Identification of core genes associated with type 2 diabetes mellitus and gastric cancer by bioinformatics analysis
Introduction
Among all malignancies worldwide, gastric cancer (GC) ranks fifth in morbidity and third in mortality. Approximately one million people are diagnosed with GC each year, with about 783,000 deaths annually (1,2). There are numerous risk factors for GC, including obesity, smoking, and Helicobacter pylori (H. pylori) infection (3). Although the overall incidence of GC has declined globally over the past 30 years, the number of new cases remains substantial.
Previous studies have demonstrated that diabetes is associated with an increased risk of breast cancer, liver cancer, pancreatic cancer, colorectal cancer, endometrial cancer, renal cancer, non-Hodgkin’s lymphoma, and bladder cancer, among others (3). This increase in the risk of cancer among diabetics may be caused by poor blood sugar control, insulin resistance (IR), and oxidative stress. Epidemiological studies have shown that diabetes or hyperglycemia may increase the incidence or mortality of GC to some extent (1,4). A large number of reactive oxygen species (ROS) such as superoxide anions were produced during the catabolism of glucose in high glucose environment. However, the expression level of antioxidant enzymes in tumor cells is low, and the ability of scavenging ROS is reduced. Therefore, the oxidative stress is aggravated by high glucose environment, resulting in DNA damage and enzyme synthesis disorder, thus inducing carcinogenesis. At the same time, higher blood glucose levels disrupt the body’s energy balance, weaken the effect of ascorbic acid on cellular metabolism, and reduce the body’s immunity (5). The GC patients are in a long-term stress state due to surgery or chemotherapy. In order to adapt to this stress state and resist the harsh tumor micro-environment, GC cells start a series of self-defense mechanisms, such as drug efflux and DNA damage repair. This process requires a large amount of ATP consumption (6). Glucose is the main source of ATP. Since cancer cells cannot fully use glucose for energy supply, they will consume more glucose than normal cells, and high blood glucose just provides energy for self-repair and growth of cancer cells. Also, the expression of pro-inflammatory cytokines, such as interleukin-1, interleukin-6, and tumor necrosis factor-α can be increased in diabetic patients (7), which may up-regulate and activate GC-associated pathways, such as the Wnt/β-catenin pathway. Long-term inflammation can lead to tumorigenesis. IL-6, as a cancer-related inflammatory cytokine, plays a key role in inflammatory effects and tumorigenesis. IL-6 binds to its receptor (IL-6R) to activate different signal transduction pathways, promoting tumor cell proliferation, survival and metastasis, and strongly inhibiting anti-tumor immune response. Studies have shown that IL-6 and tumor necrosis factor-α released by infiltrating macrophages in GC can induce programmed death protein ligand 1 (PD-L1) expression in GC cells by activating NF-κB and STAT3 signaling pathways. PD-L1 helps GC cells avoid cytotoxic T cell killing and promotes the proliferation of GC cells (8). Studies have shown that insulin is a key regulator of many cancers, including GC (9). Persistent hyperinsulinemia caused by IR, which could inhibit insulin-like growth factor 1 (IGF-1) binding protein production and in turn increase the bioavailability of IFG-1, could be a contributor to the pathogenesis of GC (10). IGF-1 can activate the insulin signaling pathway through insulin-like growth factor 1 receptor (IGF1R) to inhibit cell apoptosis, stimulate cell proliferation, and promote the occurrence of GC. However, most previous studies were focused on epidemiology, with only a few studies investigating the possible genetic relationships between GC, diabetes mellitus (DM), and type 2 diabetes mellitus (T2DM).
In this study, we highlighted the potential pathophysiological link between DM, T2DM, and GC by bioinformatics methods. The GeneCards database was used to screen DM-, T2DM-, and GC-related genes, and a protein-protein interaction (PPI) network of the genes/proteins associated with overlapping genes between DM, T2DM, and GC was constructed. Functional enrichment analysis was performed for the two gene clusters, and ten genes with high connectivity were identified as pivot genes. Prognostic analysis and gene expression analysis of hub genes were also performed. Finally, caspase 3 (CASP3), and tumor protein P53 (TP53) were identified as core genes. We explored whether diabetes or hyperglycemia are risk factors for the occurrence and development of GC, deeply revealed the molecular biological relationship between diabetes and GC for the first time, and provided theoretical basis for the prevention of the occurrence and development of GC. We present the following article in accordance with the STREGA reporting checklist (available at https://atm.amegroups.com/article/view/10.21037/atm-21-3635/rc).
