Population pharmacokinetics of polymyxin B: a systematic review
Introduction
In recent years, the problem of carbapenem-resistant organisms (CROs) has become increasingly prominent. According to the data from the China Antimicrobial Resistance Surveillance System (CARSS) (1), the resistance rate of CROs increased year by year from 2014 to 2019, the resistance rate of Klebsiella pneumoniae to carbapenems increased more than one fold, and the resistance rate of Acinetobacter baumannii to carbapenems was as high as 54.4–59.8%. Due to their narrow antibacterial spectrum and significant side effects, polymyxins were replaced by new antimicrobial agents after they entered the market in the 1850s. However, due to growing pressure related to CROs infection, polymyxins have re-captured the attention of clinical experts due to their above-mentioned strong susceptibility to resistant bacteria. Thus, polymyxins have become the last line of defense for the treatment of CROs (2,3).
Among the 5 known polymyxin subtypes, polymyxin B (PMB) has direct antibacterial activity, and the treatment regimen has a low degree of dependence on renal function and is widely used in clinical treatment in China. PMB is a sulfate preparation formed by more than 30 peptides, of which PMB1 and PMB2 sulfate are the main components, and account for about 85% of PMB sulfate, and the rest include PMB3 and PMBI-1 (see Figure 1) (4). PMB can be administered intravascularly, intrathecally, or by nebulization, with a steady-state volume of distribution of approximately 12.7–34.3 L and a half-life of approximately 9–11.5 h after intravenous administration (5-7). The elimination mechanisms involve both renal and non-renal routes (5-7). Numerous studies have shown that the urinary recovery of PMB is low (<5%) in both animals and humans; thus non-renal clearance is considered the major route of clearance for PMB (5-7). Selective uptake and retention are present in PMB tubular epithelial cells and preferentially accumulate in renal tissue (7,8). In non-renal clearance, there is a certain proportion of biliary excretion (6), and other routes require further study.
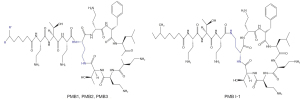
PMB is a concentration-dependent antibiotic with a short post-antibiotic effect, and bacteria can grow and multiply quickly when the PMB concentration is reduced below the effective inhibitory concentration. Further, neurotoxicity and PMB-induced nephrotoxicity are mainly characterized by acute kidney injury (AKI) and acute tubular necrosis, while PMB-induced neurotoxicity is characterized by paresthesia and respiratory depression. In 2019, several international institutions and academic organizations jointly issued the international consensus guidelines for optimal use of polymyxins (9). These guidelines recommend an area under the curve at steady state (AUCss,0–24 h) of 50–100 mg·h/L for the therapeutic drug concentration of PMB, which is equivalent to a steady-state plasma concentration (Css) of 2–4 mg/L. If PMB exceeds this range, the incidence and severity of AKI increase significantly.
In 2016, the American Society of Infectious Diseases and the American Thoracic Society jointly issued the guidelines for nosocomial pneumonia and ventilator-associated pneumonia (10), in which they emphasized that antibacterial drugs should be used according to pharmacokinetics/pharmacodynamics (PK/PD). Under the current increasingly severe epidemic of CROs, the clinical application of PK/PD theory is a powerful means for ensuring the maximum therapeutic benefit of PMB. However, the process of antibacterial drug disposition differs between patients with severe infections and patients from special populations and healthy individuals (11-13). In order to fully study the pharmacokinetic differences due to physiological, pathological, and genetic factors and adjust the dose and improve the effect of drug treatment, population pharmacokinetics (PPK) has emerged. The medication method can be optimized to achieve individualized dosing by combining PPK model-PD metrics with Bayesian methods (14).
Over the last decades, several PPK studies on PMB have been conducted. This review systematically compared the published PMB PPK models and explored the covariates that have been shown to affect PMB PK models.
We present the following article in accordance with the PRISMA reporting checklist (available at https://atm.amegroups.com/article/view/10.21037/atm-22-236/rc).
