A validated nomogram integrating baseline peripheral T-lymphocyte subsets and NK cells for predicting survival in stage I–IIIA non-small cell lung cancer after resection
Introduction
Lung cancer remains the leading cause of cancer-related death worldwide, with non-small cell lung cancer (NSCLC) representing approximately 85% of all lung cancer cases (1,2). Advances in medical examination techniques have increased detection rates of early-stage lung cancer (3). Surgical resection is the first optimal treatment for non-advanced lung cancer (4,5). In spite of progress in diagnosis and treatment, the 5-year recurrence-free survival (RFS) rate and the 5-year overall survival (OS) rate of clinical stage I NSCLC patients were 73.2% and 79.5%, respectively (6). For patients with stage II–IIIA NSCLC, the 5-year OS rate after resection is estimated to be between 41–65% (7). Although postoperative adjuvant therapy can improve the 5-year OS of NSCLC patients, it has also been recently confirmed that neoadjuvant chemotherapy may achieve similar outcomes as adjuvant chemotherapy (8). For postoperative patients with NSCLC, recurrence and metastasis remain the key factors related to long-term survival (9). The tumor-node-metastasis (TNM) staging system is used to determine prognosis and provide guidance for treatment of NSCLC (10). However, nomograms have been proposed as an alternative standard over the traditional TNM staging system as predicting prognosis via TNM staging appears to be insufficient (11,12).
Nomograms are graphical calculating tools that have been widely applied in clinical practice to predict cancer outcomes by integrating patient factors and relevant hematological parameters (13). For many types of cancer, nomograms have been identified as a reliable approach for predicting a particular endpoint using statistical methods (14-17). There were nomograms which included immune genes as prognostic factors via The Cancer Genome Atlas (TCGA) database and Gene Expression Omnibus (GEO) (18). Besides, numerous studies have explored the influence of T-lymphocyte subsets and natural killer (NK) cells on the prognosis of cancers (19-21). For lung cancer in particular, T-lymphocyte subsets and NK cells are considered to be independent factors related to clinical outcomes such as progression-free survival (PFS) and OS (22,23). The relationship between immunity and lung cancer is complex. The composition of immune cells may predict response and act as an indicator of the ability of the immune system to eliminate residual disease after therapy. It has been found that the absence of lung CD4 cells with an effector-like phenotype (CD45RA+/CD27−) is a predictor for a favorable outcome (24). NK cells are generally defined as the first line of defense in the fight against infection and circulating cancer cells which can accelerate metastasis (25,26). Functionally, NK cells can produce cytokines that support T helper polarization and T cell activation, as well as stimulate dendritic cell (DC) and B cell maturation to bridge and orchestrate innate and adaptive immune responses (27). Hence, NK cells play an important role in tumor surveillance and can be manipulated by artificial activation techniques to present a highly effective anticancer tool against malignancies, and dependent on successful further rearming and mobilization, against solid tumors in the future (28). However, there are currently few studies on the relationship between the prognosis of stage I–IIIA NSCLC after resection and peripheral T-lymphocyte subsets combined with CD16+56 NK cells.
Several researches have confirmed that T-lymphocyte subsets and NK cells play important roles in the prognosis of NSCLC. The cancer cells may escape from the immune attack in the presence of immune function and pathological changes when aberrant alternation in the quantity and function of the T cell subsets happens (29). The high percentages of peripheral CD3+, CD4+T cells and CD4/CD8 ratio were associated with longer OS in lung cancer patients (30). The increased risk of lung cancer is related to low CD4 cell count and low CD4/CD8 ratio (31). Peripheral CD8+T cells was higher in lung cancer patients compared with the healthy control population, while CD3+, CD4+T cells and CD4/CD8 ratio was significantly lower (32). Moreover, the correlation between NK cell activity and PFS of NSCLC patients was verified (33). The median PFS was significantly better in the high NK group. In addition, CD16+56-NK cell subset is highly enriched in the tumor infiltrate and show activity markers such as CD69, HLA-DR, NKp44, etc. The CD16+56-NK cells enhance protumor neoangiogenesis through secretion of vascular endothelial growth factor (VEGF) (34).
It is essential to construct an efficient clinical model to predict the long-term survival of postoperative NSCLC patients. Hence, this study aimed to establish and validate an easy-to-use nomogram based on an integration of objective baseline clinical characteristics and hematological parameters, including T-lymphocyte subsets and CD16+56 NK cells, that could accurately predict the risk of recurrence and metastasis in patients who have received radical resection of pulmonary carcinoma. We present the following article in accordance with the TRIPOD reporting checklist (available at https://atm.amegroups.com/article/view/10.21037/atm-21-6347/rc).
