Identification of key biomarkers and immune infiltration in renal interstitial fibrosis
Introduction
Chronic kidney disease (CKD), which is characterized by renal dysfunction, has become an important public health problem because of its high rate of morbidity and mortality. CKD affects approximately 10–13% of the population worldwide (1). Renal fibrosis, particularly renal interstitial fibrosis (RIF), is the final common outcome of various types of CKD. Research has found that RIF is the most reliable predictor of the progression of CKD to end-stage renal failure (ESRD). However, the underlying mechanisms of RIF are yet to be fully elucidated, and current therapies only delay disease progression (2). Therefore, exploring the underlying mechanism and discovering new potential drug targets for RIF are important for future treatments of CKD.
RIF, a progressive and irreversible pathological feature, is characterized by inflammation, myofibroblast activation and migration, and matrix deposition and remodeling. It is currently believed that the RIF develops in response to the accumulation of extracellular matrix (ECM) due to epithelial-mesenchymal transition (EMT), transforming growth factor (TGF)-β signaling, oxidative stress and proteinuria (3). The immune system plays an important role in these changes (4). The immune system can be divided into the innate immune system, which mainly consists of monocytes, natural killer (NK), and dendritic cells (DC), and the adaptive immune system, which is represented by B and T lymphocytes. However, in patients with RIF, a comprehensive evaluation of the distribution of immune cells—the so-called immune infiltration, which might provide key insights into the development of novel therapeutic strategies to prevent the progression of RIF and CKD has not yet been reported.
With the development of microarray technologies, bioinformatics analyses have been widely used to identify disease-specific biomarkers. Recently, some critical genes with tubulointerstitial lesion in CKD were identified using weighted gene co-expression network analysis (WGCNA) by the analysis of GSE104954 and GSE47185 downloaded from the Gene Expression Omnibus (GEO) database (5). Samples of these two datasets were from CKD patients with various pathological type. These findings provided novel biomarkers associated with renal tubulointerstitial injury in CKD. However, this report has not solely focused on RIF. Most importantly, this report did not give any information related to immune system in RIF, which plays important roles in the progression of CKD.
In the current study, a bioinformatics analysis of expression data from RIF patients downloaded from GEO database was performed to explore the diagnostic biomarkers for RIF via WGCNA and machine learning. ISG20 and CORO1A were identified as key hub genes closely correlated with RIF, which was further verified by biochemical studies with fibrotic tubular cells and renal tissue. Furthermore, potential drugs targeting ISG20 and CORO1A were predicted. Most importantly, evaluation of immune cell infiltration showed that the abundance of most immune cells was found to significantly differ between RIF and normal samples. Collectively, these findings provide two novel molecular markers to further explore the pathological mechanisms and potential drug targets for RIF.
We present the following article in accordance with the MDAR reporting checklist (available at https://atm.amegroups.com/article/view/10.21037/atm-22-366/rc).
Methods
Data source
The expression data of 24 RIF and 25 normal samples in GSE22459, and 42 RIF and 99 normal samples in GSE76882, were downloaded from the GEO database (https://www.ncbi.nlm.nih.gov/geo/). To identify genes that are key to the progression of RIF, the genes in GSE22459 and GSE76882 datasets were first overlapped to obtain 15,779 genes from 66 RIF and 124 samples for further analysis (Figure S1A). Thereafter, the data of GSE22459 and GSE76882 were combined. The Sangerbox online tool (http://sangerbox.com) was applied to remove the batch effect of the two datasets (Figure S1B). Thereafter, a sample clustering tree map was constructed to detect and eliminate outliers, which showed that outlier samples were not detected (Figure S1C).
The Sangerbox online tool (http://sangerbox.com) was used to remove the batch effects of the two datasets.
WGCNA
WGCNA was performed based on gene expression profiles and sample traits (normal and RIF). To select the best soft threshold, the pick soft threshold function of WGCNA was used to calculate the value of β from 1 to 30. Based on the selected soft threshold, the adjacency matrix was converted to a topological overlap matrix to construct the network, and the gene dendrogram and module color were established using the degree of dissimilarity. Further, the initial module was divided using dynamic tree cutting, and similar modules were merged. The Pearson correlation coefficients between the module eigengenes and sample traits were calculated to determine the relevant modules associated with RIF, where modules with |Correlation coefficient (cor)| >0.3 and a P value <0.05 were considered as key modules related to RIF. Using |module membership (MM)| >0.8 and |gene significance (GS)| >0.2 as criteria, genes in the relevant modules associated with RIF were further screened for downstream analysis.