Methods
Search strategy
Data for this review were identified by the systemic review of publications listed in the PubMed and Embase databases from the inception of the databases to August 2021 using the following search terms: “Polymyxin B” AND (“populationpharmacokinetic” OR “pharmacometrics” OR “pharmacokineticmodel” OR “popPK” OR “popPK” OR “PPK” OR “nonlinear mixed effect model” OR “NONMEM”). Additional publications were identified by reviewing the reference lists of the articles identified in the search.
Inclusion/exclusion criteria
Original studies describing PPK models for PMB were included in the review. Reviews, methodological articles, in-vitro and animal studies, studies that used previously described pharmacokinetic models and those that involved non-compartmental analyses were excluded. The selection process is described in Figure 2.
Data extraction
Data extraction was carried by two authors independently, and discrepancies were solved by discussion. The following information was recorded from the identified: first author, year of publication, patient numbers and characteristics, treatment regimen and determination method of PMB, structural and statistical models, tested and retained covariates, and model evaluation method.
Results
Literature search
Eighty-six relevant articles were initially retrieved, and 42 duplicates were removed. After screening the titles and abstracts, 10 articles published between 2008 and 2021 were ultimately included in the review. Figure 2 shows the selection process. The following data were extracted from the included articles the publication year, sample size, patient characteristics, dosing regimen, test objects, and methods (see Table 1). Three hundred and twenty-nine patients (range, 9–70; median, 33.5) were included in the review. The patients’ ages ranged from 16–94 years; only 1 patient was aged 16 years old; the others were adult patients aged 18 years and older. The characteristics of the study subjects included infections caused by multi-drug-resistant bacteria, such as respiratory tract infections, sepsis, and abdominal infections. Most studies excluded patients receiving continuous renal replacement therapy (CRRT) and extracorporeal membrane oxygenation (ECMO) support. Only 1 study included 2 patients receiving CRRT.
Table 1
Study | Site | N (male/female) | Age (year) | Weight (kg) | Renal function index | Subject characteristicsb | Dose regimen | Assay |
---|---|---|---|---|---|---|---|---|
2021 Yu (15) | China | 32 (26/6) | 63.63±12.92 | 61.73±11.77 | Scr: 116.69±127.60 μmol/L | Adult critically ill patients (respiratory tract, sepsis/bacteremia, intra-abdominal infections) | 100–200 mg/day (1.04–3.45 mg/kg/day) | PMB1, PMB2; plasma; LC-MS/MS |
2021 Crass (16) | America | 9 (8/1) | 31±12.5 | 58±11.1 | CrCL: 112±16 mL/min | Adult CF patients | TBW ≥40 kg, 75 mg Q12H; TBW <40 kg, 50 mg Q12H; infusion time: 1–2 h | PMB1, PMB2; plasma; LC-MS/MS |
2021 Wang (17) | China | 70 (58/12) | 47.3±17.7; 54.2±17.5 | 68.6±11.6; 66.9±11.1 | Normal renal function: Scr: 50.0 [21–116] μmol/L; renal insufficiency Scr: 163 [77.7–387] μmol/L | Adult critically ill patients (respiratory tract, sepsis/bacteremia, intra-abdominal infections) | Loading dose: 100–150 mg; maintenance dose: 50–100 mg Q12H; infusion time: 1 h | PMB1, PMB2; plasma; LC-MS/MS |