Methods
Study design and patient selection
We performed a retrospective study of stage I–IIIA NSCLC patients who had received radical resection. Patients were collected at Shanghai Municipal Hospital of Traditional Chinese Medicine between 2010 and 2020. This study was approved by an independent ethics committee review board at Shanghai Municipal Hospital of Traditional Chinese Medicine (No. 2020SHL-KY-48). The procedures in this study were carried out in accordance with the principles of the Helsinki Declaration (as revised in 2013). Participants gave informed consent before taking part in this research.
Patients included in the study met the following criteria: (I) patients had undergone radical resection of pulmonary carcinoma; (II) patients had pathological diagnosis of NSCLC; (III) patients had stage I–IIIA disease; (IV) patients agreed to participate in the study, including regular follow-up; and (V) patients with Eastern Cooperative Oncology Group (ECOG) performance ≤2 (35). The exclusion criteria were as follows: (I) patients diagnosed with secondary lung cancer; (II) patients with severe/uncontrolled systemic diseases, including infection, rheumatic immune system disease, cardiovascular disease or liver and kidney insufficiency; (III) patients with other malignant tumors; (IV) patients who were pregnant; (V) patients with severe psychiatric disorders; and (VI) Karnofsky (KPS) score <60.
Assessment of clinical and laboratory parameters
The following data were collected for each patient: (I) basic information: admission number, patient name, age at diagnosis, gender, identity card number, and cellphone number; (II) cancer-related information: date of diagnosis, TNM stage, pathological category, and type of treatment; (III) personal history: smoking and drinking history; (IV) family history: tumor-related family history; and (V) peripheral T-lymphocyte subsets and CD16+56 NK cells.
Clinical outcome assessment and patient follow-up
The patients were followed up via telephone and clinical in-person visits. Disease-free survival (DFS) was defined as the time from the date of first diagnosis to the date of first relapse, cancer-related death, or the last follow-up time. OS was defined as the time from the date of first diagnosis to the date of cancer-related death. Additionally, the date of death was determined from the department of Cancer Control and Prevention via a database maintained by the Shanghai Municipal Center for Disease Control and Prevention of Cancer Patient Registration System. The last follow-up date was December 30, 2020.
X-tile analysis
X-tile software (Yale University School of Medicine, New Haven, USA) can provide a quantitative evaluation of every possible way of dividing a cohort into high- and low-marker expression (36). Further, when separate training and validation cohorts are not available, the software can provide a best P value evaluation by dividing a single cohort into training and validation subsets in order to produce a rigorous statistical estimation. In this study, the optimal cut-off values of CD3+T, CD4+T, CD8+T, CD4+T/CD8+T, and CD16+56 NK cells were determined by X-tile.
Data and statistical methods
Data were analyzed using IBM SPSS standard version 21.0 (SPSS Inc., Chicago, IL, USA) and R software version 4.1.0 (http://www.R-project.org). All data for continuous variables were presented as mean ± standard deviation (SD), and categorical variables were presented as a rate (%). Normally distributed data were analyzed using either Student’s t-test for 2 groups or 1-way ANOVA for 3 or more groups. Data with non-normal distribution were analyzed using nonparametric tests. Count data were analyzed with the Chi-square test. Prognostic factors for DFS and OS were assessed by univariate and multivariate analysis. The log-rank test were used to compare survival curves among different groups. All statistical tests were 2-tailed. Statistical significance was set at P<0.05.
Receiver operating characteristics (ROC) curves and the area under the ROC (AUC) curve were used to compare prognostic factors. In addition, we calculated the concordance index (C-index) to assess the accuracy of the nomogram for predicting DFS. The bootstrap method was used to validate the nomogram.
Results
Patient clinical characteristics
The baseline characteristics of study population are shown in Table 1. A total of 306 NSCLC patients met the inclusion criteria and were enrolled in the retrospective study. There were 206 patients in the training cohort and 100 patients in the validation cohort. In the training cohort, 60 patients (29.13%) were male and 146 patients (70.87%) were female. There were 176 patients (85.44%) at stage I, 18 patients (8.74%) at stage II, and 12 patients (5.83%) at stage IIIA. There were 196 cases (95.15%) of adenocarcinoma and 6 cases (2.91%) of squamous cell cancer. In total, 67 patients (32.52%) had a family history of cancer. Former smokers and former drinkers made up 16.02% and 5.83% of the subjects, respectively. The optimal cut-off values for CD3+T, CD4+T, CD8+T, CD4+T/CD8+T, and CD16+56 NK cells were determined via X-tile and were 75.30% (Figure 1), 43.60% (Figure 2), 32.00% (Figure 3), 1.3 (Figure 4), and 15.81% (Figure 5), respectively.
