Clinical and molecular impacts of tumor mutational burden in histological and cytological specimens from cancer patients
Introduction
The tumor mutational burden (TMB) reflects cancer mutation quantity per tumor genome and is recognized as a predictive biomarker for therapy response of immune checkpoint inhibitor (ICI) (1). A high TMB has been observed to be associated with positive treatment outcomes (2) and indicates enriched clonal neoantigens and elevated immunogenicity of tumor cells, improving the reaction to immunotherapy (3).
Previous study has proven that accurate TMB estimation is full of challenges, possibly resulting in contradictory outcomes in different research with regard to its predictive role (4). Immunosenescence refers to the weakening in the immune system seen with aging which may lead to reduced clearance efficiency in tumor cells, contributing to the increased incidence of cancer in the elderly (5). Applying different specimen types as deoxyribonucleic acid (DNA) sources, such as cytological samples acquired via needle aspiration or brushing and formalin-fixed paraffin-embedded (FFPE) samples, can affect TMB estimation (6). While many studies have relied only on FFPE tissues for TMB assessment (7), for predictive biomarker analyses, cytological specimens fixed by ethanol may be more appropriate, as they present higher DNA quality (8).
The microsatellite instability (MSI) phenotype in tumor cells is broadly employed as a diagnostic biomarker for DNA mismatch repair (MMR) deficiency, which commonly represents the declined or deficient action of the mutSα protein complex (9). Studies have shown that TMB along with MSI-high (MSI-H) are related biomarkers for forecasting tumor reaction to ICI, whereas TMB varies considerably among MSI-H tumors (10). Although MSI is frequently applied as a biomarker in colorectal cancer, MSI-H has been seen in only a few patients with pulmonary adenocarcinoma (11). Patients with low microsatellite stable/microsatellite instability (MSS/MSI) tumors have been observed to be correlated with high TMB (12).
TMB has been demonstrated to vary noticeably among different tumor types as well as among patients with the same tumor type (13). Retrospective studies have associated a high TMB with reaction to ICI in NSCLC (14) and colorectal cancer (15). TMB can be beneficial in recognizing patients with metastatic squamous or non-squamous NSCLC (16), and a smoking-associated cancer type in lung cancer, squamous cell carcinomas (SCC), is likely to have higher TMB (17,18). Tumors possessing the epidermal growth factor receptor (EGFR) mutations have lower TMB, explaining why EGFR-mutated tumors have poor reaction to ICI (19). EGFR T790M mutation is the most common incident contributing to the resistance of EGFR mutant NSCLC patients to the initial EGFR-tyrosine kinase inhibitor (TKI) treatment (20). Previous study shows that Kirsten rat sarcoma viral oncogene homologue (KRAS) mutation can be a genetic molecular marker beneficial to ICI (21), while KRAS mutant tumors have higher TMB as well as better clinical outcomes with ICI in NSCLC (22). Nevertheless, not all KRAS mutations have benefited from immunotherapy, indicating differences in efficacy among KRAS-mutated subtypes (23).
Although most TMB studies have evaluated its association and reaction to ICI, its relationship with clinical parameters has not been well recognized. Based on this, we assessed TMB in cancer patients and evaluated potential factors including age, MSI state, tumor types, and gene mutations that might influence TMB values.
We present the following article in accordance with the MDAR reporting checklist (available at https://atm.amegroups.com/article/view/10.21037/atm-22-358/rc).
Methods
Patient cohort
A total of 496 blood samples and 1,504 tissue samples were collected from cancer patients at the department of Thoracic Oncology in Jiangxi Tumor Hospital between January 2019 and January 2021 for this study. All procedures performed in this study involving human participants were in accordance with the Declaration of Helsinki (as revised in 2013). The study was approved by the ethics committee of Jiangxi Tumor Hospital (No. 2021ky229) and informed consent was taken from all the patients.
DNA extraction and quality control
As previously stated, DNA extraction was performed in accordance with the protocol. Briefly, a QIAamp DNA Blood & Tissue Mini Kit (Qiagen, Hilden, Germany) was used for extracting genomic DNA in whole blood or fresh tumor tissues in accordance with the manufacturer’s instructions. For FFPE specimens, a QIAamp DNA FFPE Tissue Kit (Qiagen) was used to extract genomic DNA, after being deparaffinized with xylene according to the manufacturer’s protocols. Total DNA quantity was evaluated using a spectrophotometer Nanodrop 2000/2000C (Thermo Scientific, Middlesex, MA), and the quality of DNA was validated by migration on agarose gel.