Methods
Data collection
We searched for keywords such as “DM”, “diabetes mellitus”; “T2DM”, “type 2 diabetes mellitus”; and “gastric cancer”, “GC” in GeneCards(https://www.genecards.org/) to screen DM-, T2DM- and GC-related genes. Venny 2.1 (http://bioinfogp.cnb.csic.es/tools/venny/) was used to overlap the screened DM-, T2DM-, and GC-related genes with restriction of the relevance score >10. The results were input into Agilent Literature Search software v. 3.1.1 (U.S., L2FProd.com) to screen genes/proteins associated with overlapping genes.
PPI network construction, module analysis, and hub gene identification
Firstly, the PPI information of the genes/proteins related to the overlapping genes was obtained by using the STRING online database (http://string-db.org). Cytoscape software (version 3.6.1) (U.S. National Institute of General Medical Sciences) was used to detect the PPI relationship network (11). The Molecular Complex Detection (MCODE) plugin in Cytoscape was used to identify the most significant module in the network based on the graph-theoretic clustering algorithm (12). Finally, CytoHubba, a Cytoscapeplugin, was utilized to explore hub genes in the PPI network, which provides a user-friendly interface to explore important nodes in biological networks and performs calculations using eleven methods (13).
Gene Ontology (GO) function and Kyoto Encyclopedia of Genes and Genomes (KEGG) pathway analyses
The Database for Annotation, Visualization, and Integrated Discovery (DAVID https://david.ncifcrf.gov/) provides practical annotation tools to recognize biological functions of genes (14). To ascertain the sophisticated functions of biological systems at the molecular level, the KEGG could be used a data resource. Meanwhile, enrichment analysis could be performed using the GO database. We performed KEGG pathway and enrichment analysis involving cellular component, biological process, and molecular function for the selected PPI networks and gene modules using DAVID.
Analysis of hub genes
The Kaplan-Meier plotter (http://kmplot.com/analysis/) could evaluate the effect of 54,675 genes on survival using samples from 18,674 cancer patients (15). These include 5,143 breast, 2,437 lung, 1,816 ovarian, 1,065 gastric, and 364 liver cancer patients, with information concerning overall and relapse-free survival, which were predominantly retrieved from the Gene Expression Omnibus (GEO), The Cancer Genome Atlas (TCGA), and European Genome-phenome Archive (EGA) databases (16). To determine the prognostic value of hub genes, Kaplan-Meier survival analysis of these genes was carried out using the Kaplan-Meier plotter database. Analysis of hub genes’ expression and their expression profiles in human tissues was conducted using Gene Expression Profiling Interactive Analysis (GEPIA) (http://gepia.cancer-pku.cn), which is an internet-based server offering cancer and normal gene expression profiling and interactive analysis. UALCAN (ualcan.path.uab.edu), an accessible, interactive Internet resource for the analysis of cancer transcriptome data (17), was utilized to confirm the expression of hub genes and to analyze their clinicopathological characteristics.
Patients
To confirm and validate the expression of the 3 common hub genes, Tumor tissues from 3 GC patients and 3 GC complicated with type 2 diabetes patients were obtained during operation in Shanxi Bethune Hospital between July 2022 and November 2022. The corresponding normal tissues were obtained from 5 cm away from the tumor tissue. The present study was approved by the Ethics Committee of Shanxi Bethune Hospital (No. YXLL-2021-063). All participants in the study signed informed consent forms. The study was conducted in accordance with the Declaration of Helsinki (as revised in 2013).
