Circulating microRNAs in seminal plasma as predictors of sperm retrieval in microdissection testicular sperm extraction
Introduction
Approximately 10–15% of couples experience infertility, and approximately 50% of these cases are caused by male infertility, of which approximately 10% represent the most severe manifestation, named nonobstructive azoospermia (NOA) (1). NOA is characterized by the complete absence of sperm in semen due to impaired spermatogenesis in the testes. However, because of focal spermatogenesis, testicular spermatozoa can be retrieved by microdissection testicular sperm extraction (micro-TESE) for intracytoplasmic sperm injection (ICSI) to achieve successful fertilization in some NOA patients (2-5). The major challenge with micro-TESE is to find predictors of active spermatogenesis foci indicating the likelihood of recovering sperm (6). It is essential to evaluate the sperm retrieval likelihood to determine whether the patient is currently suitable for micro-TESE. In particular, for patients receiving certain medications to enhance spermatogenesis recovery before the operation, the optimal operation time must be estimated according to the prognosis (7). Currently, testicular biopsy is widely performed for the prognosis of micro-TESE; however, it might miss foci with active spermatogenesis because of the “blind manner” of puncture (8-11). Additionally, as an invasive method, testicular biopsy may lead to severe complications (12). Therefore, a more reliable and safer prognostic method is needed.
Seminal plasma is the liquid component of semen, which arises from secretions from the seminal vesicles (~65%), prostate (~25%), testes and epididymides (~10%) and periurethral glands (~1%) (13). Secretions from the testes and epididymides provide molecules related to spermatogenesis and spermatozoa maturation, which could be potential biomarkers prompting spermatogenesis conditions (13-15). Several studies have reported proteins, circulating cells and cell-free DNA/RNA as potential biomarkers of nonobstructive azoospermia subtypes (16-18). Among these factors, circulating microRNAs could be ideal candidates because of their smaller size and encapsulation within proteins as they are less susceptible to degradation than other RNA species. MicroRNAs are a family of noncoding RNAs of approximately 19–23 nucleotides (nt) long that function as regulators of messenger RNA (mRNA) stability and translation (19). Many studies have indicated that microRNAs are involved in spermatogenesis and that aberrant expression of some microRNAs is related to male infertility (20-25). MicroRNAs have been reported as reliable biomarkers in various biopsies due to their stable characteristics (26,27). As one of the most important regulators of spermatogenesis, they have also been detected in human spermatozoa and seminal plasma (28). Several studies have investigated microRNAs in seminal plasma using either microarray or exosome microRNA profiling for indicators of spermatogenesis (29-31). However, neither microarray nor exosome microRNA profiling represents the total microRNAs that exist in the seminal plasma. Furthermore, those studies were limited by one or more of the following reasons: a lack of model establishment, a limited number of patients, and a lack of clinical validation.
In the present study, we screened whole microRNAs in seminal plasma from different groups of patients by improved absolute quantitative high-throughput small RNA sequencing (sRNA-seq) for more reliable biomarkers, and 4 microRNAs were identified as potential predictors through multiplex reverse transcription-quantitative polymerase chain reaction (RT-qPCR). A microRNA predictive model was established by ordered logistic regression through machine learning with qPCR data. The model was used to assess testicular spermatogenesis ratings to accurately estimate the likelihood of sperm retrieval in micro-TESE. Independent clinical validation of the model was performed to evaluate the predictive accuracy of the model (32). We present the following article in accordance with the STARD reporting checklist (available at https://atm.amegroups.com/article/view/10.21037/atm-21-5100/rc).
Methods
Patients and study design
All seminal plasma samples in this study were collected from Guangdong Provincial Reproductive Science Institute (Guangdong Provincial Fertility Hospital). The study was conducted in accordance with the Declaration of Helsinki (as revised in 2013). The study was approved by the Research Ethics Committee of Guangdong Provincial Reproductive Science Institute (Guangdong Provincial Fertility Hospital) (No. 2019025), and individual consent for this retrospective analysis was waived.
