Subpopulations of cancer-associated fibroblasts link the prognosis and metabolic features of pancreatic ductal adenocarcinoma
Introduction
Pancreatic ductal adenocarcinoma (PDAC) is one of the most malignant tumors of digestive tract and the seventh leading cause of cancer death in all sexes, with a median survival of 6 months and a 5-year survival rate of <8% (1). Despite improvements in surgical techniques and therapy regimens, existing systemic medications for advanced PDAC patients give only transient relief, underscoring the need for novel therapeutic solutions.
PDAC is distinguished by extensive desmoplasia, which accounts for 90% of the total tumor volume and includes extracellular matrix (ECM), immune cells, vasculature, and cancer-associated fibroblasts (CAFs) (2,3). Of these, CAFs are the majority. CAFs may originate from bone marrow-derived mesenchymal stem cells attracted to the tumor, or may be transformed from adipocytes, pericytes, or endothelial cells, but are predominantly derived from tissue resident fibroblasts in the pancreas. CAFs have a wide range of functions due to their adaptability and plasticity. The alterations in extracellular matrix production and tumor mechanics that result in a large part from the action of CAFs have complex consequences for tumors. Increased tissue stiffness triggers pro-survival and pro-proliferation signaling in cancer cells (4). Increased mechanical stress could collapse blood vessels, leading to hypoxia, promoting more aggressive cancer phenotypes, and reducing drug delivery (5-8). Contrary to these tumor-facilitated principles, 2 studies indicated that removing stroma completely resulted in more aggressive tumors with worse overall survival (OS), highlighting the complexities of the stromal-tumor interaction (9,10).
This paradoxical phenomenon has provoked wide debate accompanied by the formulation of a hypothesis that subtypes of CAFs with distinct phenotypes exist in PDAC. To address this possibility, several groups have performed gene-expression profiling on genetically engineered PDAC mouse models or patient samples. Öhlund et al. identified 2 distinct CAF subpopulations, one with elevated expression of α-smooth muscle actin (α-SMA) located immediately adjacent to neoplastic cells and producing desmoplastic stroma, which were termed myofibroblastic CAFs (myCAFs); another subpopulation of CAFs, located more distantly from neoplastic cells that lacked elevated α-SMA expression and instead secreted interleukin (IL)-6 and additional inflammatory mediators were termed inflammatory CAFs (iCAFs). Furthermore, CAFs were shown to be dynamic, with variable phenotypes depending on their spatial and biochemical niche within the PDAC microenvironment (11). A recent study based on single-cell RNA-seq (scRNA-seq) analysis also highlighted the existence of different CAF populations in PDAC. Beside the classical myCAFs and iCAFs, a third population named antigen-presenting CAFs (apCAFs) was found, expressing the MHC II complex and CD74 that were able to present antigen to T cells in vitro (12). The findings all suggest the existence of multiple subpopulations of CAFs with distinct transcriptional programs. However, the functions of these subgroups remain unclear, particularly the relationship between these subgroups and patient prognosis. In this study, we take advantage of all published human PDAC scRNA-seq samples and identified ~7,000 CAFs for robust cluster discovery, and introduced a novel algorism, scFrac, allowing calculation of enrichment score of all subgroups of CAFs in bulk RNA-seq of PDAC, to determine the effect of the different CAF subtypes on patient survival based on the patient cohort of our center.
We present the following article in accordance with the REMARK reporting checklist (available at https://atm.amegroups.com/article/view/10.21037/atm-22-407/rc).
Methods
Clinical samples
An external validation group of 66 patients from the Shanghai General Hospital and Minhang Hospital between 2016 and 2021 were enrolled in this study. All procedures performed in this study involving human participants were in accordance with the Declaration of Helsinki (as revised in 2013). All clinical samples were collected with informed consent from the patients, and the research was approved by the ethics committees of Shanghai General Hospital, Shanghai Jiao Tong University School of Medicine (China) (No. 2021-130) and Minhang Hospital, Fudan University (China) (No. 2021-066-01K).