Verification in the clinical samples: western blotting
We extracted proteins from normal and adjacent tumor tissues and tested their concentrations using BCA protein kits (Boster, Wuhan, China). Target proteins were isolated with SDS-PAGE (10%), and transferred to nitrate fiber membrane (4 ℃, 270 mA, 1.5 h). Then we sealed the nitrate fiber membrane with 5% skim milk powder for 3 h. The membrane was incubated with rabbitanti-CASP3 antibody (1:2,000; cat. no. ab184787; Abcam) or mouse anti‑glyceraldehyde-3-phosphate dehydrogenase (GAPDH) antibody (1:5,000; cat. no. ab8245; Abcam) orrabbit anti-TP53 antibody (1:500; cat. no. M00001-4; Boster) orrabbit anti-β-actin antibody (1:500; cat. no. WL0002d; Wanleibio) at 4 ℃ over-night. Wash unbound protein 3 times with 5% TBST solution., and the membrane was incubated with HRP Goat Anti-Rabbit IgG (H+L) secondary antibody (1:4,000; AS014; Abclonal) or HRP Goat Anti-Mouse IgG (H+L) secondary antibody (1:4,000; E-AB-1001; ELAbscience) for 1 h. Following washing with TBST (3 times). ECL western blot detection kit (Affinity Biosciences, USA) was used to visualize the protein bands. β-actin was used as internal references for protein. The molecular weight and net optical density of target band were analyzed by Image J. analysis software, and the results represented the relative content of target protein.
Statistical analysis
SPSS 20.0 was used for statistical analysis of the data. Measurement data were expressed as mean ± standard deviation, conforming to normal distribution and homogeneity of variance. t-test was used to compare the mean values of two samples, and P<0.05 was considered statistically significant.
Results
Identification of overlapping DM-, T2DM-, and GC-related genes
We screened 1,152 DM-related genes, 466 GC-related genes, and 531 T2DM-related genes obtained from GeneCards, with restriction of the relevance score >10. A total of 59 overlapping genes were identified in the three diseases (Figure 1). Next, we screened 401 genes/proteins associated with these 59 overlapping genes using Agilent Literature Search software v. 3.1.1 (U.S., L2FProd.com).
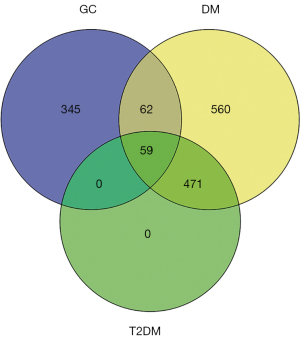
Construction of the PPI network, module analysis, and identification of hub genes
PPIs of the 401 genes/proteins associated with the 59 overlapping genes were constructed with a confidence score of ≥0.4. Subsequently, the PPI network was identified using Cytoscape software (version 3.6.1) (U.S. National Institute of General Medical Sciences). The PPI network maps yielded 359 nodes and 8,104 edges. Whole PPI network analysis was carried out with MCODE (U.S. National Institute of General Medical Sciences), and two significant modules (module 1 and 2), which had higher scores, were later chosen (Figure 2A,2B). Module 1 yielded 52 nodes and 898 edges, while module 2 yielded 69 nodes and 1,101 edges. The 10 genes with the highest degree of connectivity (using the Degree method) were selected by the CytoHubba plugin and were arranged in a sequence as follows: TNF, MAPK14, EGFR, CXCL8, BCL2L1, MAPK8, MMP9, ESR1, PTGS2, FOS were hub nodes with higher node degrees in module 1; and IL6, MAPK3, AKT1, TP53, GAPDH, STAT3, JUN, CASP3, VEGFA, and MYC were hub nodes with higher node degrees in module 2.
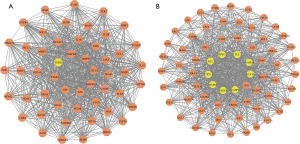
GO function and KEGG pathway analyses
GO analysis revealed PPI network genes that are involved in numerous biological processes (BP), including positive regulation of transcription from ribonucleic acid (RNA) polymerase II promoter, negative regulation of apoptosis, and positive regulation of gene expression. Concerning cellular components, the PPI network genes were enriched in the cytosol, nucleoplasm, and extracellular space, and were primarily associated with protein binding, enzyme binding, and transcription factor activity with regards to molecular functions (Figure 3A). The PPI network genes were enriched in three KEGG pathways, including Pathways in cancer, Proteoglycans in cancer, and the hypoxia inducible factor-1 (HIF-1) signaling pathway (Figure 3B, Table 1).