Library preparation and sequencing
The genomic DNA obtained from each sample was fragmented and tailing by TIANSeq Fragment/Repair/Tailing Module (TIANGEN, China). The library was constructed using NEXTflex Rapid DNA-Seq Kit (Bioo Scientific, USA) according to the manufacturer’s guidelines. The WES libraries acquired were purified by AmpureXP beads (Beckman Coulter. Indianapolis, IN, US) and quantified using quantitative PCR (qPCR) by Ion Universal Quantitation Kit (Thermo Fisher). Libraries were diluted into 50 pM prior to loading, and the pools were finally sequenced on an Illumina NavoSeq S4 flowcell (Illumina Inc., San Diego, CA, USA).
Data analysis
Trimmomatic quality control software was used to remove joint sequences, and low-quality sequences in data and comparison software (BWA) was applied to compare the sequenced reads to the reference genome. Error correction and sequence reconstruction software (ECR) was used to identify redundant sequences according to the alignment position of the reads. SNV/InDel detection by MuTect2 software for somatic cell, copy number amplification by cnvPicker software, and fusion gene testing by fusionPicker software was performed. The panel used in the present study was matched and corrected by WES data of 565 lung adenocarcinoma samples from the TCGA-LUAD project in the TCGA database, and reliable values of SNV/InDel were then screened by sequencing error rate and Poisson distribution model to calculate the TMB value. TMB was presented as the number of mutations per megabase, which was determined by dividing the number of coding indels and somatic SNVs (missense and nonsense) by the number of exonic bases (at least 60× coverage). MSI analysis was performed using MSIsensor software, combined with its own algorithm. Statistical analyses were achieved applying GraphPad Prism.
Statistical analysis
The differences of TMB value in different groups were compared using Kruskal-Walis or Wilcoxon test. The relationship between TMB value and MSI ratio were compared by the Simple Linear Regression analysis. P<0.05 was considered as statistical significance.
Results
Patient characteristics
A total of 496 blood samples and 1,504 tissue samples were enrolled, and their characteristics are summarized in Table 1, respectively. We then evaluated the relationship of age, MSI, tumor types, and gene mutations (KRAS and EGFR) with TMB in blood samples and tissue samples.
Table 1
Characteristic | Blood samples | Tissue samples |
---|---|---|
Number of patients (n) | 496 | 1,504 |
Age (mean ± SD) | 61.58±12.48 | 57.42±14.67 |
Sex, n (%) | ||
Male | 314 (63.31) | 866 (57.58) |
Female | 182 (36.69) | 638 (42.42) |
Cancer, n (%) | ||
AUBT (abdominal tumor) | 1 (0.20) | 7 (0.47) |
ASC (lung adenosquamous carcinoma) | 1 (0.20) | 1 (0.07) |
BLCA (bladder cancer) | 3 (0.60) | 23 (1.53) |
BRCA (breast cancer) | 6 (1.21) | 33 (2.19) |
CESC (cervical squamous cell carcinoma) | 12 (2.42) | 13 (0.86) |
CHOL (cholangiocarcinoma) | 9 (1.81) | 46 (3.06) |
COAD (colon adenocarcinoma) | 11 (2.22) | 152 (10.1) |
COAD READ (colorectal cancer) | 4 (0.81) | 31 (2.06) |
DUCA (duodenal cancer) | 1 (0.20) | 5 (0.33) |
ESCA (esophageal cancer) | 11 (2.22) | 18 (1.2) |
GACA (gastrointestinal cancer) | 1 (0.20) | 2 (0.13) |
GBC (gallbladder carcinoma) | 1 (0.20) | 9 (0.6) |