Table 1
Variables | Training cohort (N=206) | Validation cohort (N=100) | |||
---|---|---|---|---|---|
n | % | n | % | ||
Gender | |||||
Male | 60 | 29.13 | 30 | 30.00 | |
Female | 146 | 70.87 | 70 | 70.00 | |
Age | |||||
<68 years | 176 | 85.44 | 79 | 79.00 | |
≥68 years | 30 | 14.56 | 21 | 21.00 | |
TNM stage (AJCC, 8th) | |||||
I | 176 | 85.44 | 82 | 82.00 | |
II | 18 | 8.74 | 9 | 9.00 | |
IIIA | 12 | 5.83 | 9 | 9.00 | |
Pathology | |||||
Adenocarcinoma | 196 | 95.15 | 86 | 86.00 | |
Squamous cell cancer | 6 | 2.91 | 7 | 7.00 | |
Other types | 4 | 1.94 | 7 | 7.00 | |
Family history | |||||
Yes | 67 | 32.52 | 41 | 41.00 | |
No | 139 | 67.48 | 59 | 59.00 | |
Smoking history | |||||
Yes | 33 | 16.02 | 20 | 20.00 | |
No | 173 | 83.98 | 80 | 80.00 | |
Drinking history | |||||
Yes | 10 | 4.85 | 9 | 9.00 | |
No | 196 | 95.15 | 91 | 91.00 | |
CD3+T | |||||
≤75.30% | 150 | 72.82 | 83 | 83.00 | |
>75.30% | 56 | 27.18 | 18 | 18.00 | |
CD4+T | |||||
≤43.60% | 137 | 66.50 | 72 | 72.00 | |
>43.60% | 69 | 33.50 | 28 | 28.00 | |
CD8+T | |||||
≤32.00% | 181 | 87.86 | 84 | 84.00 | |
>32.00% | 25 | 12.14 | 16 | 16.00 | |
CD4+T/CD8+T | |||||
≤1.3 | 52 | 25.24 | 22 | 22.00 | |
>1.3 | 154 | 74.76 | 78 | 78.00 | |
CD16+56 NK cells | |||||
≤15.81% | 87 | 42.23 | 38 | 38.00 | |
>15.81% | 119 | 57.77 | 62 | 62.00 |
NSCLC, non-small-cell lung cancer; TNM, tumor-node-metastasis; AJCC, American Joint Committee on Cancer.
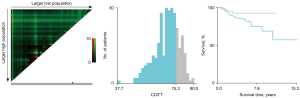
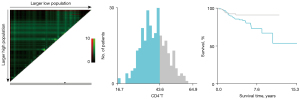
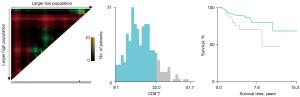
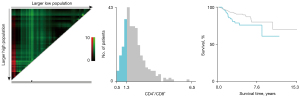
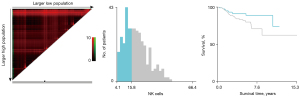
Univariate and multivariate Cox analysis of survival time
A univariate regression model was used to investigate DFS prediction based on the baseline demographic, clinical, and laboratory variables, including CD3+T, CD4+T, CD8+T, CD4+T/CD8+T, and CD16+56 NK cells. As shown in Table 2, the prognostic factors with P value <0.1 in the training cohort were as follows: gender, TNM stage, smoking history, drinking history, and CD4+T/CD8+T. Further multivariate analysis found that gender, TNM stage, drinking history, and CD4+T/CD8+T were independent factors for DFS. In addition, gender, TNM stage, and drinking history were related to OS.