Data collection
The FPKM-normalized mRNA expression profiles and clinical features of ~170 patients with PDAC were downloaded from The Cancer Genome Atlas (https://portal.gdc.cancer.gov/). For single-cell RNA-seq data, 24 human PDAC tumor samples were collected from the Genome Sequence Archive (GSA) with accession number CRA001160 [NGDC-GSA (cncb.ac.cn)]. WE also collected 4 mouse KPC tumor samples from the Gene Expression Omnibus with accession number GSE129455.
scRNA-seq data procession
We applied Seurat 4.0 for scRNA-seq data procession. We routinely excluded single cells with <1,000 or >5,000 UMIs. To remove batch effects among samples, we applied the “IntergrateData” method. The “FindAllMarkers” method was used to identify major cell types. Each cell type was recognized by marker genes using “CellMarker” database (http://biocc.hrbmu.edu.cn/CellMarker/).
Gene-set enrichment analysis
Single-sample gene-set enrichment analysis (ssGSEA) was performed to evaluate the enrichment of the gene set of interest in TCGA-PDAC samples using R package GSVA. Gene signature collections were downloaded from the Molecular Signatures Database (http://www.gsea-msigdb.org/gsea/msigdb).
Evaluation of metabolic activity at single-cell resolution
A newly introduced algorithm, “scMetabolism”, generally supports the quantification and visualization of metabolism at single-cell resolution. We applied the algorithm to decipher the metabolic activity of 78 metabolic-associated pathways in human PDAC CAFs.
Transcription factor (TF) analysis
In an attempt to understand the regulatory activity differences of TFs in the CAF subtypes, single‐cell regulatory network inference and clustering (SCENIC) was used to score the regulon. The adj.P value<0.05 was considered as significant. The activity of the top regulons in each subgroup was displayed by heatmap.
Relative enrichment fraction of CAF subtypes in TCGA-PDAC
To allow for the convenient estimation of cell type proportions discovered by scRNA-seq in bulk-sample RNA-seq data, we introduced an SVR-based machine learning algorithm, “scFrac” (https://github.com/shenxiaotianCNS/scFrac). Similar deconvolution method has been introduced to analyze human and mouse sequence data (13,14). To our knowledge, this is the first time the full scRNA data set has been used to generate the X matrix. In this algorithm, we valued and made full usage of each single cell in the scRNA-seq, by deciphering the mixed matrix to the true, real, equal single-cell level, and using the summary of cell fractions belonging to the same subtype as the final enrichment fraction. Generally, biological mixed samples are modeled as a system of linear equations: a single mixture matrix X with n genes can be viewed as the product of Y and F, where Y is the n*m single-cell matrix containing n genes in X and m cells identified by scRNA-Seq. F represent a vector of size m, referring to the relative cell proportions for each cell. The sum of m is referred to as M, which is considered the relative enrichment fraction of the cell type.
Survival analysis
R package “Survminer” was used to performed survival analysis. Gene set used in this manuscript was downloaded from http://www.gsea-msigdb.org/gsea/msigdb. These calculation steps are taken from publicly available resources on the web.
Statistical analysis
Using R, Pearson correlation coefficients were calculated; survival analysis and Kaplan-Meier curves were constructed using the “survival” R package, and log-rank tests were used to determine significance. All statistical P values were two-tailed, with a significance level of P<0.05 being considered statistically significant. R 3.6.3 software was used to process all data.
Multiplex immunofluorescence (IF)
The IF was accomplished by staining 4 μm-thick formalin-fixed, paraffin-embedded whole-tissue sections sequentially with standard primary antibodies and then pairing with the TSA 7-color kit (D110071-50T, WiSee Bio), followed by DAPI staining. For instance, deparaffinized slides were incubated with antibody for 30 minutes and then treated for 10 minutes with an anti-rabbit/mouse horseradish peroxidase (HRP) secondary antibody (#A10011-60, Yuanxibio). The IF labeling was created for a rigorously enforced 10 minutes using TSA 620 in accordance with the manufacturer’s recommendations. Slides were washed in TBST buffer and then transferred to a warmed citrate solution (90 ℃) for 15 minutes. Slides were chilled to room temperature in the same solution. The slides were rinsed with Tris buffer in between each stage. The procedure was repeated with each antibody/fluorescent dye. Following that, each slide was treated with two drops of DAPI, rinsed in distilled water, and cover-slipped manually. The slides were dried in the air, mounted with anti-fade mounting media, and photographed using the Aperio Versa 8 tissue imaging equipment (Leica). Indica Halo software was used to analyze the images. The antibodies used in this study were CXCL12 (ab9797), α-SMA (ab7817), S100A4 (ab155326), pan-CK (ab7753), and PDGFR-alpha (ab203491).