The samples were divided into 3 sets for different purposes in the study, including the screening set, training set and test set (Figure 1). Each set contained 3 groups that were divided according to the following testicular spermatogenesis conditions: (I) the “good” (G) group comprised fertile semen from the sperm bank, representing normal spermatogenesis; (II) the “fair” (F) group comprised NOA patients with 100% sperm retrieval in micro-TESE, representing hypospermatogenesis (existence of focal spermatogenesis); and (III) the “poor” (P) group comprised NOA patients with no sperm retrieval in micro-TESE, representing no active spermatogenesis. The screening set was used to identify significantly differentially expressed microRNAs as potential biomarkers for spermatogenesis. The training set was used for machine learning to construct ordered logistic regression modeling and establish a predictive model. The test set evaluated the model in which the RT-qPCR data of this set were applied to the model for prognosis, and the output result was compared with the clinical micro-TESE record to test the accuracy.
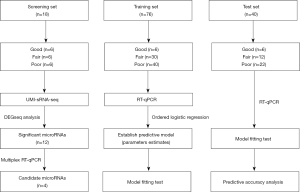
Seminal plasma small RNA purification
Polysaccharides make seminal plasma highly viscous, and routine small RNA (sRNA) purification methods for liquid samples, such as the solid-phase reversible immobilization (SPRI) bead-based method and TRIzol-LS method, produce significantly low amounts of sRNAs with large amounts of polysaccharide retention. To improve the quantity and quality of seminal plasma sRNAs, we combined the TRIzol-LS precipitation and column purification methods (Appendix 1). The concentration and purity of the enriched sRNAs were checked with NanoDrop One (Thermo Fisher Scientific, Waltham, MA, USA). The size distribution of the sRNAs was detected on an Agilent 2100 Bioanalyzer (Agilent Technologies, Santa Clara, CA, USA) (Table S1).
Absolute quantitative high-throughput sRNA sequencing (HTS) using a unique molecular index (UMI) and bioinformatic analysis
MicroRNA library construction for HTS includes a PCR amplification step, which can introduce a vast amount of bias because of the complicated structures of microRNAs. For seminal plasma microRNA, which has a low input of the starting materials, more cycles of PCR amplification are needed to obtain a sufficient amount for sequencing. Increased PCR amplification cycles produce more bias than microRNAs from other samples. To reduce PCR-derived artifacts, we adopted absolute quantitative microRNA sequencing with UMIs (Figure S1A) (33). This method could distinguish original copies of microRNAs from their amplification products by barcoding a random hexamer sequence as the UMI to each microRNA before PCR. After amplification, although multiple counts of each UMI could be observed, we precisely quantified the original copy number of each microRNA by simply counting each UMI. This UMI-based microRNA sequencing provided us with more reliable differentially expressed genes (DEGs) between groups and increased the efficiency of the validation progress by reducing the number of false DEGs. For normalization, we synthesized cel-miR-39 (TaKaRa Bio., Dalian, China) as a spike-in reference microRNA. Exactly 250 fmol of cel-miR-39 was added to each seminal plasma sample before sRNA purification. Approximately 100 ng of sRNAs per sample was used in library construction for HTS. During library construction, UMI-labeled primers were added (Figure S1A). All the samples were sequenced on a BGISEQ-500 system (Beijing Genomics Institution, Shenzhen, China), and bioinformatic analysis was performed following the standard pipeline (Figure S1B).
Multiplex RT-qPCR for microRNAs
Five microliters of sRNA were used as the template for a 20-µL reaction. RT-qPCR was designed based on the stem-loop method (Figure S2) (34). All the primers and probes used in this study are provided in Table S2. A multiplex RT reaction with 13 microRNA-specific stem-loop primers (SLPs) was performed, and an optimized pulsed RT reaction condition was applied to reduce nonspecific reactions and increase the RT efficiency (Appendix 1) (35). To increase the sensitivity of the qPCR, preamplification (pre-PCR) of the complementary DNA (cDNA) templates was necessary. The total RT product was used as a template, together with a specific forward primer (FP) cocktail (mixture of 13 microRNA-specific primers) and universal reverse primer (URP) (Appendix 1). To remove additional primers and deoxy-ribonucleoside triphosphate (dNTPs) in the pre-PCR product, which might affect qPCR, we performed cleanup treatment with ExoSAP-IT reagent (Thermo Fisher Scientific, Waltham, MA, USA; #75001) (Appendix 1). The products were purified using a MinElute PCR Purification Kit (Qiagen, Hilden, Germany; #28004) to obtain template pools for qPCR. Fluorescent dye-based qPCR was performed for candidate biomarker selection, and hydrolysis probe-based qPCR was performed for the construction of the predictive model (Appendix 1). To test the sensitivity of the stem-loop RT-qPCR-based microRNA expression profiling method in the multiplex format, we constructed the standard curve and R2 of each microRNA qPCR (Figure S3).