Table 2
Variables | DFS | OS | ||||||
---|---|---|---|---|---|---|---|---|
Univariate analysis | Multivariate analysis | Univariate analysis | ||||||
HR (95% CI) | P | HR (95% CI) | P | HR (95% CI) | P | |||
Gender | ||||||||
Male | 4.232 (1.862–9.616) | 3.799 (1.239–11.655) | 5.471 (1.796–16.665) | |||||
Female | Reference | 0.001* | Reference | 0.020* | Reference | 0.003* | ||
Age | ||||||||
<68 years | Reference | Reference | ||||||
≥68 years | 1.397 (0.520–3.751) | 0.507 | 1.447 (0.404–5.178) | 0.570 | ||||
TNM stage (AJCC, 8th) | ||||||||
I | Reference | Reference | Reference | |||||
II | 4.703 (1.626–13.600) | 0.004* | 5.792 (1.862–18.012) | 0.002* | 2.170 (0.460–10.242) | 0.328 | ||
IIIA | 11.944 (4.793–29.765) | <0.001* | 14.903 (5.249–42.307) | <0.001* | 9.697 (3.096–30.371) | <0.001* | ||
Pathology | ||||||||
Adenocarcinoma | Reference | Reference | ||||||
Squamous cell cancer | 1.146 (0.154–8.519) | 0.894 | NA | 0.640 | ||||
Other types | NA | 0.974 | NA | 0.547 | ||||
Family history | ||||||||
Yes | 0.492 (0.190–1.270) | 0.142 | 0.757 (0.246–2.333) | 0.628 | ||||
No | Reference | Reference | ||||||
Smoking history | ||||||||
Yes | 3.738 (1.628–8.585) | 0.002* | 0.612 (0.187–2.008) | 0.418 | 2.401 (0.747–7.722) | 0.142 | ||
No | Reference | Reference | ||||||
Drinking history | ||||||||
Yes | 3.663 (1.240–10.819) | 0.019* | 8.779 (2.222–34.684) | 0.002* | 4.594 (1.273–16.577) | 0.020* | ||
No | Reference | Reference | Reference | |||||
CD3+T | ||||||||
≤75.30% | Reference | Reference | ||||||
>75.30% | 0.506 (0.173–1.476) | 0.212 | 0.406 (0.092–1.801) | 0.236 | ||||
CD4+T | ||||||||
≤43.60% | Reference | Reference | ||||||
>43.60% | 0.529 (0.198–1.411) | 0.203 | 0.821 (0.260–2.590) | 0.737 | ||||
CD8+T | ||||||||
≤32.00% | Reference | Reference | ||||||
>32.00% | 1.390 (0.477–4.053) | 0.546 | 0.537 (0.071–4.087) | 0.548 | ||||
CD4+T/CD8+T | ||||||||
≤1.3 | Reference | Reference | Reference | |||||
>1.3 | 0.437 (0.194–0.986) | 0.046* | 0.312 (0.124–0.790) | 0.014* | 0.813 (0.255–2.595) | 0.727 | ||
CD16+56 NK cells | ||||||||
≤15.81% | Reference | Reference | 0.842 | |||||
>15.81% | 1.659 (0.715–3.846) | 0.238 | 0.900 (0.320–2.530) |
*P<0.05. DFS, disease-free survival; OS, overall survival; HR, hazard ratio; TNM, tumor-node-metastasis; AJCC, American Joint Committee on Cancer.
Development of a prognostic nomogram
Based on the multivariate cox analysis, significant independent factors for DFS were integrated to create the nomogram, including gender, TNM stage, drinking history, and CD4+T/CD8+T (Figure 6). The nomogram demonstrated that TNM stage and drinking history contributed the most to prognosis, followed by the ratio of CD4+T/CD8+T. Gender had relatively little impact on survival. By calculating the total score and comparing it to the highest possible score, the predicted 3- and 5-year DFS were easy to obtain.
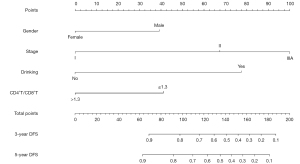
Calibration and validation of the nomogram
The calibration plot indicated an excellent consistency between the nomogram prediction and the actual survival rate for 3- and 5-year DFS in the training cohort (Figure 7A,7B). Further, it also showed an acceptable agreement in the external validation cohort (Figure 8A,8B). In the training cohort, the C-index for the nomogram to predict DFS was 0.839, which indicated good prediction efficiency of the model. In addition, the prediction efficiency of the nomogram model was assessed by ROC analysis. In the training cohort, 3- and 5-year AUC were 0.860 and 0.847, respectively (Figure 9A). In the validation cohort, 3- and 5-year AUC were 0.726 and 0.748, respectively (Figure 9B). The above findings suggested that our nomogram had more significant potential for the prediction of recurrence compared to the American Joint Committee on Cancer (AJCC) staging system.
