An analysis of lncRNA-miRNA-mRNA networks to investigate the effects of HDAC4 inhibition on skeletal muscle atrophy caused by peripheral nerve injury
Introduction
Skeletal muscle is the largest tissue in the human body, and its structural integrity and functional maintenance are all under the control of the nervous system. The treatment of peripheral nerve injury caused by traumatic or chemical insult, still remains a challenge to be resolved. Following peripheral nerve injury, nerve regeneration occurs at a very slow rate. The target organ loses the trophic support of the nerve, triggering a series of reactions, such as oxidative stress, inflammation, mitochondrial autophagy, and catabolism. These series of reactions can cause skeletal muscle atrophy, which can be extended and aggravated over time (1,2). In severe cases, patients often experience irreversible atrophy, and atrophic muscle may be unable to be innervated by regenerated nerves, eventually resulting in a loss of function (3). Therefore, it is crucial to find the trigger factors that initiate skeletal muscle atrophy in the early stage of denervation and to intervene to delay the process of skeletal muscle atrophy after nerve injury.
We used a microarray to analyze the changes in gene expression levels of the tibialis anterior in rats at 15 minutes to 28 days after denervation. Our findings led us to propose the following 4 stages for the transcriptional regulation of denervation-induced skeletal muscle atrophy: oxidative stress stage (0.25–12 hours post-nerve injury), inflammation stage (24 hours post-nerve injury), atrophy stage (3–7 days post-nerve injury), and atrophic fibrosis stage (14–28 days post-nerve injury) (1). We further analyzed microarray data and screened out hub gene-histone deacetylase 4 (HDAC4) (1). HDAC4 is a class IIa histone deacetylase, belonging to an important epigenetic modifying enzyme family. HDAC4 affects the binding of transcription factors to DNA by transforming the status of histone acetylation and/or deacetylation. Studies have shown the expression of HDAC4 to be increased in a variety of muscle atrophy models, and HDAC4 can cause the deacetylation of MyHC subtypes, PGC-1α, and Hsc70 (4-6). In our recent work, we found that HDAC4 inhibition can inhibit proteolysis and mitochondrial autophagy caused by denervation and reversed denervation-induced tibialis anterior muscle atrophy (7).
Noncoding RNAs (ncRNAs), including microRNA (miRNAs) and long noncoding RNA (lncRNAs), have a regulatory role in skeletal muscle production and atrophy (8,9). miRNAs are a type of noncoding RNA with a length of 20–25 nucleotides, and they enact their biological activities by binding to the 3’-untranslated region (3’-UTR) of target gene messenger RNAs (mRNAs). Studies have shown that various miRNAs, such as miR-23a and miR-1, can bind to the E3 ubiquitin ligase MuRF1 and MAFbx of the ubiquitin protease system to inhibit their translation activation, regulate muscle atrophy thereby (10,11). Our previous studies also found that miR-125b-5p and miR-351 can alleviate muscle atrophy by targeting TRAF6 (12,13). lncRNAs are a type of regulatory RNAs with a length ranging from 200 bp to >100 kb, and they have an extremely abundant number of miRNA binding sites. According to competing endogenous RNA (ceRNA) theory, lncRNAs, acting as miRNA sponge by miRNA response elements (MREs), form a ceRNA network to modulate mRNA expression (14). For example, LINC-MD1 can regulate the expression of transcription factors, MAML1 and MEF2C, which activate late-differentiated muscle genes by binding to miR-133 and miR-135 (15). LNCIRS1 can regulate the expression of IRS1 via sponging the miR-15 family, thereby controlling skeletal muscle myogenesis and atrophy (16). Although a few lncRNAs have been functionally annotated, the role of most lncRNAs in myogenesis and atrophy is still unclear. Thus, the purpose of this study was to clarify the regulatory mechanism by which HDAC4 regulates skeletal muscle atrophy based on ceRNA theory.
In this study, we processed high-throughput sequencing data. First, we analyzed the miRNAs related to HDAC4 regulation of muscle atrophy, based on which we constructed the lncRNA-miRNA-mRNA network and performed functional enrichment analysis and annotations. We further explored the importance of lncRNAs in muscle atrophy. Finally, we found the core regulatory RNA molecules in the ceRNA network through analysis. Our findings provide important clues to exploring the mechanism by which HDAC4 regulates muscle atrophy and enriches the potential functions of lncRNA-based ceRNA networks in the process of HDAC4 regulating muscle atrophy. Studying the potential mechanism of HDAC4 in the process of muscle atrophy provides an important experimental basis for discovering new targets for the prevention and treatment of denervation-induced skeletal muscle atrophy. We present the following article in accordance with the ARRIVE (Animal Research: Reporting of In Vivo Experiments) reporting checklist (available at https://atm.amegroups.com/article/view/10.21037/atm-21-6512/rc).
Methods
Animal experiment
Adult male 8 to12-week-old Institute of Cancer Research (ICR) mice weighing 25±2 g, were provided by the Experimental Animal Center of Nantong University. All animals were caged at 22 ℃ in a 12-hour light-dark cycle environment and moved freely with free access to water and food. The ICR mice were randomly divided into 3 groups (n=3 per group). The left tibialis anterior of the mice was exposed under anesthesia, and lentivirus (25 µL, 1×108 TU) expressing HDAC4-shRNA (Hi + Den) was injected into the tibialis anterior muscles as previously described (7). An identical amount of empty vector virus was injected in the control group (Ctr) and denervation group (Den). The lentivirus vector for shRNA was GV298, and the component sequence was U6-MCS-Ubiquitin-Cherry-IRES-puromycin. After 3 days, the left sciatic nerve of the mice in the HDAC4 inhibition and denervation groups was cut, and the proximal end of the sciatic nerve was reflexed and sutured under the skin to produce a 10-mm defect model (17,18). In the control group, the sciatic nerve was only exposed but not dissected. Mice were euthanized under anesthesia on day 7, and the tibialis anterior was dissected and frozen on liquid nitrogen. Experiments were performed under a project license (No. 20180305-004) granted by the Jiangsu Laboratory Animal Management Committee, in compliance with Nantong University guidelines for the care and use of animals.
Transcriptome sequencing
Library construction and sequencing were implemented as previously described (19). In short, total RNA was extracted using the mirVana miRNA Isolation Kit (Ambion, Austin, TX, USA) according to the manufacturer’s protocol. Quantitation of total RNA was carried out using the Nanodrop 2000 (Thermo Fisher Scientific Inc., Waltham, MA, USA). RNA integrity was assessed using Agilent 2100 Bioanalyzer (Agilent Technology, Santa Clara, CA, USA). The total RNA (1 µg) of each sample was used for the small RNA library construction using TruSeq Small RNA Sample Prep Kits (Illumina, San Diego, CA, USA) following the manufacturer’s instructions, and 1 µg of total RNA of each sample was used for lncRNA library construction using TruSeq Stranded Total RNA with Ribo-Zero Gold (Illumina) according to the manufacturer’s instructions. Briefly, total RNA was ligated to adapters at each end. Then, the adapter-ligated RNA was reverse transcribed to circular DNA (cDNA), and polymerase chain reaction (PCR) amplification was performed. The PCR products ranging from 140–160 bp were isolated and purified as small RNA libraries. Library quality was assessed using the Agilent Bioanalyzer 2100 system (Agilent Technologies) using DNA High Sensitivity Chips. The libraries were finally sequenced using the Illumina HiSeq X Ten platform, and 150-bp paired-end reads were generated. The miRNA and lncRNA expression profiles are available on ArrayExpress (https://ftp.ebi.ac.uk/pub/databases/microarray/data), and the accession numbers. Are E-MTAB-11362 and E-MTAB-11361, respectively.