Statistical analysis
The input data for the analysis were the ∆Cq values (the Cq values of specific microRNAs minus the Cq values of the reference microRNA cel-miR-39). The fold change (FC) of the expression level was calculated as 2−∆Cq or 2−∆∆Cq. All statistical analyses were performed using R script. We established a predictive microRNA model based on training data using the function Polr (proportional odds logistic regression) of the MASS package in R-studio. A model fitting test was performed to evaluate the model. Receiver operating characteristic (ROC) curves were constructed to test the predictive accuracy of the model.
Results
Patient characteristics
The baseline characteristics of the NOA patients in this study are presented in Table 1. Testicular sperm was successfully retrieved in the fair group (age 30±4), and no sperm was retrieved in the poor group (age 31±5). There was no significant difference in the distribution of age, female partner age or testicular volume between the fair group and poor group. The mean follicle-stimulating hormone (FSH) and luteinizing hormone (LH) levels in the fair group (FSH 15.33 IU/L, 95% CI: 12.22–18.43; LH 7.02 IU/L, 95% CI: 5.06–8.97) were not significantly different (P=0.84; P=0.55) from those of the poor group (FSH 15.75 IU/L, 95% CI: 12.60–18.90; LH 7.87 IU/L, 95% CI: 5.64–10.09).
Table 1
Characteristics (mean ± SD) | Fair group (n=48) | Poor group (n=68) |
---|---|---|
Male age (years) | 30±4 | 31±5 |
Female partner age (years) | 28±5 | 28±4 |
Infertile duration (years) | 4±2 | 4±2 |
Testicular volume (mL) | 14±3* | 12±5* |
Serum FSH (IU/L) | 15.33±8.01* | 15.75±8.12* |
Serum LH (IU/L) | 7.02±5.05* | 7.87±5.73* |
*, no significant difference, Student’s t-test. NOA, nonobstructive azoospermia; FSH, follicle-stimulating hormone; LH, luteinizing hormone.
MicroRNA screening for candidate biomarkers
Our RNA-seq detected 786 known microRNAs from 18 samples, among which 158 were identified as differentially expressed microRNAs among groups based on the bioinformatic analysis with DEGseq, and 80 were defined as significantly differentially expressed microRNAs based on the expression FC (log2FC >2; P<0.01) (Figure 2). We further narrowed the range of candidates to 12 microRNAs (hsa-miR-34b-3p, hsa-miR-676-3p, hsa-miR-376a-3p, hsa-miR-449b-3p, hsa-miR-202-5p, hsa-miR-942-5p, hsa-miR-518e-3p, hsa-miR-891b, hsa-miR-4446-3p, hsa-miR-3065-3p, hsa-miR-30b-3p, and hsa-miR-34c-3p) based on their expression levels and patterns. We first eliminated 41 low-abundance microRNAs (expression level <20) and then divided the other 39 microRNAs into 4 groups based on their expression patterns in the 3 groups (Figure 3). We selected 2 groups of microRNAs whose expression patterns were consistent with the trend of spermatogenic situations from good to poor (Figure 3C,3D). The selected 2 groups contained 12 microRNAs in total, among which 9 microRNAs showed a positive correlation with active spermatogenesis, and 3 showed an opposite trend (Figure 3C,3D). In summary, 12 significantly differentially expressed microRNAs were identified as candidates for further validation via RT-qPCR.
