Identification and validation of novel gene markers of osteoporosis by weighted co expression analysis
Introduction
Osteoporosis has been recognized as a chronic skeletal illness that is more prevalent in postmenopausal women. Osteoporosis is associated with a high risk of bone fractures and loss of bone mass (1). Osteogenic osteoblasts and bone-resorbing osteoclasts need to be balanced for maintaining bone homeostasis (2). When this homeostasis is disturbed, normal bone remodeling becomes ineffective at maintaining constant bone mass, resulting in bone loss and osteoporosis (3). Conventionally, dual X-ray bone densitometers (DEXA), which measure bone density (BMD), are commonly used to examine the risk of fractures in osteoporosis (4).
Over the last several years, screening techniques, including the Fracture Risk Assessment System (FRAX), have been created and shown to be both scientifically sound and cost-effective (5,6). The clinical risk factors that have been identified include a family history of fractures (especially hip fractures), female gender, history of low trauma fractures, age, reduced BMD, smoking and excessive alcohol consumption, low body mass index (BMI), and use of glucocorticoids (7). In addition to the prescription of sufficient vitamin D, calcium, and exercise for long-term bone health, it is advised that high-risk populations be given medication to prevent the progression of osteoporosis. Current osteoporosis therapies fall into two categories, namely, anti-resorptive drugs and anabolic medication. Anti-resorptive agents reduce bone resorption in osteoclasts (8), and include raloxifene, bisphosphonates, and Denosumab which is a fully humanized antibody against RANKL (receptor activator of nuclear factor kappa-Β ligand). Anabolic drugs promote bone formation, and include teriparatide (1 to 34) which is the amino-terminal fragment of human parathyroid hormone (PTH) (9,10), parathyroid hormone-related peptide (PTHrP) (1 to 34) (abaloparatide) which is an analog of PTHrP (11), and Romososumab which is a monoclonal antibody against sclerostin (12).
In recent years, the advent of high-throughput technology has made it possible to use gene microarray analysis to effectively identify differentially expressed genes (DEGs), thereby facilitating the identification of potential biomarkers in a variety of disorders. Gene microarray analysis has been used to discover important genes involved in the etiology of osteoporosis in numerous studies (13,14). It is possible that integrated multi-gene microarray analysis will aid in the identification of more promising gene biomarkers.
Chen et al. reported that a diagnostic model established based on nine key genes could reliably separate Osteoporosis patients from healthy subjects (15). Chen et al. have identified a group of circulating miRNAs as non-invasive biomarkers for the detection of postmenopausal and mechanical unloading Osteoporosis through a large-scale screening based on microarray (13). Xia et al. showed that genes such as VPS35, FCGR2A, TBCA, HIRA, TYROBP, JUND, PHF20, NFKB2, RPL35A and BICD2 may be considered to be potential pathogenic genes of osteoporosis and may be useful for further study of the mechanisms underlying osteoporosis (16). Although a large number of biomarkers have been identified in different ways, there is still a lack of model analyses based on multiple key genes that are valid in Osteoporosis for clinical application.
The current study adopted a bioinformatics approach to identify potential prognostic markers of osteoporosis by performing differential gene expression studies, weighted gene co-expression network analysis (WGCNA), protein-protein interaction (PPI) analysis, Kyoto Encyclopedia of Genes and Genomes (KEGG) analysis, and Gene Ontology (GO) analysis on the expression profiles of osteoporosis samples from the Gene Expression Omnibus (GEO) database. The aim was to identify early predictors of osteoporosis based on the expression profiles of the GEO dataset. The findings of this research could enable the screenings of high-risk populations to facilitate early diagnosis, treatment, and management of these patients.
We present the following article in accordance with the REMARK reporting checklist (available at https://atm.amegroups.com/article/view/10.21037/atm-22-229/rc).
Methods
The research methods used in this study is shown in Figure 1.
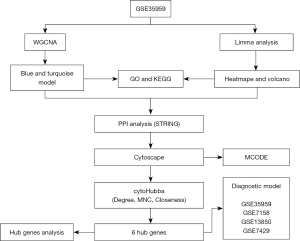
Microarray profiles and data processing
The RNA sequencing and methylation data of osteoporosis samples were obtained from the National Center for Biotechnology Information (NCBI) Gene Expression Omnibus (GEO) (17) public database under the data series accession number GSE35959 (18), GSE7158 (19), GSE13850 (14), and GSE7429 (20) on July 20, 2020. The osteoporosis samples were kept and the probes were transferred to Gene Symbol. When a probe corresponds to multiple genes, the probe was removed, and expressions with multiple gene symbols were taken as the median value. The clinical characteristics of the samples are shown in Table 1.