Results
Identification of 3 subtypes of CAFs in PDAC
We first explored the heterogeneity of CAFs in human and mice pancreatic cancer using 2 single-cell sequencing data collections (12,15). A total of 6,933 CAFs in human tumor tissue and 4,000 CAFs in a mouse KPC model were included. Similar to previous findings (16), in the murine KPC model, 3 subtypes of CAF were identified: iCAF, myCAF and apCAF (Figure 1A). Notably, Elyada et al. did not discover apCAFs in human PDAC using single-cell analysis, probably due to the restricted cell number (12). In a larger PDAC data collection with ~60,000 cells, we identified a subpopulation of CAFs with the feature of high CD74 and MHCII molecule expressions (Figure 1B), indicating the presence of apCAFs in human PDAC, and they were transcriptome distinct against other subtype of CAFs. The markers of the CAF subtypes between humans and mice showed high consistency (Figure 1C,1D). Specifically, IL6, CXCL12, and DPT were overexpressed in iCAFs; HPOX (NDUFA4L2), ACTA2, and MYL9 were highly expressed in myCAFs; and CD74, HLA-DRB1 (H2-Ab1), and HLA-DRA were upregulated in apCAF. Taken together, these results suggested 3 subpopulations of CAFs existed in human PDAC and the mouse KPC model.
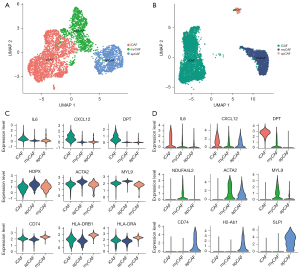
Molecular characterization of CAFs in PDAC
We established the gene signatures of each CAF subtype using their marker genes, which were chosen using a “1 vs. others” strategy. Overall, 204, 159 and 193 genes were used to construct the iCAF, myCAF and apCAF signatures, respectively. The most different expressed markers are shown in Figure 2A. KEGG pathway annotation demonstrated that complement and coagulation cascades, and cytokine-cytokine receptor interaction were dominant in iCAFs, and pathways related to focal adhesion, and ECM-receptor interaction showed dominance in myCAFs, while antigen processing and presentation were the top enriched pathways in apCAFs (Figure 2B-2D), which was consistent with the functional annotation of markers of CAF subtypes in the murine KPC model (12). We calculated the “ssGSEA enrichment score” of the signatures in the TCGA-PDAC cohort to score the enrichment of the 3 subtypes of CAFs in human pancreatic cancer, and calculated the Pearson correlation value between the enrichment score and “HALLMARK” gene sets to reveal their connection (Figure 2E). R value >0.7 or <−0.3 was considered as significant. Results indicated that all types of CAFs were inflammatory regulators. Some malignant-related “HALLMARKs”, such as epithelial-mesenchymal transition (EMT) or angiogenesis, were all positively correlated with CAFs. Oxidative phosphorylation (OXPHOS), however, negatively correlated with the iCAF and apCAF signatures. Hence, CAF subtypes exhibit specific genetic signatures and functional roles in PDAC.
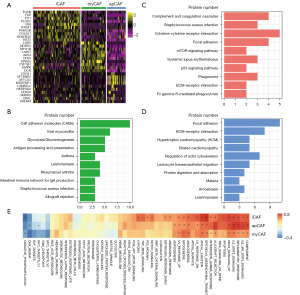
Metabolic heterogeneity of CAFs
The results showed that metabolic-related hallmarks such as hypoxia, OXPHS and adipogenesis correlated with different subtypes of CAF, indicating that other than cytokine secreting, matrix regulating or antigen-presenting, some more significant biological differences, such as metabolism alterations, may be present in the CAF subtypes. We then applied “scMetabolism” to evaluate the CAF metabolic activity at a single-cell resolution and found the 3 subtypes of CAFs were highly different metabolically. iCAF showed the highest activity in carbohydrates, fatty acid, and amino acid metabolism, with the highest score in 47 of 78 metabolic pathways. myCAF had lowest metabolic activity, with the highest score in 13 pathways. apCAF was moderate, with 18 pathways having the highest score (Figure 3A). Notably, iCAF had the highest score for glycolysis, while myCAF had the highest score for the tricarboxylic acid (TCA) cycle and TCA derivatives such as glycine and serine metabolism. myCAF also had the highest score for OXPHOS (Figure 3B). These results suggest metabolic reprogramming from OXPHOS to glycolysis during CAF reshaping, especially towards iCAF. As shown in Figure 3C, the iCAF signature showed the highest correlation with glycolysis, while myCAF and apCAF had much lower R values (R=0.43, 0.084 and 0.2, respectively). One of the main reasons for increased glycolysis is hypoxia in the TME. Further exploration proved the iCAF signature also had the highest correlation with the hypoxia signature (0.81 vs. 0.56 and 0.63). Taken together, local hypoxia in PDAC may reshape fibroblasts towards iCAF subtype, with increased glycolysis.
