Molecular mechanism and candidate biomarkers of morphine for analgesia and addiction effects
Introduction
Morphine is an opioid drug, originally extracted and purified from opium poppy (1). Morphine is also the main psychoactive chemical substance in opium. With the deepening understanding of the structure of morphine, researchers began to synthesize morphine hydrochloride solution with higher purity and more convenient preparation and use (2). Because of its good sedative effect, it is widely used to relieve pain, cough, and diarrhea in patients undergoing surgery and those with advanced cancer (3). However, with the inappropriate use of morphine, its side effects are also becoming apparent. For example, excessive dosage of morphine may lead to hypotension, bradycardia, and respiratory failure (4). Patients with advanced cancer require increased frequency and dose of medication to achieve pain relief (4). Long-term irregular use of morphine can result in serious dependence, which can lead to addiction (5). After entering the body, morphine first binds to opioid receptors and triggers a cascade of downstream reactions through signal transduction, thus exerting its effect (6). The opioid receptor is a G protein-coupled receptor and therefore has a basic structure consisting of an external amino terminus region, 7 transmembrane domains in the middle, and an internal carboxy-terminus region (7).
Neuropathic pain afflicts many people worldwide. It can be caused by nerve damage, by diseases that affect peripheral nerve function, or as a manifestation of diseases such as cancer (8). Today, with some exceptions, clinical treatment for patients with acute severe pain or advanced cancer relies on the oral opioid morphine and its substitutes (9). Although morphine has high analgesic activity, it is also accompanied by certain side effects, including poor stability of enzymatic hydrolysis, difficulty in crossing the blood-brain barrier, incurable analgesic effect, and lack of oral activity (10). Morphine also has certain effects on the endocrine system of animals, including lowering the testosterone concentration and affecting the production of gonadotropin (11). In addition to the above effects, morphine also has a certain regulatory effect on the cardiovascular system (12). Exogenous opioid peptides can inhibit the activity of cultured osteoblasts, and some opioid peptides and their modified analogues can have indirect effects on bone metabolism (13). However, the molecular mechanism of morphine analgesia still needs to be explored.
The mechanism of drug addiction involves various complex neural adaptive changes. Under the stimulus of addictive drugs, the brain’s reward system is triggered, involving a variety of neurotransmitters and receptors (14). Drug addiction can be considered a disease of learning and memory, and many experiments have confirmed that synaptic plasticity is the neurological basis of learning and memory (15). At present, many studies have been conducted to explore the molecular mechanism of morphine addiction (16). Dopamine is a monoamine neurotransmitter that plays an important role in the communication between neurons (17). A growing number of studies have found that dopamine receptors are involved in the regulation of drug addiction (18). In addition, dopamine receptors also play an important regulatory role in morphine addiction (19). Glutamate is a major excitatory neurotransmitter in the brain, regulating up to 70% of synaptic neurotransmission in the central nervous system (20). Drug-induced synaptic plasticity is associated with adaptive changes in the limbic midbrain circuits resulting from chronic drug exposure, ultimately leading to addictive behavior, and glutamate receptors are involved in this process (21). Long-term abuse of morphine can lead immune dysfunction, an increase in the susceptibility of the body to infection including viruses, liver injury, and the susceptibility of offspring (22). However, the key genes for the addictive effects of morphine remain unknown.
The aim of this study is to identify the genetic mechanisms of the analgesic effects and addictive effects of morphine. Using transcriptional expression datasets, the mRNA-miRNA network and protein-protein interaction (PPI) network were constructed. Several differentially expressed miRNAs and mRNAs were obtained which showed high correlations with the phenotypes. Our results may highlight new causal candidate genes or miRNAs which underlie both the addictive and analgesic effects of morphine. We present the following article in accordance with the STREGA reporting checklist (available at https://atm.amegroups.com/article/view/10.21037/atm-21-7037/rc).