Table 1
Data set | Expression | Platforms |
---|---|---|
GSE35959 | GPL570 | |
Normal | 9 | |
Osteoporosis | 5 | |
GSE7158 | GPL570 | |
High PBM | 14 | |
Low PBM | 12 | |
GSE13850 | GPL96 | |
High BMD | 20 | |
Low BMD | 20 | |
GSE7429 | GPL96 | |
High BMD | 10 | |
Low BMD | 10 |
PBM is an important determinant of osteoporosis. BMD can be reliably and accurately measured and has high genetic determination with heritability of 0.5–0.9, indicating that genetic factors play an important role in the risk of osteoporosis. GEO, Gene Expression Omnibus; PBM, peak bone mass; BMD, bone mineral density.
DEGs
Limma package (https://www.bioconductor.org/packages/release/bioc/html/limma.html) (21) was used to identify the DEGs in the GSE35959 dataset between osteoporosis samples and normal samples, with the threshold for P<0.05 and |log2 fold change (FC)| >1.
Weighted gene co-expression network analysis (WGCNA) for identifying enriched DEGs
To establish a scale-free co-expression network for the DEGs in the GSE35959 dataset, the WGCNA (22) package in R was used. DEGs were studied using Pearson’s correlation matrices as well as the average-linkage approach. A soft-thresholding factor of β was used to reveal significant correlations between DEGs while penalizing weak correlations, followed by conversion of the adjacency into a topological overlap matrix (TOM), which was determined by the summation of its adjacency with all of the other DEGs across DEGs network, and the calculation of the matching dissimilarity (1-TOM). The criterion for identifying modules with considerable enrichment in DEGs was set at P<0.05.
Protein-protein interaction (PPI)
All human PPIs were obtained from the STRING (https://string-db.org/) database (23). Protein IDs were translated into gene symbols and PPIs without corresponding gene names were eliminated. PPIs were collected if the values of the combined scores were ≥0.8. Cytoscape (Version: 3.7.2) (24) was used to filter and find the network module, and the molecular complex detection (MCODE) plug-in algorithm was used to find the module. The Degree, MNC, and Scloseness algorithm of cytoHubba plug-ins for Cytoscape (Version: 3.7.2) were used to obtain the PPI networks for the DEGs.
Functional enrichment analyses
The analysis of the enrichment pathways of GO and KEGG were conducted utilizing the R program ClusterProfiler (25) for genes to detect the GO terms that displayed over-representation in 3 distinct categories (cellular component, molecular function, and biological processes) and the KEGG pathways. A P value <0.05 was considered statistically significant.
Model creation and verification
The GSE35959 dataset was used as the training dataset and the GSE62402, GSE7158, and GSE13850 datasets were used as the validation dataset. In the training dataset, the hub gene was used as the feature to obtain their corresponding expression spectrum and construct the classification model of support vector machine (26). The survival function was constructed using the receiver operating characteristic (ROC) curve (27).
Ethical statement
The study was conducted in accordance with the Declaration of Helsinki (as revised in 2013).
Statistical analysis
The R package pROC is used for AUC analysis, and the R package ComplexHeatmap is used for heat map drawing. All analysis, except for special instructions, uses default parameters, and data visualization is performed using ggplot2 in version 3.4.3 of R software.
Results
Identification and functional analysis of the DEGs
A total of 1,589 DEGs were detected between osteoporosis and normal samples in the GSE35959 dataset, of which 855 genes were found to be upregulated and 734 genes were downregulated (Figure 2A,2B). GO analysis demonstrated that these DEGs were most significantly enriched in the negative regulation of calcium ion transmembrane transport, contractile actin filament bundle, and transmembrane-ephrin receptor activity. Figure 2C-2E illustrates the top ten GO terms for the DEGs. KEGG pathway analysis illustrated that the DEGs were enriched in 6 pathways, including dilated cardiomyopathy and leukocyte transendothelial migration (Figure 2F).