HCC (hepatocellular carcinoma) | 98 (19.8) | 84 (5.59) |
HNSC (head and neck squamous cell carcinoma) | 3 (0.60) | 6 (0.4) |
KICA (kidney cancer) | 5 (1.01) | 33 (2.19) |
KIPAN (mixed kidney cancer) | 1 (0.20) | 1 (0.07) |
KIRC (kidney renal clear cell carcinoma) | 3 (0.60) | 2 (0.13) |
LEIO (leiomyosarcoma) | 1 (0.20) | 3 (0.2) |
LUAD (lung adenocarcinoma) | 210 (42.30) | 482 (32.04) |
LUSC (lung squamous cell carcinoma) | 7 (1.41) | 36 (2.39) |
MEDI (mediastinal tumor) | 1 (0.20) | 0 (0) |
NACA (nasopharyngeal carcinoma) | 6 (1.21) | 7 (0.47) |
NECA (neuroendocrine carcinoma) | 1 (0.20) | 2 (0.13) |
NHL (non-Hodgkin’s lymphoma) | 1 (0.20) | 4 (0.27) |
NSCLC (non-small cell lung cancer) | 5 (1.01) | 5 (0.33) |
ORCA (oropharynx carcinoma) | 4 (0.81) | 3 (0.2) |
Other (other) | 3 (0.6) | 14 (0.93) |
OVCA (ovarian cancer) | 6 (1.21) | 26 (1.73) |
PAAD (pancreatic adenocarcinoma) | 25 (5.04) | 42 (2.79) |
PAGLCA (parotid gland carcinoma) | 1 (0.2) | 3 (0.2) |
PPC (primary peritoneal carcinoma) | 4 (0.81) | 8 (0.53) |
PRAD (prostate adenocarcinoma) | 6 (1.21) | 16 (1.06) |
READ (rectal adenocarcinoma) | 11 (2.22) | 114 (7.58) |
SARC (sarcoma) | 3 (0.60) | 20 (1.33) |
SCLC (small cell lung cancer) | 3 (0.60) | 10 (0.66) |
SEVECA (seminal vesicle carcinoma) | 1 (0.2) | 0 (0) |
SICA (sinonasal carcinomas) | 2 (0.4) | 6 (0.4) |
STAD (stomach adenocarcinoma) | 14 (2.82) | 72 (4.79) |
STES (esophagocardial cancer) | 3 (0.60) | 9 (0.6) |
THCA (thyroid cancer) | 2 (0.40) | 1 (0.07) |
TOCA (tongue cancer) | 1 (0.20) | 0 (0) |
UCEC (uterine corpus endometrial carcinoma) | 2 (0.40) | 13 (0.86) |
URCA (ureter carcinoma) | 1 (0.20) | 4 (0.27) |
UVM (uveal melanoma) | 1 (0.20) | 5 (0.33) |
ACC (adrenocortical carcinoma) | 0 (0) | 3 (0.2) |
AGCS (archos adenocarcinoma) | 0 (0) | 1 (0.07) |
AMCA (bowel cancer) | 0 (0) | 5 (0.33) |
BOCA (cecum cancer) | 0 (0) | 21 (1.4) |
CECA (cecum cancer) | 0 (0) | 3 (0.2) |
ESCC (esophageal squamous cell carcinoma) | 0 (0) | 2 (0.13) |
GBM (glioblastoma) | 0 (0) | 7 (0.47) |
GCT (germ cell tumor) | 0 (0) | 2 (0.13) |
GECECA (germ cell carcinoma) | 0 (0) | 1 (0.07) |
GLIO (gliomas) | 0 (0) | 66 (4.39) |
MB (medulloblastic carcinoma) | 0 (0) | 3 (0.2) |
MENI (meningioma) | 0 (0) | 6 (0.4) |
MESO (mesothelioma pleura) | 0 (0) | 3 (0.2) |
ONB (olfactory neuroblastoma) | 0 (0) | 1 (0.07) |
PECA (penis carcinoma) | 0 (0) | 1 (0.07) |
THYM (thymic carcinoma) | 0 (0) | 4 (0.27) |
UBC (urothelium carcinoma) | 0 (0) | 4 (0.27) |
SD, standard deviation.
TMB characterization
For blood samples (Figure 1), there was no significant difference in TMB between the age 1 group (age ≤56 years old) and age 2 group (56< age <68 years old), while compared to both groups, the TMB value in the age 3 group (age ≥68 years old) was significantly higher (P<0.05, Kruskal-Wallis) (Figure 1A). We then evaluated the MSI state and MSI ratio (%) in blood samples. In a total 496 blood samples, 12 were MSI-H, while 484 were MSS/MSI-L (Figure 1B). The results showed that the ratio (%) of MSI had no linear correlation with TMB (R=−0.093, P=0.038) (Figure 1C). The relationship between tumor type and TMB was also assessed and showed no significant differences in TMB among ASC, LUAD, LUSC, NSCLC, and SCLC samples (P=0.33, P=0.26, respectively, Kruskal-Wallis) (Figure 1D,1E).
