Network pharmacology and molecular docking analysis reveals the mechanism of asiaticoside on COVID-19
Introduction
Coronavirus disease 2019 (COVID-19) was induced by severe acute respiratory syndrome coronavirus 2 (SARS-CoV-2), a haunting coronavirus discovered in humans. The initial outbreak of SARS was caused by the peer of the same species of SARS-CoV-2, a SARS-CoV (1). Later, the middle east respiratory syndrome-related coronavirus (MERS-CoV) caused the MERS outbreak in 2015 (2). Like its predecessors, COVID-19 has wreaked severe outbreaks of acute respiratory diseases and provoked great panic worldwide (3). Since its first report in Wuhan, Hubei, China, COVID-19 has spread to the whole world with almost 100 million diagnosed patients and caused more than 2,141,468 deaths by 11:55 am Central European Time (CET) 27 January 2021, as reported by the World Health Organization (WHO). Patients with COVID-19 exhibited hyperinflammatory response, also called a cytokine storm, which has been observed and is suspected of causing the detrimental progression of COVID-19 (4). The common symptoms of COVID-19 patients have been dry cough, fever, upper airway congestion, shortness of breath, sputum production, fatigue, arthralgia, and myalgia (5). Older populations and those with reduced immunity have proven more likely to exhibit severe symptoms like pneumonia, acute respiratory distress syndrome (ARDS), acute respiratory failure, kidney failure, and even death (5). Currently, the disease’s exact pathogenesis is not fully understood, and effective disease-specific drugs are greatly anticipated.
Traditional Chinese medicine (TCM) is a comprehensive system with multicomponent and multi-target characteristics that exert synergistic effects on many diseases with fewer side effects. The potential of TCM in the prevention and treatment of COVID-19 has attracted mounting interest. Based on the classification system of TCM, the core pathogenesis of COVID-19 is a wet epidemic caused by cold and humidity outside the lung and spleen, which transforms into heat and leads to heat stagnation (4). Throughout the thousands of years of Chinese civilization, TCM has acquired rich clinical experience in diagnosing and treating epidemics and has been shown to reduce the mortality rate and improve the prognosis of a range of diseases. It has been demonstrated that TCM could shorten fever duration and accelerate symptomatic relief in patients with severe COVID-19 (6,7). In the Diagnosis and Treatment Program of COVID-19 (trial version 7; available at: http://www.nhc.gov.cn/xcs/zhengcwj/202003/46c9294a7dfe4cef80dc7f5912eb1989.shtml), jointly issued by the National Health Commission and the National Administration of Traditional Chinese Medicine, Chinese herbal decoctions, and Chinese patent medicine, TCM has been suggested as a possible option for the treatment of COVID-19. More than 85% of SARS-CoV-2-infected patients have received TCM treatment in China (8). Early intervention with TCM could delay the disease progression, shorten the disease course, improve the cure rate, and reduce the mortality rate (7,9). However, the complicated ingredients of TCM make it challenging to understand the mechanisms.
Asiaticoside (AS) is a natural Chinese medicine monomer extracted from Centella Asiatica, which has been used for many years to treat dermal disorders, venous insufficiency, and microangiopathy (10). In 2008, AS was launched in China with the approval of the State Food and Drug Administration (Z20083081). It has two pharmaceutical forms, including AS tablet and AS ointment. It has been reported that AS has potent pharmacological activity as well as broader pharmacological effects including anti-oxidant and scavenging free radicals, antidepressant and anti-anxiety, immune regulation and anti-inflammatory, anti-ulcer, hepato-protective, and antitumor activities (11,12). Researchers have found that AS exerts therapeutic effects on diarrhea, asthma, tuberculosis, atherosclerosis, wound healing, as well as antifungal and antibacterial (13,14). It has the functions of relieving asthma, clearing heat, and detoxifying dampness (15). It is effective against pulmonary infection and prevents lipopolysaccharide (LPS)-induced acute lung injury through potent anti-inflammatory effects (16). Also, AS has been shown to have an impact on the prevention and treatment of H5N1/H1N1 by inhibiting the mTOR pathway (17). Based on the evidence above, we proposed that AS could exert a positive therapeutic effect on COVID-19.
In regard to the working mechanism, TCM regulates the human body synergistically through multiple targets, although it is challenging to identify specific action mechanisms. Network pharmacology is an emerging method that merges computer science and clinical medicine. Meanwhile, it constructs and visualizes the ‘multi-gene, multi-target, and multi-pathway’ interaction network to assess the molecular mechanism of medicine (18). This approach is especially suitable for researching multicomponent medicines such as TCM due to their complex matrix nature (19). Molecular docking is a pathway for structural molecular biology and computer-aided drug design in novel medicines (20). The targets found by network pharmacology are considered as receptors. Therefore, the integration of network pharmacology and molecular docking helps accelerate experimental verification and target discovery (4). With the help of network pharmacology and molecular docking, we explored the molecular mechanism of how AS improves COVID-19 and laid the foundation for investigating the molecular basis of COVID-19 treatment with AS. The detailed procedure is shown in Figure 1. Although AS has not been applied for COVID-19 in China at present, our study would show it was beneficial in the treatment of COVID-19 and it may be a potential agent. The research also provided a rapid channel for the discovery and application of new anti-CoV therapeutics.
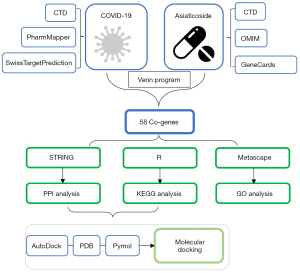
Methods
Predicting the targets of AS
The study was conducted in accordance with the Declaration of Helsinki (as revised in 2013). The protein targets related to AS were retrieved from SwissTargetPrediction (http://www.swisstargetprediction.ch/), Comparative Toxicogenomics Database (CTD; (https://ctdbase.org/;jsessionid=84E0B5D0353A4B9A77545215380F0CA7), and the PharmMapper database (http://www.lilab-ecust.cn/pharmmapper/). After the line notations (from Simplified Molecular-Input Line-Entry System) of AS were imported into the SwissTargetPrediction database, target information was then obtained. The SwissTargetPrediction database is a web server that accurately predicts the similarity in the targets of bioactive molecules based on their chemistry (21). PharmMapper (22) could predict potential biological targets for a given small molecule against all the experimentally determined three-dimensional (3D) structures of proteins available on PharmTargetDB. The candidate targets of AS were predicted by PharmMapper after the 3D structures had been submitted, and all the parameters were kept as default. The CTD is a publicly available database providing curated core information about chemical genes/protein interactions, chemical diseases, and gene-disease relationships from peer-reviewed literature (23). The potential targets of AS were predicted using CTD with default setting parameters.
After removal of duplicated data, the desired targets were obtained. Then, the target proteins screened from the three databases were standardized in The Universal Protein Resource (UniProt; https://www.uniprot.org/), and the targets of “Homo sapiens” were reserved for further analysis.