Expression analysis
The original data generated by sequencing were filtered out to obtain high-quality clean reads. Clean data were obtained by removing reads containing adapter and ploy-N or low-quality reads from raw data. Candidate lncRNA transcripts were screened out using Stringtie2 software (1.3.3b version, John Hopkins University, Baltimore, MD, USA) and Cuffcompare software (2.2.1 version, The University of Maryland, Baltimore, MD, USA), and transcripts with coding potential were then removed to obtain the predicted sequence of lncRNA. The sequencing reads of each sample was aligned to the combined sequence of mRNA transcript sequence, known lncRNA sequence, and lncRNA-predicted sequence using bowtie2 (2.2.9 version, John Hopkins University) (20), and eXpress software (version 1.5.1, Express Software Production, East Granby, CT, United States) was used for gene quantification to obtain the FPKM (fragments per kilobase per million) value and counts. The counts were standardized through the estimateSizeFactors function of the DESeq R package (1.18.0 version, Fred Hutchinson Cancer Research Center, Seattle, WA, USA), and the P and fold change values for difference comparison were calculated using the nbinomTest function. lncRNAs (fold change <1.5 or >1.5 and P<0.05) were sorted out.
The original reads obtained by small RNA sequencing were first removed of adaptor sequences and filtered using Cutadapt (1.14 version, National Bioinformatics Infrastructure, Uppsala, Sweden). High-quality clean reads were then obtained following filtration using fastx_toolkit (0.0.13 version, Cancer Research UK Cambridge Institute, Cambridge, UK) and National Institute of Plant Genome Research Toolkit (2.3.2 version, National Institute of Plant Genome Research, New Delhi, India), which were used for subsequent analysis. Filtered data were compared using Blastn (2.2.26 version, National Center for Biotechnology, Bethesda, MD, USA) and RepeatMasker (4.0.9 version, http://www.repeatmasker.org)) software. A new miRNA prediction using Mirdeep2 (2.0.0.8 version, Max Delbrueck Center for Molecular Medicine, Berlin, Germany) was conducted in the unannotated small RNA sequences, and the secondary structure of the newly predicted miRNA was predicted using RNAfold (2.4.9 version, University of Vienna, Vienna, Austria). Based on the known mature miRNA and newly predicted miRNA, miRNA expression was statistically quantified as transcripts per million (TPM). TPM is equal to the number of reads mapped to each miRNA/the total number of mapped reads ×106. TPM indicates that counts come from a transcript per million reads mapped, and the total number of paired-end reads mapped is used to normalize the expression level. The Audic_Claverie formula was used to calculate P value, and the miRNAs with fold change <–1.5 or >1.5 and P<0.05 were selected. The mRNA expression profile was analyzed based on our published RNA sequence data, which is available on ArrayExpress (accession no. E-MTAB-11361) (7). Differentially expressed mRNAs were defined as mRNAs with P<0.05 and a fold change <–1.5 or >1.5.
CeRNA network analysis
Based on the expression of different mRNAs, miRNAs, and lncRNAs, Pearson’s correlation coefficients and P values were calculated for miRNA-target pairs. Pearson correlation coefficients ≥0.8 or ≤–0.8 with P<0.05 were considered statistically significant. Among these negatively correlated miRNA-lncRNAs, candidate mRNAs and lncRNAs regulated by the miRNAs were predicted using miRanda v3.3a (http://www.microrna.org/microrna/). The threshold parameter was set as described previously: S ≥150, ΔG ≤−30 kcal/mol and strict 5’ seed pairing (21). Subsequently, shared pairs of miRNA-mRNA and miRNA-lncRNA were used to predict ceRNA scores using MuTaME (22) according to the following formula: ceRNA_score = MRE_for_share_miRNA/MRE_for_lncRNA_miRNA; the related P values were calculated using Benjamini and Hochberg’s approach for controlling the false discovery rate (23). The positively correlated pairs of lncRNA-mRNA based on the ceRNA score principle were then considered as the true ceRNAs.
Construction of lncRNA-associated ceRNA networks
Based on the above sequencing data analysis, Cytoscape v. 3.8.2 software (Institute for Systems Biology, Seattle, WA, USA) was used to construct a lncRNA-related ceRNA network. The subnetwork containing the hub gene was identified using a Cytoscape plugin, MCODE.
Functional annotation
The Kyoto Encyclopedia of Genes and Genomes (KEGG) pathway was used to analyze the significance of the target genes of differentially expressed miRNA with the mirPath v.3 online tool of DIANA TOOLS (http://snf-515788.vm.okeanos.grnet.gr/index.php?r=mirpath). Gene Ontology (GO) and KEGG pathway analyses were completed online for mRNAs in the ceRNA network (https://david.ncifcrf.gov/summary.jsp). KEGG or GO terms with P<0.05 were considered to be significantly enriched.
Statistical analysis
When RNA-seq data are used to compare and analyze whether there is differential expression of the same gene in 2 samples, 2 criteria can be selected: one is fold change, which is the change multiple of the same gene expression level in two samples; the other is the P value. The default screening condition was P<0.05, and the difference multiple was more than 1.5.
Results
Effect of HDAC4 inhibition on miRNA expression changes of skeletal muscle atrophy caused by peripheral nerve injury
We performed a miRNA-seq analysis to determine the effect of HDAC4 inhibition on miRNAs in mice with denervation-induced skeletal muscle atrophy. There were a total of 1,148 annotated miRNAs and 841 newly predicted miRNAs in all the samples. Hierarchical clustering showed that miRNAs were well distinguished in the control group (Ctr), denervation group (Den), and HDAC4 inhibition group (Hi + Den), and all samples were correctly classified (Figure 1A). Principal component analysis (PCA) results indicated there were different expression characteristics of miRNAs in samples from different groups (Figure 1B). Compared with the Ctr group, there were 155 downregulated miRNAs and 97 upregulated miRNAs in the Den group; compared with the Den group, there were 123 upregulated miRNAs and 73 downregulated miRNAs in the Hi + Den group (Figure 1C). The intersection of downregulated miRNAs after denervation and upregulated miRNAs after HDAC4 inhibition was identified, and these intersected miRNAs were then referred to as upregulated miRNAs, including mmu-miR-669l-5p, mmu-miR-3095-3p, mmu-miR-449a-5p, mmu-miR-146a-5p, mmu-miR-592-5p, mmu-miR-298-5p, mmu-miR-376b-3p, mmu-miR-296-3p, mmu-miR-362-3p, mmu-miR-296-5p, mmu-miR-362-5p, mmu-miR-181c-3p, mmu-miR-500-3p, mmu-miR-501-3p, mmu-miR-181a-1-3p, and mmu-miR-335-3p (Table 1). The intersection of upregulated miRNAs after denervation and downregulated miRNAs after HDAC4 inhibition was identified, and these intersected miRNAs were then referred to as downregulated miRNAs, including novel159_mature, mmu-miR-9-5p, mmu-miR-9-3p, novel489_mature, novel630_mature, novel6_mature, mmu-miR-128-3p, mmu-miR-671-3p, novel162_ mature>novel498_mature, mmu-miR-27a-5p, novel374_mature>novel461_mature, mmu-miR-30a-5p, mmu-miR-30c-2-3p, mmu-miR-5099, mmu-miR-149-5p, and novel356_mature (Table 1). KEGG analysis of target genes was conducted using the mirPath online tool to determine their related signal pathways. KEGG analysis results showed that the upregulated miRNAs were mainly involved in the regulation of pathways in cancer, thyroid hormone signaling pathway, adherens junction, FoxO signaling pathway, ubiquitin-mediated proteolysis, Wnt signaling pathway, and protein processing in endoplasmic reticulum pathways (Figure 1D), while the downregulated miRNAs were mainly involved in the regulation of fatty acid biosynthesis, protein processing in endoplasmic reticulum, axon guidance, glycosaminoglycan biosynthesis-chondroitin sulfate/dermatan sulfate, and neurotrophin signaling pathway processes (Figure 1E).