Methods
Data sources
The Gene Expression Omnibus (GEO, http://www.ncbi.nlm.nih.gov/geo/) database was used to download the expression matrix associated with morphine. The study was conducted in accordance with the Declaration of Helsinki (as revised in 2013). The GSE62346 dataset contained 9 miRNA samples and 15 mRNA samples which were used to explore the analgesic effect of morphine. Among the 9 miRNA samples, 3 samples belonged to control groups with 0 mg/kg morphine treatment. The other 6 samples were treated with 2 mg/kg morphine and 5 mg/kg morphine. The 15 mRNA samples were also treated with 0, 2, and 5 mg/kg morphine. The other mRNA dataset for the analgesic effect of morphine was GSE50382. This dataset contained 2 groups. The control group contained 3 samples with saline treatment while the analgesic group received morphine treatment.
Three mRNA datasets for studying the addictive effects of morphine were downloaded, namely GSE9525, GSE7762, and GSE78280. The GSE9525 dataset contained 8 hypothalamus samples and 10 pituitary samples. The GSE7762 dataset contained 12 control samples treated with saline and 12 samples treated with chronic morphine. The GSE78280 dataset contained 6 samples with saline treatment and 6 samples with protracted morphine treatment. GSE15774 was selected from an mRNA time series dataset for morphine addiction studies.
Data pre-processing
The mRNA and miRNA combined dataset GSE62346 for analgesia was extracted from the Series Matrix File. The probe ID was converted into gene symbol or miRNA ID through the platform annotation information table. The same gene symbol or miRNA ID was incorporated. Expression matrixes of mRNA and miRNA samples were screened out according to ID. Other datasets were preprocessed in the same way.
Correlation and principal component analysis
Principal component analysis (PCA) is an unsupervised feature learning data dimensionality reduction algorithm, which shows the classification of data through the expression data of samples. The R packages ggplot2 (23), plyr (24), ggbiplot (25), and scales (26) were used to perform PCA based on the genes with significant mean differences (ANOVA, P≤0.05) in the sample.
The correlation of gene expression levels between samples is an important reference index for experimental reliability and reasonable selection of samples. The correlation coefficient can represent the similarity between samples, and the closer the correlation coefficient is to 1, the higher the similarity between 2 samples. The R packages hmisc (27) and corrplot (28) were used to perform correlation analysis between 2 samples.
Differential expression analysis
The R package limma (29) was used to perform differential expression analysis of genes in the mRNA and miRNA datasets. Differentially expressed genes (DEGs) were defined by P value <0.05, fold change >1.2, or fold change <1/1.2. Datasets for morphine addiction were also analyzed by the R package limma. The screening criteria for differential genes were fold change >1.5, fold change <1, and P value <0.05.
Prediction of the miRNA-mRNA regulatory relationship
TargetScan, miRTarBase, miRDB, miRanda, and miRMap databases were used to predict the regulatory relationships between up-regulated miRNAs and down-regulated mRNAs, and between down-regulated miRNAs and up-regulated mRNAs.
PPI network prediction
The DEGs in the miRNA-mRNA regulatory network were extracted and input into the STRING database (https://string-db.org/) for protein-protein interaction (PPI) network prediction. Interaction score >0.7 (highest confidence) was selected as the threshold of PPI. After the PPI relation pairs were obtained, Cytoscape (30) software was used to analyze the topology structure of the PPI relation network.
Functional enrichment analysis
Based on the Gene Ontology (GO) and Kyoto Encyclopedia of Genes and Genomes (KEGG) pathway databases, the functions of the genes in the miRNA-mRNA networks were analyzed. Fisher’s exact test was used to calculate the correlations between DEGs and functional terms. On the other hand, Gene Set Enrichment Analysis (GSEA) were performed using the R package clusterProfiler. P values were used to evaluate significance, and smaller P values represented a more significant relationship.
Analysis of gene expression trends
The expression trends of the expression values at 1, 2, 4, and 8 h in the mRNA time series dataset used for addiction research were clustered, and those with similar expression patterns were grouped into 1 group. The genes in the classes whose overall expression trend was increasing or decreasing were selected, and the genes in the addiction-related pathways in the functional enrichment analysis of DEGs in the mRNA dataset used for addiction research were intersected.
Statistical analysis
Student’s t-test was used for assessing differences between groups. P value less than 0.05 was considered to be statistically significant. R software (version: 4.0.2) was used to perform all statistical analyses.