Predicting the targets of COVID-19
The COVID-19-related target proteins were screened from the following three sources: (I) GeneCards database (https://www.genecards.org/), which is an online database of human genes and genetic diseases enabling navigate gene-disease linkages (24); (II) Online Mendelian Inheritance in Man database (OMIM; https://www.omim.org/), which possesses over 15,500 gene entries, and focuses on explaining the relationships of gene-phenotype (25); (III) CTD database. We established a COVID-19-related gene set by combining these search results.
Intersection of drug targets and disease targets
Using the Venn diagram intersection, we mapped the COVID-19 targets to those of AS and located the overlapping drug-disease targets. These targets were considered potential targets of AS action in the treatment of COVID-19.
Protein-protein interactions (PPIs) network construction
We gathered the common targets of COVID-19 and AS as the co-targets of AS for COVID-19. The Search Tool for the Retrieval of Interacting Genes/Proteins database (STRING; https://cn.string-db.org/cgi/input.pl) was used to integrate all publicly available sources of PPI information and complement these with computational predictions. The co-targets were input into STRING and a PPI network was built to construct a comprehensive global network, including physical and functional interactions. The cutoff of the PPI confidence score was 0.4. Degree stood for the number of connections of the node in the whole network, reflecting the interaction information between nodes and the importance of the core targets. The targets were sorted by degree, and the top 30 nodes were displayed.
Construction of the whole network
The intersection of PPI target and drug-disease common targets were taken for further analysis. A visual drug-disease-target network was established based on data mentioned above through Cytoscape (version 3.8.0) to visualize the complicated relationships between drug-disease and their potential targets (26). In the network, nodes represented the drug, targets, and disease, while the connections between them represented these biological interactions. The top 16 targets were listed in the central panel, which was screened based on the degree value. The molecular degree value reflected the number of connections between the molecular and target in the network (27). A larger value indicated a greater possibility for the component to become the critical target of AS for COVID-19.
Gene Ontology (GO) and Kyoto Encyclopedia of Genes and Genomes (KEGG) pathway enrichment analysis
Metascape (https://metascape.org/gp/index.html#/main/) is a portal website designed to provide an extensive gene list annotation and analysis resource. Metascape integrates gene annotation, functional enrichment, membership search, and interactome analysis to leverage over 40 independent knowledgebases within one integrated portal in the field of design features. Therefore, Metascape was useful in processing data for GO analysis on following aspects: biological process (BP), molecular function (MF), and cellular component (CC) (28).
To understand gene functions and signaling pathways of potential targets, KEGG pathway analysis (29) was carried out using the clusterProfiler package and org.Hs.eg.db (30,31) to locate the gene ID of the potential targets. The statistical significance threshold of enrichment analysis was P<0.05 and adjusted P<0.05. The top 20 enriched terms were shown on a chord plot if exist.
AS-target molecular docking
After taking the intersection of genes in the whole network and involved in the KEGG pathways, the top 9 targets (ranked by their degree values) were chosen for molecular docking analysis. In detail, the 9 targets and AS were used as receptors and ligands, respectively. The 3D structure of these targets was obtained from the Protein Data Bank (PDB) database (https://www.rcsb.org/). AutoDock 1.5.6 (https://autodock.scripps.edu/) was used to remove the water molecules, add the nonpolar hydrogen, isolate proteins, calculate Gasteiger charges for the structure, and perform molecular docking using local search parameters. The generated conformation with the best affinity was then selected and visualized in Pymol 2.3 (https://pymol.org/2/).
Statistical analysis
The cutoff of the PPI confidence score was 0.4 by STRING. GO analysis was performed by Metascape and KEGG pathway analysis was conducted using R as mentioned above. The statistical significance threshold of enrichment analysis was P<0.05 and adjusted P<0.05. Molecular docking was carried out using local search parameters. The generated conformation with the best affinity was then selected and visualized in Pymol.
Results
Screening of potential drug-disease targets
As shown in Table 1, 97 potential targets for AS action were mined. Additionally, a total of 7,415 COVID-19 associated genes were acquired from the CTD, GeneCards, and OMIM databases (online available: https://cdn.amegroups.cn/static/public/atm-22-51-01.pdf). The mapping of the AS targets with the COVID-19 targets yielded 58 genes, which were the possible targets of the AS action in treating the disease (Figure 2 and Table 2).
Table 1
Symbol | Source |
---|---|
RARG | PharmMapper |
NF2 | PharmMapper |
CSDE1 | PharmMapper |
PRPS1 | PharmMapper |
MAPK9 | PharmMapper |
UAP1 | PharmMapper |
PCNA | PharmMapper |
CCNE1 | PharmMapper |
NUDT18 | PharmMapper |
FES | PharmMapper |
ZEB2 | PharmMapper |
MUC1 | PharmMapper |
ITPKC | PharmMapper |
IQUB | PharmMapper |
TIMM9 | PharmMapper |
HBEGF | PharmMapper |
ARHGAP5 | PharmMapper |
MSN | PharmMapper |
TRIM21 | PharmMapper |
NAGK | PharmMapper |
RND1 | PharmMapper |
BTK | PharmMapper |
FABP2 | PharmMapper |
RARB | PharmMapper |
VAV2 | PharmMapper |
HSCB | PharmMapper |
IGHV4-59 | PharmMapper |
GALM | PharmMapper |
KYNU | PharmMapper |
SULT2A1 | PharmMapper |
ACADVL | PharmMapper |
NR1I3 | PharmMapper |
NR3C2 | PharmMapper |
NR3C2 | PharmMapper |
MAP3K3 | PharmMapper |
AOC3 | PharmMapper |
HBB | PharmMapper |
MAPKAPK2 | PharmMapper |
PDK3 | PharmMapper |
MYSM1 | PharmMapper |
NEO1 | PharmMapper |
PDK2 | PharmMapper |
HIBCH | PharmMapper |
HFE | PharmMapper |
GALK1 | PharmMapper |
DTYMK | PharmMapper |
ACVR2B | PharmMapper |
ASAP1 | PharmMapper |
NFATC1 | PharmMapper |
NAE1 | PharmMapper |
CUX2 | PharmMapper |
HSP90AA1 | PharmMapper |
TUT1 | PharmMapper |
CDK5R1 | PharmMapper |
MAGEA4 | PharmMapper |
NMRK1 | PharmMapper |
RRM1 | PharmMapper |
MMP2 | PharmMapper |
HLA-E | PharmMapper |
RAN | PharmMapper |
MAPK6 | PharmMapper |
POU2F1 | PharmMapper |
PAH | PharmMapper |
HMGCS1 | PharmMapper |
IRF4 | PharmMapper |
MMP1 | PharmMapper |
FCGR2A | PharmMapper |
NCOA3 | PharmMapper |
PRPSAP2 | PharmMapper |
ZCWPW1 | PharmMapper |
BCL2L1 | SwissTargetPrediction |
HSD11B2 | SwissTargetPrediction |
HSD11B1 | SwissTargetPrediction |
JUN | SwissTargetPrediction |
PTPA | SwissTargetPrediction |
GLI1 | SwissTargetPrediction |
F2 | SwissTargetPrediction |
GLRA1 | SwissTargetPrediction |
GLRA2 | SwissTargetPrediction |
PTAFR | SwissTargetPrediction |
STAT3 | SwissTargetPrediction |
RORC | SwissTargetPrediction |
FDFT1 | SwissTargetPrediction |
PTPN1 | SwissTargetPrediction |
BDNF | CTD |
NGF | CTD |
PDPK1 | CTD |
CAT | CTD |
CYP2C19 | CTD |
CYP3A4 | CTD |
MAPK14 | CTD |
MTOR | CTD |
PEBP1 | CTD |
PIK3R6 | CTD |
SLC18A2 | CTD |
STX1A | CTD |
SYNJ1 | CTD |
TH | CTD |
AS, asiaticoside; CTD, Comparative Toxicogenomics Database.