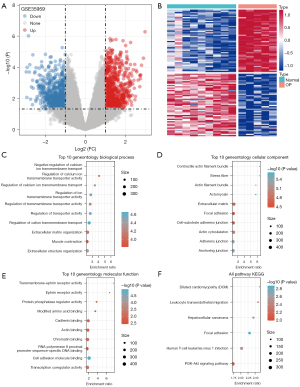
Identification of co-expression modules using WGCNA
The expression profiles of the osteoporosis samples from the GSE35959 dataset were mined using the WGCNA co-expression algorithm for co-expression of coding genes and co-expression modules. The weighted co-expression network was built using the R package WGCNA. When β=12, the co-expression network was shown to be consistent with the scale-free network (Figure 3A). When setting minModuleSize =80, deepSplit =2, and height =0.25, a sum of 16 modules was attained (Figure 3B). The analysis of the association between each module and sample type (osteoporosis vs. normal) as well as age and gender showed that the blue module exhibited a significantly positive association with osteoporosis and a significantly negative correlation with normal samples. The turquoise module showed a significant negative correlation with osteoporosis and a significant negative correlation with normal samples (Figure 3C). The blue and turquoise modules contained 4,172 and 4,637 genes, respectively.
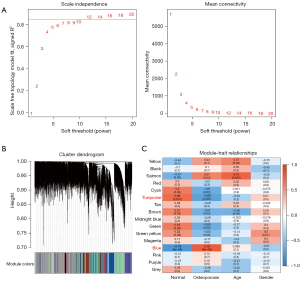
Furthermore, through the R software package WebGestaltR (v0.4.3), 4,172 blue module genes and 4,637 turquoise module genes were analyzed by KEGG and GO function enrichment analysis. Figure 4A-4C depicts the top ten GO terms of the 4,172 genes in the blue model, which were enriched in biological processes, cellular components, as well as molecular functions. The KEGG pathway analysis showed that the 4,172 genes in the blue model were enriched in 56 pathways, including the thyroid hormone signaling pathway, apelin signaling pathway, and tumor necrosis factor (TNF) signaling pathway (Figure 4D). Figure 4E,4F illustrates the top ten enriched GO terms of the 4,637 genes in the turquoise model in cellular components and molecular functions. The KEGG pathway analysis of the 4,637 genes in the turquoise model showed enrichment in 29 pathways, including type I diabetes mellitus (Figure 4G).
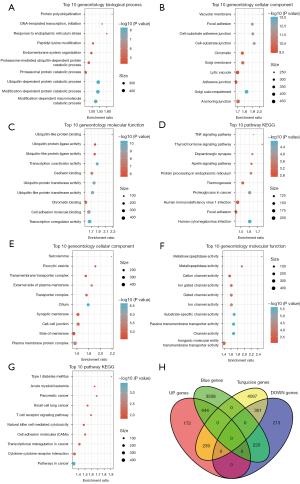
Specifically, we calculated the correlation between genes and modules in key modules and selected key genes with correlation greater than 0.7. Based on this, clusterProfile was used for functional enrichment analysis. A total of 77 GO terms (available online: https://cdn.amegroups.cn/static/public/atm-22-229-1.xlsx) are enriched in blue modules, 242 GO Term pathways and 4 KEGG pathways (available online: https://cdn.amegroups.cn/static/public/atm-22-229-2.xlsx) are enriched in Turquoise modules. In general, the results were similar to those of all genes in the module.
The genes in the blue module and the turquoise module were intersected with the DEGs in the GSE35959 dataset, and 664 genes and 540 genes, respectively, were obtained (Figure 4H).
PPI analysis
The network of protein interactions aids in the discovery of the core modulatory genes. The STRING (https://string-db.org/) database is a repository for searching recognized and anticipated interactions between proteins. STRING analysis of the PPI networks for the 1,204 DEGs produced result files that were filtered using Cytoscape (Version: 3.7.2) to find network modules, and the MCODE plug-in algorithm found one module, MCODE1 (Figure 5). Furthermore, the 56 genes of the MCODE1 module were analyzed by KEGG pathway analysis and GO function enrichment analysis. Figure 6A-6C depicts the top ten GO terms enriched in the MCODE1 model in biological processes, cellular components, and molecular functions. The KEGG pathway analysis showed enrichment in 5 pathways, including the synaptic vesicle cycle (Figure 6D).