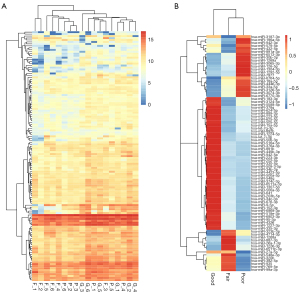
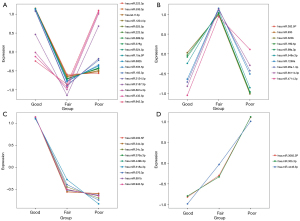
Differential expression profile of selected microRNAs
The 12 candidate microRNAs were tested by RT-qPCR with the 3 groups of sequencing samples to validate the FC of the expression level. We first tested the sensitivity of the stem-loop RT-qPCR-based microRNA expression profiling method in multiplex format. The standard curve and R2 of each microRNA qPCR were constructed. Among the 12 microRNAs, hsa-miR-202-5p, hsa-miR-518e-3p and hsa-miR-891b had R2 values of 0.9748, 0.9535 and 0.9312, respectively, and the other 9 microRNAs all had R2 values >0.99, indicating a sensitive and optimal amplification efficiency (Figure S3). Among these 12 microRNAs, only 4 (hsa-miR-34b-3p, hsa-miR-34c-3p, hsa-miR-3065-3p, and hsa-miR-4446-3p) displayed significantly differential expression patterns (Figure 4). The expression levels of hsa-miR-34b-3p and hsa-miR-34c-3p were significantly decreased from the “G” to the “P”, indicating that they are potential biomarkers for active spermatogenesis. The FC of the expression level of hsa-miR-34b-3p for “F”/“G” was 0.12 (P<0.001) and that for “P”/“G” was 0.03 (P<0.001). The FC of the expression level of hsa-miR-34c-3p for “F”/“G” was 0.62 (P<0.05) and that for “P”/“G” was 0.09 (P<0.001). The expression levels of hsa-miR-3065-3p and hsa-miR-4446-3p were significantly increased from the “G” group to the “P” group, indicating the severity of impaired spermatogenesis. The FC of the expression level of hsa-miR-3065-3p for “F”/“G” was 2.94 (P<0.01) and that for “P”/“G” was 3.44 (P<0.05). The FC of the expression level of hsa-miR-4446-3p for “F”/“G” was 2.66 (P<0.001) and that for “P”/“G” was 3.36 (P<0.01). Therefore, hsa-miR-34b-3p, hsa-miR-34c-3p, hsa-miR-3065-3p and hsa-miR-4446-3p were identified as candidate biomarkers for model establishment with the training set.
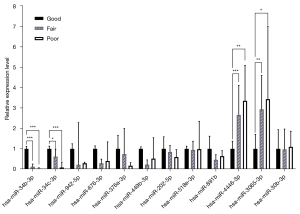
Establishment of the microRNA predictive model
Higher expression levels of hsa-miR-34b-3p and hsa-miR-34c-3p prompted more normal spermatogenesis and a higher possibility of retrieving sperm from micro-TESE. In contrast, higher expression levels of hsa-miR-3065-3p and hsa-miR-4446-3p implied more seriously impaired spermatogenesis and a lower likelihood of retrieving viable sperm. Thus, to examine how the expression levels of these 4 microRNAs predict the sperm retrieval outcome of micro-TESE, we performed an ordered logistic regression analysis. The input data for the ordered logistic regression analysis were the ∆Cq values of the 4 microRNAs from the training set (G group =6; F group =20; P group =30). We established the formula by estimating regression coefficients and the intercept in R using the Polr function in the MASS package (Table 2). The predicted probabilities of being estimated as “good”, “fair” and “poor” were denoted as “Pg”, “Pf” and “Pp”, respectively. According to the ordered logistic regression, we established the following formulas: Logit (Pg) = 2.9414 + 0.08932 hsa.miR.34b.3p + 0.59866 hsa.miR.34c.3p + 0.23658 hsa.miR.3065.3p + 0.19814 hsa.miR.4446.3p; Logit (Pg + Pf) = 3.9007 + 0.08932 hsa.miR.34b.3p + 0.59866 hsa.miR.34c.3p + 0.23658 hsa.miR.3065.3p + 0.19814 hsa.miR.4446.3p. Pp = 1 − (Pg + Pf).