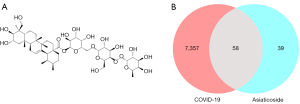
Table 2
Symbol |
NF2, CSDE1, MAPK9, PCNA, CCNE1, NUDT18, ZEB2, MUC1, ITPKC, TIMM9 |
HBEGF, MSN, TRIM21, RND1, BTK, FABP2, RARB, VAV2, GALM, ACADVL |
NR3C2, HBB, PDK3, PDK2, HFE, ACVR2B, ASAP1, NFATC1, NAE1, HSP90AA1 |
TUT1, CDK5R1, MMP2, POU2F1, HMGCS1, IRF4, MMP1, NCOA3, PRPSAP2, BCL2L1 |
HSD11B2, HSD11B1, JUN, F2, GLRA1, PTAFR, STAT3, PTPN1, BDNF, NGF |
CAT, CYP2C19, CYP3A4, MAPK14, MTOR, PEBP1, SLC18A2, TH |
AS, asiaticoside; COVID-19, coronavirus disease 2019.
PPI network of the co-targets
To understand the mechanism of AS in treating COVID-19, it was necessary to determine the interactive effect of the co-target proteins. By overlapping the AS targets with those involved in COVID-19, 58 potential co-targets were identified and fed into the STRING database to obtain the interlaced network containing correlations among targets. After hiding the disconnected nodes, 45 targets were presented in the whole network (Figure 3A and Table 3). The top 30 targets are shown in Figure 3B, which were expected as the core targets in the PPI network. They probably participate in the treatment mechanism of AS for COVID-19. It was assumed that AS carries out its medicinal effect and treats COVID-19 by acting on these core targets.
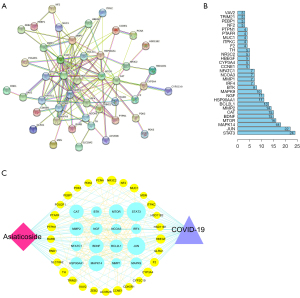
Table 3
Target | Degree |
---|---|
STAT3 | 24 |
JUN | 22 |
MAPK14 | 18 |
MTOR | 16 |
BDNF | 15 |
CAT | 15 |
MMP2 | 14 |
BCL2L1 | 13 |
HSP90AA1 | 11 |
NGF | 11 |
MAPK9 | 10 |
BTK | 8 |
IRF4 | 7 |
MMP1 | 7 |
NCOA3 | 7 |
NFATC1 | 7 |
CCNE1 | 5 |
CYP3A4 | 5 |
HBEGF | 5 |
NR3C2 | 5 |
TH | 5 |
F2 | 4 |
ITPKC | 4 |
MUC1 | 4 |
PTAFR | 4 |
PTPN1 | 4 |
NF2 | 3 |
PEBP1 | 3 |
TRIM21 | 3 |
VAV2 | 3 |
ACVR2B | 2 |
CDK5R1 | 2 |
HSD11B1 | 2 |
PCNA | 2 |
PDK2 | 2 |
POU2F1 | 2 |
RARB | 2 |
SLC18A2 | 2 |
ZEB2 | 2 |
CYP2C19 | 1 |
GLRA1 | 1 |
HSD11B2 | 1 |
MSN | 1 |
PDK3 | 1 |
RND1 | 1 |
The construction of the disease-target-drug network
After taking the intersection of drug-disease co-targets and PPI targets, we condensed the disease-target-drug network containing 47 nodes (including AS, COVID-19, and 45 genes) and 233 edges (Figure 3C). A larger node reflected greater importance. Interestingly, the genes in the network were precisely consistent with those in PPI, which illustrated the accuracy of the analysis results. The top 16 genes with a degree above 9 were involved in transcription 3 (STAT3), jun proto-oncogene (JUN), mitogen-activated protein kinase 14 (MAPK14), mechanistic target of rapamycin (mTOR), brain-derived neurotrophic factor (BDNF), catalase (CAT), matrix metallopeptidase 2 (MMP2), BCL2 like 1 (BCL2L1), nerve growth factor (NGF), heat shock protein 90 alpha family class a member 1 (HSP90AA1), MAPK9, Bruton tyrosine kinase (BTK), nuclear factor of activated T cells 1 (NFATC1), interferon regulatory factor 4 (IRF4), MMP1, and nuclear receptor coactivator 3 (NCOA3).
GO enrichment analysis
Currently, a problem exists in most GO analyses. The redundancies in ontologies and descriptors can sometimes complicate the interpretation of the output. For instance, ontology terms found in GO form a hierarchical structure of increasing granularity, which makes the terms unnecessarily redundant. Besides, terms from different ontology sources can be closely related. As the functional enrichment analysis can identify overlapping or related terms, it is not effortless to extract non-redundant and representative processes to report in the analysis output. To further understand the core genes in different GO terms, Metascape, which could cluster the redundant terms, was used to perform GO enrichment analysis. The GO chord plots which could represent the core targets related to GO annotations were constructed.
In the right panel of Figure 4 (P<0.05), the color transition from red to purple represents the increasing P value and the decreasing significance of a term. In the left panel, the genes from top to bottom implies the descending degree and importance of the core genes. As shown in Figure 4A (P<0.05), the 20 top-ranking terms about BP were chosen. The positive regulation of transferase activity, response to acid chemical, reactive oxygen species metabolic process, muscle cell proliferation, positive regulation of CC biogenesis, leukocyte activation, and so on, were included. Based on CC enrichment analysis, the targets contained serine/threonine protein kinase complex, presynapse, perinuclear region of cytoplasm, Golgi lumen, and ficolin-1-rich granule lumen, RNA polymerase II transcription factor complex, and mitochondrial matrix (Figure 4B; P<0.05). Simultaneously, MF terms mainly contained protein kinase binding, transcription factor binding, kinase activity, MAPK binding, lipid binding, oxygen binding, nuclear receptor activity, protein homodimerization activity, growth factor activity, protein tyrosine kinase binding, protein domain specific binding, protein phosphatase binding, and serine-type endopeptidase activity (Figure 4C; P<0.05).