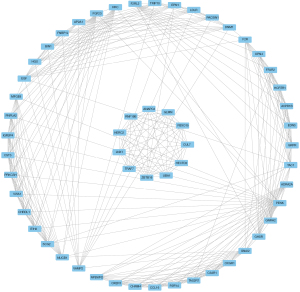
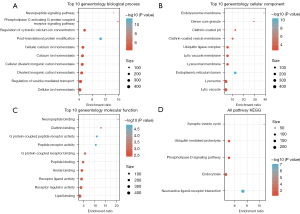
Detection of hub genes
The PPI network of the 1,204 DEGs were analyzed to identify the top 10 hub genes (Figure 7A-7C). The hub genes detected were then traversed, resulting in 6 genes, namely, epidermal growth factor (EGF), tumor protein p53 (TP53), intercellular adhesion molecule 1 (ICAM1), protein kinase camp-activated subunit alpha (PRKACA), paxillin (PXN), and nerve growth factor (NGF) (Figure 7D).
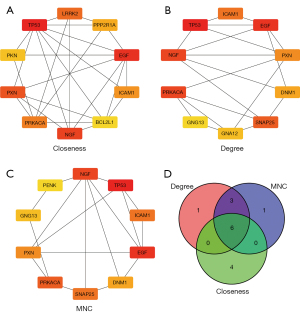
Establishment and confirmation of the diagnostic models
The GSE35959 dataset was used as the training set, while the GSE7158, GSE13850, and GSE7429 datasets were used as the validation set. The 6 hub genes identified above were used as features in the training dataset, and the corresponding expression spectra were acquired to build a support vector machine categorization model with 100% classification accuracy, and 14 out of 14 samples were correctly classified. As illustrated in Figure 8A, the specificity and sensitivity of the model were both 100%, and the area under the ROC curve (AUC) was 1. Validation using the GSE7158 dataset revealed that 25 out of the 26 samples were properly categorized, resulting in a classification accuracy of 96.2%, a model sensitivity of 100%, a specificity of 91.7%, and an AUC of ROC curve of 0.958 (Figure 8B). Verification with the GSE13850 dataset showed that all 40 samples evaluated were properly categorized, resulting in a classification accuracy of 100%, a sensitivity of 100%, a specificity of 100%, and an AUC of ROC curve of 1 (Figure 8C). Validation of the GSE7429 dataset also demonstrated that all 20 samples tested were accurately categorized, resulting in a classification accuracy of 100%, a sensitivity of 100%, a specificity of 100%, and an AUC of ROC curve of 1 (Figure 8D). These findings demonstrated that the diagnostic predictive model developed here could successfully discriminate between osteoporosis samples and normal samples and that the 6 genes identified in this investigation may be employed as viable markers for diagnosing patients with osteoporosis.
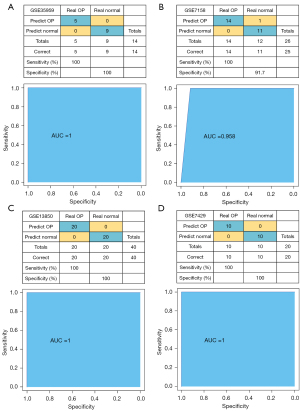
Discussion
Osteoporosis continues to affect millions of people worldwide each year. The disease is characterized by impaired bone mass, resulting in reduced bone formation, a high incidence of brittle fractures, and significant loss of quality of life, especially in the elderly population. Further studies examining bone biology, as well as the molecular mechanisms and signaling networks involved will provide crucial insights that may lead to the discovery of novel treatments (7). Osteoporosis patients in the GSE35959 dataset, as well as healthy controls, were analyzed and compared, and the support vector machine (SVM) classifier method was adopted in combination with the significantly expressed mRNAs. The results showed that the SVM index of the 6 mRNA expression features showed a high AUC in the GSE35959 dataset. The data from different GEO datasets were verified, and the SVM classification algorithm was used for cross-validation analysis. This research was a bioinformatics study, involving differential expression analysis, WGCNA, PPI, KEGG, and GO analysis, of osteoporosis samples in the GEO database. The aim was to identify diagnostic models of osteoporosis for clinical use.