2020 Wang (18) | China | 46 (39/7) | 46 [18–94] | 70 [45–98] | Scr: 73.0 [21.0–387.0] μmol/L | MDR gram-negative bacterial infection patients | 50–100 mg Q12H; infusion time: 0.5–2 h | PMB1, PMB2; plasma; LC-MS/MS |
2018 Manchandani (19) | America | 35 (23/12) | 58.7±15.1 | 57.7±15.6 | CrCL: 66.8±42.4 mL/min | MDR gram-negative bacterial infection patients | 65–240 mg/day (1.3–2.8 mg/kg/day); infusion time: 1–3 h | PMB1, PMB2, PMB3, PMB I-1; plasma; LC-MS/MS |
2018 Miglis (20) | America | 52 (33/19) | 47 | 73 [30–122] | CrCL: 68 [16–389] mL/min | MDR gram-negative bacterial infection patients | Loading dose: 90–350 mg/day; maintenance dose: 20–360 mg/day (0.34–3.45 mg/kg/day) | PMB1, PMB2; plasma; LC-MS/MS |
2018 Kubin (21) | America | 43 (30/13) | 58 [39–69] | 78 [59–95] | CrCL: 73 [40–113] mL/min | MDR gram-negative bacterial infection patients | 120–240 mg/day (1.5–3.0 mg/kg/day); infusion time ≥1 h | PMB1, PMB2; plasma; LC-MS/MS |
2018 Avedissian (22) | America | 9 (6/3) | CF group: 32 [25–44.5]; non-CF group: 55.5 [40.5–69] | CF group: 57 [52.15–62.05]; non-CF group: 74.33 [57.88–92.03] | CF group: CrCL: 105 [74–118] mL/min; non-CF group: CrCL: 73 [42.25–116.5] mL/min | Adult CF patients | Loading dose: 2.67–3.05 mg/kg/day; maintenance dose: 55.8–130 mg/day (1.43–1.65 mg/kg/day) | PMB1, PMB2; plasma; LC-MS/MS |
2013 Sandri (23) | Brazil | 24 (13/11) | 61.5 [21–87] | 62.5 [41–250] | CrCL: 33 [10–143] mL/min | Critically ill patients | 0.45–3.38 mg/kg/day; infusion time: 1–4 h | PMB1, PMB2; plasma; LC-MS/MS |
2008 Kwa (24) | Singapore | 9 (8/1) | 42.9±17.9 | 65.6±12.8 | Scr: 1.0±0.3 mg/dL | MDR gram-negative bacterial infection patients | 20–150 mg/day (0.13–1.97 mg/kg/day; infusion time: 2–6 h | PMB1; plasma; LC-MS/MS |
a, values are median [range] or mean ± SD. CrCL was estimated from serum creatinine using the C-G equation; b, patients with CRRT, ECMO, or other rapid fluctuations in renal function were excluded. CF, cystic fibrosis; Scr, serum creatine; CrCL, creatinine clearance; MDR, multi-drug resistant; TBW, total body weight; Q12H, every 12 hours; PMB, polymyxin B; C-G, Cockcroft-Gault; CRRT, continuous renal replacement therapy; ECMO, extracorporeal membrane oxygenation.
Analytical methods used in pharmacokinetic model development
In relation to the test specimens collected, blood was collected in all studies, and urine was also collected in 2 studies for the study of renal excretion recovery. In relation to the test methods, LC-MS/MS was used in all studies. In relation to the blood samples, plasma was collected in most studies, and serum was collected for PMB testing in 1 study. Most of the studies quantitatively calculated PMB concentration as the sum of PMB1 and PMB2, but 1 study only used PMB1 as the concentration of PMB, and 1 study used the sum of PMB1, PMB2, PMB3, and PMB I-1.
The dosing regimen for patients was complex. In 7 studies, clinicians administered loading doses or directly administered maintenance doses based on clinical experience and instructions or guidelines. In these 7 studies, the dosing regimen was 0.13–3.45 mg/kg/day. While 3 studies administered fixed doses, with maintenance doses of 50–100 mg twice a day, and 1 of the three study administered a loading dose of 100–150 mg. The number of measured concentrations ranged from 19 to 462 (median, 136.5), and an average of 4.9 samples was measured per patient. The fitting software included NONMEM, PhoenxiNLME, ADAPT5, Pmetrics, Monolix, and S-ADAPT.