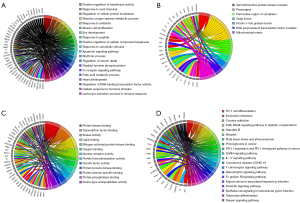
KEGG enrichment analyze
To comprehensively clarify the numerous mechanisms of AS on COVID-19, KEGG pathway enrichment analyses were conducted using org.Hs.eg.db and the clusterProfiler package of R. The primary pathology of COVID-19 is viral pneumonia with patchy inflammatory cellular infiltration and pulmonary edema. From the results of the 20 top-ranking pathways that were screened out (Figure 4D; P<0.05), those were enriched in: (I) immune- and inflammation-related signaling pathways such as T cell receptor signaling pathway, T helper 17 (Th17) cell differentiation, and interleukin-17 (IL-17) signaling pathway. (II) Cancer related pathways included proteoglycans in cancer, programmed death-ligand 1 (PD-L1), and programmed cell death protein 1 (PD-1) checkpoint pathway in cancer. (III) Virus infection-related pathways included hepatitis B, measles, herpes virus infection-associated with Kaposi sarcoma, and even directedly targeting COVID-19. Beyond that, as shown in Table 4, the Epstein-Barr virus infection, Yersinia infection, Th1 and Th2 cell differentiation, relaxin signaling pathway, human T-cell leukemia virus 1 infection, apoptosis, tumor necrosis factor (TNF) signaling pathway, MAPK signaling pathway, PI3K-Akt signaling pathway, and so on, were also significantly enriched.
Table 4
ID | Description | P value | P.adjust | Gene | Count |
---|---|---|---|---|---|
hsa04659 | Th17 cell differentiation | 4.85E-08 | 8.19E-06 | JUN/MAPK14/HSP90AA1/STAT3/MTOR/NFATC1/MAPK9/IRF4 | 8 |
hsa01522 | Endocrine resistance | 5.00E-07 | 4.23E-05 | JUN/MMP2/HBEGF/MAPK14/MTOR/MAPK9/NCOA3 | 7 |
hsa05030 | Cocaine addiction | 4.24E-06 | 0.000238848 | BDNF/JUN/SLC18A2/TH/CDK5R1 | 5 |
hsa04933 | AGE-RAGE signaling pathway in diabetic complications | 9.81E-06 | 0.000414381 | JUN/MMP2/MAPK14/STAT3/NFATC1/MAPK9 | 6 |
hsa05161 | Hepatitis B | 1.45E-05 | 0.000491039 | CCNE1/JUN/PCNA/MAPK14/STAT3/NFATC1/MAPK9 | 7 |
hsa05162 | Measles | 6.39E-05 | 0.0013971 | CCNE1/JUN/MSN/STAT3/BCL2L1/MAPK9 | 6 |
hsa05418 | Fluid shear stress and atherosclerosis | 6.39E-05 | 0.0013971 | ACVR2B/JUN/MMP2/MAPK14/HSP90AA1/MAPK9 | 6 |
hsa05205 | Proteoglycans in cancer | 6.61E-05 | 0.0013971 | MSN/MMP2/HBEGF/MAPK14/STAT3/MTOR/VAV2 | 7 |
hsa05235 | PD-L1 expression and PD-1 checkpoint pathway in cancer | 7.95E-05 | 0.001493273 | JUN/MAPK14/STAT3/MTOR/NFATC1 | 5 |
hsa04912 | GnRH signaling pathway | 9.81E-05 | 0.001585918 | JUN/MMP2/HBEGF/MAPK14/MAPK9 | 5 |
hsa04657 | IL-17 signaling pathway | 0.000103225 | 0.001585918 | JUN/MMP1/MAPK14/HSP90AA1/MAPK9 | 5 |
hsa05171 | Coronavirus disease—COVID-19 | 0.000140101 | 0.001973088 | JUN/HBEGF/MMP1/MAPK14/STAT3/F2/MAPK9 | 7 |
hsa04660 | T cell receptor signaling pathway | 0.000166623 | 0.002166098 | JUN/MAPK14/NFATC1/VAV2/MAPK9 | 5 |
hsa04722 | Neurotrophin signaling pathway | 0.00031295 | 0.003777751 | BDNF/JUN/MAPK14/NGF/MAPK9 | 5 |
hsa04664 | Fc epsilon RI signaling pathway | 0.000368061 | 0.003839622 | MAPK14/BTK/VAV2/MAPK9 | 4 |
hsa05167 | Kaposi sarcoma-associated herpesvirus infection | 0.000385726 | 0.003839622 | JUN/MAPK14/STAT3/MTOR/NFATC1/MAPK9 | 6 |
hsa04917 | Prolactin signaling pathway | 0.000411368 | 0.003839622 | MAPK14/STAT3/TH/MAPK9 | 4 |
hsa05120 | Epithelial cell signaling in Helicobacter pylori infection | 0.000411368 | 0.003839622 | JUN/HBEGF/MAPK14/MAPK9 | 4 |
hsa04380 | Osteoclast differentiation | 0.000438409 | 0.003839622 | JUN/MAPK14/BTK/NFATC1/MAPK9 | 5 |
hsa04926 | Relaxin signaling pathway | 0.000454393 | 0.003839622 | JUN/MMP2/MMP1/MAPK14/MAPK9 | 5 |
hsa05169 | Epstein-Barr virus infection | 0.00049199 | 0.00395935 | CCNE1/JUN/MAPK14/STAT3/BTK/MAPK9 | 6 |
hsa05212 | Pancreatic cancer | 0.000563112 | 0.004296484 | STAT3/MTOR/BCL2L1/MAPK9 | 4 |
hsa05135 | Yersinia infection | 0.000598652 | 0.004296484 | JUN/MAPK14/NFATC1/VAV2/MAPK9 | 5 |
hsa04915 | Estrogen signaling pathway | 0.000618852 | 0.004296484 | JUN/MMP2/HBEGF/HSP90AA1/NCOA3 | 5 |
hsa05170 | Human immunodeficiency virus 1 infection | 0.000635575 | 0.004296484 | JUN/MAPK14/MTOR/NFATC1/BCL2L1/MAPK9 | 6 |
hsa04662 | B cell receptor signaling pathway | 0.000751018 | 0.004881614 | JUN/BTK/NFATC1/VAV2 | 4 |
hsa04012 | ErbB signaling pathway | 0.000859924 | 0.005382489 | JUN/HBEGF/MTOR/MAPK9 | 4 |
hsa05219 | Bladder cancer | 0.001132067 | 0.006740745 | MMP2/HBEGF/MMP1 | 3 |
hsa04658 | Th1 and Th2 cell differentiation | 0.001156696 | 0.006740745 | JUN/MAPK14/NFATC1/MAPK9 | 4 |
hsa04530 | Tight junction | 0.001539769 | 0.008674033 | NF2/JUN/PCNA/MSN/MAPK9 | 5 |