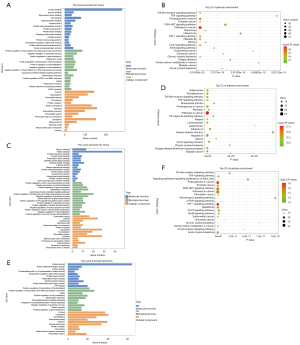
Table 1
Category | Term | Count | % | P value |
---|---|---|---|---|
KEGG_PATHWAY | hsa05200: Pathways in cancer | 78 | 21.36986 | 1.28E-33 |
KEGG_PATHWAY | hsa05205: Proteoglycans in cancer | 53 | 14.52055 | 1.10E-28 |
KEGG_PATHWAY | hsa04066: HIF-1 signaling pathway | 39 | 10.68493 | 1.33E-28 |
KEGG_PATHWAY | hsa04068: FoxO signaling pathway | 42 | 11.50685 | 1.09E-25 |
KEGG_PATHWAY | hsa05161: Hepatitis B | 43 | 11.78082 | 3.03E-25 |
PPI, protein-protein interaction; KEGG, Kyoto Encyclopedia of Genes and Genomes; HIF-1, hypoxia-inducible factor 1; FoxO, Forkhead boxO.
GO analysis revealed module 1 genes involved in numerous BP, including positive regulation of nitric oxide biosynthetic process, the cellular response to mechanical stimulus, and positive regulation of gene expression. Regarding cellular components, module 1 genes were most probably enriched in the extracellular space, cytosol, and cell surface, and were mainly associated with enzyme binding, protein binding, and protein N-terminus binding in terms of molecular functions (Figure 3C). The module 1 genes were enriched in three KEGG pathways, including Pathways in cancer, Hepatitis B, and bladder cancer (Figure 3D, Table 2).
Table 2
Category | Term | Count | % | P value |
---|---|---|---|---|
KEGG_PATHWAY | hsa05200: Pathways in cancer | 26 | 50 | 2.36E-18 |
KEGG_PATHWAY | hsa05161: Hepatitis B | 17 | 32.69231 | 2.53E-15 |
KEGG_PATHWAY | hsa05219: Bladder cancer | 12 | 23.07692 | 3.11E-15 |
KEGG_PATHWAY | hsa05140: Leishmaniasis | 13 | 25 | 6.41E-14 |
KEGG_PATHWAY | hsa05142: Chagas disease (American trypanosomiasis) | 14 | 26.92308 | 2.97E-13 |
KEGG, Kyoto Encyclopedia of Genes and Genomes.
GO analysis identified module 2 genes that play a part in numerous BP, including positive regulation of transcription, deoxyribonucleic acid (DNA) templating, positive regulation of transcription from RNA polymerase II promoter, aging, and positive regulation of cell proliferation. In terms of cellular components, module 2 genes were mostly enriched in the cytosol, cytoplasm, nucleoplasm, extracellular space, and were mainly associated with protein binding, protein heterodimerization activity, and kinase activity (Figure 3E). The module 2 genes were enriched in three KEGG pathways, including the HIF-1 signaling pathway, Proteoglycans in cancer, and acute myeloid leukemia (Figure 3F, Table 3).
Table 3
Category | Term | Count | % | P value |
---|---|---|---|---|
KEGG_PATHWAY | hsa04066: HIF-1 signaling pathway | 19 | 27.53623 | 5.40E-19 |
KEGG_PATHWAY | hsa05205: Proteoglycans in cancer | 23 | 33.33333 | 5.85E-18 |
KEGG_PATHWAY | hsa05221: Acute myeloid leukemia | 15 | 21.73913 | 8.53E-17 |
KEGG_PATHWAY | hsa05200: Pathways in cancer | 27 | 39.13043 | 1.04E-15 |
KEGG_PATHWAY | hsa05215: Prostatecancer | 16 | 23.18841 | 3.01E-15 |
KEGG, Kyoto Encyclopedia of Genes and Genomes; HIF-1, hypoxia-inducible factor 1.