Results
PCA and differential expression analysis
To verify the gene expression differences between groups, PCA was performed based on the gene expression matrixes. As shown in Figure 1, gene expression was significantly different between each group in the 8 datasets.
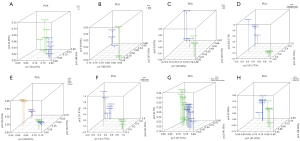
Using the R package limma, DEGs were obtained. The mRNA data of the GSE62346 dataset used to study analgesia contained 440 DEGs, among which 163 were up-regulated and 277 were down-regulated (Table 1). This dataset also contained 23 differentially expressed miRNAs which contained 12 up-regulated miRNAs and 11 down-regulated miRNAs. Using the same method, a total of 192 DEGs were obtained from the validation dataset GSE50382, containing 79 up-regulated genes and 192 down-regulated genes. Then, the DEGs of the 2 datasets were combined and 627 DEGs were finally obtained for further analysis. Four mRNA datasets were downloaded and analyzed to study morphine addiction. Dataset GSE9525 contained 796 up-regulated mRNAs and 993 down-regulated mRNAs among the 1,789 DEGs. Another 3 datasets contained 1,738, 136, and 27 DEGs. A total of 1,482 up-regulated and 1,754 down-regulated DEGs were obtained by combining the 4 datasets. In addition, heatmaps were generated based on the DEGs among the 6 datasets. As shown in Figure 2, the DEGs of different groups could be well clustered together.
Table 1
Type | Analgesia | Addiction | ||||||
---|---|---|---|---|---|---|---|---|
GSE62346 (mRNA) | GSE62346 (miRNA) | GSE50382 | GSE9525 | GSE9525 | GSE7762 | GSE78280 | ||
Up-regulated | 163 | 12 | 79 | 796 | 805 | 45 | 16 | |
Down-regulated | 277 | 11 | 113 | 993 | 933 | 91 | 11 | |
Total | 440 | 23 | 192 | 1,789 | 1,738 | 136 | 27 |
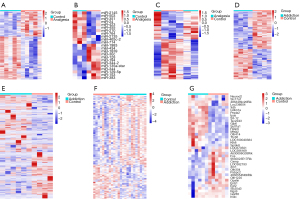
The common DEGs for the analgesic and addictive effects of morphine
Based on the 1,482 up-regulated and 1,754 down-regulated mRNAs for addictive effects and the 230 up-regulated and 367 down-regulated mRNAs for analgesic effects, the intersection which contained 8 common up-regulated mRNAs and 22 common down-regulated mRNAs was obtained (Figure 3). The 8 common up-regulated genes were Aloxe3, Chrm2, Epha8, Folh1, Has1, Hes3, Palm2, and Rgs17. The 22 common down-regulated genes were 1700028P14Rik, 1700047G07Rik, Antxr2, Celsr3, Col8a1, Crhr2, Ctf1, Cxcr4, Glb1l, Ins2, Lass4, Lect1, Lrrc17, Mfap4, Myo5a, Plxna3, Postn, Prrx2, Six5, Slc28a3, Tcn2, and Thbs1.
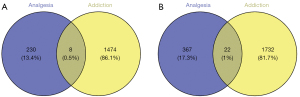
MiRNA-mRNA regulatory networks
Combining the differently expressed mRNAs and miRNAs, an mRNA-miRNA regulatory network was generated (Figure 4). The network contained 101 regulatory relation pairs, among which 54 up-regulated miRNA and 47 down-regulated miRNA regulatory relation pairs were obtained. As shown in Figure 4, up-regulated miR-129-5p showed regulatory pairs with 54 down-regulated mRNAs. Three down-regulated miRNAs, namely miR-714, miR-2145, and miR-2135, showed regulatory relations with 47 up-regulated mRNAs. Among the up-regulated mRNAs, Tcf712 showed common regulatory relationships with miR-714 and miR-2145. Meanwhile, miR-2145 indirectly correlated with miR-2135 by regulating 3 mRNAs, namely Vwc2, Upk1a, and Gad1.