hsa04625 | C-type lectin receptor signaling pathway | 0.001821846 | 0.009931999 | JUN/MAPK14/NFATC1/MAPK9 | 4 |
hsa04621 | NOD-like receptor signaling pathway | 0.002083434 | 0.01071649 | JUN/MAPK14/HSP90AA1/BCL2L1/MAPK9 | 5 |
hsa04931 | Insulin resistance | 0.002092569 | 0.01071649 | PTPN1/STAT3/MTOR/MAPK9 | 4 |
hsa05145 | Toxoplasmosis | 0.002389993 | 0.01187967 | MAPK14/STAT3/BCL2L1/MAPK9 | 4 |
hsa04670 | Leukocyte transendothelial migration | 0.002549124 | 0.01230863 | MSN/MMP2/MAPK14/VAV2 | 4 |
hsa04935 | Growth hormone synthesis, secretion and action | 0.002978636 | 0.01398304 | MAPK14/STAT3/MTOR/MAPK9 | 4 |
hsa04010 | MAPK signaling pathway | 0.00339358 | 0.015500405 | BDNF/JUN/MAPK14/NFATC1/NGF/MAPK9 | 6 |
hsa00140 | Steroid hormone biosynthesis | 0.003562811 | 0.015845134 | CYP3A4/HSD11B1/HSD11B2 | 3 |
hsa04068 | FoxO signaling pathway | 0.004206855 | 0.01781729 | MAPK14/STAT3/MAPK9/CAT | 4 |
hsa05321 | Inflammatory bowel disease | 0.004263871 | 0.01781729 | JUN/STAT3/NFATC1 | 3 |
hsa04728 | Dopaminergic synapse | 0.004322538 | 0.01781729 | MAPK14/SLC18A2/TH/MAPK9 | 4 |
hsa04024 | cAMP signaling pathway | 0.004468315 | 0.017979648 | BDNF/JUN/NFATC1/VAV2/MAPK9 | 5 |
hsa05166 | Human T-cell leukemia virus 1 infection | 0.004737377 | 0.018134522 | CCNE1/JUN/NFATC1/BCL2L1/MAPK9 | 5 |
hsa04210 | Apoptosis | 0.004806845 | 0.018134522 | JUN/NGF/BCL2L1/MAPK9 | 4 |
hsa04137 | Mitophagy - animal | 0.004840979 | 0.018134522 | JUN/BCL2L1/MAPK9 | 3 |
hsa04920 | Adipocytokine signaling pathway | 0.005043329 | 0.018134522 | STAT3/MTOR/MAPK9 | 3 |
hsa05031 | Amphetamine addiction | 0.005043329 | 0.018134522 | JUN/SLC18A2/TH | 3 |
hsa05133 | Pertussis | 0.006603137 | 0.023248544 | JUN/MAPK14/MAPK9 | 3 |
hsa01521 | EGFR tyrosine kinase inhibitor resistance | 0.007350205 | 0.025350707 | STAT3/MTOR/BCL2L1 | 3 |
hsa05131 | Shigellosis | 0.007703447 | 0.025786816 | JUN/MAPK14/MTOR/BCL2L1/MAPK9 | 5 |
hsa04218 | Cellular senescence | 0.00778182 | 0.025786816 | CCNE1/MAPK14/MTOR/NFATC1 | 4 |
hsa04151 | PI3K-Akt signaling pathway | 0.008333712 | 0.026853609 | BDNF/CCNE1/HSP90AA1/MTOR/NGF/BCL2L1 | 6 |
hsa05204 | Chemical carcinogenesis | 0.008421546 | 0.026853609 | CYP3A4/HSD11B1/CYP2C19 | 3 |
hsa05022 | Pathways of neurodegeneration - multiple diseases | 0.009155285 | 0.028246247 | BDNF/MAPK14/MTOR/BCL2L1/MAPK9/CDK5R1/CAT | 7 |
hsa05210 | Colorectal cancer | 0.009282394 | 0.028246247 | JUN/MTOR/MAPK9 | 3 |
hsa00591 | Linoleic acid metabolism | 0.009359703 | 0.028246247 | CYP3A4/CYP2C19 | 2 |
hsa05222 | Small cell lung cancer | 0.011154207 | 0.032999848 | RARB/CCNE1/BCL2L1 | 3 |
hsa04215 | Apoptosis - multiple species | 0.011325392 | 0.032999848 | BCL2L1/MAPK9 | 2 |
hsa05215 | Prostate cancer | 0.012869454 | 0.036652662 | CCNE1/HSP90AA1/MTOR | 3 |
hsa04750 | Inflammatory mediator regulation of TRP channels | 0.013229659 | 0.036652662 | MAPK14/NGF/MAPK9 | 3 |
hsa05231 | Choline metabolism in cancer | 0.013229659 | 0.036652662 | JUN/MTOR/MAPK9 | 3 |
hsa04914 | Progesterone-mediated oocyte maturation | 0.013967343 | 0.038072273 | MAPK14/HSP90AA1/MAPK9 | 3 |
hsa05142 | Chagas disease | 0.014728143 | 0.039508827 | JUN/MAPK14/MAPK9 | 3 |
hsa04960 | Aldosterone-regulated sodium reabsorption | 0.014966286 | 0.039520349 | HSD11B2/NR3C2 | 2 |
hsa04620 | Toll-like receptor signaling pathway | 0.015512154 | 0.040331601 | JUN/MAPK14/MAPK9 | 3 |
hsa05130 | Pathogenic Escherichia coli infection | 0.017191602 | 0.044020921 | JUN/MAPK14/F2/MAPK9 | 4 |
hsa04668 | TNF signaling pathway | 0.018881874 | 0.047627414 | JUN/MAPK14/MAPK9 | 3 |
KEGG, Kyoto Encyclopedia of Genes and Genomes.
Results of molecular docking
The intersection of the top 16 genes in the disease-target-drug network and the top 16 genes in the KEGG pathways were taken for molecular docking. The result showed that AS had a strong affinity with the chosen proteins STAT3, JUN, MAPK14, MTOR, MMP2, HSP90AA1, MAPK9, BTK, and NFATC1. As shown in Figure 5, the drug and target proteins could form a stable complex by binding different hydrogen bonds with the residues at very close distance.
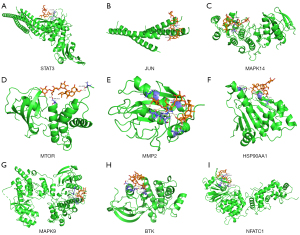
Discussion
The COVID-19 pandemic is a public health emergency causing worldwide concern, which has had an enormous impact on the global health economy (32). The pathogenesis of COVID-19 is extremely complicated. Patients who are susceptible to this pathogenic coronavirus face a risk of developing many fatal complications, such as organ failure, pulmonary edema, septic shock, severe pneumonia, and ARDS (33,34). However, a drug that can effectively treat COVID-19 has not yet been identified.