Structural pharmacokinetic models
The reported model structure, pharmacokinetic parameters, and covariates, and final model are summarized in Table 2. Of the 10 studies included in the review, 5 used a 1-compartment model, and 5 used a 2-compartment model, and all followed a 0-order input, first-order linear elimination. The median values of total clearance (CL) and corresponding inter-individual variability (IIV) were 2.43 L/h (1.58–4.3 L/h) and 29.80% (13.0–53.63%), respectively. In the review, the median (range) estimated value of the distribution volume (V) and corresponding IIV using 1-compartment model was 29.8 L (12.7–47.2 L) and 41.4% (15.7–60.8%), respectively. The median (rang) estimated value of the central compartment distribution volume, peripheral compartment distribution volume, and distribution clearance were 13.4 L (6.2–33.8 L), 51.6 L (10.6–174.7 L), and 8.78 L/h (2.32–13.83 L/h), respectively. All the models were internally validated by a visual predictive check (15,17), normalized prediction distribution error (15,21), diagnostic plots (16,18,20-24), boot-strap analysis (17,18), and the prediction error test (19). Mo model was externally tested.
Table 2
Study | Sampling schedule | N (subject/samples) | Structure model | Pharmacokinetic parameters | Covariates |
---|---|---|---|---|---|
2021 Yu (15) | Sparse sampling | 32/112 | 1-CMT | CL =1.59 L/h, IIV =13.0%; CL (L/h) = 1.59 × (CrCL/80)0.408; V =20.5 L; RV (CV%) =40.5% |
CrCL |
2021 Crass (16) | Intensive sampling | 9/45 | 1-CMT | CL =2.09 L/h, IIV =21.5%; V (L) = 12.7 × (TBW/58)0.784; RV (CV%) =18.8% |
TBW |
2021 Wang (17) | Intensive sampling | 70/462 | 2-CMT | Normal renal function: CrCL ≥80 mL/min; CL =2.19 L/h, IIV =22%; Vc =6.87 L, IIV =78%; Vp =11.97 L, IIV =32%; Q =13.83 L/h, IIV =68%; RV (SD) =0.13 |
CrCL |
Renal insufficiency: CrCL <80 mL/min; CL =1.58 L/h, IIV =26%; Vc =6.98 L, IIV =38%; Vp =10.57 L, IIV =74%; Q =10.28 L/h; RV (SD) =0.10 |
|||||
2020 Wang (18) | Intensive sampling | 46/331 | 2-CMT | CL =1.79 L/h, IIV =20.8%; CL (L/h) = 1.79 × (CrCL/105.9)0.362; Vc =6.22 L, IIV =31.8%; Vp =11.92 L, IIV =69%; Q =13.52 L/h, IIV =150.8%; RV (SD) =0.11 |
CrCL |
2018 Manchandani (19) | Sparse sampling | 35/139 | 1-CMT | CL =2.5 L/h, IIV =43.8%; V=34.3 L, IIV =47.8% | – |
2018 Miglis (20) | Sparse sampling | 52/156 | 2-CMT | CL =2.63 L/h, IIV =53.63%; Vc =33.77 L, IIV =45.03%; Vp =78.20 L, IIV =47.90%; Q =2.32 L/h, IIV =57.41%; CL (L/h) = 2.63 × (TBW/75)0.75; RV (SD) =0.1; RV (CV%) =15% |
TBW |
2018 Kubin (21) | Sparse sampling | 43/134 | 1-CMT | CL =2.37 L/h, IIV =37.7%; V =34.4 L, IIV =15.7%; RV (SD) =0.00693; RV (CV%) =23.3% |
– |
2018 Avedissian (22) | Sparse sampling | 9/33 | 2-CMT | CLmax =8.65 L/h, IIV =35.74%; CLnon-renal =0.07 L/h, IIV =31.35%; CrCL50 (mL/min) =141.24, IIV =25.56; CL (L/h) = CLnon-renal + CLmax × CrCLH/ (CrCL50H + CrCLH); Vc =20.39 L, IIV =20.62%; Vp =174.69 L, IIV =20.56%; Q =2.85 L/h, IIV =85.08%; H =7.84, IIV =29.44% |
CrCL |
2013 Sandri (23) | Intensive sampling | 24/192 | 2-CMT | CL =0.0276 L/h/kg, IIV =32.4%; Vc =0.0939 L/kg, IIV =73.3%; Vp =0.330 L/kg, IIV =70.1%; Q =0.146 L/h/kg, IIV =50.4%; RV (SD) =0.0392; RV (CV%) =9.59% |
TBW |
2008 Kwa (24) | Sparse sampling | 9/19 | 1-CMT | Ke =0.051 h−1; V =47.2 L | – |
CV, coefficient of variation; Vc, volume of distribution in the central compartment; CL, total body clearance; IIV, inter-individual variability; Q, intercompartmental flow; Vp, volume of distribution in the peripheral compartment; CLmax, maximum PMB clearance; CLnon-renal, non-renal clearance; H, Hill coefficient; CrCL50, creatinine clearance at the 50% maximal rate of PMB clearance; Ke, elimination rate constant; TBW, total body weight; 1-CMT, one-compartment model; 2-CMT, two-compartment model; RV, residual variability; PMB, polymyxin B.