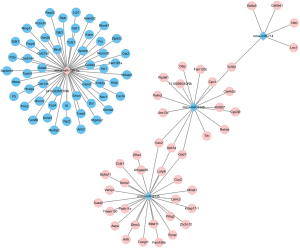
PPI network prediction
The STRING database was used to predict the PPI network with the highest confidence (interaction score >0.7). A total of 12 relation pairs were obtained with 17 DEGs, comprising 7 up-regulated mRNAs and 10 down-regulated mRNAs (Figure S1). There was no significant difference in the degree of connectivity between the protein interaction network nodes, which ranged from 1 to 2. Then, Cytoscape was used to integrate the PPI and mRNA-miRNA networks. As shown in Figure 5, the separated subnetworks were integrated into whole networks by several mRNA-mRNA regulatory pairs, such as Ins1-Sorcs1, Dst-Lamc2, Reln-Gad1, Kalm-Trh, and Ryr2-Camk2d.
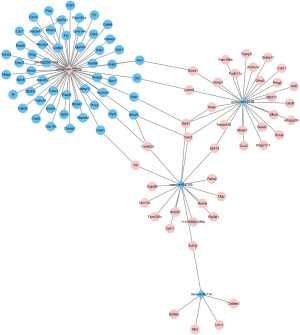
GO and KEGG analysis of the genes in the mRNA-miRNA network
By blasting genes with the GO and KEGG pathway databases, genes in the mRNA-miRNA network were annotated. As for biological processes (BP) in the GO analysis (Figure 6A), most genes were enriched in anatomical structure development, animal organ development, cell differentiation, and multicellular organism development. KEGG results (Figure 6B) showed that the DEGs were enriched in insulin secretion, circadian entrainment, Cushing syndrome, and alanine aspartate and glutamate metabolism pathways.
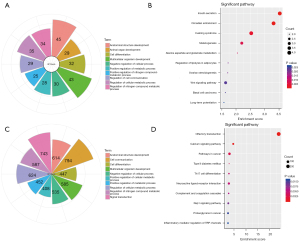
As for the DEGs for addiction function, the enriched BP terms were cell communication, signal transduction, regulation of cellular metabolic process, and regulation of nitrogen compound metabolic process (Figure 6C). The KEGG results (Figure 6D) showed that olfactory transduction, calcium signaling, neuroactive ligand-receptor interaction, and cancer pathways were enriched.
Cluster analysis of gene expression trends
The expression trends of mRNA time series datasets used for addiction research at 1, 2, 4, and 8 h were clustered by the WKNN (weighted K-Nearest neighbor) method, and those with similar expression patterns were grouped into 1 class. The results showed that the expression trends could be divided into 4 categories including down-up trend (cluster 1), continuous-down trend (cluster 2), up-down trend (cluster 3), and continuous-up trend (cluster 4) (Figure 7). Then, genes with the continuous up (cluster 3) or down (cluster 2) expression trends were selected for intersection analysis with the genes involved in the GO or KEGG terms. The results showed that Fos was the only gene in cluster 2, which intersected with the cAMP signaling pathway. In order to further explore the function of Fos gene, we performed GSEA analysis after grouping based on the expression level of Fos gene (divide by median). According to GSEA analysis, we found that high expression group is more enriched in the microtubule anchoring AND mRNA metabolism related pathway (Figure 8).
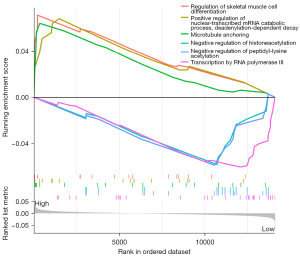
Discussion
Compared with other analgesic drugs, morphine has strong analgesic effect and strong addiction, and is mainly used for the treatment of cancer in clinic. However, the molecular mechanisms of morphine’s analgesic and addictive effects still need to be uncovered. In this study, we used expression data on the analgesic and addictive effects of morphine to identify the mRNAs and miRNAs involved in transcriptome alteration. A comprehensive network combining an mRNA-miRNA network which contained 101 regulatory relation pairs and a PPI network was built. From the network, 4 miRNAs including 1 up-regulated miRNA (miR-129-5p) and 3 down-regulated miRNAs (miR-714, miR-2135, and miR-2145) were found.