Identification and functional analysis of candidate key genes in RIF
The Limma R package was used to identify differentially expressed genes (DEGs) between RIF and normal samples, with a threshold of |log2FC| >0.5 and an adjusted P value <0.05. The ggplot and pheatmap R package were used to plot the volcano diagram and heatmap of DEGs, respectively. To obtain candidate key genes in RIF, we intersected DEGs with genes obtained from WGCNA. The ClusterProfiler R package was used to perform Gene Ontology (GO) and Kyoto Encyclopedia of Genes and Genomes (KEGG) enrichment analyses of candidate key genes. Statistical significance was set at P<0.05. Furthermore, the protein-protein interaction network of candidate key genes was constructed and visualized using the STRING database (https://www.string-db.org/) and Cytoscape software (version 3.7.2).
Selection of hub gene signatures by machine learning
We applied the least absolute shrinkage and selection operator (LASSO) algorithm using the glmnet package in R software and screened the gene signatures under the optimal lambda with the smallest classification error. The random forest (RF) R package was used to construct the RF model to identify the important variables. The performance of the RF model was evaluated using the receiver operating curve (ROC). Support vector machine-recursive feature elimination (SVM-RFE) was also performed using the e1071 package in R software to identify the best variables (gene signatures) via the deletion of SVM-generated eigenvectors in conjunction with 5× fold cross validation. Finally, we identified hub gene signatures by overlapping gene signatures from the LASSO, RF, and SVM-RFE algorithms.
Identification of hub biomarkers in RIF
The diagnostic value of the hub gene signature was determined using ROC curves. Hub genes with areas under the curve (AUC) greater than 0.8 were identified as potential diagnostic biomarkers in RIF. The expression of diagnostic biomarkers in RIF and control samples were displayed in the box plot. The Wilcoxon test was used for the comparison with the ggpubr R package. Moreover, the chemicals interacting with the diagnostic biomarkers were predicted using the Comparative Toxicogenomics Database (CTD) (https://ctdbase.org). Accordingly, a chemical-diagnostic biomarker interaction network was constructed using the Cytoscape software.
Verification of hub biomarkers through biochemical experiments
Reagents and antibodies
TGF-β (240-B) was purchased from Bio-Techne (China). Adding TGF-β to epithelial cells in culture is a convenient way to construct fibrosis cell models, due to the important role of TGF-β in the induction of MCT (6). Therefore, TGF-β was used as stimulator to construct the renal fibrosis cell models in the present study. The following antibodies were used: anti-α-SMA antibody (19245T) from CST (USA); anti-CORO1A antibody (ab203698) from Abcam (Cambridge, UK); anti-ISG20 antibody (22097-1-AP) from Proteintech (USA); anti-Vimentin antibody (ab92547) from Abcam (Cambridge, UK); and anti-GAPDH antibody (60004-1-Ig) from Proteintech (USA).
Cell line and cell culture
HK-2 cells (Human renal tubular epithelial cells, 339833) were obtained from the Beijing Beina Chuanglian Biotechnology Research Institute (China). Cells were cultured in RPMI-1640 supplemented with 10% fetal bovine serum (FBS, EallBio, China), 100 U/mL penicillin, and 100 µg/mL streptomycin (NCM Biotech, China), and incubated at 37 °C in a 10% CO2 atmosphere.
Western blotting analysis
Cell lysates from HK-2 cells stimulated with TGF-β were mixed with an appropriate amount of 5× sample loading buffer. Proteins were separated using sodium dodecylsulphate polyacrylamide gel electrophoresis (SDS-PAGE) and transferred onto a polyvinylidene fluoride membrane. The membrane was blocked with 5% non-fat milk and incubated with primary and secondary antibodies. The membrane was washed three times with Tris-Buffered Saline and Tween 20 (TBST) and incubated with each antibody. Protein bands were visualized with a hypersensitive ECL chemiluminescence kit (NCM Biotech, China), and signals were recorded using a Tanon 5200 chemiluminescence imaging system (China).