Analysis of hub genes
The Kaplan-Meier survival plot was used for the analysis of overall survival (OS) in 876 patients with GC. The 10 hub genes (IL6, MAPK3, EGFR, AKT1, TP53, GAPDH, STAT3, CASP3, VEGFA, and MYC) were uploaded to the database, and Kaplan-Meier curves were plotted. The results of the prognostic analysis showed that GAPDH [hazard rate (HR) =1.84 (1.55−2.18), P=1.6e−12], CASP3 (apopain) [HR =0.5 (0.42−0.6), P=7.2e−14], MYC [HR =0.65 (0.55–0.78), P=2.7e−06], TP53 [HR =1.7 (1.41−2.05), P=1.9e−08], AKT1 [HR =2.33 (1.91−2.86), P<1E−16], EGFR [HR =1.35 (1.08−1.7), P=0.0092], MAPK3 [HR =1.58 (1.33−1.88), P=1.2e−07], and VEGFA [HR =1.53 (1.27−1.84), P=7.6e−06] were considerably associated with OS in patients with GC (Figure 4). IL6 and STAT3 expression was not related to survival. The results of GEPIA analysis showed that the messenger RNA (mRNA) expression levels of GAPDH, CASP3 and TP53 were significantly elevated in tumor tissues compared to normal tissues (Figure 5). Therefore, according to the differential expression, GAPDH, CASP3 and TP53 were selected as key factors. To verify the above results, the expression profiles of GAPDH, CASP3, and TP53 in tumor tissues higher than that in normal tissues, and their associations with patients’ clinical characteristics were determined using UALCAN (Figure 6A-6F). The UALCAN results were consistent with those of GEPIA.
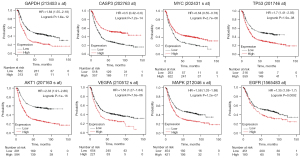
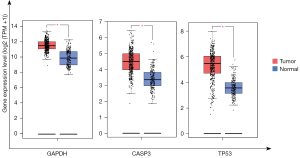
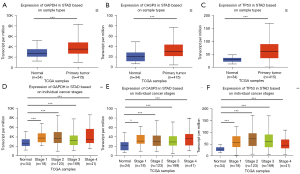
Validation by western blotting
In the present study, to confirm protein expression levels of 3 hub genes, western blotting was performed on GC patient and GC complicated with type 2 diabetes samples. Figure 7 and Figure 8 showed the protein expression results of all the key genes. We observed that the protein expression of TP53, CASP3 in normal tissues was significantly lower than that in tumor tissues (P<0.05).
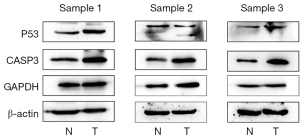
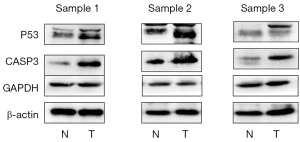
Discussion
In the current study, a total of 59 overlapping genes were identified among DM, T2DM, and GC. We then screened 401 genes/proteins associated with 59 overlapping genes, and the PPIs of the 401 genes/proteins associated with these 59 overlapping genes were constructed. We analyzed the entire PPI network using MCODE, and two significant modules, which had higher scores, were chosen. To obtain a more in-depth understanding of these genes, DAVID was used for both KEGG pathway and GO enrichment analyses of the selected PPI networks and module genes. Ten genes with the highest degree of connection were chosen as follows: IL6, MAPK3, EGFR, AKT1, TP53, GAPDH, STAT3, CASP3, VEGFA, and MYC. In order to confirm the results of the bioinformatics analysis, the Kaplan-Meier plotter database was used to predict the prognostic value of these hub genes, and analysis of their expression levels was carried out using GEPIA and UALCAN. Finally, we identified CASP3, and TP53 as core genes.