MiR-129 has long been studied as a biomarker for cancers such as gastric, rectal, and bladder cancer. In this study, the up-regulated miR-129-5c was recognized to respond to treatment with morphine in the transcriptome datasets for analgesia. These results were consistent with Tian et al.’s study which indicated that miR-129-5p alleviates neuropathic pain through regulating HMGB1 expression in chronic constriction injury (CCI) rat models (31). Peregud et al. also demonstrated that miR-129 that may regulate the content of brain-derived neurotrophic factor in the frontal cortex of rats after spontaneous morphine withdrawal (32). Yu et al. discovered the analgesic effects of miR-129-5p against bone cancer pain through the EphB1/EphrinB2 signaling pathway in mice (33). Our results confirmed that miR-129 may be closely related to the analgesic effect of morphine. Another 3 differently expressed miRNAs, namely miR-714, miR-2135, and miR-2145, also showed significant regulatory effects after treatment with morphine. However, no research has associated morphine or its analgesic effect with these 3 miRNAs. Further research needs to be conducted to demonstrate the effects of miR-714, miR-2135, and miR-2145 on morphine analgesia.
The insulin I (Ins1) gene encodes insulin to regulate the metabolism of sugars and fats. In our study, up-regulated miR-129 could directly regulate the expression of Ins1. However, there is still no evidence that Ins1 is associated with analgesia. By taking the intersection of genes, 8 common up-regulated and 22 down-regulated genes were obtained, which respond to both the analgesic and addictive effects of morphine. Lipoxygenase-3 (ALOXE3) encodes a hydroperoxide isomerase and was implicated in skin differentiation (34). In previous studies, ALOXE3 was shown to play a role in analgesia by inhibiting the expression of IL-6 (35-37). The corticotropin-releasing hormone receptor 2 (CRHR2) gene has been linked to mood and depression (38). In this study, CRHR2 was associated with both the analgesic and addictive effects of morphine. Pirnik et al. also found the expression of CRHR2 changed with the treatment of morphine (39). Mousa et al. also proved that CRHR2 could mediate the inhibition of inflammatory pain (40). Drug addiction and heroin addiction were discovered and evaluated in an African American population and in rats, respectively (41,42). CXCR4 is the receptor for SDF1, which has important functions in T lymphocyte trafficking (43). Wilson et al. revealed that CXCR4 signaling could mediate morphine-induced tactile hyperalgesia (44). In addition, numerous studies have linked this gene to the analgesic effects of morphine (45-47). Activation of CXCR4 by CXCL12 triggered the release of glutamate, which played a crucial role in alcohol and drug addiction (48).
Combining the results of genes in the continuously down-regulated cluster and KEGG analysis results, the Fos gene was obtained. Fos encodes a nuclear phosphoprotein to detect nerve cells that have recently been activated (49). Chang et al. demonstrated that morphine activated c-fos expression in the rat brain (50). In addition, Fos expression induced by electroacupuncture in the periaqueductal gray led to higher levels of analgesia (51). Takeda et al. revealed that Fos expression showed a high correlation with analgesia in the anterior cingulate cortex in a rat neuropathic pain model (52). For addiction effects, Cruz et al. used Fos to investigate neuronal ensembles in corticostriatal circuitry in addiction and found correlative evidence supporting a role for neuronal ensembles in addiction (53). Interestingly, alcohol, nicotine, and cocaine addiction were related to Fos mRNA expression (54-56). In summary, Fos is a key gene involved in pain relief and addiction, the development of inhibitors targeting the Fos gene may be beneficial to reduce the adverse effects of morphine addiction in clinical treatment.
Acknowledgments
Funding: This work was supported by Changning District Medical and Health Research Special Project (CNKW2020Y28).
Footnote
Reporting Checklist: The authors have completed the STREGA reporting checklist. Available at https://atm.amegroups.com/article/view/10.21037/atm-21-7037/rc
Conflicts of Interest: All authors have completed the ICMJE uniform disclosure form (available at https://atm.amegroups.com/article/view/10.21037/atm-21-7037/coif). The authors have no conflicts of interest to declare.
Ethical Statement: The authors are accountable for all aspects of the work in ensuring that questions related to the accuracy or integrity of any part of the work are appropriately investigated and resolved. The study was conducted in accordance with the Declaration of Helsinki (as revised in 2013).