Quantitative polymerase chain reaction (qPCR)
Total RNA was isolated from HK-2 cells stimulated with TGF-β using TRIzol (Vazyme, China), and reverse transcribed into cDNA using the HiScript II Q RT SuperMix kit (Vazyme). qPCR was performed on an Applied Biosystems 7500 Real-Time PCR system using the 2× SYBR Green qPCR Master Kit (Biotool). The primer sequences were as follows: α-SMA, 5'- GACAATGGCTCTGGGCTCTGTAA -3' (forward) and 5'-CTGTGCTTCGTCACCCACGTA-3' (reverse); CORO1A, 5'-GCACCCAGACACGATCTACAG-3' (forward) and 5'-GGACGGTCCTTCTCAGCTAC-3' (reverse); ISG20, 5'-CTTCCAGGCACTGAAAGAGG-3' (forward) and 5'-ATCTTCCACCGAGCTGTGTC-3' (reverse); Vimentin 5'-GCGTGACGTACGTCAGCAATATGA-3' (forward) and 5'-GTTCCAGGGACTCATTGGTTCCTT-3' (reverse); and GAPDH 5'-TGCACCACCAACTGCTTAGC-3' (forward) and 5'-ACAGTCTTCTGGGTGGCAGTG (reverse).
Immunohistochemistry
Twenty formalin-fixed and paraffin-embedded fibrotic renal tissue samples were obtained from CKD patients who underwent a renal biopsy at the Second Affiliated Hospital of Soochow University from August 2019 to October 2021. All procedures performed in this study involving human participants were in accordance with the Declaration of Helsinki (as revised in 2013). The study was approved by the ethics committee of the Second Affiliated Hospital of Soochow University (No. JD-HG-2021-52) and informed consent was taken from all the patients.
Immunohistochemical staining was performed to detect expression of ISG20 and CORO1A according to a previously described method (7). The staining intensity was scored as 0 (negative), 1 (weak), 2 (medium), or 3 (strong), whereas the staining area was scored as 0 (0% of the staining area), 1 (1–25%), 2 (26–50%), 3 (51–75%), and 4 (76–100%). The final scores for ISG20 and CORO1A expression were obtained by calculating the products of the scores of staining intensity and staining area.
Masson staining
To evaluate the degree of interstitial fibrosis in fibrotic renal tissues, 3-µm-thick sections were generated for Masson trichrome staining. Aipathwell (Wuhan servicebio technology Co., Ltd., China) was used to quantify the positive staining area. Thereafter, the proportion of positive staining was calculated.
Distribution of immune cells in RIF
The single sample gene set enrichment analysis (ssGSEA) algorithm in “GSVA” R package was applied to calculate the infiltration levels of 28 immune cell types in RIF and normal samples, including immature B cells, memory B cells, activated B cells, central memory CD8+ T cells, central memory CD4+ T cells, activated CD4+ T cells, activated CD8+ T cells, NK cells, effector memory CD8+ T cells, MDSCs, type 2 T helper cells, regulatory T cells, effector memory CD4+ T cells, gamma delta T cells, CD56dim NK cells, CD56bright NK cells, type 1 T helper cells, type 17 T helper cells, monocytes, macrophages, NK T cells, immature DCs, activated DCs, plasmacytoid DCs, mast cells, T follicular helper cells, eosinophils, and neutrophils. The correlations of the infiltration levels among the 28 immune cells were calculated. Comparisons of immune cell infiltration between RIF and normal samples were conducted using the Wilcoxon test. Immune cells with a P value <0.05 were considered to be differentially infiltrated immune cells (DIICs). The t-SNE method was then applied to cluster and visualize the distribution of DIICs in the GEO cohort. Spearman correlations between DIICs and diagnostic biomarkers were calculated.
Statistical analysis
Both the correlations between the module eigengenes and sample traits and the correlations among immune cells were calculated by Pearson’s correlation coefficient. The comparisons of immune cell infiltration between RIF and normal samples were calculated by Wilcoxon test. Student’s t-test (two-sided) was performed to compare the data from different groups in the biochemical experiments. The values are expressed as mean ± standard deviation (SD). All values of P<0.05 were considered to be statistically significant.
Results
Identification of the key modules associated with RIF
By using the pick soft threshold function of WGCNA, we found that the optimal soft threshold power was 14, with an R2 of approximately 0.85 (Figure 1A). After merging similar modules, 18 modules were identified from the co-expression network (Figure S2 and Figure 1B). According to the module-trait relationships in Figure 1C, the blue module cor =0.45; P value <0.05) and light cyan module (cor =0.31; P value <0.05) were found to be relevant to RIF. Therefore, 746 genes in the blue module and 390 genes in the light cyan module were extracted. Then, using |GS| >0.2 and |MM| >0.8, a total of 121 genes were selected from the blue and light cyan modules for downstream analysis.
