Lu Y, Li D, et al. All-trans retinoic acid suppresses the angiopoietin-Tie2 pathway and inhibits angiogenesis and metastasis in esophageal squamous cell carcinoma. PLoS One 2017;12:e0174555. [Crossref] [PubMed]
- Liu X, Wu J, Zhang D, et al. Identification of Potential Key Genes Associated With the Pathogenesis and Prognosis of Gastric Cancer Based on Integrated Bioinformatics Analysis. Front Genet 2018;9:265. [Crossref] [PubMed]
- Zhang Z, Bao Y, Zhou L, et al. DOCK8 Serves as a Prognostic Biomarker and Is Related to Immune Infiltration in Patients With HPV Positive Head and Neck Squamous Cell Carcinoma. Cancer Control 2021;28:10732748211011951. [Crossref] [PubMed]
- Ogawa R, Ishiguro H, Kuwabara Y, et al. Identification of candidate genes involved in the radiosensitivity of esophageal cancer cells by microarray analysis. Dis Esophagus 2008;21:288-97. [Crossref] [PubMed]
- Dimitrova D, Freeman AF. Current Status of Dedicator of Cytokinesis-Associated Immunodeficiency: DOCK8 and DOCK2. Dermatol Clin 2017;35:11-9. [Crossref] [PubMed]
- Zhang Q, Davis JC, Lamborn IT, et al. Combined immunodeficiency associated with DOCK8 mutations. N Engl J Med 2009;361:2046-55. [Crossref] [PubMed]
- Takahashi K, Kohno T, Ajima R, et al. Homozygous deletion and reduced expression of the DOCK8 gene in human lung cancer. Int J Oncol 2006;28:321-8. [Crossref] [PubMed]
- Wang SJ, Cui HY, Liu YM, et al. CD147 promotes Src-dependent activation of Rac1 signaling through STAT3/DOCK8 during the motility of hepatocellular carcinoma cells. Oncotarget 2015;6:243-57. [Crossref] [PubMed]
- Liu D, Ma X, Yang F, et al. Discovery and validation of methylated-differentially expressed genes in Helicobacter pylori-induced gastric cancer. Cancer Gene Ther 2020;27:473-85. [Crossref] [PubMed]
- Zhang J, Huang S, Quan L, et al. Determination of Potential Therapeutic Targets and Prognostic Markers of Ovarian Cancer by Bioinformatics Analysis. Biomed Res Int 2021;2021:8883800. [Crossref] [PubMed]
- Oshima S, Asai S, Seki N, et al. Identification of Tumor Suppressive Genes Regulated by miR-31-5p and miR-31-3p in Head and Neck Squamous Cell Carcinoma. Int J Mol Sci 2021;22:6199. [Crossref] [PubMed]
- Hummel R, Wang T, Watson DI, et al. Chemotherapy-induced modification of microRNA expression in esophageal cancer. Oncol Rep 2011;26:1011-7. [Crossref] [PubMed]
- Hummel R, Sie C, Watson DI, et al. MicroRNA signatures in chemotherapy resistant esophageal cancer cell lines. World J Gastroenterol 2014;20:14904-12. [Crossref] [PubMed]
- Chung HH, Lee CT, Hu JM, et al. NKX6.1 represses tumorigenesis, metastasis, and chemoresistance in colorectal cancer. Int J Mol Sci 2020;21:5106. [Crossref] [PubMed]
(English Language Editor: A. Muijlwijk)