Pharmacological treatment could prevent osteoporotic fractures. Currently, available treatments for osteoporosis are antiresorptive, which inhibits osteoclasts, bone forming, which stimulates osteoblasts, and dual acting that simultaneously stimulates osteoblasts and inhibits osteoclasts (28). Generally, the anti-resorptive options for treatment are receptor activator of nuclear factor κ-B ligand (RANKL) antibody, bisphosphonates, and selective oestroger receptor modulators (SERMs) that either suppress osteoclast recruitment (selective oestrogen receptor modulators and RANKL antibody) or cause osteoclast apoptosis (bisphosphonates). Abaloparatide and Teriparatide [parathyroid hormone (PTH), amino acids 1–34] are regarded as bone-forming treatments, but abaloparatide is currently available only in the US (28). As a dual-acting treatment option, romosozumab is could inhibit bone resorption while at the same time stimulates bone formation (29).
In the present study, a total of 1,589 DEGs were identified and these were mainly associated with the PI3K-Akt signaling pathway and the extracellular matrix. Six hub genes, including EGF, TP53, ICAM1, PRKACA, PXN, and NGF, were identified and used to build a diagnostic model for osteoporosis. Over the past few years, the function of the EGF family members in bone biology has been established. EGF can enhance the proliferation and migration of bone marrow stromal cells (BMSC), which are the major source of interstitial tissue regeneration as they can produce osteoblasts, chondrocytes, and adipocytes. Many human disorders are associated with mutations in the TP53 gene, which results in loss-of-function of the p53 protein (30). The P53 gene transcription modulator not only serves as an advanced age marker, but also performs an important role in the occurrence and progression of osteogenesis (31). Researchers have discovered that p53 may suppress osteogenesis by interfering with the activity of mesenchymal stem cells, which is controlled by the microRNA (miRNA) signaling pathway. Several earlier investigations have shown that ICAM-1 is involved in osteogenic differentiation as well as bone regeneration. Moreover, ICAM-1 has been shown to suppress the osteogenesis of BMSCs and may provide a novel molecular target for accelerating bone re-growth and repair in an inflammatory milieu (32,33). Paxillin is a speck-related regulatory protein that regulates actin dynamics and the cytoskeleton at the onset of cell migration (34). NGF is involved in the differentiation of bones and the repair of fractures (35,36). In osteoporosis, NGF regulates the growth of pro-inflammatory cytokines such as TNF and interleukin (IL)-1 in the bone marrow (37). There have been no reports of protein kinase camp-activated subunit alpha (PRKACA) related to osteoporosis. These results suggested that the 6 hub genes may be used as potential prognostic markers of osteoporosis. Also, we obtained lncRNA-miRNA and gene-miRNA interaction data from starBaseV3 database. Based on ceRNA hypothesis, we constructed statistical models to evaluate the potential relationship between gene and lncRNA. A total of 388 ceRNAs were obtained by selecting FDR <0.01 (available online: https://cdn.amegroups.cn/static/public/atm-22-229-3.xlsx).
This report identified the potential genes for predicting the prognosis of osteoporosis using bioinformatics techniques from a large sample. However, there were some limitations in the present research. First, the clinical follow-up data for the samples were not available, and thus, other possible confounding variables, such as health status, were not considered when identifying biomarkers. Second, the findings acquired from bioinformatics analysis alone are insufficiently persuasive and require additional experimental validation. Therefore, it is necessary to conduct experimental and genetic investigations with larger sample sizes for further validation.
Overall, the 6-gene signature appears to be a potential diagnostic marker for osteoporosis that could separate patients from the general population. These six genes may be potential targets for the treatment of osteoporosis. Therefore, in-depth exploration of the functions of these genes in osteoporosis may provide further important insights for the early detection, prevention, and treatment of osteoporosis.
Acknowledgments
Funding: None.
Footnote
Reporting Checklist: The authors have completed the REMARK reporting checklist. Available at https://atm.amegroups.com/article/view/10.21037/atm-22-229/rc
Conflicts of Interest: All authors have completed the ICMJE uniform disclosure form (available at https://atm.amegroups.com/article/view/10.21037/atm-22-229/coif). The authors have no conflicts of interest to declare.
Ethical Statement: The authors are accountable for all aspects of the work in ensuring that questions related to the accuracy or integrity of any part of the work are appropriately investigated and resolved. The study was conducted in accordance with the Declaration of Helsinki (as revised in 2013).