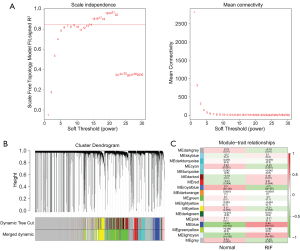
Identification of candidate key genes in RIF
We explored the DEGs involved in the RIF. A total of 523 DEGs were identified, including 386 upregulated and 137 downregulated genes in RIF samples compared to normal samples (https://cdn.amegroups.cn/static/public/atm-22-366-1.xlsx) (Figure 2A). The expression levels of the top 100 DEGs in each sample are displayed in a heatmap (Figure 2B). To screen for RIF-related DEGs, we overlapped 121 genes identified via WGCNA and 523 DEGs and obtained a total of 78 genes that may have been key to the progression of RIF (candidate key genes; Figure 2C). The potential biological functions of candidate key genes in RIF were investigated using clusterProfiler. A total of 378 biological processes, 29 cellular components, and 11 molecular functions were significantly enriched (https://cdn.amegroups.cn/static/public/atm-22-366-2.xlsx). The top 10 biological processes were closely associated with immunity and inflammation, such as leukocyte proliferation, regulation of immune effector processes, regulation of lymphocyte proliferation, regulation of mononuclear cell proliferation, regulation of innate immune response, and neutrophil degranulation (Figure 3A). Further, candidate key genes were found to be significantly enriched in 32 KEGG pathways (https://cdn.amegroups.cn/static/public/atm-22-366-3.xlsx). Consistent with the GO results, the top 10 pathways related to immune diseases and inflammation, such as pertussis, leishmaniasis, NK cell-mediated cytotoxicity and NOD-like receptor signaling pathways (Figure 3B). These results indicate that immunity and inflammation play an important role in RIF. In the protein-protein interaction (PPI) network, 78 candidate key genes in RIF were found to interact with each other (Figure 3C).
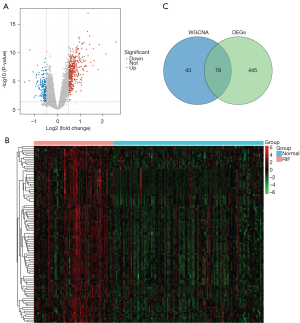
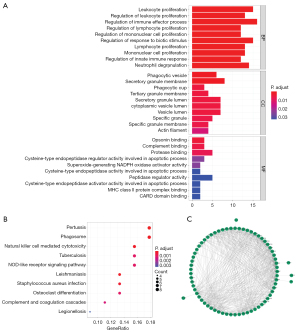
Identification of ISG20 and CORO1A as key biomarkers of RIF
The 78 candidate key genes were employed in the LASSO, RF, and SVM-RFE analyses to screen hub signatures in RIF. First, we performed the LASSO algorithm, which showed nine signatures, including CORO1A, ISG20, LCP1, PLEK, PROM1, RASSF2, RASSF2, TIMP1, and TNFAIP3 (Figure 4A,4B). Using RF, we selected the top five most important variables, which were PROM1, CSF1R, CORO1A, ISG20, and TIMP1 (Figure 4C). The ROC of the RF model showed good performance, with an AUC of 0.817 (Figure 4D). We also performed the SVM-RFE algorithm and identified 41 gene signatures at 5-fold cross-validation (Figure 4E,4F). After overlapping the gene signatures selected by LASSO, RF, and SVM-RFE, PROM1, CORO1A, ISG20, and TIMP1 were identified as hub signatures in RIF (Figure 5A). Subsequently, ROC curves were then used to evaluate the diagnostic value of the hub signature. The AUC values of CORO1A, ISG20, PROM1, and TIMP1 were 0.816, 0.832, 0.726, and 0.763, respectively (Figure 5B-5E), suggesting that ISG20 and CORO1A had better performance at distinguishing RIF and normal samples. Therefore, ISG20 and CORO1A were identified as diagnostic biomarkers of RIF. The expression levels of ISG20 and CORO1A were markedly elevated in RIF samples compared with normal samples (Figure 5F,5G).