The tumor suppressor gene, TP53, encodes p53, a key transcription factor with a wide target gene pool. P53 is activated and stabilized during DNA damage and oncogene activation functions, leading to cell cycle arrest, apoptosis, or senescence. Altered TP53 is the most frequent mutation in GC, and is associated with unfavorable clinical outcomes. The findings of Jiang et al. (18) suggested that p53 may play an important role in activating tumor immunity in some tumor types, including GC. Mansouri et al. (19) identified that TP53 was a common remarkable gene between GC and chronic gastritis. In addition, disruption of p53 function is closely associated with several key BP leading to diabetes, including loss of pancreatic beta cells, interference with glucose homeostasis at the body level, and IR in peripheral tissues. Studies have shown that oxidative stress and elevated levels of free fatty acids lead to activation of p53, which leads to pancreatic beta cell death (20-25). Meanwhile, p53 can affect peripheral glucose metabolism by directly regulating glucose uptake, glycolysis and gluconeogenesis. P53-mediated changes in glucose homeostasis can lead to elevated circulating glucose levels (26). Feng et al. showed that p53 activity and insulin-PI3K-Akt signaling pathway are interwoven in various cell types, especially in peripheral tissues such as skeletal muscle, heart, WAT, liver and kidney, which respond to insulin to regulate glucose metabolism (27). In the other side, p53 can influence insulin sensitivity through the autocrine cycle. Therefore, overnutrition stimulates the pro-inflammatory activity of P53 in AT and weakens insulin sensitivity (28,29). Another study showed that increased p53 in white adipose tissue increases aging and chronic inflammation, which aggravates systemic IR (30). Sliwinska et al. (31) found that the serum level of TP53 in T2DM patients was significantly higher than that of non-diabetic healthy controls (1.69 vs. 2.07 ng/mL, P<0.001). The serum level of TP53 in T2DM patients was increased with age, diabetes course, and waist-to-hip ratio (WHR). Logistic regression analysis showed that elevated serum levels of TP53 were considerably related to family history of diabetes, age, and WHR (31).
CASP3 is the main mediator of apoptosis activation during cytotoxic drugs, radiotherapy, or immunotherapy. It is frequently used to assess the efficacy of cancer treatment. However, recent reports have shown that CASP3 also has non-apoptotic effects, such as promoting tumor recurrence and tumor angiogenesis. Ni et al.’s (32) current findings suggest that the polymorphism of CASP3 rs12108497 might be associated with the risk of GC. A study by Zhou et al. (33) also showed that CASP3 knockout of HCT116 cells were markedly less clonogenic in soft agar assays. Moreover, they were considerably less invasive and more sensitive to radiation and mitomycin C compared to control cells.
However, there are some limitations to our study that should be noted. Firstly, the expression and prognostic roles of the core genes requires further investigation. Secondly, the lack of functional experiments limits our mechanical analysis to be merely descriptive, and we still need to verify the role of hub genes in vitro and in vivo. Thirdly, due to the lack of relevant data sets, we were unable to verify the expression changes of core genes during anti-diabetic drug therapy.
In conclusion, in order to study whether hyperglycemia or type 2 diabetes is a risk factor affecting the occurrence and development of GC, and the potential molecular biological relationship between type 2 diabetes and GC, our study identified two genes that may play key roles in T2DM and GC: CASP3, and TP53 by bioinformatics. Our study will contribute to further understanding of the possible mechanism of diabetes progression to GC and provide useful information to identify a new biomarker for GC.
Acknowledgments
Funding: This work was funded by the Fund Program for the Scientific Activities of Selected Returned Overseas Professionals in Shanxi Province (No. 2017-1389); Bethune-Merck Diabetes Research Fund (No. G2017044); the Key Project of Research and Development Plan in Shanxi Province (No. 201803D31133); and the Research Project Supported by the Shanxi Scholarship Council of China (No. 2020-180).
Footnote
Reporting Checklist: The authors have completed the STREGA reporting checklist. Available at https://atm.amegroups.com/article/view/10.21037/atm-21-3635/rc
Data Sharing Statement: Available at https://atm.amegroups.com/article/view/10.21037/atm-21-3635/dss
Conflicts of Interest: All authors have completed the ICMJE uniform disclosure form (available at https://atm.amegroups.com/article/view/10.21037/atm-21-3635/coif). The authors have no conflicts of interest to declare.