Covariates
Many factors were investigated in the process of modeling, such as age, gender, total body weight (TBW), ideal body weight, plasma albumin concentration, creatinine clearance (CrCL), and APACHEII score. Four studies included CrCL calculated by the Cockcroft-Gault (C-G) equation as a covariate, and CrCL was positively correlated with clearance. Three studies included TBW as a covariate, and TBW was positively correlated with clearance too.
Discussion
The estimation methods of population pharmacokinetic modeling parameters mainly include the parametric method, non-parametric method, and Bayesian method. The main difference between the parametric method and non-parametric method is that the former assumes that the parameter and error distribution obey normal distribution or log-normal distribution, while the latter does not make any assumption on the shape of the basic parameter distribution. To date, published studies have used both parametric and non-parametric methods to establish population pharmacokinetic models of PMB. First-order conditional estimation (17,18), first-order conditional estimation with inter- and intra-subject variability interaction (15,16), and Monte-Carlo parametric expectation maximization (23) are involved in the parametric method. While the maximum-likelihood method (19,21) and non-parametric adaptive grid (20,22,24) are involved in the non-parametric method. It is not yet known which method is more suitable for the treatment of PMB in specific populations. Thus, more studies comparing these 2 major classes of methods are necessary.
The published studies draw different conclusions about which compartment model is more suitable for PMB. Five of the 10 studies concluded that the 1-compartment model was more appropriate, and 5 studies concluded that the 2-compartment model better described the pharmacokinetic curve of PMB. Five studies used a 2-compartment model and 3 studies used an intensive sampling strategy to collect 6–8 blood samples during 1 treatment cycle. In the study by Sandri (23), 8 blood samples were collected in 1 treatment cycle, and the fits of the 1, 2, and 3 compartment models were compared, and the prediction results of the 2-compartment model were found to be better than the 1- or 3- compartment models. The sampling points of the five studies used a 1-compartment model were sparse, normally contains only random steady-state concentration, or trough/peak concentrations of PMB, which may be related to the insufficient sample information provided by these studies to detect the 2-compartment model of PMB.
There was little difference in clearance between the different studies (see Figure 3), which may be due to: (I) the similarity of the types of patients included in the study. Almost all the studies excluding the patient with ECMO, CRRT, or other conditions that caused significant fluctuations in creatinine. With the exception of Avedissian’s (22) study that included 9 patients with cystic fibrosis (CF), the other 9 studies all included critically ill patients without CF; (II) the extensiveness of the PMB excretion pathways. The proportion of PMB eliminated by the kidneys is very low, and some PMB is excreted unchanged in the bile (6), but other pathways require further study; and (III) the unified sample methods and determinations between the studies; the plasma samples were collected and detected by liquid-mass spectrometer. The clearance of PMB in patients with CF was similar to that of other populations, and as the results of previous studies (25) have shown the pharmacokinetic parameters of most drugs in patients with fibrosis do not differ significantly to those in other patient populations.