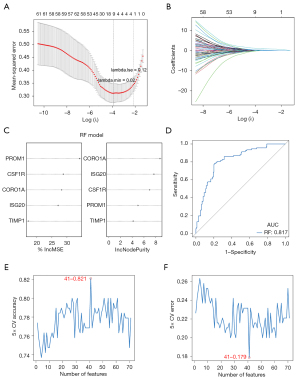
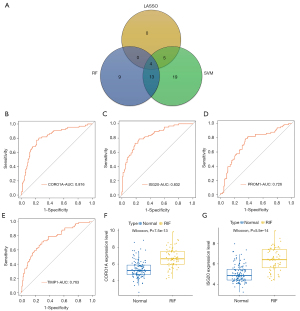
Validation of the expression of ISG20 and CORO1A in fibrotic tubular cells and renal tissue
To further determine the contribution of ISG20 and CORO1A to RIF, we validated the expression levels of ISG20 and CORO1A in fibrotic tubular cells and renal tissue. The mRNA expression levels of ISG20 and CORO1A in TGF-β-stimulated HK-2 cells were significantly higher than levels in the control (Figure 6A). Immunoblotting analyses further showed that fibrotic tubular cells expressed higher protein levels of ISG20 and CORO1A (Figure 6B). Finally, 20 fibrotic renal tissues were selected from patients with CKD receiving renal biopsy. The characteristics of included patients were showed in https://cdn.amegroups.cn/static/public/atm-22-366-4.xlsx. The samples were divided into two groups based on the median score of the percentages of positive staining for Masson on wax-embedded renal tissues. Samples with a higher amount of Masson staining had higher CORO1A and ISG20 protein levels (Figure 6C,6D), indicating that ISG20 and CORO1A were positively correlated with the degree of renal fibrosis. Collectively, these data further highlight the key role of ISG20 and CORO1A in RIF.
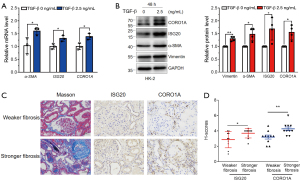
Identification of potential drugs for the treatment of RIF
We predicted the potential 77 and 46 chemicals that could either increase or decrease the expression of ISG20 and CORO1A, respectively, using the CTD database. Based on their interaction, we constructed a chemical-diagnostic biomarker network, which showed that ISG20 and CORO1A had common and specific interacting chemicals (Figure 7). Based on the elevated expression patterns of ISG20 and CORO1A in RIF, 29 and 25 chemicals may serve as potential drugs that can reduce the expression of ISG20 and CORO1A, respectively (https://cdn.amegroups.cn/static/public/atm-22-366-5.xlsx), and ultimately treat RIF. Notably, antirheumatic agents, cadmium, chenodeoxycholic acid, dimethylselenide, methotrexate, methyl methanesulfonate, tetrachlorodibenzodioxin, and tretinoin were common chemicals targeting both ISG20 and CORO1A (https://cdn.amegroups.cn/static/public/atm-22-366-6.xlsx).
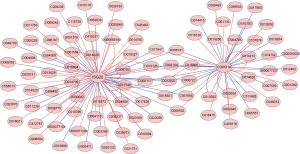
Distribution of immune cells in RIF
Based on the GO and KEGG results, we investigated and compared the immune microenvironment between RIF and normal samples. Using ssGSEA, the infiltration levels of 28 immune cells in the RIF and normal samples were calculated (Figure 8A and Figure S3). Moderate to high correlations were found among these immune cells (Figure 8B), indicating their interactions in the immune microenvironment. Further, the abundance of 21 immune cells was found to significantly differ between RIF and normal samples, including activated B cells, central memory CD4+ T cells, activated CD4+ T cells, activated CD8+ T cells, NK cells, effector memory CD8+ T cells, MDSCs, type 2 T helper cells, regulatory T cells, gamma delta T cells, CD56dim NK cells, CD56bright NK cells, type 1 T helper cells, monocytes, macrophages, NK T cells, immature DCs, activated DCs, plasmacytoid DCs, mast cells, and T follicular helper cells (Figure 8C). Based on the t-SNE results, the GEO cohort could be classified into two distinct clusters (RIF and normal) (Figure 8D), which indicates that DIIC may also serve as a biomarker in the diagnosis of RIF. We also calculated the correlations between DIICs and the two key biomarkers. However, the results showed that only a weak relationship existed between most DIIC and the two key genes. Further, CD56dim NK cells and plasmacytoid DCs were found to be positively correlated with ISG20 and CORO1A to some degree (Figure 8E).
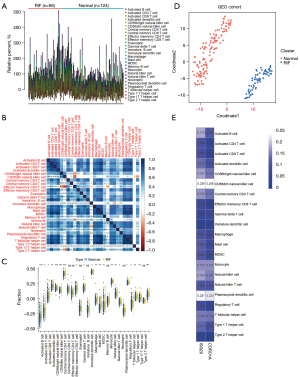
Discussion
RIF is a crucial and complex metabolic change in the late stages of CKD. Therefore, there is an urgent need to better understand the detailed mechanisms to develop novel strategies to diagnose and treat RIF. Based on datasets downloaded from the GEO database, ISG20 and CORO1A were identified as key genes in RIF; such findings were further verified via biochemical experiments. Additionally, 29 and 25 chemicals were predicted as potential drugs for the treatment of RIF as they could reduce the expression of ISG20 and CORO1A, respectively. According to the results of ssGSEA, most immune cells were differentially infiltrated in the RIF and normal samples, and the abundance of some immune cells was positively correlated with these two key genes.