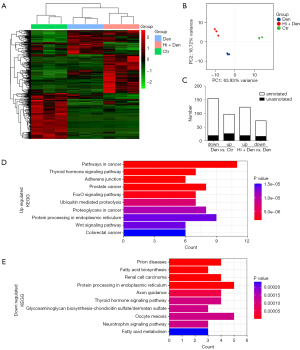
Table 1
miRNA_id | Den vs. Ctr | Hi + Den vs. Den | Hi + Den vs. Ctr | |||||
---|---|---|---|---|---|---|---|---|
log2(FC) | P val | log2(FC) | P val | log2(FC) | P val | |||
Upregulated | ||||||||
mmu-miR-669l-5p | −2.32 | 0.0474 | 3.68 | <0.0001 | 1.40 | 0.0315 | ||
mmu-miR-3095-3p | −2.71 | 0.0012 | 2.89 | 0.0006 | 0.22 | 0.8114 | ||
mmu-miR-449a-5p | −1.11 | 0.0467 | 2.89 | 0.0023 | 1.83 | 0.0220 | ||
mmu-miR-146a-5p | −0.69 | <0.0001 | 2.84 | <0.0001 | 2.19 | <0.0001 | ||
mmu-miR-592-5p | −0.80 | 0.0108 | 2.27 | <0.0001 | 1.51 | <0.0001 | ||
mmu-miR-298-5p | −3.14 | <0.0001 | 2.04 | <0.0001 | −1.07 | 0.0002 | ||
mmu-miR-376b-3p | −3.16 | <0.0001 | 1.81 | 0.0019 | −1.31 | 0.0016 | ||
mmu-miR-296-3p | −2.56 | <0.0001 | 1.59 | 0.0030 | −0.94 | 0.0083 | ||
mmu-miR-362-3p | −0.72 | 0.0009 | 1.38 | <0.0001 | 0.70 | 0.0041 | ||
mmu-miR-296-5p | −2.50 | <0.0001 | 1.16 | 0.0002 | −1.30 | 0.0002 | ||
mmu-miR-362-5p | −1.01 | <0.0001 | 1.07 | <0.0001 | 0.10 | 0.6494 | ||
mmu-miR-181c-3p | −1.76 | <0.0001 | 1.01 | 0.0020 | −0.72 | 0.0162 | ||
mmu-miR-500-3p | −0.89 | 0.0003 | 0.97 | 0.0002 | 0.11 | 0.7105 | ||
mmu-miR-501-3p | −0.85 | <0.0001 | 0.85 | <0.0001 | 0.04 | 0.8429 | ||
mmu-miR-181a-1-3p | −0.88 | 0.0001 | 0.78 | 0.0016 | −0.07 | 0.7900 | ||
mmu-miR-335-3p | −2.48 | <0.0001 | 0.77 | 0.0028 | −1.67 | <0.0001 | ||
Downregulated | ||||||||
novel159_mature | 2.12 | 0.0284 | −5.21 | 0.0007 | −3.07 | 0.1407 | ||
mmu-miR-9-5p | 2.89 | <0.0001 | −3.05 | <0.0001 | −0.13 | 0.5589 | ||
mmu-miR-9-3p | 1.72 | 0.0143 | −2.67 | 0.0010 | −0.91 | 0.0638 | ||
novel489_mature | 1.38 | 0.0438 | −1.33 | 0.0328 | 0.07 | 1.0000 | ||
novel630_mature | 1.03 | 0.0121 | −1.33 | 0.0019 | −0.26 | 0.6446 | ||
novel6_mature | 1.64 | <0.0001 | −1.19 | <0.0001 | 0.49 | 0.1288 | ||
mmu-miR-128-3p | 0.64 | 0.0003 | −1.10 | <0.0001 | −0.41 | 0.0237 | ||
mmu-miR-671-3p | 1.19 | <0.0001 | −1.06 | 0.0001 | 0.17 | 0.5866 | ||
novel162_mature>novel498_mature | 2.63 | <0.0001 | −1.01 | 0.0202 | 1.67 | 0.0086 | ||
mmu-miR-27a-5p | 1.75 | <0.0001 | −0.88 | 0.0004 | 0.92 | 0.0019 | ||
novel374_mature>novel461_mature | 0.93 | 0.0218 | −0.85 | 0.0402 | 0.11 | 0.8516 | ||
mmu-miR-30a-5p | 0.92 | <0.0001 | −0.83 | 0.0001 | 0.12 | 0.6070 | ||
mmu-miR-30c-2-3p | 0.63 | 0.0051 | −0.80 | <0.0001 | −0.13 | 0.4555 | ||
mmu-miR-5099 | 1.55 | <0.0001 | −0.70 | 0.0035 | 0.89 | 0.0014 | ||
mmu-miR-149-5p | 0.80 | <0.0001 | −0.69 | 0.0003 | 0.14 | 0.4986 | ||
novel356_mature | 0.63 | 0.0484 | −0.60 | 0.0360 | 0.07 | 0.8608 |
FC, fold change; Ctr, control group; Den, denervation group; Hi + Den, HDAC4 inhibition group; miRNA, microRNA.
Effect of HDAC4 inhibition on lncRNA expression changes of denervation-induced skeletal muscle atrophy
Subsequently, we conducted a lncRNA-Seq analysis, and there were 34,458 lncRNAs detected in total, from which 678 newly predicted lncRNAs were identified. Hierarchical clustering analysis showed that the lncRNAs were well distinguished in the Ctr, Den, and Hi + Den groups, and all samples were correctly classified (Figure 2A). The PCA results showed the different expression characteristics of lncRNAs in samples from different groups (Figure 2B). Compared with the Ctr group, there were 377 downregulated lncRNAs and 454 upregulated lncRNAs in the Den group. Compared with the denervation group, there were 304 upregulated lncRNAs and 178 downregulated lncRNAs in Hi + Den group (Figure 2C). Among the differentially expressed lncRNAs, 20% were located in the exons of protein-coding genes, 23% were located in the introns, and 26% and 18% were located in the upstream and downstream of the intragenic region, respectively (Figure 2D). Furthermore, when the chromosome distribution and sequence length distribution of the lncRNAs were analyzed, there were more lncRNAs located on chromosome 1 (Figure 2E,2F).
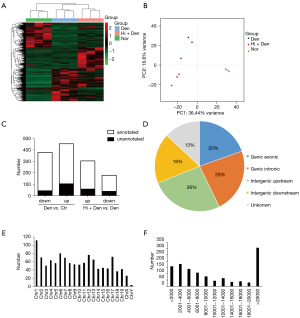
DE lncRNAs, miRNAs, and mRNAs formed gene-gene networks by mutual interaction
The upregulated lncRNAs were defined as the intersection of downregulated lncRNAs after denervation and upregulated lncRNA after HDAC4 inhibition. The downregulated lncRNAs were defined as the intersection of upregulated lncRNAs after denervation and downregulated lncRNA after HDAC4 inhibition. The final sample included 27 upregulated lncRNAs and 84 downregulated lncRNAs (Table 2). To better understand the regulatory role of lncRNA and miRNA in HDAC4 regulation of muscle atrophy, we constructed a lncRNA-miRNA-mRNA ceRNA network to further clarify the interaction of specific differential lncRNAs, miRNAs, and mRNAs. A ceRNA regulatory network was composed of 15 downregulated lncRNAs, 14 upregulated miRNAs, and 61 downregulated mRNAs. In this network, E3 ubiquitin ligase genes, Fbxo32 and Trim63, were closely related to muscle atrophy (Figure 3A). In addition, 6 upregulated lncRNAs, 8 downregulated miRNAs, and 66 upregulated mRNAs constituted another ceRNA regulatory network (Figure 3B).