In relation to the covariates, the relationship between renal function and the PK parameters of PMB was uncertain. The proportion of PMB excreted by the kidneys is very low and theoretically independent of clearance. Nine of the included studies compared the pharmacokinetic effects of renal function-related parameters, such as serum creatinine values and CrCL (the C-G equation) on PMB. 5 studies (15,17-19,22) included CrCL as a covariate. Yu (15) concluded that there is a significant relationship between CrCL and clearance, and that the dose should be reduced in patients with renal insufficiency, which is consistent with the dosing recommendations in the FDA-approved PMB package insert. However, this study had some limitations, such as the small sample size of the included patients (32 patients), of whom only 3 had CrCL <20 mL/min. In the remaining 4 studies that included CrCL as a covariate, CrCL was statistically correlated with the clearance of PMB, but the effect on the AUC may not have been sufficient to be clinically meaningful. Sandri (23,26) included 2 patients receiving CRRT with a CL of 2.17 and 6.66 L/h, respectively, and continuous venovenous hemofiltration (CVVH) provided clearance of 0.26 and 0.37 L/h. In future studies, more patients with abnormal renal function and measures of renal function need to be included as covariates.
The relationship between body weight and the PK parameters of PMB was also uncertain. Theoretically, obesity leads to physiological changes, such as an increase the in glomerular filtration rate that accelerates the renal excretion of PMB. Additionally, body weight may affect the non-renal excretion of PMB, such as the size of body weight to the liver, the ability to excrete bile, and the extent to which PMB is bound to tissues or released into serum. 9 of the included studies compared the pharmacokinetic effects of body weight indicators, such as TBW and ideal body weight, on PB. Three studies (16,18,23) included TBW as a covariate. Among these 3 studies, Sandri (23) suggested that inter-individual differences in CL and V in the population, which included extremely obese patients, were better described by TBW, and loading and maintenance doses of PB were calculated based on TBW; however, only 2 patients with extreme body weights (41 and 250 kg) were included in the study. The other 2 studies also found that TBW affected clearance, but the way of dosing in total weight proportion was not ideal, but dosing by body weight interval or only loading dose by body weight, maintenance dose fixed dose. The body weight ranges of the included populations were 38.3–70.4 and 30–122 kg, respectively. In one study (20), in which the body weight distribution of the included population was good, a significant relationship between overall weight and clearance was still not found, as an allometric weight scale model was used to adjust the dosing regimen according to body weight. This regimen remains the current clinical practice and is the FDA-approved dosing strategy. Some studies have shown that the total dose of PB is highly associated with the risk of AKI development and is independent of patient weight. Weight-based dosing strategies may increase the risk of AKI in high-weight patients. Future studies need to be conducted to specifically examine the effects of body weight on the PK parameters of PB to address clinical real-world application issues.
Sandri (23) used rapid equilibrium dialysis and found that the plasma protein binding of PMB in critically ill infected patients was 58%, and its corresponding unbound fraction (fu) was 42%. For drugs with moderate plasma protein binding rates, when patients present with hypoproteinemia which has little effect on the concentration of the free drug. In animal experiments, PMB plasma concentrations ranged from 0.9–37 mg/L, and fu showed no change. Six of the studies included plasma albumin as a potential covariate, but none of them were included in the PPK model. Thus, plasma albumin levels are likely to have a weak effect on the PK of PMB.
Compared to internal validation, the external assessment of a model is considered the most rigorous validation method of model testing. Five of the 10 studies were externally validated by Tam (27). At the individual patient level, the correlation between the observed and predicted AUC values was poor (r2<0.2). The Sandri model (23) was the least biased and the Kubin model (21) was the most precise, with significant inter-patient variability. These 5 models had no common demographic variables that could be used to measure PMB exposure at the individual patient level regardless of the structure. The poor correlation between predicted PMB exposure and observed values may be related to the fact that the main metabolic excretion pathways of PMB in the body are unknown.
PMB has the irreplaceable position in the treatment of CROs infection. At present, the metabolic and excretion processes of PMB in the body are still poorly understood. The establishment of any population kinetic model is based on the pharmacological, pharmaceutical, physiopathological knowledge of the drug, the screening and evaluation of various potential covariates, and the construction of covariate models. In the future, on the one hand, the in vivo metabolic excretion process of PMB will be deeply studied, and on the other hand, the kinetic characteristics of PMB in patients supported by CRRT and ECMO will be further studied.