Open Access Statement: This is an Open Access article distributed in accordance with the Creative Commons Attribution-NonCommercial-NoDerivs 4.0 International License (CC BY-NC-ND 4.0), which permits the non-commercial replication and distribution of the article with the strict proviso that no changes or edits are made and the original work is properly cited (including links to both the formal publication through the relevant DOI and the license). See: https://creativecommons.org/licenses/by-nc-nd/4.0/.
References
- Wade SW, Strader C, Fitzpatrick LA, et al. Estimating prevalence of osteoporosis: examples from industrialized countries. Arch Osteoporos 2014;9:182. [Crossref] [PubMed]
- Kanis JA, Cooper C, Rizzoli R, et al. European guidance for the diagnosis and management of osteoporosis in postmenopausal women. Osteoporos Int 2019;30:3-44. [Crossref] [PubMed]
- Zhao W, Shen G, Ren H, et al. Therapeutic potential of microRNAs in osteoporosis function by regulating the biology of cells related to bone homeostasis. J Cell Physiol 2018;233:9191-208. [Crossref] [PubMed]
- Siris ES, Adler R, Bilezikian J, et al. The clinical diagnosis of osteoporosis: a position statement from the National Bone Health Alliance Working Group. Osteoporos Int 2014;25:1439-43. [Crossref] [PubMed]
- Compston J, Cooper A, Cooper C, et al. Guidelines for the diagnosis and management of osteoporosis in postmenopausal women and men from the age of 50 years in the UK. Maturitas 2009;62:105-8. [Crossref] [PubMed]
- Brown JP, Josse RGScientific Advisory Council of the Osteoporosis Society of Canada. 2002 clinical practice guidelines for the diagnosis and management of osteoporosis in Canada. CMAJ 2002;167:S1-34. [PubMed]
- Rachner TD, Khosla S, Hofbauer LC. Osteoporosis: now and the future. Lancet 2011;377:1276-87. [Crossref] [PubMed]
- Cummings SR, San Martin J, McClung MR, et al. Denosumab for prevention of fractures in postmenopausal women with osteoporosis. N Engl J Med 2009;361:756-65. [Crossref] [PubMed]
- Daddona PE, Matriano JA, Mandema J, et al. Parathyroid hormone (1-34)-coated microneedle patch system: clinical pharmacokinetics and pharmacodynamics for treatment of osteoporosis. Pharm Res 2011;28:159-65. [Crossref] [PubMed]
- Kawai M, Mödder UI, Khosla S, et al. Emerging therapeutic opportunities for skeletal restoration. Nat Rev Drug Discov 2011;10:141-56. [Crossref] [PubMed]
- Boyce EG, Mai Y, Pham C. Abaloparatide: Review of a Next-Generation Parathyroid Hormone Agonist. Ann Pharmacother 2018;52:462-72. [Crossref] [PubMed]
- Saag KG, Petersen J, Brandi ML, et al. Romosozumab or Alendronate for Fracture Prevention in Women with Osteoporosis. N Engl J Med 2017;377:1417-27. [Crossref] [PubMed]
- Chen J, Li K, Pang Q, et al. Identification of suitable reference gene and biomarkers of serum miRNAs for osteoporosis. Sci Rep 2016;6:36347. [Crossref] [PubMed]
- Yang C, Ren J, Li B, et al. Identification of gene biomarkers in patients with postmenopausal osteoporosis. Mol Med Rep 2019;19:1065-73. [PubMed]
- Chen X, Liu G, Wang S, et al. Machine learning analysis of gene expression profile reveals a novel diagnostic signature for osteoporosis. J Orthop Surg Res 2021;16:189. [Crossref] [PubMed]
- Xia B, Li Y, Zhou J, et al. Identification of potential pathogenic genes associated with osteoporosis. Bone Joint Res 2017;6:640-8. [Crossref] [PubMed]
- Clough E, Barrett T. The Gene Expression Omnibus Database. Methods Mol Biol 2016;1418:93-110. [Crossref] [PubMed]