Open Access Statement: This is an Open Access article distributed in accordance with the Creative Commons Attribution-NonCommercial-NoDerivs 4.0 International License (CC BY-NC-ND 4.0), which permits the non-commercial replication and distribution of the article with the strict proviso that no changes or edits are made and the original work is properly cited (including links to both the formal publication through the relevant DOI and the license). See: https://creativecommons.org/licenses/by-nc-nd/4.0/.
References
- Lee S, Park Y, Han E, et al. Thebaine in hair as a marker for chronic use of illegal opium poppy substances. Forensic Sci Int 2011;204:115-8. [Crossref] [PubMed]
- Goldberg J. Anatomy of a Scientific Discovery: The Race to Find the Body's Own Morphine. New York: Skyhorse Publishing, Inc., 2013.
- Meuser T, Pietruck C, Radbruch L, et al. Symptoms during cancer pain treatment following WHO-guidelines: a longitudinal follow-up study of symptom prevalence, severity and etiology. Pain 2001;93:247-57. [Crossref] [PubMed]
- Mostert JW, Evers JL, Hobika GH, et al. Cardiorespiratory effects of anaesthesia with morphine or fentanyl in chronic renal failure and cerebral toxicity after morphine. Br J Anaesth 1971;43:1053-60. [Crossref] [PubMed]
- Mercadante S. Pain treatment and outcomes for patients with advanced cancer who receive follow-up care at home. Cancer 1999;85:1849-58. [Crossref] [PubMed]
- Dalton GD, Bass CE, Van Horn CG, et al. Signal transduction via cannabinoid receptors. CNS Neurol Disord Drug Targets 2009;8:422-31. [Crossref] [PubMed]
- Paila YD, Chattopadhyay A. Membrane cholesterol in the function and organization of G-protein coupled receptors. Subcell Biochem 2010;51:439-66. [Crossref] [PubMed]
- Tsuda M, Inoue K, Salter MW. Neuropathic pain and spinal microglia: a big problem from molecules in "small" glia. Trends Neurosci 2005;28:101-7. [Crossref] [PubMed]
- Cherny N, Ripamonti C, Pereira J, et al. Strategies to manage the adverse effects of oral morphine: an evidence-based report. J Clin Oncol 2001;19:2542-54. [Crossref] [PubMed]
- Greene NM. The metabolism of drugs employed in anesthesia. I. Anesthesiology 1968;29:127-44. [Crossref] [PubMed]
- Cicero TJ, Meyer ER, Bell RD, et al. Effects of morphine and methadone on serum testosterone and luteinizing hormone levels and on the secondary sex organs of the male rat. Endocrinology 1976;98:367-72. [Crossref] [PubMed]
- Whistler JL, von Zastrow M. Morphine-activated opioid receptors elude desensitization by beta-arrestin. Proc Natl Acad Sci U S A 1998;95:9914-9. [Crossref] [PubMed]
- Vuong C, Van Uum SH, O'Dell LE, et al. The effects of opioids and opioid analogs on animal and human endocrine systems. Endocr Rev 2010;31:98-132. [Crossref] [PubMed]
- Schultz W. Potential vulnerabilities of neuronal reward, risk, and decision mechanisms to addictive drugs. Neuron 2011;69:603-17. [Crossref] [PubMed]
- Mazzucchelli C, Vantaggiato C, Ciamei A, et al. Knockout of ERK1 MAP kinase enhances synaptic plasticity in the striatum and facilitates striatal-mediated learning and memory. Neuron 2002;34:807-20. [Crossref] [PubMed]
- Liu LW, Lu J, Wang XH, et al. Neuronal apoptosis in morphine addiction and its molecular mechanism. Int J Clin Exp Med 2013;6:540-5. [PubMed]
- Basu S, Dasgupta PS. Dopamine, a neurotransmitter, influences the immune system. J Neuroimmunol 2000;102:113-24. [Crossref] [PubMed]
- Volkow ND, Fowler JS, Wang GJ, et al. Role of dopamine, the frontal cortex and memory circuits in drug addiction: insight from imaging studies. Neurobiol Learn Mem 2002;78:610-24. [Crossref] [PubMed]
- Hooks SB, Martemyanov K, Zachariou V. A role of RGS proteins in drug addiction. Biochem Pharmacol 2008;75:76-84. [Crossref] [PubMed]
- Marmiroli P, Cavaletti G. The glutamatergic neurotransmission in the central nervous system. Curr Med Chem 2012;19:1269-76. [Crossref] [PubMed]
- Lüscher C, Malenka RC. Drug-evoked synaptic plasticity in addiction: from molecular changes to circuit remodeling. Neuron 2011;69:650-63. [Crossref] [PubMed]
- Carter CJ. Toxoplasmosis and Polygenic Disease Susceptibility Genes: Extensive Toxoplasma gondii Host/Pathogen Interactome Enrichment in Nine Psychiatric or Neurological Disorders. J Pathog 2013;2013:965046. [Crossref] [PubMed]
- Wickham H. ggplot2: elegant graphics for data analysis. New York: Springer, 2016.