A previous study using the same GEO datasets screened CD2, CCL5, and CCR5 as potential therapeutic target genes in RIF by overlapping DEGs identified from GSE22459 and GSE76882 datasets and PPI network analysis (8). However, unlike the previous study, the candidate key genes of RIF in the present study were first identified by overlapping the RIF-related genes from WGCNA with DEGs from the two datasets. Thereafter, the hub genes related to RIF were selected by machine learning methods, including LASSO, RF, and SVM-RFE. Finally, ROC curves were generated to determine the diagnostic value of the hub gene signature. The two genes, ISG20 and CORO1A, with better performance at distinguishing between RIF and normal samples, were ultimately identified. The differences in the analysis methods and procedures may account for the different hub genes screened. In the present study, the different mRNA and protein expression patterns of ISG20 and CORO1A were further verified in fibrotic tubular cells stimulated with TGF-β and fibrotic renal tissues from patients with varying degrees of fibrosis. In accordance with the results of bioinformatics analysis, the expression levels of ISG20 and CORO1A were significantly higher in the fibrotic tubular cells; the more severe the fibrosis in renal tissues, the higher the expression level of the two hub genes.
Tryptophan aspartate-containing coat protein (TACO, also known as CORO1A or coronin-1), which is almost exclusively expressed in hematopoietic lineages, has been studied in the immunological field over the last few years and has been found to play an important role in T lymphocyte activation, CD4+ effector/memory T cell differentiation, and innate immunity (9-11). Many reports have demonstrated the contribution of CORO1A to inflammation and immune-related diseases, including multiple microorganism infection (12-14), spontaneous osteoarthritis (15), severe combined immune deficiency (16), and cancer (17). Recently, CORO1A was identified as a hub gene in chronic classic cardiomyopathy (CCC), characterized by severe cardiac inflammation and myocardial fibrosis (18). However, the relationship between CORO1A and renal-related diseases and the underlying molecular mechanisms have not been reported. Nevertheless, our current study identified CORO1A as a novel key gene that was positively correlated with the degree of RIF.
Interferon-stimulated exonuclease gene 20 (ISG20), an interferon-regulated gene, codes for a 20-kDa protein with 181 amino acids, induced by both type I (IFN-α/β) and type II (IFN-γ) IFNs (19). According to a previous report (20), ISG20 expression can be induced in renal mesangial cells by polyinosinic-polycytidylic acid [poly (I:C)], an authentic double-stranded RNA that mimics viral infections when applied to cells. Renal mesangial cells have been known to play an important role in the immune and inflammatory responses in the kidney. Additionally, the common immune response module (CRM) scores of seven genes, including ISG20, were significantly higher in kidney transplant patients with acute rejection (AR) and progressive interstitial fibrosis and tubular atrophy (pIFTA) than those without rejection response (21). Therefore, these findings may provide indirect evidence of the correlation between ISG20 and renal fibrosis, a pathological change driven by various immune and inflammatory responses and served as a feature of renal transplantation failure. In addition to the findings of previous studies (20,21), our results imply that ISG20 might act as a key gene in RIF. Thus, targeting this novel biomarker may be a promising therapeutic strategy for the treatment of RIF.
Wet experiments that identify drugs targeting specific genes are time-consuming and expensive. However, many drug-gene associations remain unobserved or unknown. The use of a database to predict unobserved drugs targeting a specific gene is an important and urgent task. By using the CTD database in the present study, 29 and 25 chemicals were identified to decrease the expression of ISG20 and CORO1A, respectively, including prednisolone, a classic glucocorticoid widely used to delay the progression of renal inflammatory and fibrosis in clinical practice. Most importantly, seven chemicals were found to simultaneously select the two targets. Among them, chenodeoxycholic acid was recently reported to improve renal fibrosis, inflammation, and oxidative stress in rats fed high fructose (22). However, the exact targets and underlying mechanisms remain unknown. Therefore, our work offers potential opportunities and drug targets for the treatment of RIF.