Table 2
Variable | Value | Std.Error | t value |
---|---|---|---|
Coefficients | |||
hsa.miR.34b.3p | 0.13448 | 0.3400 | 0.3956 |
hsa.miR.34c.3p | 0.58679 | 0.3704 | 1.5843 |
hsa.miR.3065.3p | 0.15636 | 0.4112 | 0.3802 |
hsa.miR.4446.3p | 0.09523 | 0.3542 | 0.2688 |
Intercepts | |||
G|F† | 2.0881 | 2.7436 | 0.7611 |
F|P‡ | 3.0533 | 2.7656 | 1.1040 |
polr(formula = as.ordered(result) − hsa.miR.34b.3p + hsa.miR.34c.3p + hsa.miR.3065.3p + hsa.miR.4446.3p). †, Logit(Pg) = 2.0881 + 0.13448 hsa.miR.34b.3p + 0.58679 hsa.miR.34c.3p + 0.15636 hsa.miR.3065.3p + 0.09523 hsa.miR.4446.3p. ‡, Logit(Pg + Pf) = 3.0533 + 0.13448 has.miR.34b.3p + 0.58679 hsa.miR.34c.3p + 0.15636 hsa.miR.3065.3p + 0.09523 hsa.miR.4446.3p. Pp = 1 − Pg-Pf. micro-TESE, microdissection testicular sperm extraction.
Accuracy test of the predictive model
To evaluate the performance of the predictive model, we tested 40 samples with the model in a “blind” manner, meaning no clinical information was provided beforehand. We compared the results acquired from the predictive model to the clinical micro-TESE results. The clinical information revealed the following: 6 samples were from fertile men, denoted as “good”; 12 samples were from NOA patients with positive sperm retrieval, denoted as “fair”; and 22 samples were from NOA patients with negative sperm retrieval, denoted as “poor”. The prediction for the 40 samples from our model showed that all 6 fertile men were identified as in the “good” group, and 21 out of 22 patients with negative sperm retrieval were identified as in the “poor” group, indicating 100% and 95.45% accuracy rates in predicting patients who might show 100% and 0% sperm retrieval rates in micro-TESE, respectively (Table 3). For the 12 patients with positive sperm retrieval in micro-TESE, 8 were identified as in the “fair” group, 2 were identified as in the “good” group and 2 were identified as in the “poor” group. Because we claimed that both the “good” and “fair” groups represented the existence of active spermatogenesis in the testes, both samples appeared in the “good” and “fair” groups, implying a positive sperm retrieval likelihood in micro-TESE. Therefore, the accuracy rate for predicting sperm retrieval was 83.33%. We also calculated the sensitivity and specificity of the predictive model, which were 94.12% and 91.30%, respectively (Table 4). ROC curves were constructed based on the predictive performance of identifying positive sperm retrieval versus negative sperm retrieval (AUC =0.927), as well as for identifying “good” versus “poor” (AUC =0.955), “fair” versus “poor” (AUC =0.913) and “good” versus “fair” (AUC =0.707) (Figure 5).
Table 3
Predictive result | Good | Fair | Poor | Total |
---|---|---|---|---|
Good | 6 | 2 | 0 | 8 |
Fair | 0 | 8 | 1 | 9 |
Poor | 0 | 2 | 21 | 23 |
Total | 6 | 12 | 22 | – |
Accuracy | 100% | 66.67% | 95.45% | – |
Table 4
Predictive result | Clinical result | ||
---|---|---|---|
Positive (+) | Negative (−) | Total | |
Positive (+) | 16 | 2 | 18 |
Negative (−) | 1 | 21 | 22 |
Total | 17 | 23 | – |
Predictive result: FPR 8.70%; FNR 5.88%; TPR 88.89%; TNR 95.45%. Clinical result: sensitivity 94.12%; specificity 91.30%. FPR, false positive rate; FNR, false negative rate; TPR, true positive rate; TNR, true negative rate.