Table 2
lncRNA_id | Den vs. Ctr | Hi + Den vs. Den | Hi + Den vs. Ctr | |||||
---|---|---|---|---|---|---|---|---|
log2(FC) | P val | log2(FC) | P val | log2(FC) | P val | |||
Downregulated | ||||||||
ENSMUST00000230832 | 5.29 | 0.0120 | −Inf | 0.0021 | −Inf | 0.9669 | ||
TCONS_00009025 | 3.64 | 0.0292 | −Inf | 0.0003 | −Inf | 0.6232 | ||
TCONS_00026499 | Inf | 0.0094 | −Inf | 0.0057 | 0.00 | 1.0000 | ||
XR_001784025.1 | Inf | 0.0211 | −Inf | 0.0062 | 0.00 | 1.0000 | ||
XR_378108.2 | Inf | 0.0307 | −Inf | 0.0188 | 0.00 | 1.0000 | ||
XR_382507.3 | Inf | 0.0268 | −Inf | 0.0149 | 0.00 | 1.0000 | ||
XR_390555.3 | 4.50 | 0.0176 | −Inf | 0.0019 | −Inf | 0.7767 | ||
XR_868997.2 | 3.85 | 0.0404 | −Inf | 0.0009 | −Inf | 0.7840 | ||
NR_003364.1 | Inf | 0.0056 | −6.49 | 0.0071 | Inf | 1.0000 | ||
XR_001779733.1 | Inf | 0.0003 | −6.37 | <0.0001 | Inf | 1.0000 | ||
ENSMUST00000174412 | Inf | 0.0065 | −5.51 | 0.0027 | Inf | 1.0000 | ||
XR_001779736.1 | 3.55 | 0.0086 | −5.47 | 0.0001 | −1.89 | 0.6545 | ||
ENSMUST00000181532 | 1.87 | 0.0151 | −5.41 | <0.0001 | −3.55 | 0.0283 | ||
XR_878681.2 | Inf | 0.0141 | −5.17 | 0.0112 | Inf | 1.0000 | ||
NR_037998.1 | Inf | 0.0344 | −4.83 | 0.0201 | Inf | 1.0000 | ||
XR_382352.3 | 2.63 | 0.0373 | −4.81 | 0.0002 | −2.21 | 0.4942 | ||
NR_045458.1 | Inf | 0.0175 | −4.62 | 0.0382 | Inf | 0.9373 | ||
XR_388161.1 | 3.72 | 0.0464 | −4.48 | 0.0087 | −0.77 | 1.0000 | ||
ENSMUST00000131907 | 6.92 | 0.0001 | −4.38 | 0.0010 | 2.55 | 0.5204 | ||
XR_001783822.1 | Inf | 0.0156 | −3.82 | 0.0254 | Inf | 0.8663 | ||
XR_873120.2 | Inf | 0.0064 | −3.81 | 0.0343 | Inf | 0.7787 | ||
ENSMUST00000221672 | 3.83 | 0.0209 | −3.63 | 0.0179 | 0.20 | 1.0000 | ||
ENSMUST00000232754 | Inf | 0.0042 | −3.62 | 0.0109 | Inf | 0.7343 | ||
ENSMUST00000163081 | Inf | 0.0057 | −3.58 | 0.0370 | Inf | 0.6962 | ||
ENSMUST00000234022 | Inf | <0.0001 | −3.52 | <0.0001 | Inf | 0.0356 | ||
XR_879834.1 | 4.71 | 0.0046 | −3.16 | 0.0233 | 1.54 | 0.6600 | ||
XR_001780251.1 | Inf | <0.0001 | −3.12 | 0.0088 | Inf | 0.0791 | ||
XR_390581.3 | 3.99 | 0.0096 | −2.96 | 0.0138 | 1.04 | 0.7675 | ||
TCONS_00007295 | 2.35 | 0.0036 | −2.92 | 0.0001 | −0.57 | 0.7765 | ||
ENSMUST00000183079 | 5.47 | 0.0036 | −2.91 | 0.0464 | 2.54 | 0.5123 | ||
ENSMUST00000220830 | 5.04 | 0.0009 | −2.80 | 0.0083 | 2.23 | 0.4355 | ||
XR_876739.2 | 4.94 | 0.0012 | −2.79 | 0.0226 | 2.16 | 0.1841 | ||
ENSMUST00000130362 | Inf | 0.0032 | −2.76 | 0.0331 | Inf | 0.4430 | ||
ENSMUST00000235440 | 2.18 | 0.0350 | −2.73 | 0.0029 | −0.54 | 0.6818 | ||
ENSMUST00000143649 | 4.44 | <0.0001 | −2.72 | 0.0004 | 1.71 | 0.1244 | ||
XR_377582.2 | 5.87 | <0.0001 | −2.70 | 0.0007 | 3.16 | 0.0173 | ||
XR_875669.2 | Inf | 0.0006 | −2.59 | 0.0205 | Inf | 0.3736 | ||
ENSMUST00000233201 | 1.69 | 0.0380 | −2.59 | 0.0017 | −0.90 | 0.3069 | ||
XR_869865.2 | 3.88 | 0.0014 | −2.52 | 0.0088 | 1.37 | 0.4492 | ||
XR_382351.2 | 3.60 | 0.0055 | −2.51 | 0.0234 | 1.08 | 0.5368 | ||
XR_877494.2 | 2.03 | 0.0113 | −2.44 | 0.0010 | −0.41 | 0.6499 | ||
XR_877458.1 | 4.85 | 0.0005 | −2.44 | 0.0280 | 2.43 | 0.1769 | ||
XR_377746.2 | Inf | 0.0006 | −2.42 | 0.0248 | Inf | 0.2223 | ||
ENSMUST00000197878 | Inf | <0.0001 | −2.36 | 0.0033 | Inf | 0.0020 | ||
ENSMUST00000187019 | 3.28 | 0.0001 | −2.35 | 0.0010 | 0.94 | 0.2181 | ||
NR_040268.1 | 3.26 | 0.0085 | −2.34 | 0.0156 | 0.91 | 0.7053 | ||
NR_040290.1 | 2.89 | 0.0021 | −2.24 | 0.0062 | 0.65 | 0.5297 | ||
XR_880009.2 | 7.76 | <0.0001 | −2.23 | 0.0048 | 5.54 | 0.0035 | ||
NR_045403.1 | 4.08 | 0.0011 | −2.21 | 0.0132 | 1.87 | 0.3256 | ||
TCONS_00005955 | 6.10 | <0.0001 | −2.17 | 0.0084 | 3.95 | 0.0025 | ||
ENSMUST00000213985 | Inf | <0.0001 | −2.05 | 0.0243 | Inf | 0.0000 | ||
XR_384799.3 | 6.13 | 0.0002 | −2.03 | 0.0357 | 4.09 | 0.0816 | ||
TCONS_00017176 | 3.44 | <0.0001 | −2.01 | 0.0035 | 1.44 | 0.1079 | ||
XR_881514.1 | 6.56 | <0.0001 | −1.91 | 0.0424 | 4.63 | 0.1937 | ||
NR_015595.3 | 9.71 | <0.0001 | −1.89 | 0.0062 | 7.82 | <0.0001 | ||
ENSMUST00000221315 | Inf | <0.0001 | −1.87 | 0.0303 | Inf | 0.0015 | ||
XR_001785029.1 | 3.46 | <0.0001 | −1.82 | 0.0057 | 1.64 | 0.0124 | ||
XR_869015.1 | 4.75 | <0.0001 | −1.8 | 0.0151 | 2.95 | 0.0039 | ||
ENSMUST00000181427 | 1.62 | 0.0394 | −1.8 | 0.0112 | −0.18 | 0.8020 | ||
NR_045843.1 | 2.86 | 0.0005 | −1.77 | 0.0127 | 1.09 | 0.1423 | ||
ENSMUST00000133752 | 4.62 | <0.0001 | −1.76 | 0.0301 | 2.86 | 0.0240 | ||
XR_875986.1 | 2.45 | 0.0034 | −1.76 | 0.0156 | 0.70 | 0.4005 | ||