The infiltration of inflammatory cells, including T cells, monocytes/macrophages, DCs, and mast cells, is a major cellular event in tubulointerstitial fibrosis (23). Despite the established correlation between sustained inflammation and fibrotic disease, the role of various inflammatory cells is highly complex (24). The infiltration and activation of macrophages, T cells, and DCs contribute to fibrogenesis, while infiltrated mast cells tend to attenuate renal fibrosis induced by kidney injury (25,26). Recently, early-stage accumulation of B lymphocytes in the kidney was reported to accelerate monocyte/macrophage mobilization and infiltration, aggravating fibrosis (27). In our study, among the 28 types of immune cells, the infiltration of 21 immune cells was significantly different between RIF and normal samples. Notably, ISG20 and CORO1A were positively correlated with CD56dim NK cells and plasmacytoid DCs to some degree. The literature evidence and our results imply that immune cell infiltration plays an important role in RIF and should be the focus of further studies.
As this study had limitations, further in vivo and in vitro experiments are needed to explore the function of these two genes and the underlying mechanism in RIF.
Conclusions
The hub genes, ISG20 and CORO1A, were identified in RIF via bioinformatics analysis using the GEO database and biochemical experiments with fibrotic tubular cells and renal tissues. Further, the drugs targeting these two genes were predicted, and the immune cell infiltration pattern of RIF was further described, thereby providing useful information and directions to further investigate the roles of ISG20 and CORO1A in RIF. Overall, our findings provide novel insights into the mechanisms and treatment of patients with RIF.
Acknowledgments
Funding: This work was supported by the Natural Science Foundation of Jiangsu Province (BK20211072) and Suzhou Bureau of Science and Technology (Basic Research in Medical and Health Sciences, SYSD2020183).
Footnote
Reporting Checklist: The authors have completed the MDAR reporting checklist. Available at https://atm.amegroups.com/article/view/10.21037/atm-22-366/rc
Data Sharing Statement: Available at https://atm.amegroups.com/article/view/10.21037/atm-22-366/dss
Conflicts of Interest: All authors have completed the ICMJE uniform disclosure form (available at https://atm.amegroups.com/article/view/10.21037/atm-22-366/coif). The authors have no conflicts of interest to declare.
Ethical Statement: The authors are accountable for all aspects of the work in ensuring that questions related to the accuracy or integrity of any part of the work are appropriately investigated and resolved. All procedures performed in this study involving human participants were in accordance with the Declaration of Helsinki (as revised in 2013). The study was approved by the ethics committee of the Second Affiliated Hospital of Soochow University (No. JD-HG-2021-52) and informed consent was taken from all the patients.
Open Access Statement: This is an Open Access article distributed in accordance with the Creative Commons Attribution-NonCommercial-NoDerivs 4.0 International License (CC BY-NC-ND 4.0), which permits the non-commercial replication and distribution of the article with the strict proviso that no changes or edits are made and the original work is properly cited (including links to both the formal publication through the relevant DOI and the license). See: https://creativecommons.org/licenses/by-nc-nd/4.0/.
References
- Ammirati AL. Chronic Kidney Disease. Rev Assoc Med Bras (1992) 2020;66:s03-9. [Crossref] [PubMed]
- Ruiz-Ortega M, Rayego-Mateos S, Lamas S, et al. Targeting the progression of chronic kidney disease. Nat Rev Nephrol 2020;16:269-88. [Crossref] [PubMed]
- Ke B, Zhu N, Luo F, Xu Y, Fang X. Targeted inhibition of endoplasmic reticulum stress: New hope for renal fibrosis Mol Med Rep 2017;16:1014-20. (Review). [Crossref] [PubMed]
- Humphreys BD. Mechanisms of Renal Fibrosis. Annu Rev Physiol 2018;80:309-26. [Crossref] [PubMed]
- Wang W, Shen J, Qi C, et al. The key candidate genes in tubulointerstitial injury of chronic kidney diseases patients as determined by bioinformatic analysis. Cell Biochem Funct 2020;38:761-72. [Crossref] [PubMed]