- Wickham H, Wickham MH. Package ‘plyr’. Available online: https://cran.rproject.org/web/packages/dplyr/dplyr.pdf
- Vu VQ. ggbiplot: A ggplot2 based biplot. R package 2011;342.
- Elliott CD, Murray G, Pearson L. Differential ability scales. San Antonio, Texas Harcourt Assessment, 1990.
- Harrell FE Jr, Harrell MFE Jr. Package ‘Hmisc’. CRAN2018 2019;2019:235-6.
- Wei T, Simko V, Levy M, et al. Package ‘corrplot’. Statistician 2017;56:e24.
- Smyth GK. Limma: linear models for microarray data. In: Gentleman R, Carey VJ, Huber W, et al. Bioinformatics and computational biology solutions using R and Bioconductor. New York: Springer, 2005; 397-420.
- Shannon P, Markiel A, Ozier O, et al. Cytoscape: a software environment for integrated models of biomolecular interaction networks. Genome Res 2003;13:2498-504. [Crossref] [PubMed]
- Tian J, Song T, Wang W, et al. miR-129-5p Alleviates Neuropathic Pain Through Regulating HMGB1 Expression in CCI Rat Models. J Mol Neurosci 2020;70:84-93. [Crossref] [PubMed]
- Peregud D, Panchenko L, Gulyaeva N. MicroRNA may regulate the content of the brain-derived neurotrophic factor in the frontal cortex of rats after spontaneous morphine withdrawal. Neurochemical Journal 2016;10:300-7. [Crossref]
- Yu SN, Liu GF, Li LY, et al. Analgesic effects of microRNA-129-5p against bone cancer pain through the EphB1/EphrinB2 signaling pathway in mice. J Cell Biochem 2019;120:2876-85. [Crossref] [PubMed]
- Yu Z, Schneider C, Boeglin WE, et al. The lipoxygenase gene ALOXE3 implicated in skin differentiation encodes a hydroperoxide isomerase. Proc Natl Acad Sci U S A 2003;100:9162-7. [Crossref] [PubMed]
- Wang XM, Hamza M, Wu TX, et al. Upregulation of IL-6, IL-8 and CCL2 gene expression after acute inflammation: Correlation to clinical pain. Pain 2009;142:275-83. [Crossref] [PubMed]
- Nada SA. Whey Protein, α-Lactalbumin and β-Lactoglobulin in Sprague Dawley Rat. The Egyptian Journal of Hospital Medicine 2009;35:335-46. [Crossref]
- Eliwa HA, El-Denshary ES, Nada SA, et al. Antinociceptive Effect Of Whey Protein And Its Fractions In Swiss Albino Mice. International Journal of Pharmaceutical Research and Bio-science 2012;1:355-81.