Ethical Statement: The authors are accountable for all aspects of the work in ensuring that questions related to the accuracy or integrity of any part of the work are appropriately investigated and resolved. The study was conducted in accordance with the Declaration of Helsinki (as revised in 2013). The present study was approved by the Ethics Committee of Shanxi Bethune Hospital (No. YXLL-2021-063). Written informed consent was obtained from patients who agreed to participate in the study.
Open Access Statement: This is an Open Access article distributed in accordance with the Creative Commons Attribution-NonCommercial-NoDerivs 4.0 International License (CC BY-NC-ND 4.0), which permits the non-commercial replication and distribution of the article with the strict proviso that no changes or edits are made and the original work is properly cited (including links to both the formal publication through the relevant DOI and the license). See: https://creativecommons.org/licenses/by-nc-nd/4.0/.
References
- Bray F, Ferlay J, Soerjomataram I, et al. Global cancer statistics 2018: GLOBOCAN estimates of incidence and mortality worldwide for 36 cancers in 185 countries. CA Cancer J Clin 2018;68:394-424. [Crossref] [PubMed]
- Chen W, Zheng R, Baade PD, et al. Cancer statistics in China, 2015. CA Cancer J Clin 2016;66:115-32. [Crossref] [PubMed]
- Tseng CH, Tseng FH. Diabetes and gastric cancer: the potential links. World J Gastroenterol 2014;20:1701-11. [Crossref] [PubMed]
- Xu B, Zhou X, Li X, et al. Diabetes mellitus carries a risk of esophageal cancer: A meta-analysis. Medicine (Baltimore) 2017;96:e7944. [Crossref] [PubMed]
- Xu HL, Tan YT, Epplein M, et al. Population-based cohort studies of type 2 diabetes and stomach cancer risk in Chinese men and women. Cancer Sci 2015;106:294-8. [Crossref] [PubMed]
- Oronsky BT, Oronsky N, Fanger GR, et al. Follow the ATP: tumor energy production: a perspective. Anticancer Agents Med Chem 2014;14:1187-98. [Crossref] [PubMed]
- Wieser V, Moschen AR, Tilg H. Inflammation, cytokines and insulin resistance: a clinical perspective. Arch Immunol Ther Exp (Warsz) 2013;61:119-25. [Crossref] [PubMed]
- Ju X, Zhang H, Zhou Z, et al. Tumor-associated macrophages induce PD-L1 expression in gastric cancer cells through IL-6 and TNF-ɑ signaling. Exp Cell Res 2020;396:112315. [Crossref] [PubMed]
- Kasuga M, Ueki K, Tajima N, et al. Report of the Japan Diabetes Society/Japanese Cancer Association Joint Committee on Diabetes and Cancer. Cancer Sci 2013;104:965-76. [Crossref] [PubMed]
- Shimoyama S. Diabetes mellitus carries a risk of gastric cancer: a meta-analysis. World J Gastroenterol 2013;19:6902-10. [Crossref] [PubMed]
- Szklarczyk D, Franceschini A, Wyder S, et al. STRING v10: protein-protein interaction networks, integrated over the tree of life. Nucleic Acids Res 2015;43:D447-52. [Crossref] [PubMed]
- Li B, Pu K, Wu X. Identifying novel biomarkers in hepatocellular carcinoma by weighted gene co-expression network analysis. J Cell Biochem 2019; Epub ahead of print. [Crossref] [PubMed]
- Chin CH, Chen SH, Wu HH, et al. cytoHubba: identifying hub objects and sub-networks from complex interactome. BMC Syst Biol 2014;8:S11. [Crossref] [PubMed]
- Ding L, Fan L, Xu X, et al. Identification of core genes and pathways in type 2 diabetes mellitus by bioinformatics analysis. Mol Med Rep 2019;20:2597-608. [Crossref] [PubMed]
- Lánczky A, Nagy Á, Bottai G, et al. miRpower: a web-tool to validate survival-associated miRNAs utilizing expression data from 2178 breast cancer patients. Breast Cancer Res Treat 2016;160:439-46. [Crossref] [PubMed]