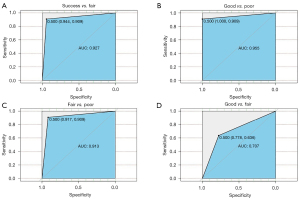
Discussion
As an effective method to retrieve testicular sperm from NOA patients, micro-TESE appears to be safe and has a higher yield of sperm than other methods. However, positive sperm retrieval was not possible from all patients. Thus, providing doctors and patients with a method for assessing the likelihood of retrieving sperm is essential, particularly when the patient requests another operation attempt following a failed sperm retrieval. Testicular biopsy, testicular ultrasound and hormone levels are assessment methods before micro-TESE; however, because of the heterogeneity of testicular tissue, these methods lack particular effectiveness (36). Several studies have been performed to identify molecular biomarkers of active spermatogenesis from seminal plasma, such as PIWI-interacting RNAs (piRNAs), mRNAs and microRNAs; however, these studies either lacked model construction or only established binary classification models (37).
In this study, we performed an ordered logistic regression with the 4 microRNAs identified from small RNA-seq and RT-qPCR for rating testicular spermatogenesis. With the predictive model we developed, patients could be sorted into 3 groups (good, fair and poor) based on their spermatogenesis conditions, allowing them to receive the appropriate treatment for a higher sperm retrieval rate. The model identified the “good” and “poor” samples with higher accuracy, and the more extreme the situation was, the more accurate the result was. However, for moderate-quality samples, the accuracy of the model must be improved. To improve the accuracy of micro-TESE outcome prediction, other factors should be considered together with the microRNA model to form a “predictive system”. For example, seminal plasma piRNAs, mRNA elements, long noncoding RNAs (lncRNAs), circulating DNAs, exfoliative cells, together with the histological diagnosis could be considered together for outcome prediction. We also summarized the histological results of the patients with different micro-TESE outcomes in this study (Table S3, Figure S4). Multiple panels should be established and combined by machine learning to make more accurate predictions. For the outcomes of assisted reproduction after micro-TESE, if testicular spermatozoa are retrieved by micro-TESE, NOA patients could achieve fatherhood via intracytoplasmic sperm injection (ICSI). The time of sperm retrieval and the time of egg retrieval from the partner might not be the same. There are 3 ways to select the timing of ICSI: egg and sperm retrieval in the same cycle, frozen egg recovery combined with sperm retrieval, and frozen sperm recovery combined with egg retrieval. Each method has certain limitations, such as the recovery rate of frozen eggs and sperm. In this study, according to the clinical records, among the 53 NOA patients who achieved successful sperm retrieval, 24 couples underwent ICSI, and 17 couples achieved a successful pregnancy. The other 29 patients froze their sperm for later use.
Conclusions
In this study, we established a predictive model to detect the possibility of sperm retrieval outcomes in micro-TESE. Our study has demonstrated that this model could provide considerable clinical value in identifying samples with a high likelihood of sperm retrieval and is valuable to direct the selection of the optimal time for operation planning.
Acknowledgments
Funding: This work was supported by the following grants: the National Natural Science Foundation of China (Grant No. 81801523 to YZ), the Guangzhou Municipal Science and Technology Bureau of China (Grant No. 201904010017 to YZ and No. 201707010394 to XZ), the Natural Science Foundation of Guangdong Province (Grant No. 2021A1515011544 to XZ), and the Chongqing Research Institute Performance Incentive and Guidance Project (No. cstc2018jxj l120004 to JH).
Footnote
Reporting Checklist: The authors have completed the STARD reporting checklist. Available at https://atm.amegroups.com/article/view/10.21037/atm-21-5100/rc
Data Sharing Statement: Available at https://atm.amegroups.com/article/view/10.21037/atm-21-5100/dss
Peer Review File: Available at https://atm.amegroups.com/article/view/10.21037/atm-21-5100/prf
Conflicts of Interest: All authors have completed the ICMJE uniform disclosure form (available at https://atm.amegroups.com/article/view/10.21037/atm-21-5100/coif). The authors have no conflicts of interest to declare.
Ethical Statement:
Open Access Statement: This is an Open Access article distributed in accordance with the Creative Commons Attribution-NonCommercial-NoDerivs 4.0 International License (CC BY-NC-ND 4.0), which permits the non-commercial replication and distribution of the article with the strict proviso that no changes or edits are made and the original work is properly cited (including links to both the formal publication through the relevant DOI and the license). See: https://creativecommons.org/licenses/by-nc-nd/4.0/.