- Tochigi M, Kato C, Otowa T, et al. Association between corticotropin-releasing hormone receptor 2 (CRHR2) gene polymorphism and personality traits. Psychiatry Clin Neurosci 2006;60:524-6. [Crossref] [PubMed]
- Pirnik Z, Schwendt M, Jezova D. Single dose of morphine influences plasma corticosterone and gene expression of main NMDA receptor subunit in the adrenal gland but not in the hippocampus. Endocr Regul 2001;35:187-93. [PubMed]
- Mousa SA, Bopaiah PC, Stein C, et al. Involvement of corticotropin-releasing hormone receptor subtypes 1 and 2 in peripheral opioid-mediated inhibition of inflammatory pain. Pain 2003;106:297-307. [Crossref] [PubMed]
- Levran O, Randesi M, Li Y, et al. Drug addiction and stress-response genetic variability: association study in African Americans. Ann Hum Genet 2014;78:290-8. [Crossref] [PubMed]
- Levran O, Correa da Rosa J, Randesi M, et al. A non-coding CRHR2 SNP rs255105, a cis-eQTL for a downstream lincRNA AC005154.6, is associated with heroin addiction. PLoS One 2018;13:e0199951. [Crossref] [PubMed]
- Caruz A, Samsom M, Alonso JM, et al. Genomic organization and promoter characterization of human CXCR4 gene. FEBS Lett 1998;426:271-8. [Crossref] [PubMed]
- Wilson NM, Jung H, Ripsch MS, et al. CXCR4 signaling mediates morphine-induced tactile hyperalgesia. Brain Behav Immun 2011;25:565-73. [Crossref] [PubMed]
- Mei HX, Zhou MH, Zhang XW, et al. Effects of miR-338 on morphine tolerance by targeting CXCR4 in a rat model of bone cancer pain. Biosci Rep 2017;37:BSR20160517. [Crossref] [PubMed]
- Rivat C, Sebaihi S, Van Steenwinckel J, et al. Src family kinases involved in CXCL12-induced loss of acute morphine analgesia. Brain Behav Immun 2014;38:38-52. [Crossref] [PubMed]
- Luo X, Wang X, Xia Z, et al. CXCL12/CXCR4 axis: an emerging neuromodulator in pathological pain. Rev Neurosci 2016;27:83-92. [Crossref] [PubMed]
- Cui C, Shurtleff D, Harris RA. Neuroimmune mechanisms of alcohol and drug addiction. Int Rev Neurobiol 2014;118:1-12. [Crossref] [PubMed]
- Cochran BH, Zullo J, Verma IM, et al. Expression of the c-fos gene and of an fos-related gene is stimulated by platelet-derived growth factor. Science 1984;226:1080-2. [Crossref] [PubMed]
- Chang SL, Squinto SP, Harlan RE. Morphine activation of c-fos expression in rat brain. Biochem Biophys Res Commun 1988;157:698-704. [Crossref] [PubMed]
- de Medeiros MA, Canteras NS, Suchecki D, et al. Analgesia and c-Fos expression in the periaqueductal gray induced by electroacupuncture at the Zusanli point in rats. Brain Res 2003;973:196-204. [Crossref] [PubMed]
- Takeda R, Watanabe Y, Ikeda T, et al. Analgesic effect of milnacipran is associated with c-Fos expression in the anterior cingulate cortex in the rat neuropathic pain model. Neurosci Res 2009;64:380-4. [Crossref] [PubMed]
- Cruz FC, Javier Rubio F, Hope BT. Using c-fos to study neuronal ensembles in corticostriatal circuitry of addiction. Brain Res 2015;1628:157-73. [Crossref] [PubMed]
- Marinelli PW, Funk D, Juzytsch W, et al. Effects of opioid receptor blockade on the renewal of alcohol seeking induced by context: relationship to c-fos mRNA expression. Eur J Neurosci 2007;26:2815-23. [Crossref] [PubMed]
- Dehkordi O, Rose JE, Dávila-García MI, et al. Neuroanatomical Relationships between Orexin/Hypocretin-Containing Neurons/Nerve Fibers and Nicotine-Induced c-Fos-Activated Cells of the Reward-Addiction Neurocircuitry. J Alcohol Drug Depend 2017;5:273. [Crossref] [PubMed]
- Chae Y, Yang CH, Kwon YK, et al. Acupuncture attenuates repeated nicotine-induced behavioral sensitization and c-Fos expression in the nucleus accumbens and striatum of the rat. Neurosci Lett 2004;358:87-90. [Crossref] [PubMed]
(English Language Editor: C. Betlazar-Maseh)