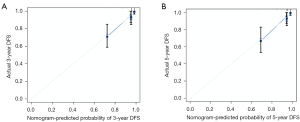
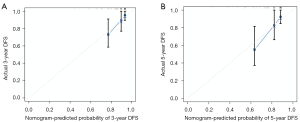
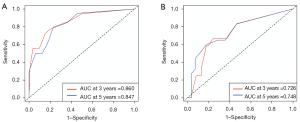
Discussion
In this retrospective study, we delivered significantly better results using one of the first nomograms that can predict DFS in stage I–IIIA NSCLC patients based on a relatively large real-world population-based database. We identified gender, drinking history, the ratio of CD4+T/CD8+T, along with TNM stage as independent factors for prediction via multivariate cox analysis. Most of these independent factors were consistent with a host of previous findings on risk factors for NSCLC (37-39). Subsequently, the above 4 predictors were integrated into a nomogram to calculate the risk probability of disease recurrence or metastasis tailored to each individual patient. In addition, CD4+T/CD8+T (≤1.3) was first identified as a risk factor for DFS. Our nomogram showed that male NSCLC patients with relatively late TNM stage, drinking history, and low ratio of CD4+T/CD8+T had a greater risk of disease progression after resection. Regrettably, CD16+56 natural killer (NK) cells were not proven to be significant independent factors for DFS, which may be related to the relatively small sample size of this study. In fact, one of our objectives for developing this new model was to show the correlation between peripheral T-lymphocyte subsets and DFS in a real-world Chinese population.
The use of immunotherapy for cancer treatment has been explored for many years, with numerous patients benefiting from long-term survival. Immune cell infiltration is considered an important determinant of the immune microenvironment of a malignant tumor, of which T cells are the main component of the anticancer immune system. For example, in metastatic castration-resistant prostate cancer, a high proportion of CD4+T, CD8+T, and CD56bright NK cells has been found to be a favorable prognostic factor (34). Similarly, an increase in the proportion of CD8+T/CD28− T cells was verified in women with lung cancer (40) and breast cancer (41) during chemotherapy. On the contrary, some types of tumor-infiltrating inflammatory cells, such as regulatory T cells (Tregs) and myeloid-derived suppressor cells (MDSCs), could accelerate angiogenesis and support tumor growth (42). Tregs are abundant in NSCLC and predicted for an increased risk of disease recurrence in early-stage disease (43). As a kind of specialized T-cell subpopulation, Tregs could suppress sterilizing immune response. As a result, it is anticipated that tumor-infiltrating immune/inflammatory cells may be significant hallmarks for immune monitoring of baseline peripheral blood in both treated and non-treated patients after resection (44).
The poor outcomes for NSCLC patients remain a major challenge for oncologists, and a more practical and valuable prognostic marker than TNM classification is urgently needed. Despite an increasing number of new nomograms for lung cancer, none of those combining peripheral T-lymphocyte subsets and CD16+56 NK cells have been established for I–IIIA resected NSCLC patients. It is notable that the Surveillance, Epidemiology, and End Result (SEER) database, which has a considerably large population, has contributed to the construction of numerous nomograms for cancers (45-47). However, the SEER database also has many imperfections, including lack of clinical data on some hematology parameters and missing outcomes such as tumor recurrence. There is no doubt that oncologists should pay more attention to cancer recurrence and metastasis rather than cancer death, particularly in patients who have undergone radical surgery.
The present study oversaw the development of the first prognostic model to predict DFS using a peripheral blood immunological index for stage I–IIIA NSCLC after resection. Nevertheless, a number of inevitable limitations existed in this work. First, this retrospective cohort study was conducted in Shanghai Municipal Hospital of Traditional Chinese Medicine. All enrolled patients received treatment involving traditional Chinese medicine. Previously, we conducted several clinical studies (9,30) confirming that traditional Chinese medicine prolonged DFS in lung cancer patients, especially those after radical resection of non-advanced lung adenocarcinoma. The intervention of traditional Chinese medicine reduced the progressive disease rate, and thus the number of outcome events was reduced correspondingly. Further, the retrospective nature and limited sample may have led to selection bias. There is a high likelihood that some untreated patients were in a worse state than those given the option of treatment, despite this not always being recorded in medical records. In addition, worse baseline clinical parameters could have given rise to longer survival due to more treatment interventions than that reported by blinded clinical trials. Finally, we did not find another independent cohort for external validation.
Conclusions
In this study, gender, drinking history, TNM stage, and CD4+T/CD8+T were shown to be independent factors for DFS. To visualize and integrate these 4 factors, we developed a prognostic model which could provide individual prediction of DFS for stage I–IIIA patients after resection. This practical prognostic tool may help oncologists in clinical treatment planning. However, more data from other cohorts are needed to further validate this nomogram.