TCONS_00011293 | 1.73 | 0.0267 | −1.75 | 0.0145 | −0.02 | 0.9960 | ||
ENSMUST00000226943 | 2.96 | 0.0005 | −1.73 | 0.0197 | 1.23 | 0.1295 | ||
TCONS_00013504 | Inf | <0.0001 | −1.71 | 0.0333 | Inf | 0.0008 | ||
NR_027896.1 | 2.21 | 0.0039 | −1.71 | 0.0126 | 0.50 | 0.4558 | ||
TCONS_00012110 | 3.39 | 0.0002 | −1.71 | 0.0200 | 1.69 | 0.0611 | ||
ENSMUST00000185272 | 1.94 | 0.0092 | −1.67 | 0.0124 | 0.28 | 0.6826 | ||
ENSMUST00000133548 | 8.84 | <0.0001 | −1.65 | 0.0174 | 7.20 | 0.0000 | ||
ENSMUST00000132294 | 1.78 | 0.0326 | −1.61 | 0.0343 | 0.16 | 0.8344 | ||
TCONS_00024432 | 4.61 | <0.0001 | −1.59 | 0.0291 | 3.02 | 0.0010 | ||
ENSMUST00000149601 | 2.51 | 0.0017 | −1.56 | 0.0269 | 0.95 | 0.1993 | ||
NR_045495.1 | 3.07 | 0.0002 | −1.55 | 0.0252 | 1.52 | 0.0452 | ||
ENSMUST00000236871 | 2.54 | 0.0010 | −1.54 | 0.0226 | 1.00 | 0.1360 | ||
XR_874798.2 | 2.44 | 0.0041 | −1.54 | 0.0318 | 0.91 | 0.4564 | ||
ENSMUST00000142076 | 8.21 | <0.0001 | −1.53 | 0.0401 | 6.67 | <0.0001 | ||
TCONS_00027579 | 2.00 | 0.0143 | −1.53 | 0.0333 | 0.48 | 0.5453 | ||
TCONS_00027611 | 2.00 | 0.0143 | −1.53 | 0.0333 | 0.48 | 0.5453 | ||
ENSMUST00000223732 | 8.00 | <0.0001 | −1.52 | 0.0260 | 6.49 | <0.0001 | ||
TCONS_00024358 | Inf | <0.0001 | −1.49 | 0.0456 | Inf | <0.0001 | ||
TCONS_00019824 | 2.06 | 0.0057 | −1.48 | 0.0249 | 0.58 | 0.3726 | ||
ENSMUST00000123048 | 1.83 | 0.0146 | −1.48 | 0.0275 | 0.35 | 0.5991 | ||
NR_045904.1 | 1.85 | 0.0136 | −1.32 | 0.0486 | 0.53 | 0.4232 | ||
ENSMUST00000185587 | 1.55 | 0.0321 | −1.32 | 0.0445 | 0.24 | 0.7013 | ||
Upregulated | ||||||||
ENSMUST00000183180 | −Inf | <0.0001 | Inf | 0.0074 | −2.05 | 0.0341 | ||
ENSMUST00000201112 | −Inf | <0.0001 | Inf | 0.0074 | −2.05 | 0.0341 | ||
NR_045337.1 | −Inf | 0.0143 | Inf | 0.0474 | −0.80 | 0.5951 | ||
XR_001778161.1 | −Inf | 0.0164 | Inf | 0.0275 | −0.56 | 0.7589 | ||
XR_865706.1 | −Inf | 0.0051 | Inf | 0.0014 | −0.15 | 0.8675 | ||
XR_871930.1 | −Inf | 0.0059 | Inf | 0.0001 | 0.69 | 0.4889 | ||
XR_875613.1 | −Inf | 0.0042 | Inf | 0.0268 | −1.51 | 0.2845 | ||
XR_879260.1 | −Inf | 0.0176 | Inf | 0.0083 | −0.04 | 0.9955 | ||
ENSMUST00000191079 | −6.46 | 0.0146 | 5.27 | 0.0063 | −1.22 | 0.4296 | ||
ENSMUST00000192778 | −6.87 | <0.0001 | 5.01 | 0.0074 | −1.86 | 0.0398 | ||
XR_001782817.1 | −5.24 | 0.0245 | 4.97 | 0.0049 | −0.30 | 0.8816 | ||
TCONS_00019186 | −5.79 | <0.0001 | 4.63 | 0.0121 | −1.13 | 0.1500 | ||
ENSMUST00000143847 | −5.18 | 0.0007 | 4.59 | 0.0023 | −0.57 | 0.5237 | ||
NR_046046.1 | −2.96 | 0.0489 | 4.44 | <0.0001 | 1.49 | 0.0955 | ||
XR_385889.3 | −7.19 | <0.0001 | 4.19 | 0.0261 | −2.98 | 0.0004 | ||
NR_045820.1 | −3.74 | 0.0242 | 4.17 | 0.0021 | 0.44 | 0.6922 | ||
NR_045821.1 | −4.31 | 0.0394 | 4.14 | 0.0130 | −0.15 | 0.9607 | ||
ENSMUST00000067599 | −4.02 | 0.0179 | 3.74 | 0.0111 | −0.26 | 0.8602 | ||
ENSMUST00000183245 | −2.55 | 0.0273 | 3.59 | 0.0001 | 1.05 | 0.1781 | ||
ENSMUST00000116345 | −3.49 | 0.0137 | 3.58 | 0.0371 | 0.07 | 0.9451 | ||
NR_040659.1 | −4.83 | 0.0042 | 3.3 | 0.0090 | −1.50 | 0.1730 | ||
NR_131197.1 | −3.44 | 0.0315 | 3.27 | 0.0207 | −0.17 | 0.9143 | ||
ENSMUST00000177248 | −3.04 | 0.0481 | 2.98 | 0.0288 | −0.09 | 0.9804 | ||
XR_877227.1 | −2.73 | 0.0182 | 2.94 | 0.0326 | 0.19 | 0.7546 | ||
ENSMUST00000135378 | −3.92 | 0.0005 | 2.78 | 0.0144 | −1.15 | 0.1898 | ||
XR_877582.2 | −2.27 | 0.0180 | 2.39 | 0.0054 | −1.15 | 0.1898 | ||
NR_028422.1 | −7.14 | 0.0002 | 2.08 | 0.0457 | −5.09 | 0.0035 |
FC, fold change; Ctr, control group; Den, denervation group; Hi + Den, HDAC4 inhibition group; lncRNA, long noncoding RNA.
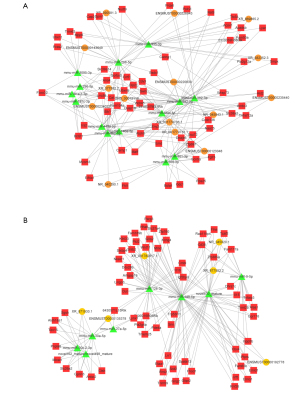
GO and KEGG pathway analyses
GO analysis and KEGG pathway analysis showed that after inhibition with HDAC4, in the ceRNA network that downregulated lncRNAs belonged to, the mRNAs participated in the biological processes, such as response to denervation involved in regulation of muscle adaptation, regulation of fatty acid beta-oxidation, and positive regulation of glucose metabolic process, These mRNAs were also found to be involved in several pathways, including autophagy, Ras signaling pathway, FoxO signaling pathway, and Jak-STAT signaling pathway (Figure 4). After HDAC4 inhibition in the ceRNA network that the upregulated lncRNAs belonged to, the mRNAs were found to participate in certain biological processes, including cell cycle and cell division, and in the pathways related to cell cycle (Figure 5).