Acknowledgments
Funding: This work was supported by the Hospital Pharmacy Special Research Grant of Zhejiang Pharmaceutical Association (2021ZYY10).
Footnote
Reporting Checklist: The authors have completed the PRISMA reporting checklist. Available at https://atm.amegroups.com/article/view/10.21037/atm-22-236/rc
Conflicts of Interest: All authors have completed the ICMJE uniform disclosure form (available at https://atm.amegroups.com/article/view/10.21037/atm-22-236/coif). The authors have no conflicts of interest to declare.
Ethical Statement: The authors are accountable for all aspects of the work in ensuring that questions related to the accuracy or integrity of any part of the work are appropriately investigated and resolved.
Open Access Statement: This is an Open Access article distributed in accordance with the Creative Commons Attribution-NonCommercial-NoDerivs 4.0 International License (CC BY-NC-ND 4.0), which permits the non-commercial replication and distribution of the article with the strict proviso that no changes or edits are made and the original work is properly cited (including links to both the formal publication through the relevant DOI and the license). See: https://creativecommons.org/licenses/by-nc-nd/4.0/.
References
- China Antimicrobial Resistance Surveillance System. Antimicrobial resistance of bacteria: surveillance report from China Antimicrobial Resistance Surveillance System in 2014-2019. Chinese Journal of Infection Control 2021;20:15-31.
- Chinese Preventive Medicine Association Nosocomial Infection Control Branch. Chinese Hospital Association Specialized Committee of Nosocomial Infection Management, et al. Technical Guideline for Prevention and Control of Carbapenem-resistant Organism (CRO) Infection in China. Chinese Journal of Nosocomiology 2019;29:2075-80.
- Chinese Society of Hematology. Management of Carbapenem-resistant Enterobacteriaceae (CRE) infection in patients with hematological malignancies: Chinese consensus (2020). Chinese Journal of Hematology 2020;41:881-9. [PubMed]
- Avedissian SN, Liu J, Rhodes NJ, et al. A Review of the Clinical Pharmacokinetics of Polymyxin B. Antibiotics (Basel) 2019;8:31. [Crossref] [PubMed]
- Zavascki AP, Goldani LZ, Cao G, et al. Pharmacokinetics of intravenous polymyxin B in critically ill patients. Clin Infect Dis 2008;47:1298-304. [Crossref] [PubMed]
- Manchandani P, Zhou J, Ledesma KR, et al. Characterization of Polymyxin B Biodistribution and Disposition in an Animal Model. Antimicrob Agents Chemother 2015;60:1029-34. [Crossref] [PubMed]
- Yun B, Azad MA, Wang J, et al. Imaging the distribution of polymyxins in the kidney. J Antimicrob Chemother 2015;70:827-9. [Crossref] [PubMed]
- Abdelraouf K, He J, Ledesma KR, et al. Pharmacokinetics and renal disposition of polymyxin B in an animal model. Antimicrob Agents Chemother 2012;56:5724-7. [Crossref] [PubMed]
- Tsuji BT, Pogue JM, Zavascki AP, et al. International Consensus Guidelines for the Optimal Use of the Polymyxins: Endorsed by the American College of Clinical Pharmacy (ACCP), European Society of Clinical Microbiology and Infectious Diseases (ESCMID), Infectious Diseases Society of America (IDSA), International Society for Anti-infective Pharmacology (ISAP), Society of Critical Care Medicine (SCCM), and Society of Infectious Diseases Pharmacists (SIDP). Pharmacotherapy 2019;39:10-39. [Crossref] [PubMed]
- Kalil AC, Metersky ML, Klompas M, et al. Management of Adults With Hospital-acquired and Ventilator-associated Pneumonia: 2016 Clinical Practice Guidelines by the Infectious Diseases Society of America and the American Thoracic Society. Clin Infect Dis 2016;63:e61-e111. [Crossref] [PubMed]
- Sakka SG, Glauner AK, Bulitta JB, et al. Population pharmacokinetics and pharmacodynamics of continuous versus short-term infusion of imipenem-cilastatin in critically ill patients in a randomized, controlled trial. Antimicrob Agents Chemother 2007;51:3304-10. [Crossref] [PubMed]
- Lorente L, Jiménez A, Martín MM, et al. Clinical cure of ventilator-associated pneumonia treated with piperacillin/tazobactam administered by continuous or intermittent infusion. Int J Antimicrob Agents 2009;33:464-8. [Crossref] [PubMed]
- Scaglione F, Esposito S, Leone S, et al. Feedback dose alteration significantly affects probability of pathogen eradication in nosocomial pneumonia. Eur Respir J 2009;34:394-400. [Crossref] [PubMed]
- Liu XQ, Jiao Z, Gao YC, et al. Progress in Development and Application of Decision-making Systems for Individualized Dosing. Chinese Pharmaceutical Journal 2019;54:1-8.