Acknowledgments
Funding: This study was supported by the National Natural Science Foundation of China (No. 81973795), Clinical Research Plan of Shanghai Shenkang Hospital Development Center (No. SHDC2020CR4052), and the Shanghai Pujiang Program (No. 2020PJD057).
Footnote
Reporting Checklist: The authors have completed the TRIPOD reporting checklist. Available at https://atm.amegroups.com/article/view/10.21037/atm-21-6347/rc
Data Sharing Statement: Available at https://atm.amegroups.com/article/view/10.21037/atm-21-6347/dss
Conflicts of Interest: All authors have completed the ICMJE uniform disclosure form (available at https://atm.amegroups.com/article/view/10.21037/atm-21-6347/coif). The authors have no conflicts of interest to declare.
Ethical Statement: The authors are accountable for all aspects of the work in ensuring that questions related to the accuracy or integrity of any part of the work are appropriately investigated and resolved. This study was approved by an independent ethics committee review board at Shanghai Municipal Hospital of Traditional Chinese Medicine (No. 2020SHL-KY-48). The procedures in this study were carried out in accordance with the principles of the Helsinki Declaration (as revised in 2013). Participants gave informed consent before taking part in this research.
Open Access Statement: This is an Open Access article distributed in accordance with the Creative Commons Attribution-NonCommercial-NoDerivs 4.0 International License (CC BY-NC-ND 4.0), which permits the non-commercial replication and distribution of the article with the strict proviso that no changes or edits are made and the original work is properly cited (including links to both the formal publication through the relevant DOI and the license). See: https://creativecommons.org/licenses/by-nc-nd/4.0/.
References
- Siegel RL, Miller KD, Jemal A. Cancer statistics, 2020. CA Cancer J Clin 2020;70:7-30. [Crossref] [PubMed]
- He Y, Chen L, Zhao L, et al. Genomic and transcriptional alterations in first-line chemotherapy exert a potentially unfavorable influence on subsequent immunotherapy in NSCLC. Theranostics 2021;11:7092-109. [Crossref] [PubMed]
- National Lung Screening Trial Research Team. Reduced lung-cancer mortality with low-dose computed tomographic screening. N Engl J Med 2011;365:395-409. [Crossref] [PubMed]
- Chen J, Gu C, Chen X, et al. Clinicopathological and prognostic analyses of 86 resected pulmonary lymphoepithelioma-like carcinomas. J Surg Oncol 2021;123:544-52. [Crossref] [PubMed]
- Bao Y, Gu C, Xie H, et al. Comprehensive study of neoadjuvant targeted therapy for resectable non-small cell lung cancer. Ann Transl Med 2021;9:493. [Crossref] [PubMed]
- Deng J, Zhao M, Wang T, et al. A modified T categorization for part-solid lesions in Chinese patients with clinical stage I Non-small cell lung cancer. Lung Cancer 2020;145:33-9. [Crossref] [PubMed]
- Goldstraw P, Chansky K, Crowley J, et al. The IASLC Lung Cancer Staging Project: Proposals for Revision of the TNM Stage Groupings in the Forthcoming (Eighth) Edition of the TNM Classification for Lung Cancer. J Thorac Oncol 2016;11:39-51. [Crossref] [PubMed]
- NSCLC Meta-analysis Collaborative Group. Preoperative chemotherapy for non-small-cell lung cancer: a systematic review and meta-analysis of individual participant data. Lancet 2014;383:1561-71. [Crossref] [PubMed]
- Zhang S, Chen W, Wang Y, et al. Chinese Herbal Prescription Fu-Zheng-Qu-Xie Prevents Recurrence and Metastasis of Postoperative Early-Stage Lung Adenocarcinoma: A Prospective Cohort Study Followed with Potential Mechanism Exploration. Oxid Med Cell Longev 2021;2021:6673828. [Crossref] [PubMed]
- Zeng Y, Mayne N, Yang CJ, et al. A Nomogram for Predicting Cancer-Specific Survival of TNM 8th Edition Stage I Non-small-cell Lung Cancer. Ann Surg Oncol 2019;26:2053-62.