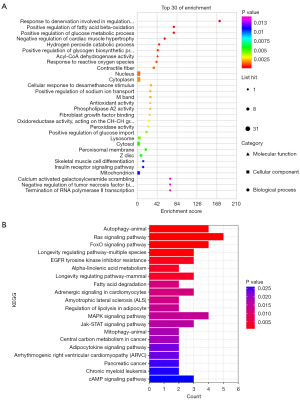
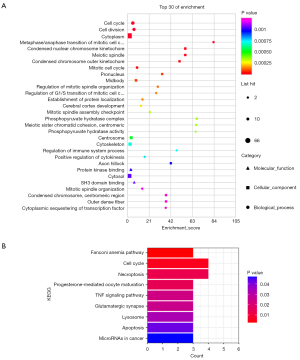
Key genes in the ceRNA sub-network
In the ceRNA network, hub molecules were identified using MCODE. A total of 19 nodes were selected as hub nodes, including mmu-miR-181c-3p, mmu-miR-181a-1-3p, mmu-miR-3095-3p, mmu-miR-296-5p, Lrrc58, Trim63, Slc39a14, Rras2, Ubxn1, Cat, Sorbs3, Fbxo32, Fam104a, Irs2, Bcl2l1, Mpp6, ENSMUST00000234022, ENSMUST00000143649, and XR_377582.2 (Figure 6). Among them, the number of miRNA-mRNA pairs in miR-3095-3p was the highest, and miR-3095-3p was identified as the core-regulated miRNA. XR_377582.2 and ENSMUST00000143649 bound to the MRE of miR-3095-3p to regulate mRNAs such as Trim63 and Fbxo32, indicating that these 2 lncRNAs might play a key role in the HDAC4 regulation of denervation-induced skeletal muscle atrophy.
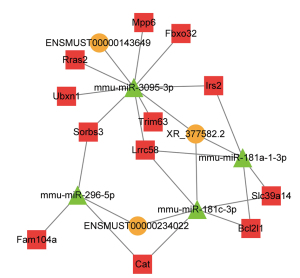
Discussion
In addition to denervation caused by peripheral nerve injury, aging, paralysis, malnutrition, a variety of diseases including cancer, cardiovascular disease, and neurodegenerative diseases can all cause skeletal muscle atrophy (24-27). Reduced protein synthesis and increased protein degradation are the main causes of skeletal muscle atrophy (28). The expression level of HDAC4 has been confirmed to be closely related to various types of muscle atrophy (4,7,29). In previous studies, we discovered and confirmed using mRNA-seq that HDAC4 regulates muscle atrophy by regulating Myog levels and affecting proteolysis (7). In this study, we first performed miRNA-seq, identified 16 upregulated and 16 downregulated miRNAs related to HDAC4-regulated denervation through the comparative analysis, and conducted the KEGG pathway analysis of target genes. Through the comparative analysis of lncRNA-seq, we then identified 111 lncRNAs (27 upregulated and 84 downregulated) related to denervation regulated by HDAC4. Then, we constructed lncRNA-miRNA-mRNA networks related to HDAC4 regulation of muscle atrophy, and conducted GO function and KEGG pathway enrichment analysis. Finally, we searched for the core regulatory molecules in the ceRNA network in the above lncRNA-miRNA-mRNA network.
Through specific binding to target mRNA, miRNA can accelerate the degradation of target mRNA or inhibit its translation regulatory gene expression. A variety of miRNAs have been reported as being involved in the regulation of skeletal muscle development, differentiation, regeneration, and dysfunction (12,30,31). In this study, we first analyzed the differentially expressed miRNAs related to HDAC4 regulation of muscle atrophy. Muscle-specific miRNAs include miR-1/206 family, miR-133, miR-208, and miR-488. Unfortunately, these muscle-specific miRNAs are not the miRNAs we analyzed here. Nevertheless, KEGG pathway analysis indicated that the target genes of upregulated miRNAs after HDAC4 inhibition were mainly involved in the FoxO signaling pathway and ubiquitin-mediated proteolysis, indicating that miRNAs regulated by HDAC4 are involved in hydrolysis related to muscle atrophy. Target genes of downregulated miRNAs were mainly involved in axon guidance, indicating that miRNAs regulated by HDAC4 are involved in nerve regeneration.
MRE-containing lncRNAs can act as molecular sponges to effectively inhibit miRNA functions. To date, ceRNA mechanisms and network construction have been mainly investigated in the field of cancer research, and only a few ceRNA interactions have been found to be related to muscle atrophy. Recent research has begun to systematically explore the ceRNA regulatory mechanism for specific muscle atrophies (8,16,25). In this study, we analyzed the differentially expressed lncRNAs related to muscle atrophy regulated by HDAC4. After inhibition of HDAC4, there were 27 upregulated lncRNAs and 84 downregulated lncRNAs. In the ceRNA regulatory network composed of 6 upregulated lncRNAs, 8 downregulated miRNAs, and 66 upregulated mRNAs, the mRNAs were involved in the biological processes and KEGG pathway was mainly related to cell cycle and cell division. For example, E2F transcription factor 1 (E2F1) and RING finger domains 1 (UHRF1) have been found to be closely related to the proliferation of muscle cells (32,33). Furthermore, the proliferation-related genes Forkhead box M1 (FoxM1) and Aurora kinase A (Aurka) play an important regulatory role in muscle regeneration (34,35). Our previous work showed that HDAC4 may be involved in denervation-induced muscle atrophy by regulating cell division and cell cycle. HDAC4 inhibition reversed the increase of CDKN1A, a cell cycle regulatory protein downstream of p53 gene, in denervated skeletal muscle (7). Here, KEGG analysis showed that the changes in target genes of upregulated miRNAs were associated with mitotically competent cells (“pathways in cancer”, “prostate cancer”, etc.). In the ceRNA network that the upregulated lncRNAs belonged to, the mRNAs participated in cell cycle-related biological processes and pathways. Muscle is a very heterogeneous tissue with many different cell types, and thus how the cell cycle-related genes participated in the HDAC4 regulation of muscle atrophy needs to be determined by further study. In another ceRNA network composed of 15 downregulated lncRNAs, 14 upregulated miRNAs, and 61 downregulated mRNAs, the mRNAs were involved in biological processes such as response to denervation implicated in regulation of muscle adaptation and positive regulation of fatty acid beta-oxidation, as well as pathways such as autophagy, FoxO signaling pathway, and Jak-STAT signaling pathway. In addition to the E3 ubiquitin ligase genes, FBXO32 and TRIM63, the SCN5A gene encoding the cardiac sodium channel alpha-subunit (Nav1.5) is involved in the biological processes related to denervation-induced skeletal muscle atrophy. SCN5A is usually expressed in neonatal skeletal muscle, which is downregulated rapidly after birth and increased again after denervation-induced skeletal muscle atrophy (36,37). Irs2 and Akt2 are involved in the positive regulation of fatty acid beta-oxidation and glucose metabolic processes. Insulin receptor substrate-1/2 (IRS1/2) is a key protein for insulin and/or insulin growth factor 1 signal transduction. IRS1 plays a leading role in skeletal muscle and is essential for normal myofiber growth and differentiation, insulin-dependent glucose uptake, and glycogen synthesis. As mentioned above, lncIRS1 can regulate the expression of IRS1 through sponging the miR-15 family, thereby alleviating muscle atrophy (16). Although IRS2 and IRS1 are very similar in structural and functional characteristics, they show tissue-specific divergence. The presence of IRS2 in skeletal muscle can be negligible for insulin-induced glucose uptake, and the role of IRS2 in muscle is still unclear (38). Our findings indicate that IRS1 is downregulated after denervation, while IRS2 is upregulated after denervation. Inhibition of HDAC4 can reverse the upregulation of IRS2 caused by denervation. In the models of different glucocorticoid-induced muscle atrophy, there are different changes in IRS2 expression. For instance, high-concentration methylprednisolone can induce an increase in IRS2 expression (39). Therefore, the role of IRS2 in denervation-induced skeletal muscle atrophy regulated by HDAC4 needs to be further explored. Akt2-deficient mice have glucose intolerance and present with systemic insulin resistance; however, normal skeletal muscle insulin signals, glucose tolerance, insulin sensitivity, and muscle weight have been detected in skeletal muscle-specific Akt2-knockout mice (40). Our previous studies have shown that fatty acid beta oxidation-related genes are generally downregulated 7 days after denervation (1). In this study, we found that β-hydroxy-coenzyme A dehydrogenase (HADH), fatty acid transporter 1 (FATP1/SLC27A1), fatty acid translocase (FAT/CD36), and membrane fatty acid binding protein (FABPpm/GOT2) were all downregulated after denervation. However, inhibition of HDAC4 could not reverse these downregulations caused by denervation. Nonetheless, downregulated miRNA target genes are involved in the regulation of fatty acid biosynthesis. Considering the complexity of miRNA regulatory networks and the expression changes of mRNA mentioned above, we speculated that the mechanism by which HDAC4 controls denervation-induced skeletal muscle atrophy might have little to do with fatty acid β-oxidation (41). In addition, we previously discovered through string analysis that SIK1, the key gene which may be involved in HDAC4 regulation of muscle atrophy is also involved in this ceRNA regulatory network (7). SIK1 is involved in the regulation of muscle cell differentiation and highly expressed after muscle injury (42).