- Deng JL, Xu YH, Wang G. Identification of Potential Crucial Genes and Key Pathways in Breast Cancer Using Bioinformatic Analysis. Front Genet 2019;10:695. [Crossref] [PubMed]
- Chandrashekar DS, Bashel B, Balasubramanya SAH, et al. UALCAN: A Portal for Facilitating Tumor Subgroup Gene Expression and Survival Analyses. Neoplasia 2017;19:649-58. [Crossref] [PubMed]
- Jiang Z, Liu Z, Li M, et al. Immunogenomics Analysis Reveals that TP53 Mutations Inhibit Tumor Immunity in Gastric Cancer. Transl Oncol 2018;11:1171-87. [Crossref] [PubMed]
- Mansouri V, Rezaei Tavirani S, Zadeh-Esmaeel MM, et al. Comparative study of gastric cancer and chronic gastritis via network analysis. Gastroenterol Hepatol Bed Bench 2018;11:343-51. [PubMed]
- Zhang Y, Zeng SX, Hao Q, et al. Monitoring p53 by MDM2 and MDMX is required for endocrine pancreas development and function in a spatio-temporal manner. Dev Biol 2017;423:34-45. [Crossref] [PubMed]
- Tornovsky-Babeay S, Dadon D, Ziv O, et al. Type 2 diabetes and congenital hyperinsulinism cause DNA double-strand breaks and p53 activity in β cells. Cell Metab 2014;19:109-21. [Crossref] [PubMed]
- Hoshino A, Ariyoshi M, Okawa Y, et al. Inhibition of p53 preserves Parkin-mediated mitophagy and pancreatic β-cell function in diabetes. Proc Natl Acad Sci U S A 2014;111:3116-21. [Crossref] [PubMed]
- Kon N, Zhong J, Qiang L, et al. Inactivation of arf-bp1 induces p53 activation and diabetic phenotypes in mice. J Biol Chem 2012;287:5102-11. [Crossref] [PubMed]
- Hinault C, Kawamori D, Liew CW, et al. Δ40 Isoform of p53 controls β-cell proliferation and glucose homeostasis in mice. Diabetes 2011;60:1210-22. [Crossref] [PubMed]
- Yuan H, Zhang X, Huang X, et al. NADPH oxidase 2-derived reactive oxygen species mediate FFAs-induced dysfunction and apoptosis of β-cells via JNK, p38 MAPK and p53 pathways. PLoS One 2010;5:e15726. [Crossref] [PubMed]
- Lacroix M, Riscal R, Arena G, et al. Metabolic functions of the tumor suppressor p53: Implications in normal physiology, metabolic disorders, and cancer. Mol Metab 2020;33:2-22. [Crossref] [PubMed]
- Feng Z, Hu W, de Stanchina E, et al. The regulation of AMPK beta1, TSC2, and PTEN expression by p53: stress, cell and tissue specificity, and the role of these gene products in modulating the IGF-1-AKT-mTOR pathways. Cancer Res 2007;67:3043-53. [Crossref] [PubMed]
- Minamino T, Orimo M, Shimizu I, et al. A crucial role for adipose tissue p53 in the regulation of insulin resistance. Nat Med 2009;15:1082-7. [Crossref] [PubMed]
- Vergoni B, Cornejo PJ, Gilleron J, et al. DNA Damage and the Activation of the p53 Pathway Mediate Alterations in Metabolic and Secretory Functions of Adipocytes. Diabetes 2016;65:3062-74. [Crossref] [PubMed]
- Krstic J, Reinisch I, Schupp M, et al. p53 Functions in Adipose Tissue Metabolism and Homeostasis. Int J Mol Sci 2018;19:2622. [Crossref] [PubMed]
- Sliwinska A, Kasznicki J, Kosmalski M, et al. Tumour protein 53 is linked with type 2 diabetes mellitus. Indian J Med Res 2017;146:237-43. [Crossref] [PubMed]
- Ni Q, Jiang X, Jin MJ, et al. Association of CASP3 and CASP9 polymorphisms with genetic susceptibility to stomach cancer. Zhonghua Yi Xue Yi Chuan Xue Za Zhi 2011;28:318-22. [PubMed]
- Zhou M, Liu X, Li Z, et al. Caspase-3 regulates the migration, invasion and metastasis of colon cancer cells. Int J Cancer 2018;143:921-30. [Crossref] [PubMed]
(English Language Editor: A. Kassem)