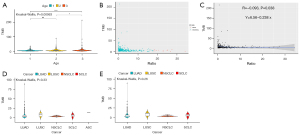
For tissue samples (Figure 2), there was no significant difference in TMB between the age 2 group (53< age <65 years old) and age 3 group (age ≥65 years old), while compared to the age 1 group (age ≤53 years old), TMB was higher in the age 2 group and lower in the age 3 group (P<0.05, Kruskal-Wallis) (Figure 2A). The MSI state and MSI ratio (%) in tissue samples were then exhibited. The results showed that, in a total 1,390 tissue samples, 33 were MSI-H, while 1,357 were MSS/MSI-L (Figure 1B), and the ratio (%) of MSI had no linear correlation with TMB (R=0.29, P<2.2e-16) (Figure 2C). The correlation between tumor type and TMB was also assessed, and differences were discovered in TMB among ASC, LUAD, LUSC, NSCLC, and SCLC samples (P=0.037, Kruskal-Wallis) (Figure 2D). The TMB between blood samples and tissue samples in colorectal cancer were significantly different (P=0.036, Wilcoxon) (Figure 2E).
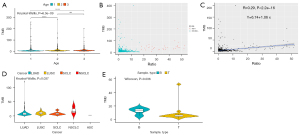
In terms of cancer-associated mutations, TMB among KRAS p.G12A, p.G12C, p.G12D, p.G12R, p.G12V, and KRAS other alterations was not significantly different according to Kruskal-Wallis analysis in blood samples (P=0.17, Kruskal-Wallis) (Figure 3A), while a significant difference of TMB between KRAS other alterations and KRAS p.G12 alteration was identified in blood samples (P=0.013, Wilcoxon) (Figure 3B). Among the 496 blood samples, there was no significance in TMB between EGFR mutation-negative patients (n=484) and EGFR mutation-positive patients (n=12) according to Wilcoxon analysis in blood samples (P=0.11, Wilcoxon) (Figure 3C). We also evaluated the correlation of TMB in blood samples between EGFR T790M mutation-negative and positive patients (Figure 3D).
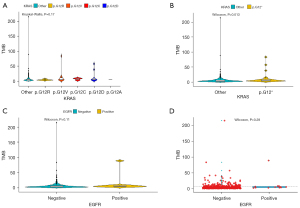
TMB among KRAS p.G12A, p.G12C, p.G12D, p.G12R, p.G12S, p.G12V, and KRAS other alterations showed significant differences in tissue samples, removing the highest value (P=0.033, Kruskal-Wallis) (Figure 4A) or removing the highest two values (P=0.032, Kruskal-Wallis) (Figure 4B). In addition, TMB between KRAS other alterations and KRAS p.G12 alteration showed significance in tissue samples (P=0.0051, Wilcoxon) (Figure 4C). Among the 1504 tissue samples, no correlation was found in TMB between EGFR mutation-negative patients and EGFR mutation-positive patients in tissue samples (P=0.59, Wilcoxon) (Figure 4D).
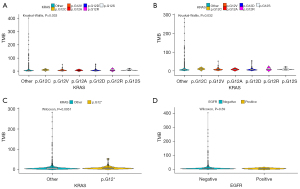
Discussion
ICI is currently applied as standard first-line treatment in NSCLC, and discovering efficient markers to screen prospective benefit is a clinical challenge (24). A high TMB enriches neoantigen-specific T cells to attack tumors, resulting in effective therapeutic outcome (25). TMB is an emerging predictive and prognostic biomarker for immunotherapy (26), and has various prognostic influence in several solid tumor types (27). Some challenges for TMB application in the standard clinical practice are existing, such as the variations of its definition (28). The magnitude of TMB as well changes across different cancer types (29). Additional prospective research on numerous hematological and solid malignancies are required to improve our understanding in TMB as a tissue biomarker (1). It is interesting that TMB and the gene expression signature triggered by T-cell can both present predictive roles for clinical reaction of patients treated in four Keynote trials (30). High TMB is related to the better clinical results, including more durable clinical benefit, longer progression-free survival time and a higher objective response rate (14,31).This study investigated the relationship between TMB and clinical parameters including age, MSI, tumor types, and gene mutations in cancer samples, which may provide a relative effectivity for estimating the TMB value.