Finally, we further identified the hub genes in the ceRNA network, including 3 lncRNAs, 4 miRNAs, and 12 mRNAs. In this subnetwork, XR_377582.2 and ENSMUST00000143649 bound to miR-3095-3p and played a core regulatory role by regulating fbxo32, trim63, Mpp6, Rras2, Irs2, Ubxn1, Sorbs3, and Lrrc58. After HDAC4 inhibition, the level of miR-3095-3p (log2 FC =2.89) was significantly upregulated, ranking second among all upregulated miRNAs, while XR_377582.2 (log2 FC =−2.703) and ENSMUST00000143649 (log2 FC =−2.723) levels were significantly downregulated. These mRNAs were mainly involved in biological processes, such as responses to denervation involved in regulation of muscle adaptation and cell migration, as well as the FoxO, Ras, and MAPK signaling pathways. The limitations of the network should be acknowledged. Systematic and rigorous experiments are warranted in the future to further verify our findings. Thus far, little has been reported on XR_377582.2, ENSMUST00000143649, and miR-3095-3p. miR-3095 can be found in the mouse version in the miRBase database.Only a few human and mouse lncRNAs are orthologues, and most of the lncRNAs are species-specific. Neither XR_377582.3 nor ENSMUST00000143649 appear to have a human orthologue on ENSEMBL or Blast. Therefore, our findings might be more important from a biological perspective and may provide a theoretical basis for the alleviation of skeletal atrophy by HDAC4.
In conclusion, all nodes in the ceRNA network directly or indirectly participate in the process of HDAC4 regulating denervation-induced skeletal muscle atrophy. XR_377582.2 and ENSMUST00000143649 may be the key lncRNAs related to HDAC4 involved in the regulation of muscle atrophy in mice. Our findings further provide a theoretical basis to explain how HDAC4 acts as a key target for effectively delaying denervation-induced skeletal muscle atrophy.
Acknowledgments
Funding: This work was supported by the Natural Science Foundation of Jiangsu Province (No. BK20201209), the National Natural Science Foundation of China (Grant Nos. 81871554 and 81901933), the QingLan Project at Jiangsu Universities, and the Priority Academic Program Development of Jiangsu Higher Education Institutions.
Footnote
Reporting Checklist: The authors have completed the ARRIVE reporting checklist. Available at https://atm.amegroups.com/article/view/10.21037/atm-21-6512/rc
Data Sharing Statement: Available at https://atm.amegroups.com/article/view/10.21037/atm-21-6512/dss
Conflicts of Interest: All authors have completed the ICMJE uniform disclosure form (available at https://atm.amegroups.com/article/view/10.21037/atm-21-6512/coif). The authors have no conflicts of interest to declare.
Ethical Statement: The authors are accountable for all aspects of the work in ensuring that questions related to the accuracy or integrity of any part of the work are appropriately investigated and resolved. Experiments were performed under a project license (No. 20180305-004) granted by the Jiangsu Laboratory Animal Management Committee, in compliance with the Nantong University guidelines for the care and use of animals.
Open Access Statement: This is an Open Access article distributed in accordance with the Creative Commons Attribution-NonCommercial-NoDerivs 4.0 International License (CC BY-NC-ND 4.0), which permits the non-commercial replication and distribution of the article with the strict proviso that no changes or edits are made and the original work is properly cited (including links to both the formal publication through the relevant DOI and the license). See: https://creativecommons.org/licenses/by-nc-nd/4.0/.
References
- Shen Y, Zhang R, Xu L, et al. Microarray Analysis of Gene Expression Provides New Insights Into Denervation-Induced Skeletal Muscle Atrophy. Front Physiol 2019;10:1298. [Crossref] [PubMed]
- Gordon T. Peripheral Nerve Regeneration and Muscle Reinnervation. Int J Mol Sci 2020;21:8652. [Crossref] [PubMed]
- Gu X, Ding F, Yang Y, et al. Construction of tissue engineered nerve grafts and their application in peripheral nerve regeneration. Prog Neurobiol 2011;93:204-30. [Crossref] [PubMed]
- Luo L, Martin SC, Parkington J, et al. HDAC4 Controls Muscle Homeostasis through Deacetylation of Myosin Heavy Chain, PGC-1α, and Hsc70. Cell Rep 2019;29:749-763.e12. [Crossref] [PubMed]
- Yoshihara T, Tsuzuki T, Chang SW, et al. Exercise preconditioning attenuates hind limb unloading-induced gastrocnemius muscle atrophy possibly via the HDAC4/Gadd45 axis in old rats. Exp Gerontol 2019;122:34-41. [Crossref] [PubMed]
- Mochalova EP, Belova SP, Kostrominova TY, et al. Differences in the Role of HDACs 4 and 5 in the Modulation of Processes Regulating MAFbx and MuRF1 Expression during Muscle Unloading. Int J Mol Sci 2020;21:4815. [Crossref] [PubMed]
- Ma W, Cai Y, Shen Y, et al. HDAC4 Knockdown Alleviates Denervation-Induced Muscle Atrophy by Inhibiting Myogenin-Dependent Atrogene Activation. Front Cell Neurosci 2021;15:663384. [Crossref] [PubMed]
- Yue B, Li H, Liu M, et al. Characterization of lncRNA-miRNA-mRNA Network to Reveal Potential Functional ceRNAs in Bovine Skeletal Muscle. Front Genet 2019;10:91. [Crossref] [PubMed]
- Hitachi K, Nakatani M, Funasaki S, et al. Expression Levels of Long Non-Coding RNAs Change in Models of Altered Muscle Activity and Muscle Mass. Int J Mol Sci 2020;21:1628. [Crossref] [PubMed]
- Wada S, Kato Y, Okutsu M, et al. Translational suppression of atrophic regulators by microRNA-23a integrates resistance to skeletal muscle atrophy. J Biol Chem 2011;286:38456-65. [Crossref] [PubMed]
- Kukreti H, Amuthavalli K, Harikumar A, et al. Muscle-specific microRNA1 (miR1) targets heat shock protein 70 (HSP70) during dexamethasone-mediated atrophy. J Biol Chem 2013;288:6663-78. [Crossref] [PubMed]