- Gu Q, Tian H, Zhang K, et al. Wnt5a/FZD4 Mediates the Mechanical Stretch-Induced Osteogenic Differentiation of Bone Mesenchymal Stem Cells. Cell Physiol Biochem 2018;48:215-26. [Crossref] [PubMed]
- Chen J, Wang L, Shen Y, et al. Key genes associated with osteoporosis revealed by genome wide gene expression analysis. Mol Biol Rep 2014;41:5971-7. [Crossref] [PubMed]
- Yan B, Li J, Zhang L. Identification of B cells participated in the mechanism of postmenopausal women osteoporosis using microarray analysis. Int J Clin Exp Med 2015;8:1027-34. [PubMed]
- Ritchie ME, Phipson B, Wu D, et al. limma powers differential expression analyses for RNA-sequencing and microarray studies. Nucleic Acids Res 2015;43:e47. [Crossref] [PubMed]
- Langfelder P, Horvath S. WGCNA: an R package for weighted correlation network analysis. BMC Bioinformatics 2008;9:559. [Crossref] [PubMed]
- von Mering C, Huynen M, Jaeggi D, et al. STRING: a database of predicted functional associations between proteins. Nucleic Acids Res 2003;31:258-61. [Crossref] [PubMed]
- Wu X, Sui Z, Zhang H, et al. Integrated Analysis of lncRNA-Mediated ceRNA Network in Lung Adenocarcinoma. Front Oncol 2020;10:554759. [Crossref] [PubMed]
- Yu G, Wang LG, Han Y, et al. clusterProfiler: an R package for comparing biological themes among gene clusters. OMICS 2012;16:284-7. [Crossref] [PubMed]
- Huang S, Cai N, Pacheco PP, et al. Applications of Support Vector Machine (SVM) Learning in Cancer Genomics. Cancer Genomics Proteomics 2018;15:41-51. [PubMed]
- Kamarudin AN, Cox T, Kolamunnage-Dona R. Time-dependent ROC curve analysis in medical research: current methods and applications. BMC Med Res Methodol 2017;17:53. [Crossref] [PubMed]
- Langdahl BL, Harsløf T. Medical treatment of osteoporotic vertebral fractures. Ther Adv Musculoskelet Dis 2011;3:17-29. [Crossref] [PubMed]
- Langdahl BL. Overview of treatment approaches to osteoporosis. Br J Pharmacol 2021;178:1891-906. [Crossref] [PubMed]
- Jia F, Sun R, Li J, et al. Interactions of Pri-miRNA-34b/c and TP53 Polymorphisms on the Risk of Osteoporosis. Genet Test Mol Biomarkers 2016;20:398-401. [Crossref] [PubMed]
- Liu W, Qi M, Konermann A, et al. The p53/miR-17/Smurf1 pathway mediates skeletal deformities in an age-related model via inhibiting the function of mesenchymal stem cells. Aging (Albany NY) 2015;7:205-18. [Crossref] [PubMed]
- Wang J, Zhao ZH, Luo SJ, et al. Expression of osteoclast differentiation factor and intercellular adhesion molecule-1 of bone marrow mesenchymal stem cells enhanced with osteogenic differentiation. Hua Xi Kou Qiang Yi Xue Za Zhi 2005;23:240-3. [PubMed]
- Xu FF, Zhu H, Li XM, et al. Intercellular adhesion molecule-1 inhibits osteogenic differentiation of mesenchymal stem cells and impairs bio-scaffold-mediated bone regeneration in vivo. Tissue Eng Part A 2014;20:2768-82. [Crossref] [PubMed]
- Brown MC, Turner CE. Paxillin: adapting to change. Physiol Rev 2004;84:1315-39. [Crossref] [PubMed]
- Nakanishi T, Takahashi K, Aoki C, et al. Expression of nerve growth factor family neurotrophins in a mouse osteoblastic cell line. Biochem Biophys Res Commun 1994;198:891-7. [Crossref] [PubMed]
- Hukkanen M, Konttinen YT, Santavirta S, et al. Rapid proliferation of calcitonin gene-related peptide-immunoreactive nerves during healing of rat tibial fracture suggests neural involvement in bone growth and remodelling. Neuroscience 1993;54:969-79. [Crossref] [PubMed]
- Mendell LM, Albers KM, Davis BM. Neurotrophins, nociceptors, and pain. Microsc Res Tech 1999;45:252-61. [Crossref] [PubMed]
(English Language Editor: J. Teoh)