Cytological samples present a crucial portion in lung cancer specimens and are sometimes the only sources of cancer cells, such as in pleural effusions (32). Cytological specimens are fixed by alcohol solution, while their DNA is regarded to have equivalent quality with DNA acquired from fresh-frozen tissues (33). We evaluated the effectiveness of blood and tissue samples as the DNA source for TMB estimation and found that both in blood and tissue samples, age and KRAS mutations could affect the TMB value. Differences in TMB were discovered only in tissue samples among several types of lung cancer, including ASC, LUAD, LUSC, NSCLC, and SCLC. It seems that tissue samples are highly suitable for TMB assessment, while at the same time, blood samples may have potential to show a meaningful landscape for its testing. Further prospective investigations are required.
TMB reflects the quantity of cancer mutations, while mutations can be processed to neo-antigens which are displayed by major histocompatibility complex (MHC) proteins (1). In other words, the higher the TMB, the greater the chance that neo-antigens will be immunogenic and recognized by T cells (29,34,35). A high TMB is related to strengthened tumor immunogenicity, which is a critical biomarker in determining immunotherapy efficacy in cancer treatment (1). A high TMB and neo-antigen load in MSI tumors contribute to immune cell infiltration (36). The infiltration of immune cells is a potent predictive and prognostic element in primary non-metastatic tumors (37). Immune cells gradually decrease in quantity and become defective in the process of aging (5), and compared to that in elderly patients, the predictive ability of TMB for ICI is better in young patients with NSCLC (38). In this study, differences in TMB were observed among low, middle, and high age cases, both in blood and tissue samples. What is noteworthy is age itself may not be a suitable independent parameter to impact TMB because of its complicated influences besides host immunity (39). Approximately 15% of MSI-H tumors are because of germline mutations of MMR genes including MSH2, MSH6, PMS2 and MLH1 (1). MSI-H may as well happen due to non-hereditary epigenetic inactivation in MMR genes apart from somatic mutations (40). Actually, approximately 83% of MSI-H cancers also displays high TMB (29). The relationship between clinical outcomes and a high TMB may further be enhanced by the co-occurrence of MSI-H (29,41). Such co-occurrences are seen more commonly in certain tumor types, particularly in gastrointestinal cancers (29). Our results demonstrated no correlation between the MSI ratio and TMB in cancer patients, both in blood and tissue samples. Essentially, MSI-H resulted from DNA mismatch repair deficiency is associated to elevated PD-L1 level. The phenomenon can be explained by the fact that MSI-H phenotype leads to the elevated number of neo-antigens, which attracts T lymphocytes infiltration and therefore stimulates PD-L1 expression (42). MSI-H phenotype and PD-L1 expression seem to be major predictive biomarkers and prognostic factors for immunotherapy efficiency in esophageal and gastric cancer (43).
Previous studies suggest that the high prevalence of TMB varies widely among tumor types (13,44). Driver mutations, including EGFR mutations, are recognized to be a powerful oncogenic phenomenon (45). Advanced patients with EGFR gene mutations in NSCLC always have lower levels of TMB (46), and differences in TMB were discovered among ASC, LUAD, LUSC, NSCLC, and SCLC tissue samples in this study. However, our results suggest EGFR mutation was not associated with TMB, as shown in both blood and tissue samples. KRAS is one of the most prevalent driver genes in NSCLC and is seen in nearly 25% of cases (47). KRAS G12D has been found to be highly mutated in colorectal cancer (48), which can promote the transformation of myeloid-derived suppressor cells (MDSCs) and Treg through blocking interferon regulatory factor 2 (IRF2)-chemokine (C-X-C motif) ligand 3 (CXCL3) signaling, driving immune inhibition and immunotherapy resistance (49). Co-mutation of TP53 and KRAS G12D has outstanding impact on declined TMB and decreased immune cell infiltration (23). Previous study has found that KRAS mutations considerably increase TMB, whereas subgroup analysis demonstrates a seriously low TMB in KRAS G12D mutation (23). A significant difference of TMB between KRAS other alterations and p.G12 alteration was identified in blood samples, while in tissue samples, TMB in KRAS p.G12A, p.G12C, p.G12D, p.G12R, p.G12S, p.G12V, and KRAS other alterations showed significant differences.
In conclusion, TMB may be affected by age, tumor types, and KRAS mutations. Given it is considered as a potent potential biomarker for ICI, it is likely that analyzing age, tumor types, and KRAS mutations may provide beneficial assistance for TMB assessment.
Acknowledgments
Funding: None.