- Iasonos A, Schrag D, Raj GV, et al. How to build and interpret a nomogram for cancer prognosis. J Clin Oncol 2008;26:1364-70. [Crossref] [PubMed]
- Liao Y, Wang X, Zhong P, et al. A nomogram for the prediction of overall survival in patients with stage II and III non-small cell lung cancer using a population-based study. Oncol Lett 2019;18:5905-16. [Crossref] [PubMed]
- Liang W, Zhang L, Jiang G, et al. Development and validation of a nomogram for predicting survival in patients with resected non-small-cell lung cancer. J Clin Oncol 2015;33:861-9. [Crossref] [PubMed]
- Yan B, Su BB, Bai DS, et al. A practical nomogram and risk stratification system predicting the cancer-specific survival for patients with early hepatocellular carcinoma. Cancer Med 2021;10:496-506. [Crossref] [PubMed]
- Chen M, Li S, Topatana W, et al. Development and Validation of a Nomogram for Predicting Survival in Gallbladder Cancer Patients With Recurrence After Surgery. Front Oncol 2021;10:537789. [Crossref] [PubMed]
- Chen D, Liu Z, Liu W, et al. Predicting postoperative peritoneal metastasis in gastric cancer with serosal invasion using a collagen nomogram. Nat Commun 2021;12:179. [Crossref] [PubMed]
- Nelson DW, Merritt C, Chang SC, et al. Development of a Risk Score and Nomogram to Predict Individual Benefit Attained from the Addition of Adjuvant Chemotherapy in the Treatment of Stage II Colon Cancer. J Gastrointest Surg 2021;25:220-32. [Crossref] [PubMed]
- Luo C, Lei M, Zhang Y, et al. Systematic construction and validation of an immune prognostic model for lung adenocarcinoma. J Cell Mol Med 2020;24:1233-44. [Crossref] [PubMed]
- Kroemer M, Turco C, Spehner L, et al. Investigation of the prognostic value of CD4 T cell subsets expanded from tumor-infiltrating lymphocytes of colorectal cancer liver metastases. J Immunother Cancer 2020;8:e001478. [Crossref] [PubMed]
- Wang M, Huang YK, Kong JC, et al. High-dimensional analyses reveal a distinct role of T-cell subsets in the immune microenvironment of gastric cancer. Clin Transl Immunology 2020;9:e1127. [Crossref] [PubMed]
- Li H, Wang C, Lan L, et al. High expression of vinculin predicts poor prognosis and distant metastasis and associates with influencing tumor-associated NK cell infiltration and epithelial-mesenchymal transition in gastric cancer. Aging (Albany NY) 2021;13:5197-225. [Crossref] [PubMed]
- Kotsakis A, Koinis F, Katsarou A, et al. Prognostic value of circulating regulatory T cell subsets in untreated non-small cell lung cancer patients. Sci Rep 2016;6:39247. [Crossref] [PubMed]
- Fend L, Rusakiewicz S, Adam J, et al. Prognostic impact of the expression of NCR1 and NCR3 NK cell receptors and PD-L1 on advanced non-small cell lung cancer. Oncoimmunology 2017;6:e1163456. [Crossref] [PubMed]
- Zikos TA, Donnenberg AD, Landreneau RJ, et al. Lung T-cell subset composition at the time of surgical resection is a prognostic indicator in non-small cell lung cancer. Cancer Immunol Immunother 2011;60:819-27. [Crossref] [PubMed]
- Luo Y, Wu J, Zhu X, et al. NK Cell-Dependent Growth Inhibition of Lewis Lung Cancer by Yu-Ping-Feng, an Ancient Chinese Herbal Formula. Mediators Inflamm 2016;2016:3541283. [Crossref] [PubMed]
- Pockley AG, Vaupel P, Multhoff G. NK cell-based therapeutics for lung cancer. Expert Opin Biol Ther 2020;20:23-33. [Crossref] [PubMed]
- Vivier E, Raulet DH, Moretta A, et al. Innate or adaptive immunity? The example of natural killer cells. Science 2011;331:44-9. [Crossref] [PubMed]
- Hamilton G, Plangger A. The Impact of NK Cell-Based Therapeutics for the Treatment of Lung Cancer for Biologics: Targets and Therapy. Biologics 2021;15:265-77. [PubMed]
- Ye L, Zhang F, Li H, et al. Circulating Tumor Cells Were Associated with the Number of T Lymphocyte Subsets and NK Cells in Peripheral Blood in Advanced Non-Small-Cell Lung Cancer. Dis Markers 2017;2017:5727815. [Crossref] [PubMed]