References
- Jarow JP, Espeland MA, Lipshultz LI. Evaluation of the azoospermic patient. J Urol 1989;142:62-5. [Crossref] [PubMed]
- Friedler S, Raziel A, Strassburger D, et al. Testicular sperm retrieval by percutaneous fine needle sperm aspiration compared with testicular sperm extraction by open biopsy in men with non-obstructive azoospermia. Hum Reprod 1997;12:1488-93. [Crossref] [PubMed]
- Schlegel PN. Testicular sperm extraction: microdissection improves sperm yield with minimal tissue excision. Hum Reprod 1999;14:131-5. [Crossref] [PubMed]
- Donoso P, Tournaye H, Devroey P. Which is the best sperm retrieval technique for non-obstructive azoospermia? A systematic review. Hum Reprod Update 2007;13:539-49. [Crossref] [PubMed]
- Ostad M, Liotta D, Ye Z, et al. Testicular sperm extraction for nonobstructive azoospermia: results of a multibiopsy approach with optimized tissue dispersion. Urology 1998;52:692-6. [Crossref] [PubMed]
- Westlander G. Utility of micro-TESE in the most severe cases of non-obstructive azoospermia. Ups J Med Sci 2020;125:99-103. [Crossref] [PubMed]
- Deruyver Y, Vanderschueren D, Van der Aa F. Outcome of microdissection TESE compared with conventional TESE in non-obstructive azoospermia: a systematic review. Andrology 2014;2:20-4. [Crossref] [PubMed]
- Takada S, Tsujimura A, Ueda T, et al. Androgen decline in patients with nonobstructive azoospemia after microdissection testicular sperm extraction. Urology 2008;72:114-8. [Crossref] [PubMed]
- Tsujimura A, Matsumiya K, Miyagawa Y, et al. Conventional multiple or microdissection testicular sperm extraction: a comparative study. Hum Reprod 2002;17:2924-9. [Crossref] [PubMed]
- Ramasamy R, Yagan N, Schlegel PN. Structural and functional changes to the testis after conventional versus microdissection testicular sperm extraction. Urology 2005;65:1190-4. [Crossref] [PubMed]
- Colpi GM, Colpi EM, Piediferro G, et al. Microsurgical TESE versus conventional TESE for ICSI in non-obstructive azoospermia: a randomized controlled study. Reprod Biomed Online 2009;18:315-9. [Crossref] [PubMed]
- Ghalayini IF, Al-Ghazo MA, Hani OB, et al. Clinical comparison of conventional testicular sperm extraction and microdissection techniques for non-obstructive azoospermia. J Clin Med Res 2011;3:124-31. [Crossref] [PubMed]
- Drabovich AP, Saraon P, Jarvi K, et al. Seminal plasma as a diagnostic fluid for male reproductive system disorders. Nat Rev Urol 2014;11:278-88. [Crossref] [PubMed]
- Ramasamy R, Lin K, Gosden LV, et al. High serum FSH levels in men with nonobstructive azoospermia does not affect success of microdissection testicular sperm extraction. Fertil Steril 2009;92:590-3. [Crossref] [PubMed]
- Osaka A, Iwahata T, Kobori Y, et al. Testicular volume in non-obstructive azoospermia with a history of bilateral cryptorchidism may predict successful sperm retrieval by testicular sperm extraction. Reprod Med Biol 2020;19:372-7. [Crossref] [PubMed]
- Drabovich AP, Dimitromanolakis A, Saraon P, et al. Differential diagnosis of azoospermia with proteomic biomarkers ECM1 and TEX101 quantified in seminal plasma. Sci Transl Med 2013;5:212ra160. [Crossref] [PubMed]
- Li HG, Huang SY, Zhou H, et al. Quick recovery and characterization of cell-free DNA in seminal plasma of normozoospermia and azoospermia: implications for non-invasive genetic utilities. Asian J Androl 2009;11:703-9. [Crossref] [PubMed]
- Wu W, Hu Z, Qin Y, et al. Seminal plasma microRNAs: potential biomarkers for spermatogenesis status. Mol Hum Reprod 2012;18:489-97. [Crossref] [PubMed]
- Bartel DP. MicroRNA Target Recognition and Regulatory Functions. Cell 2009;136:215-33. [Crossref] [PubMed]