- Xu J, Lamouille S, Derynck R. TGF-beta-induced epithelial to mesenchymal transition. Cell Res 2009;19:156-72. [Crossref] [PubMed]
- Yakirevich E, Resnick MB, Mangray S, et al. Oncogenic ALK Fusion in Rare and Aggressive Subtype of Colorectal Adenocarcinoma as a Potential Therapeutic Target. Clin Cancer Res 2016;22:3831-40. [Crossref] [PubMed]
- Zhang C, Hu X, Qi F, et al. Identification of CD2, CCL5 and CCR5 as potential therapeutic target genes for renal interstitial fibrosis. Ann Transl Med 2019;7:454. [Crossref] [PubMed]
- Shiow LR, Roadcap DW, Paris K, et al. The actin regulator coronin 1A is mutant in a thymic egress-deficient mouse strain and in a patient with severe combined immunodeficiency. Nat Immunol 2008;9:1307-15. [Crossref] [PubMed]
- Kaminski S, Hermann-Kleiter N, Meisel M, et al. Coronin 1A is an essential regulator of the TGFβ receptor/SMAD3 signaling pathway in Th17 CD4(+) T cells. J Autoimmun 2011;37:198-208. [Crossref] [PubMed]
- Pick R, Begandt D, Stocker TJ, et al. Coronin 1A, a novel player in integrin biology, controls neutrophil trafficking in innate immunity. Blood 2017;130:847-58. [Crossref] [PubMed]
- Cohen JI. Primary Immunodeficiencies Associated with EBV Disease. Curr Top Microbiol Immunol 2015;390:241-65. [Crossref] [PubMed]
- Fei X, Li Z, Yang D, et al. Neddylation of Coro1a determines the fate of multivesicular bodies and biogenesis of extracellular vesicles. J Extracell Vesicles 2021;10:e12153. [Crossref] [PubMed]
- Heidari M, Pakdel A, Bakhtiarizadeh MR, et al. Integrated Analysis of lncRNAs, mRNAs, and TFs to Identify Regulatory Networks Underlying MAP Infection in Cattle. Front Genet 2021;12:668448. [Crossref] [PubMed]
- Wang Y, Wu C, Tao J, et al. Differential proteomic analysis of tibial subchondral bone from male and female guinea pigs with spontaneous osteoarthritis. Exp Ther Med 2021;21:633. [Crossref] [PubMed]
- Vignesh P, Rawat A, Kumrah R, et al. Clinical, Immunological, and Molecular Features of Severe Combined Immune Deficiency: A Multi-Institutional Experience From India. Front Immunol 2021;11:619146. [Crossref] [PubMed]
- He Y, Jiang Z, Chen C, et al. Classification of triple-negative breast cancers based on Immunogenomic profiling. J Exp Clin Cancer Res 2018;37:327. [Crossref] [PubMed]
- Wu J, Cao J, Fan Y, et al. Comprehensive analysis of miRNA-mRNA regulatory network and potential drugs in chronic chagasic cardiomyopathy across human and mouse. BMC Med Genomics 2021;14:283. [Crossref] [PubMed]
- Zheng Z, Wang L, Pan J. Interferon-stimulated gene 20-kDa protein (ISG20) in infection and disease: Review and outlook. Intractable Rare Dis Res 2017;6:35-40. [Crossref] [PubMed]
- Imaizumi T, Tanaka H, Mechti N, et al. Polyinosinic-polycytidylic acid induces the expression of interferon-stimulated gene 20 in mesangial cells. Nephron Exp Nephrol 2011;119:e40-8. [Crossref] [PubMed]
- Sigdel TK, Bestard O, Tran TQ, et al. A Computational Gene Expression Score for Predicting Immune Injury in Renal Allografts. PLoS One 2015;10:e0138133. [Crossref] [PubMed]
- Hu Z, Ren L, Wang C, et al. Effect of chenodeoxycholic acid on fibrosis, inflammation and oxidative stress in kidney in high-fructose-fed Wistar rats. Kidney Blood Press Res 2012;36:85-97. [Crossref] [PubMed]
- Zeisberg M, Neilson EG. Mechanisms of tubulointerstitial fibrosis. J Am Soc Nephrol 2010;21:1819-34. [Crossref] [PubMed]
- Andrade-Oliveira V, Foresto-Neto O, Watanabe IKM, et al. Inflammation in Renal Diseases: New and Old Players. Front Pharmacol 2019;10:1192. [Crossref] [PubMed]
- Liu L, Kou P, Zeng Q, et al. CD4+ T Lymphocytes, especially Th2 cells, contribute to the progress of renal fibrosis. Am J Nephrol 2012;36:386-96. [Crossref] [PubMed]
- Beghdadi W, Madjene LC, Claver J, et al. Mast cell chymase protects against renal fibrosis in murine unilateral ureteral obstruction. Kidney Int 2013;84:317-26. [Crossref] [PubMed]
- Han H, Zhu J, Wang Y, et al. Renal recruitment of B lymphocytes exacerbates tubulointerstitial fibrosis by promoting monocyte mobilization and infiltration after unilateral ureteral obstruction. J Pathol 2017;241:80-90. [Crossref] [PubMed]
(English Language Editor: C. Mullens)