- Dai S, Ren P, Ren J, et al. The Relationship between Lymphocyte Subsets and the Prognosis and Genomic Features of Lung Cancer: A Retrospective Study. Int J Med Sci 2021;18:2228-34. [Crossref] [PubMed]
- Sigel K, Wisnivesky J, Crothers K, et al. Immunological and infectious risk factors for lung cancer in US veterans with HIV: a longitudinal cohort study. Lancet HIV 2017;4:e67-73. [Crossref] [PubMed]
- Woo EY, Yeh H, Chu CS, et al. Cutting edge: Regulatory T cells from lung cancer patients directly inhibit autologous T cell proliferation. J Immunol 2002;168:4272-6. [Crossref] [PubMed]
- Choi MG, Kim YJ, Lee JC, et al. Efficacy of natural killer cell activity as a biomarker for predicting immunotherapy response in non-small cell lung cancer. Thorac Cancer 2020;11:3337-45. [Crossref] [PubMed]
- Pachynski RK, Morishima C, Szmulewitz R, et al. IL-7 expands lymphocyte populations and enhances immune responses to sipuleucel-T in patients with metastatic castration-resistant prostate cancer (mCRPC). J Immunother Cancer 2021;9:e002903. [Crossref] [PubMed]
- Oken MM, Creech RH, Tormey DC, et al. Toxicity and response criteria of the Eastern Cooperative Oncology Group. Am J Clin Oncol 1982;5:649-55. [Crossref] [PubMed]
- Camp RL, Dolled-Filhart M, Rimm DL. X-tile: a new bio-informatics tool for biomarker assessment and outcome-based cut-point optimization. Clin Cancer Res 2004;10:7252-9. [Crossref] [PubMed]
- Xu LL, Zhang SF, Wang YL, et al. The Efficacy of Long-Term Chinese Herbal Medicine Use on Lung Cancer Survival Time: A Retrospective Two-Center Cohort Study with Propensity Score Matching. Evid Based Complement Alternat Med 2021;2021:5522934. [Crossref] [PubMed]
- Wang Z, Li H, Liu T, et al. Development and External Validation of a Nomogram for Predicting Cancer-Specific Survival of Non-Small Cell Lung Cancer Patients With Ipsilateral Pleural Dissemination. Front Oncol 2021;11:645486. [Crossref] [PubMed]
- Wu Z, Ouyang C, Peng L. A Novel Nomogram Based on Immune Scores for Predicting Survival in Patients with Early-Stage Non-Small Cell Lung Cancer (NSCLC). Med Sci Monit 2020;26:e923231. [Crossref] [PubMed]
- Meloni F, Morosini M, Solari N, et al. Foxp3 expressing CD4+ CD25+ and CD8+CD28- T regulatory cells in the peripheral blood of patients with lung cancer and pleural mesothelioma. Hum Immunol 2006;67:1-12. [Crossref] [PubMed]
- Song G, Wang X, Jia J, et al. Elevated level of peripheral CD8(+)CD28(-) T lymphocytes are an independent predictor of progression-free survival in patients with metastatic breast cancer during the course of chemotherapy. Cancer Immunol Immunother 2013;62:1123-30. [Crossref] [PubMed]
- Grivennikov SI, Greten FR, Karin M. Immunity, inflammation, and cancer. Cell 2010;140:883-99. [Crossref] [PubMed]
- Principe DR, Chiec L, Mohindra NA, et al. Regulatory T-Cells as an Emerging Barrier to Immune Checkpoint Inhibition in Lung Cancer. Front Oncol 2021;11:684098. [Crossref] [PubMed]
- Ino Y, Yamazaki-Itoh R, Shimada K, et al. Immune cell infiltration as an indicator of the immune microenvironment of pancreatic cancer. Br J Cancer 2013;108:914-23. [Crossref] [PubMed]
- Yu X, Gao S, Xue Q, et al. Development of a nomogram for predicting the operative mortality of patients who underwent pneumonectomy for lung cancer: a population-based analysis. Transl Lung Cancer Res 2021;10:381-91. [Crossref] [PubMed]
- Zuo Z, Zhang G, Song P, et al. Survival Nomogram for Stage IB Non-Small-Cell Lung Cancer Patients, Based on the SEER Database and an External Validation Cohort. Ann Surg Oncol 2021;28:3941-50. [Crossref] [PubMed]
- Liu Q, Li W, Xie M, et al. Development and validation of a SEER-based prognostic nomogram for cervical cancer patients below the age of 45 years. Bosn J Basic Med Sci 2021;21:620-31. [PubMed]
(English Language Editor: A. Muijlwijk)