When exposed to human coronaviruses pathogens, the host immune system reacts immediately by triggering related defense mechanisms to achieve a more efficacious shield. This process can be characterized by the increase of inflammatory cytokines and chemokines (35). The deteriorated clinical presentation of COVID-19 is also associated with arrantly elevated pro-inflammatory cytokines, including IL-1β, IL-6, IL-8, and IL-17 (36). As reported, Chinese medicine with anti-inflammatory function has exhibited a tremendous beneficial effect in treating COVID-19 by markedly relieving primary symptoms like fever and cough, and could accelerate recovery (7). For example, Lian Hua Qing Wen notably inhibited the replication of SARS-CoV-2 in Vero E6 cells as well as reduced pro-inflammatory cytokine TNF-α and IL-6 expression at the messenger RNA (mRNA) level (37). Shen Fu injection has been shown to reduce the lung inflammation and decrease the expression levels of IL-1β and IL-6 in COVID-19 patients (9).
AS, which is well tolerated with minimal side effects and superior efficacy as many other herbal medicines, has a broad-spectrum anti-inflammatory and anti-oxidant effect and has the functions of clearing heat, detoxifying dampness, and relieving asthma (11,12,15). Moreover, it is likely to have value as a potential intervention for ARDS (38).
In this study, 45 potential co-targets (Table 3) and 67 significantly enriched signaling pathways (Table 4) were identified by network pharmacology in treating COVID-19 with AS, among which many were involved in the cytokine storms and ARDS. The results of the PPI network showed that AS might weaken cytokine storms by regulating STAT3, JUN, MAPK14, mTOR, MMP2, HSP90AA1, MAPK9, BTK, NFATC1, and other genes (Figure 3). Among them, mTOR is a critical factor elevated during a cytokine storm of COVID-19 and participates in cell metabolism and proliferation (39). As reported, AS has an influence on the treatment of H5N1/H1N1 by inhibiting the mTOR pathway (17), which shows the potential of AS in treating COVID-19 by inhibition of mTOR.
The KEGG enrichment analysis indicated that the core targets were mostly enriched in inflammation- and immune-related signaling pathways: Th17 cell differentiation, IL-17, TNF, MAPK, and the PI3K-Akt signaling pathway. Previous studies have demonstrated that the MAPK signaling pathway contributes to the development of ARDS (40). Currently, the MAPK signaling pathway is considered to be deeply involved in the COVID-19 pathogenesis (41). Our analysis showed the hub genes of JUN, MAPK14, and MAPK9 were involved in the MAPK signaling pathway. It was reported AS exhibited extraordinary anti-inflammatory activity by inhibiting the MAPK pathways (42). The results indicated that the synergistic therapy of AS inhibited COVID-19 partly by suppressing the MAPK pathways. The STAT3 gene plays a significant part in inflammation control and immunity (43). In cells infected by SARS-CoV-2, increasing STAT3 induced the secretion of pro-inflammatory cytokines and chemokines, which results in the infection of regional endothelial cells (44). It has been reported that AS attenuates neonatal hypoxic-ischemic brain damage by suppressing the STAT3 pathway (45), which indicates that AS could silence COVID-19 by attenuating the STAT3 signaling pathway. In the process of cytokine storm, the up-regulation of Th17 and Th17 cell cytokine IL-17A is mainly responsible for the immunopathology of COVID-19 and ARDS (36). Also, the activation of STAT3 leads to the deposition of IL-17 (46). Growing evidence indicates that the host Th17 inflammatory responses contribute to the severe lung pathology and induce the mortality of lower airway infection of coronaviruses (47). Researchers have suggested targeting IL-17A signaling to manage COVID-19 patients by effectively inhibiting cytokine storm syndrome (48). Our network analysis demonstrated that AS could regulate Th17 and the IL-17A signaling pathway to exert a therapeutically beneficial effect on COVID-19. The PI3K/Akt signaling pathway regulates the release of inflammatory transmitters in inflammatory response in the lungs and airways (49). The latest studies demonstrated that during the SARS-CoV-2 infection, the PI3K/Akt/mTOR pathway was activated in a dose-dependent manner which activated cell apoptosis process (50). A previous study found that AS was beneficial to the treatment of diabetes-associated cognitive deficits through inhibiting the PI3K/Akt/NF-κB pathway (51). We conjectured that AS regulates the PI3K-Akt signaling pathway to potentiate the healing of COVID-19 (52). Severe oxidative stress triggered by SARS-CoV-2 can aggravate the severity of COVID-19 (53). Previous studies have demonstrated the anti-oxidation and anti-inflammatory effect of a homologous agent of AS by increasing the expression level of anti-oxidant factors and decreasing inflammatory factors IL-6 and IL-17 (54). Such findings might imply AS participates in the treatment of COVID-19 through its anti-inflammation and anti-oxidant abilities. The TNF signaling pathway is a critical pathway in the inflammatory response, which could also induce apoptosis and oxidative stress (55). Additionally, AS could promote cell growth and attenuate cell apoptosis by inhibiting the TNF signaling pathway (56).
Furthermore, clinical data indicate that SARS-CoV-2 infection is related to neurological and neuropsychiatric disease, including encephalopathies, encephalitis, acute disseminated encephalomyelitis, and inflammatory central nervous system (CNS) syndromes (57). It has been found that AS protects against neurotoxicity and accelerates nerve regeneration (58). It has been applied as a psychoactive drug for memory enhancement and in India for a long time (59). As shown in Figure 4, AS has a potential neuroprotective effect in COVID-19 by regulating the neurotrophin signaling pathway. Human coronaviruses could regulate numerous cellular processes such as apoptosis (60). In our research, the co-target genes enriched in the apoptosis pathway indicated that AS could exert a regulatory effect on COVID-19 through this pathway. Moreover, AS could even directedly act on the coronavirus disease-COVID-19 pathway. These features indicate that AS is an attractive potential drug for COVID-19 treatment.
In molecular docking, AS showed a strong affinity with these co-core proteins, indicating that AS might indeed exert a potent role in treating SARS-CoV-2 by effectively targeting the core proteins and the corresponding pathway involved. This study has presented a comprehensive and systematic understanding of the potential curative mechanism of AS in the treatment of COVID-19.
Conclusions
This study systematically explored the potential mechanism of AS and found it has the possibility to treat COVID-19 through numerous targets and pathways, mainly associated with immune regulation and the inflammatory response. However, there are limitations of network pharmacology concerning its prediction. These potential targets and pathways predicted by network pharmacology tools and bioinformatic techniques need to be confirmed by further experimental evidence.
Acknowledgments
Funding: The study was supported by the Interdisciplinary Research Program of the Shanghai Ninth People’s Hospital, Shanghai Jiao Tong University School of Medicine (No. YG2019QNA13), and the National Natural Science Foundation of China (No. 82104485).
Footnote
Conflicts of Interest: All authors have completed the ICMJE uniform disclosure form (available at https://atm.amegroups.com/article/view/10.21037/atm-22-51/coif). The authors have no conflicts of interest to declare.
Ethical Statement: The authors are accountable for all aspects of the work in ensuring that questions related to the accuracy or integrity of any part of the work are appropriately investigated and resolved. The study was conducted in accordance with the Declaration of Helsinki (as revised in 2013).