- Qiu J, Zhu J, Zhang R, et al. miR-125b-5p targeting TRAF6 relieves skeletal muscle atrophy induced by fasting or denervation. Ann Transl Med 2019;7:456. [Crossref] [PubMed]
- Qiu J, Wang L, Wang Y, et al. MicroRNA351 targeting TRAF6 alleviates dexamethasone-induced myotube atrophy. J Thorac Dis 2018;10:6238-46. [Crossref] [PubMed]
- Salmena L, Poliseno L, Tay Y, et al. A ceRNA hypothesis: the Rosetta Stone of a hidden RNA language? Cell 2011;146:353-8. [Crossref] [PubMed]
- Cesana M, Cacchiarelli D, Legnini I, et al. A long noncoding RNA controls muscle differentiation by functioning as a competing endogenous RNA. Cell 2011;147:358-69. [Crossref] [PubMed]
- Li Z, Cai B, Abdalla BA, et al. LncIRS1 controls muscle atrophy via sponging miR-15 family to activate IGF1-PI3K/AKT pathway. J Cachexia Sarcopenia Muscle 2019;10:391-410. [Crossref] [PubMed]
- Qiu J, Fang Q, Xu T, et al. Mechanistic Role of Reactive Oxygen Species and Therapeutic Potential of Antioxidants in Denervation- or Fasting-Induced Skeletal Muscle Atrophy. Front Physiol 2018;9:215. [Crossref] [PubMed]
- Wang W, Shen D, Zhang L, et al. SKP-SC-EVs Mitigate Denervated Muscle Atrophy by Inhibiting Oxidative Stress and Inflammation and Improving Microcirculation. Antioxidants (Basel) 2021;11:66. [Crossref] [PubMed]
- Cui Q, Yang H, Gu Y, et al. RNA sequencing (RNA-seq) analysis of gene expression provides new insights into hindlimb unloading-induced skeletal muscle atrophy. Ann Transl Med 2020;8:1595. [Crossref] [PubMed]
- Langmead B, Salzberg SL. Fast gapped-read alignment with Bowtie 2. Nat Methods 2012;9:357-9. [Crossref] [PubMed]
- Zhao Y, Lin Q, Li N, et al. MicroRNAs profiles of Chinese Perch Brain (CPB) cells infected with Siniperca chuatsi rhabdovirus (SCRV). Fish Shellfish Immunol 2019;84:1075-82. [Crossref] [PubMed]
- Tay Y, Kats L, Salmena L, et al. Coding-independent regulation of the tumor suppressor PTEN by competing endogenous mRNAs. Cell 2011;147:344-57. [Crossref] [PubMed]
- Wu G, Wan X, Xu B. A new estimation of protein-level false discovery rate. BMC Genomics 2018;19:567. [Crossref] [PubMed]
- Madaro L, Passafaro M, Sala D, et al. Denervation-activated STAT3-IL-6 signalling in fibro-adipogenic progenitors promotes myofibres atrophy and fibrosis. Nat Cell Biol 2018;20:917-27. [Crossref] [PubMed]
- Hitachi K, Nakatani M, Kiyofuji Y, et al. An Analysis of Differentially Expressed Coding and Long Non-Coding RNAs in Multiple Models of Skeletal Muscle Atrophy. Int J Mol Sci 2021;22:2558. [Crossref] [PubMed]
- Sartori R, Romanello V, Sandri M. Mechanisms of muscle atrophy and hypertrophy: implications in health and disease. Nat Commun 2021;12:330. [Crossref] [PubMed]
- Yang X, Ji Y, Wang W, et al. Amyotrophic Lateral Sclerosis: Molecular Mechanisms, Biomarkers, and Therapeutic Strategies. Antioxidants (Basel) 2021;10:1012. [Crossref] [PubMed]
- Bonaldo P, Sandri M. Cellular and molecular mechanisms of muscle atrophy. Dis Model Mech 2013;6:25-39. [Crossref] [PubMed]
- Moresi V, Williams AH, Meadows E, et al. Myogenin and class II HDACs control neurogenic muscle atrophy by inducing E3 ubiquitin ligases. Cell 2010;143:35-45. [Crossref] [PubMed]
- Horak M, Novak J, Bienertova-Vasku J. Muscle-specific microRNAs in skeletal muscle development. Dev Biol 2016;410:1-13. [Crossref] [PubMed]
- Huang QK, Qiao HY, Fu MH, et al. MiR-206 Attenuates Denervation-Induced Skeletal Muscle Atrophy in Rats Through Regulation of Satellite Cell Differentiation via TGF-β1, Smad3, and HDAC4 Signaling. Med Sci Monit 2016;22:1161-70. [Crossref] [PubMed]
- Luo W, Li G, Yi Z, et al. E2F1-miR-20a-5p/20b-5p auto-regulatory feedback loop involved in myoblast proliferation and differentiation. Sci Rep 2016;6:27904. [Crossref] [PubMed]
- Zheng TF, Liu XL, Li X, et al. Dickkopf-1 promotes Vascular Smooth Muscle Cell proliferation and migration through upregulating UHRF1 during Cyclic Stretch application. Int J Biol Sci 2021;17:1234-49. [Crossref] [PubMed]
- Wang YX, Feige P, Brun CE, et al. EGFR-Aurka Signaling Rescues Polarity and Regeneration Defects in Dystrophin-Deficient Muscle Stem Cells by Increasing Asymmetric Divisions. Cell Stem Cell 2019;24:419-432.e6. [Crossref] [PubMed]
- Chen Z, Li L, Xu S, et al. A Cdh1-FoxM1-Apc axis controls muscle development and regeneration. Cell Death Dis 2020;11:180. [Crossref] [PubMed]
- Carreras D, Martinez-Moreno R, Pinsach-Abuin ML, et al. Epigenetic Changes Governing Scn5a Expression in Denervated Skeletal Muscle. Int J Mol Sci 2021;22:2755. [Crossref] [PubMed]
- Hendrickse P, Galinska M, Hodson-Tole E, et al. An evaluation of common markers of muscle denervation in denervated young-adult and old rat gastrocnemius muscle. Exp Gerontol 2018;106:159-64. [Crossref] [PubMed]
- Eckstein SS, Weigert C, Lehmann R. Divergent Roles of IRS (Insulin Receptor Substrate) 1 and 2 in Liver and Skeletal Muscle. Curr Med Chem 2017;24:1827-52. [Crossref] [PubMed]
- Fappi A, Neves JC, Sanches LN, et al. Skeletal Muscle Response to Deflazacort, Dexamethasone and Methylprednisolone. Cells 2019;8:406. [Crossref] [PubMed]
- Jaiswal N, Gavin MG, Quinn WJ 3rd, et al. The role of skeletal muscle Akt in the regulation of muscle mass and glucose homeostasis. Mol Metab 2019;28:1-13. [Crossref] [PubMed]
- Fritzen AM, Lundsgaard AM, Kiens B. Tuning fatty acid oxidation in skeletal muscle with dietary fat and exercise. Nat Rev Endocrinol 2020;16:683-96. [Crossref] [PubMed]
- Stewart R, Akhmedov D, Robb C, et al. Regulation of SIK1 abundance and stability is critical for myogenesis. Proc Natl Acad Sci U S A 2013;110:117-22. [Crossref] [PubMed]
(English Language Editors: B. Meiser and J. Gray)