Footnote
Reporting Checklist: The authors have completed the MDAR reporting checklist. Available at https://atm.amegroups.com/article/view/10.21037/atm-22-358/rc
Data Sharing Statement: Available at https://atm.amegroups.com/article/view/10.21037/atm-22-358/dss
Conflicts of Interest: All authors have completed the ICMJE uniform disclosure form (available at https://atm.amegroups.com/article/view/10.21037/atm-22-358/coif). CC is from Shenzhen Cheerland Biotechnology Co., Ltd. CL and LN are from CheerLand Clinical Laboratory Co., Ltd. The other authors have no conflicts of interest to declare.
Ethical Statement: The authors are accountable for all aspects of the work in ensuring that questions related to the accuracy or integrity of any part of the work are appropriately investigated and resolved. All procedures performed in this study involving human participants were in accordance with the Declaration of Helsinki (as revised in 2013). The study was approved by the ethics committee of Jiangxi Tumor Hospital (No. 2021ky229) and informed consent was taken from all the patients.
Open Access Statement: This is an Open Access article distributed in accordance with the Creative Commons Attribution-NonCommercial-NoDerivs 4.0 International License (CC BY-NC-ND 4.0), which permits the non-commercial replication and distribution of the article with the strict proviso that no changes or edits are made and the original work is properly cited (including links to both the formal publication through the relevant DOI and the license). See: https://creativecommons.org/licenses/by-nc-nd/4.0/.
References
- Jardim DL, Goodman A, de Melo Gagliato D, et al. The Challenges of Tumor Mutational Burden as an Immunotherapy Biomarker. Cancer Cell 2021;39:154-73. [Crossref] [PubMed]
- Chan TA, Yarchoan M, Jaffee E, et al. Development of tumor mutation burden as an immunotherapy biomarker: utility for the oncology clinic. Ann Oncol 2019;30:44-56. [Crossref] [PubMed]
- McGranahan N, Furness AJ, Rosenthal R, et al. Clonal neoantigens elicit T cell immunoreactivity and sensitivity to immune checkpoint blockade. Science 2016;351:1463-9. [Crossref] [PubMed]
- McNamara MG, Jacobs T, Lamarca A, et al. Impact of high tumor mutational burden in solid tumors and challenges for biomarker application. Cancer Treat Rev 2020;89:102084. [Crossref] [PubMed]
- Elias R, Hartshorn K, Rahma O, et al. Aging, immune senescence, and immunotherapy: A comprehensive review. Semin Oncol 2018;45:187-200. [Crossref] [PubMed]
- Jain D, Roy-Chowdhuri S. Molecular Pathology of Lung Cancer Cytology Specimens: A Concise Review. Arch Pathol Lab Med 2018;142:1127-33. [Crossref] [PubMed]
- Allgäuer M, Budczies J, Christopoulos P, et al. Implementing tumor mutational burden (TMB) analysis in routine diagnostics-a primer for molecular pathologists and clinicians. Transl Lung Cancer Res 2018;7:703-15. [Crossref] [PubMed]
- Pisapia P, Malapelle U, Roma G, et al. Consistency and reproducibility of next-generation sequencing in cytopathology: A second worldwide ring trial study on improved cytological molecular reference specimens. Cancer Cytopathol 2019;127:285-96. [Crossref] [PubMed]
- Salem ME, Bodor JN, Puccini A, et al. Relationship between MLH1, PMS2, MSH2 and MSH6 gene-specific alterations and tumor mutational burden in 1057 microsatellite instability-high solid tumors. Int J Cancer 2020;147:2948-56. [Crossref] [PubMed]