Open Access Statement: This is an Open Access article distributed in accordance with the Creative Commons Attribution-NonCommercial-NoDerivs 4.0 International License (CC BY-NC-ND 4.0), which permits the non-commercial replication and distribution of the article with the strict proviso that no changes or edits are made and the original work is properly cited (including links to both the formal publication through the relevant DOI and the license). See: https://creativecommons.org/licenses/by-nc-nd/4.0/.
References
- Drosten C, Günther S, Preiser W, et al. Identification of a novel coronavirus in patients with severe acute respiratory syndrome. N Engl J Med 2003;348:1967-76. [Crossref] [PubMed]
- Zaki AM, van Boheemen S, Bestebroer TM, et al. Isolation of a novel coronavirus from a man with pneumonia in Saudi Arabia. N Engl J Med 2012;367:1814-20. [Crossref] [PubMed]
- Cui J, Li F, Shi ZL. Origin and evolution of pathogenic coronaviruses. Nat Rev Microbiol 2019;17:181-92. [Crossref] [PubMed]
- Su J, Liu Z, Liu C, et al. Network Pharmacology Integrated Molecular Docking Reveals the Mechanism of Anisodamine Hydrobromide Injection against Novel Coronavirus Pneumonia. Evid Based Complement Alternat Med 2020;2020:5818107. [Crossref] [PubMed]
- Tao Q, Du J, Li X, et al. Network pharmacology and molecular docking analysis on molecular targets and mechanisms of Huashi Baidu formula in the treatment of COVID-19. Drug Dev Ind Pharm 2020;46:1345-53. [Crossref] [PubMed]
- Li Y, Liu X, Guo L, et al. Traditional Chinese herbal medicine for treating novel coronavirus (COVID-19) pneumonia: protocol for a systematic review and meta-analysis. Syst Rev 2020;9:75. [Crossref] [PubMed]
- Yang Y, Islam MS, Wang J, et al. Traditional Chinese Medicine in the Treatment of Patients Infected with 2019-New Coronavirus (SARS-CoV-2): A Review and Perspective. Int J Biol Sci 2020;16:1708-17. [Crossref] [PubMed]
- Ksiazek TG, Erdman D, Goldsmith CS, et al. A novel coronavirus associated with severe acute respiratory syndrome. N Engl J Med 2003;348:1953-66. [Crossref] [PubMed]
- Zhang D, Zhang B, Lv JT, et al. The clinical benefits of Chinese patent medicines against COVID-19 based on current evidence. Pharmacol Res 2020;157:104882.
- Ju-Lin X, Shao-Hai Q, Tian-Zeng L, et al. Effect of asiaticoside on hypertrophic scar in the rabbit ear model. J Cutan Pathol 2009;36:234-9. [Crossref] [PubMed]
- Arora R, Kumar R, Agarwal A, et al. Comparison of three different extracts of Centella asiatica for anti-amnesic, antioxidant and anticholinergic activities: in vitro and in vivo study. Biomed Pharmacother 2018;105:1344-52. [Crossref] [PubMed]
- Wan J, Gong X, Jiang R, et al. Antipyretic and anti-inflammatory effects of asiaticoside in lipopolysaccharide-treated rat through up-regulation of heme oxygenase-1. Phytother Res 2013;27:1136-42. [Crossref] [PubMed]
- Lee JH, Kim HL, Lee MH, et al. Asiaticoside enhances normal human skin cell migration, attachment and growth in vitro wound healing model. Phytomedicine 2012;19:1223-7. [Crossref] [PubMed]
- Qiu J, Yu L, Zhang X, et al. Asiaticoside attenuates lipopolysaccharide-induced acute lung injury via down-regulation of NF-κB signaling pathway. Int Immunopharmacol 2015;26:181-7. [Crossref] [PubMed]
- Al-Saeedi FJ. Study of the cytotoxicity of asiaticoside on rats and tumour cells. BMC Cancer 2014;14:220. [Crossref] [PubMed]
- An X, Sun X, Hou Y, et al. Protective effect of oxytocin on LPS-induced acute lung injury in mice. Sci Rep 2019;9:2836. [Crossref] [PubMed]
- Zulkipli NN, Zakaria R, Long I, et al. In Silico Analyses and Cytotoxicity Study of Asiaticoside and Asiatic Acid from Malaysian Plant as Potential mTOR Inhibitors. Molecules 2020;25:3991. [Crossref] [PubMed]
- Yuan H, Ma Q, Cui H, et al. How Can Synergism of Traditional Medicines Benefit from Network Pharmacology? Molecules 2017;22:1135. [Crossref] [PubMed]
- Boezio B, Audouze K, Ducrot P, et al. Network-based Approaches in Pharmacology. Mol Inform 2017; [Crossref] [PubMed]
- Saikia S, Bordoloi M. Molecular Docking: Challenges, Advances and its Use in Drug Discovery Perspective. Curr Drug Targets 2019;20:501-21. [Crossref] [PubMed]
- Daina A, Michielin O, Zoete V. SwissTargetPrediction: updated data and new features for efficient prediction of protein targets of small molecules. Nucleic Acids Res 2019;47:W357-64. [Crossref] [PubMed]
- Huang H, Zhang G, Zhou Y, et al. Reverse Screening Methods to Search for the Protein Targets of Chemopreventive Compounds. Front Chem 2018;6:138. [Crossref] [PubMed]
- Davis AP, Grondin CJ, Johnson RJ, et al. The Comparative Toxicogenomics Database: update 2017. Nucleic Acids Res 2017;45:D972-8. [Crossref] [PubMed]
- Paolacci S, Precone V, Acquaviva F, et al. Genetics of lipedema: new perspectives on genetic research and molecular diagnoses. Eur Rev Med Pharmacol Sci 2019;23:5581-94. [PubMed]
- Amberger JS, Bocchini CA, Schiettecatte F, et al. OMIM.org: Online Mendelian Inheritance in Man (OMIM®), an online catalog of human genes and genetic disorders. Nucleic Acids Res 2015;43:D789-98. [Crossref] [PubMed]
- Kohl M, Wiese S, Warscheid B. Cytoscape: software for visualization and analysis of biological networks. Methods Mol Biol 2011;696:291-303. [Crossref] [PubMed]
- Tang S, Jing H, Huang Z, et al. Identification of key candidate genes in neuropathic pain by integrated bioinformatic analysis. J Cell Biochem 2020;121:1635-48. [Crossref] [PubMed]
- Zhou Y, Zhou B, Pache L, et al. Metascape provides a biologist-oriented resource for the analysis of systems-level datasets. Nat Commun 2019;10:1523. [Crossref] [PubMed]
- Kanehisa M, Goto S, Kawashima S, et al. The KEGG resource for deciphering the genome. Nucleic Acids Res 2004;32:D277-80. [Crossref] [PubMed]