- Yu XB, Jiao Z, Zhang CH, et al. Population pharmacokinetic and optimization of polymyxin B dosing in adult patients with various renal functions. Br J Clin Pharmacol 2021;87:1869-77. [Crossref] [PubMed]
- Crass RL, Al Naimi T, Wen B, et al. Pharmacokinetics of Polymyxin B in Hospitalized Adults with Cystic Fibrosis. Antimicrob Agents Chemother 2021;65:e0079221. [Crossref] [PubMed]
- Wang P, Zhang Q, Zhu Z, et al. Comparing the Population Pharmacokinetics of and Acute Kidney Injury Due to Polymyxin B in Chinese Patients with or without Renal Insufficiency. Antimicrob Agents Chemother 2021;65:e01900-20. [Crossref] [PubMed]
- Wang P, Zhang Q, Zhu Z, et al. Population Pharmacokinetics and Limited Sampling Strategy for Therapeutic Drug Monitoring of Polymyxin B in Chinese Patients With Multidrug-Resistant Gram-Negative Bacterial Infections. Front Pharmacol 2020;11:829. [Crossref] [PubMed]
- Manchandani P, Thamlikitkul V, Dubrovskaya Y, et al. Population Pharmacokinetics of Polymyxin B. Clin Pharmacol Ther 2018;104:534-8. [Crossref] [PubMed]
- Miglis C, Rhodes NJ, Avedissian SN, et al. Population Pharmacokinetics of Polymyxin B in Acutely Ill Adult Patients. Antimicrob Agents Chemother 2018;62:e01475-17. [Crossref] [PubMed]
- Kubin CJ, Nelson BC, Miglis C, et al. Population Pharmacokinetics of Intravenous Polymyxin B from Clinical Samples. Antimicrob Agents Chemother 2018;62:e01493-17. [Crossref] [PubMed]
- Avedissian SN, Miglis C, Kubin CJ, et al. Polymyxin B Pharmacokinetics in Adult Cystic Fibrosis Patients. Pharmacotherapy 2018;38:730-8. [Crossref] [PubMed]
- Sandri AM, Landersdorfer CB, Jacob J, et al. Population pharmacokinetics of intravenous polymyxin B in critically ill patients: implications for selection of dosage regimens. Clin Infect Dis 2013;57:524-31. [Crossref] [PubMed]
- Kwa AL, Lim TP, Low JG, et al. Pharmacokinetics of polymyxin B1 in patients with multidrug-resistant Gram-negative bacterial infections. Diagn Microbiol Infect Dis 2008;60:163-7. [Crossref] [PubMed]
- De Sutter PJ, Gasthuys E, Van Braeckel E, et al. Pharmacokinetics in Patients with Cystic Fibrosis: A Systematic Review of Data Published Between 1999 and 2019. Clin Pharmacokinet 2020;59:1551-73. [Crossref] [PubMed]
- Sandri AM, Landersdorfer CB, Jacob J, et al. Pharmacokinetics of polymyxin B in patients on continuous venovenous haemodialysis. J Antimicrob Chemother 2013;68:674-7. [Crossref] [PubMed]
- Tam VH, Lee LS, Ng TM, et al. Performance of Population Pharmacokinetic Models in Predicting Polymyxin B Exposures. Microorganisms 2020;8:1814-21. [Crossref] [PubMed]