- Ro S, Park C, Sanders KM, et al. Cloning and expression profiling of testis-expressed microRNAs. Dev Biol 2007;311:592-602. [Crossref] [PubMed]
- Yuan S, Schuster A, Tang C, et al. Sperm-borne miRNAs and endo-siRNAs are important for fertilization and preimplantation embryonic development. Development 2016;143:635-47. [PubMed]
- Wu J, Bao J, Kim M, et al. Two miRNA clusters, miR-34b/c and miR-449, are essential for normal brain development, motile ciliogenesis, and spermatogenesis. Proc Natl Acad Sci U S A 2014;111:E2851-7. [Crossref] [PubMed]
- Zhang Y, Tang C, Yu T, et al. MicroRNAs control mRNA fate by compartmentalization based on 3’ UTR length in male germ cells. Genome Biol 2017;18:105. [Crossref] [PubMed]
- Bao J, Li D, Wang L, et al. MicroRNA-449 and microRNA-34b/c function redundantly in murine testes by targeting E2F transcription factor-retinoblastoma protein (E2F-pRb) pathway. J Biol Chem 2012;287:21686-98. [Crossref] [PubMed]
- Wu Q, Song R, Ortogero N, et al. The RNase III enzyme DROSHA is essential for microRNA production and spermatogenesis. J Biol Chem 2012;287:25173-90. [Crossref] [PubMed]
- Chen X, Ba Y, Ma L, et al. Characterization of microRNAs in serum: a novel class of biomarkers for diagnosis of cancer and other diseases. Cell Res 2008;18:997-1006. [Crossref] [PubMed]
- Huang S, Li H, Ding X, et al. Presence and characterization of cell-free seminal RNA in healthy individuals: implications for noninvasive disease diagnosis and gene expression studies of the male reproductive system. Clin Chem 2009;55:1967-76. [Crossref] [PubMed]
- Daneshmandpour Y, Bahmanpour Z, Hamzeiy H, et al. MicroRNAs association with azoospermia, oligospermia, asthenozoospermia, and teratozoospermia: a systematic review. J Assist Reprod Genet 2020;37:763-75. [Crossref] [PubMed]
- Wang C, Yang C, Chen X, et al. Altered profile of seminal plasma microRNAs in the molecular diagnosis of male infertility. Clin Chem 2011;57:1722-31. [Crossref] [PubMed]
- Zhang W, Zhang Y, Zhao M, et al. MicroRNA expression profiles in the seminal plasma of nonobstructive azoospermia patients with different histopathologic patterns. Fertil Steril 2021;115:1197-211. [Crossref] [PubMed]
- Barceló M, Mata A, Bassas L, et al. Exosomal microRNAs in seminal plasma are markers of the origin of azoospermia and can predict the presence of sperm in testicular tissue. Hum Reprod 2018;33:1087-98. [Crossref] [PubMed]
- Choi SH, Labadorf AT, Myers RH, et al. Evaluation of logistic regression models and effect of covariates for case-control study in RNA-Seq analysis. BMC Bioinformatics 2017;18:91. [Crossref] [PubMed]
- Fishman A, Light D, Lamm AT. QsRNA-seq: a method for high-throughput profiling and quantifying small RNAs. Genome Biol 2018;19:113. [Crossref] [PubMed]
- Tang F, Hajkova P, Barton SC, et al. MicroRNA expression profiling of single whole embryonic stem cells. Nucleic Acids Res 2006;34:e9. [Crossref] [PubMed]
- Moltzahn F, Olshen AB, Baehner L, et al. Microfluidic-based multiplex qRT-PCR identifies diagnostic and prognostic microRNA signatures in the sera of prostate cancer patients. Cancer Res 2011;71:550-60. [Crossref] [PubMed]
- Gnessi L, Scarselli F, Minasi MG, et al. Testicular histopathology, semen analysis and FSH, predictive value of sperm retrieval: supportive counseling in case of reoperation after testicular sperm extraction (TESE). BMC Urol 2018;18:63. [Crossref] [PubMed]
- Cao C, Wen Y, Wang X, et al. Testicular piRNA profile comparison between successful and unsuccessful micro-TESE retrieval in NOA patients. J Assist Reprod Genet 2018;35:801-8. [Crossref] [PubMed]