- Salem ME, Puccini A, Grothey A, et al. Landscape of Tumor Mutation Load, Mismatch Repair Deficiency, and PD-L1 Expression in a Large Patient Cohort of Gastrointestinal Cancers. Mol Cancer Res 2018;16:805-12. [Crossref] [PubMed]
- Warth A, Körner S, Penzel R, et al. Microsatellite instability in pulmonary adenocarcinomas: a comprehensive study of 480 cases. Virchows Arch 2016;468:313-9. [Crossref] [PubMed]
- Pedersen KS, Foster NR, Overman MJ, et al. ZEBRA: A Multicenter Phase II Study of Pembrolizumab in Patients with Advanced Small-Bowel Adenocarcinoma. Clin Cancer Res 2021;27:3641-8. [Crossref] [PubMed]
- Yarchoan M, Albacker LA, Hopkins AC, et al. PD-L1 expression and tumor mutational burden are independent biomarkers in most cancers. JCI Insight 2019;4:e126908. [Crossref] [PubMed]
- Hellmann MD, Ciuleanu TE, Pluzanski A, et al. Nivolumab plus Ipilimumab in Lung Cancer with a High Tumor Mutational Burden. N Engl J Med 2018;378:2093-104. [Crossref] [PubMed]
- Le DT, Durham JN, Smith KN, et al. Mismatch repair deficiency predicts response of solid tumors to PD-1 blockade. Science 2017;357:409-13. [Crossref] [PubMed]
- Ettinger DS, Wood DE, Aggarwal C, et al. NCCN Guidelines Insights: Non-Small Cell Lung Cancer, Version 1.2020. J Natl Compr Canc Netw 2019;17:1464-72. [Crossref] [PubMed]
- Greillier L, Tomasini P, Barlesi F. The clinical utility of tumor mutational burden in non-small cell lung cancer. Transl Lung Cancer Res 2018;7:639-46. [Crossref] [PubMed]
- Kobayashi H, Serizawa M, Naito T, et al. Characterization of tumour mutation burden in patients with non-small cell lung cancer and interstitial lung disease. Respirology 2020;25:850-4. [Crossref] [PubMed]
- Offin M, Rizvi H, Tenet M, et al. Tumor Mutation Burden and Efficacy of EGFR-Tyrosine Kinase Inhibitors in Patients with EGFR-Mutant Lung Cancers. Clin Cancer Res 2019;25:1063-9. [Crossref] [PubMed]
- Ishii H, Azuma K, Sakai K, et al. Determination of Somatic Mutations and Tumor Mutation Burden in Plasma by CAPP-Seq during Afatinib Treatment in NSCLC Patients Resistance to Osimertinib. Sci Rep 2020;10:691. [Crossref] [PubMed]
- Dong ZY, Zhong WZ, Zhang XC, et al. Potential Predictive Value of TP53 and KRAS Mutation Status for Response to PD-1 Blockade Immunotherapy in Lung Adenocarcinoma. Clin Cancer Res 2017;23:3012-24. [Crossref] [PubMed]
- Lee CK, Man J, Lord S, et al. Clinical and Molecular Characteristics Associated With Survival Among Patients Treated With Checkpoint Inhibitors for Advanced Non-Small Cell Lung Carcinoma: A Systematic Review and Meta-analysis. JAMA Oncol 2018;4:210-6. [Crossref] [PubMed]
- Gao G, Liao W, Ma Q, et al. KRAS G12D mutation predicts lower TMB and drives immune suppression in lung adenocarcinoma. Lung Cancer 2020;149:41-5. [Crossref] [PubMed]
- Sholl LM, Hirsch FR, Hwang D, et al. The Promises and Challenges of Tumor Mutation Burden as an Immunotherapy Biomarker: A Perspective from the International Association for the Study of Lung Cancer Pathology Committee. J Thorac Oncol 2020;15:1409-24. [Crossref] [PubMed]
- Schumacher TN, Schreiber RD. Neoantigens in cancer immunotherapy. Science 2015;348:69-74. [Crossref] [PubMed]
- Klempner SJ, Fabrizio D, Bane S, et al. Tumor Mutational Burden as a Predictive Biomarker for Response to Immune Checkpoint Inhibitors: A Review of Current Evidence. Oncologist 2020;25:e147-59. [Crossref] [PubMed]
- Panda A, Betigeri A, Subramanian K, et al. Identifying a Clinically Applicable Mutational Burden Threshold as a Potential Biomarker of Response to Immune Checkpoint Therapy in Solid Tumors. JCO Precis Oncol 2017;2017:PO.17.00146.