- Yu G, Wang LG, Han Y, et al. clusterProfiler: an R package for comparing biological themes among gene clusters. OMICS 2012;16:284-7. [Crossref] [PubMed]
- Zhang C, Zheng Y, Li X, et al. Genome-wide mutation profiling and related risk signature for prognosis of papillary renal cell carcinoma. Ann Transl Med 2019;7:427. [Crossref] [PubMed]
- Xia QD, Xun Y, Lu JL, et al. Network pharmacology and molecular docking analyses on Lianhua Qingwen capsule indicate Akt1 is a potential target to treat and prevent COVID-19. Cell Prolif 2020;53:e12949. [Crossref] [PubMed]
- Rasmussen SA, Smulian JC, Lednicky JA, et al. Coronavirus Disease 2019 (COVID-19) and pregnancy: what obstetricians need to know. Am J Obstet Gynecol 2020;222:415-26. [Crossref] [PubMed]
- Chen N, Zhou M, Dong X, et al. Epidemiological and clinical characteristics of 99 cases of 2019 novel coronavirus pneumonia in Wuhan, China: a descriptive study. Lancet 2020;395:507-13. [Crossref] [PubMed]
- Kong Q, Wu Y, Gu Y, et al. Analysis of the molecular mechanism of Pudilan (PDL) treatment for COVID-19 by network pharmacology tools. Biomed Pharmacother 2020;128:110316. [Crossref] [PubMed]
- Shibabaw T. Inflammatory Cytokine: IL-17A Signaling Pathway in Patients Present with COVID-19 and Current Treatment Strategy. J Inflamm Res 2020;13:673-80. [Crossref] [PubMed]
- Deng W, Xu Y, Kong Q, et al. Therapeutic efficacy of Pudilan Xiaoyan Oral Liquid (PDL) for COVID-19 in vitro and in vivo. Signal Transduct Target Ther 2020;5:66. [Crossref] [PubMed]
- Peiseler M, Kubes P. Macrophages play an essential role in trauma-induced sterile inflammation and tissue repair. Eur J Trauma Emerg Surg 2018;44:335-49. [Crossref] [PubMed]
- Ramaiah MJ. mTOR inhibition and p53 activation, microRNAs: The possible therapy against pandemic COVID-19. Gene Rep 2020;20:100765. Erratum in: Gene Rep 2021;24:101054. [Crossref] [PubMed]
- Mizutani T, Fukushi S, Saijo M, et al. Phosphorylation of p38 MAPK and its downstream targets in SARS coronavirus-infected cells. Biochem Biophys Res Commun 2004;319:1228-34. [Crossref] [PubMed]
- Grimes JM, Grimes KV. p38 MAPK inhibition: A promising therapeutic approach for COVID-19. J Mol Cell Cardiol 2020;144:63-5. [Crossref] [PubMed]
- Zhang C, Chen S, Zhang Z, et al. Asiaticoside Alleviates Cerebral Ischemia-Reperfusion Injury via NOD2/Mitogen-Activated Protein Kinase (MAPK)/Nuclear Factor kappa B (NF-κB) Signaling Pathway. Med Sci Monit 2020;26:e920325. [Crossref] [PubMed]
- Fu XY. STAT3 in immune responses and inflammatory bowel diseases. Cell Res 2006;16:214-9. [Crossref] [PubMed]
- Matsuyama T, Kubli SP, Yoshinaga SK, et al. An aberrant STAT pathway is central to COVID-19. Cell Death Differ 2020;27:3209-25. [Crossref] [PubMed]
- Zhou Y, Wang S, Zhao J, et al. Asiaticoside attenuates neonatal hypoxic-ischemic brain damage through inhibiting TLR4/NF-κB/STAT3 pathway. Ann Transl Med 2020;8:641. [Crossref] [PubMed]
- Milovanovic J, Arsenijevic A, Stojanovic B, et al. Interleukin-17 in Chronic Inflammatory Neurological Diseases. Front Immunol 2020;11:947. [Crossref] [PubMed]
- Hotez PJ, Bottazzi ME, Corry DB. The potential role of Th17 immune responses in coronavirus immunopathology and vaccine-induced immune enhancement. Microbes Infect 2020;22:165-7. [Crossref] [PubMed]
- Pacha O, Sallman MA, Evans SE. COVID-19: a case for inhibiting IL-17? Nat Rev Immunol 2020;20:345-6. [Crossref] [PubMed]
- Lin J, Li J, Shu M, et al. The rCC16 Protein Protects Against LPS-Induced Cell Apoptosis and Inflammatory Responses in Human Lung Pneumocytes. Front Pharmacol 2020;11:1060. [Crossref] [PubMed]
- Appelberg S, Gupta S, Svensson Akusjärvi S, et al. Dysregulation in Akt/mTOR/HIF-1 signaling identified by proteo-transcriptomics of SARS-CoV-2 infected cells. Emerg Microbes Infect 2020;9:1748-60. [Crossref] [PubMed]
- Yin Z, Yu H, Chen S, et al. Asiaticoside attenuates diabetes-induced cognition deficits by regulating PI3K/Akt/NF-κB pathway. Behav Brain Res 2015;292:288-99. [Crossref] [PubMed]
- Khezri MR. PI3K/AKT signaling pathway: a possible target for adjuvant therapy in COVID-19. Hum Cell 2021;34:700-1. [Crossref] [PubMed]
- Rowaiye AB, Onuh OA, Oli AN, et al. The pandemic COVID-19: a tale of viremia, cellular oxidation and immune dysfunction. Pan Afr Med J 2020;36:188. [Crossref] [PubMed]
- Huang J, Tu T, Wang W, et al. Asiatic Acid Glucosamine Salt Alleviates Ultraviolet B-induced Photoaging of Human Dermal Fibroblasts and Nude Mouse Skin. Photochem Photobiol 2020;96:124-38. [Crossref] [PubMed]
- Han D, Ybanez MD, Ahmadi S, et al. Redox regulation of tumor necrosis factor signaling. Antioxid Redox Signal 2009;11:2245-63. [Crossref] [PubMed]
- Song D, Jiang X, Liu Y, et al. Asiaticoside Attenuates Cell Growth Inhibition and Apoptosis Induced by Aβ1-42 via Inhibiting the TLR4/NF-κB Signaling Pathway in Human Brain Microvascular Endothelial Cells. Front Pharmacol 2018;9:28. [Crossref] [PubMed]
- Paterson RW, Brown RL, Benjamin L, et al. The emerging spectrum of COVID-19 neurology: clinical, radiological and laboratory findings. Brain 2020;143:3104-20. [Crossref] [PubMed]
- Qi FY, Yang L, Tian Z, et al. Neuroprotective effects of Asiaticoside. Neural Regen Res 2014;9:1275-82. [Crossref] [PubMed]
- Chen S, Yin ZJ, Jiang C, et al. Asiaticoside attenuates memory impairment induced by transient cerebral ischemia-reperfusion in mice through anti-inflammatory mechanism. Pharmacol Biochem Behav 2014;122:7-15. [Crossref] [PubMed]
- Lim YX, Ng YL, Tam JP, et al. Human Coronaviruses: A Review of Virus-Host Interactions. Diseases 2016;4:26. [Crossref] [PubMed]
(English Language Editor: J. Jones)