- Stenzinger A, Endris V, Budczies J, et al. Harmonization and Standardization of Panel-Based Tumor Mutational Burden Measurement: Real-World Results and Recommendations of the Quality in Pathology Study. J Thorac Oncol 2020;15:1177-89. [Crossref] [PubMed]
- Chalmers ZR, Connelly CF, Fabrizio D, et al. Analysis of 100,000 human cancer genomes reveals the landscape of tumor mutational burden. Genome Med 2017;9:34. [Crossref] [PubMed]
- Cristescu R, Mogg R, Ayers M, et al. Pan-tumor genomic biomarkers for PD-1 checkpoint blockade-based immunotherapy. Science 2018;362:eaar3593. [Crossref] [PubMed]
- Ready N, Hellmann MD, Awad MM, et al. First-Line Nivolumab Plus Ipilimumab in Advanced Non-Small-Cell Lung Cancer (CheckMate 568): Outcomes by Programmed Death Ligand 1 and Tumor Mutational Burden as Biomarkers. J Clin Oncol 2019;37:992-1000. [Crossref] [PubMed]
- Roy-Chowdhuri S, Chow CW, Kane MK, et al. Optimizing the DNA yield for molecular analysis from cytologic preparations. Cancer Cytopathol 2016;124:254-60. [Crossref] [PubMed]
- Balla A, Hampel KJ, Sharma MK, et al. Comprehensive Validation of Cytology Specimens for Next-Generation Sequencing and Clinical Practice Experience. J Mol Diagn 2018;20:812-21. [Crossref] [PubMed]
- Chabanon RM, Pedrero M, Lefebvre C, et al. Mutational Landscape and Sensitivity to Immune Checkpoint Blockers. Clin Cancer Res 2016;22:4309-21. [Crossref] [PubMed]
- Rooney MS, Shukla SA, Wu CJ, et al. Molecular and genetic properties of tumors associated with local immune cytolytic activity. Cell 2015;160:48-61. [Crossref] [PubMed]
- Picard E, Verschoor CP, Ma GW, et al. Relationships Between Immune Landscapes, Genetic Subtypes and Responses to Immunotherapy in Colorectal Cancer. Front Immunol 2020;11:369. [Crossref] [PubMed]
- Pagès F, Kirilovsky A, Mlecnik B, et al. In situ cytotoxic and memory T cells predict outcome in patients with early-stage colorectal cancer. J Clin Oncol 2009;27:5944-51. [Crossref] [PubMed]
- Wu Y, Xu J, Xu J, et al. The predictive value of tumor mutation burden for immune checkpoint inhibitors therapy in non-small cell lung cancer is affected by patients' age. Biomark Res 2020;8:9. [Crossref] [PubMed]
- Pawelec G. Does patient age influence anti-cancer immunity? Semin Immunopathol 2019;41:125-31. [Crossref] [PubMed]
- Bonneville R, Krook MA, Kautto EA, et al. Landscape of Microsatellite Instability Across 39 Cancer Types. JCO Precis Oncol 2017;2017:PO.17.00073.
- Strickland KC, Howitt BE, Shukla SA, et al. Association and prognostic significance of BRCA1/2-mutation status with neoantigen load, number of tumor-infiltrating lymphocytes and expression of PD-1/PD-L1 in high grade serous ovarian cancer. Oncotarget 2016;7:13587-98. [Crossref] [PubMed]
- Kim JH, Park HE, Cho NY, et al. Characterisation of PD-L1-positive subsets of microsatellite-unstable colorectal cancers. Br J Cancer 2016;115:490-6. [Crossref] [PubMed]
- Vrána D, Matzenauer M, Neoral Č, et al. From Tumor Immunology to Immunotherapy in Gastric and Esophageal Cancer. Int J Mol Sci 2018;20:13. [Crossref] [PubMed]
- Alexandrov LB, Nik-Zainal S, Wedge DC, et al. Signatures of mutational processes in human cancer. Nature 2013;500:415-21. [Crossref] [PubMed]
- Harrison PT, Vyse S, Huang PH. Rare epidermal growth factor receptor (EGFR) mutations in non-small cell lung cancer. Semin Cancer Biol 2020;61:167-79. [Crossref] [PubMed]
- Dong ZY, Zhang JT, Liu SY, et al. EGFR mutation correlates with uninflamed phenotype and weak immunogenicity, causing impaired response to PD-1 blockade in non-small cell lung cancer. Oncoimmunology 2017;6:e1356145. [Crossref] [PubMed]
- Pinto R, Petriella D, Lacalamita R, et al. KRAS-Driven Lung Adenocarcinoma and B Cell Infiltration: Novel Insights for Immunotherapy. Cancers (Basel) 2019;11:1145. [Crossref] [PubMed]
- Knight JRP, Alexandrou C, Skalka GL, et al. MNK Inhibition Sensitizes KRAS-Mutant Colorectal Cancer to mTORC1 Inhibition by Reducing eIF4E Phosphorylation and c-MYC Expression. Cancer Discov 2021;11:1228-47. [Crossref] [PubMed]
- Liao W, Overman MJ, Boutin AT, et al. KRAS-IRF2 Axis Drives Immune Suppression and Immune Therapy Resistance in Colorectal Cancer. Cancer Cell 2019;35:559-572.e7. [Crossref] [PubMed]