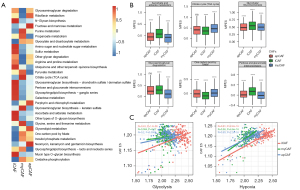
Distinct transcriptional activity and gene regulatory networks of CAF subtypes
TFs and their downstream‐regulated genes constitute a complex and intermingled network of gene regulation that determines the cell-to-cell variability in cancer. We applied “SCENIC” analysis to infer the activity of each TF and its regulated genes (which is the regulon) in the CAF subtypes. SCENIC (Figure 4A) showed a similar clustering of CAFs as with “Seurat” (Figure 4B). We can assess the difference in regulator activity between CAF subtypes using the regulator activity scores of TFs. (Figure 4C). By comparing the regulon specificity score (RSS), we examined the crucial regulon of each cell type and visualized the top 5 regulons (Figure 4D). For myCAF, NRF2, CEBPD and YBX1 were the top regulon. Notably, a study has revealed a connection between fibroblast CEBPD and post-chemotherapy angiogenesis (17), and YBX1 has been identified as a target to suppress the myofibroblast phenotype in oral submucous fibrosis (18). For iCAF, we identified CREB3L1, EGR2 and SOX4 as the top regulon, among which CREB3L1 is a well-known hypoxia-induced TF (19). Moreover, SOX4 has been found to be activated by hypoxia signaling in non-small-cell lung cancer cells (20). For apCAF, MAFB, SPL1 and REL were the top regulon. Collectively, these results indicated different TF activity and gene regulatory networks among the CAF subtypes in line with their specific functions.
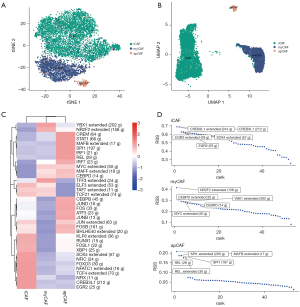
Association of CAF subtypes and different survival prognosis
In order to assess the composition of CAF subtypes in the TCGA-PDAC cohort, we built an SVM-based deconvolution algorithm to analyze the cell component of a mixture using the Seurat object, Seurat-derived cell markers and FPKM-normalized RNA-seq data as input. We tested the algorithm using a pseudo pooled RNA-seq data comprising 1,000 cells withdrawn from 24 tumor tissue samples, which showed a high consistency with scRNA data (Figure 5A). For fibroblasts, the Pearson correlation R value was 0.93 respectively (Figure 5B).
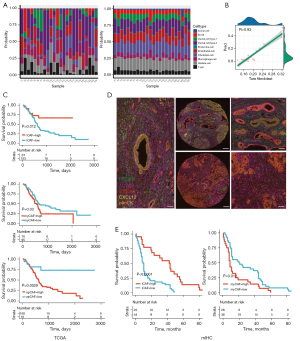
We assessed the prognostic difference between high or low abundance groups. Interestingly, the higher iCAF was related to a better prognosis, whereas higher myCAF was related to a worse one (log-rank P=0.003 and 0.021). apCAF seemed to have no prognostic impact (P=0.18) (Figure 5C). We then validated the prognostic impact of iCAF and myCAF in an independent cohort using multiplex immunohistochemistry (mIHC). The cohort included 66 patients with PDAC, as an external validation group, from the Shanghai General Hospital and Minhang Hospital between 2016 and 2021. Four widely recognized molecules were selected as biomarkers to distinguish iCAF from myCAF: PDGFRα and CXCL12 for iCAF, and S100A4 and a-SMA for myCAF. In addition, we used pan-CK as a marker for PDAC to identify cancerous and non-cancerous tissues (Figure 5D) (21). The mIHC results demonstrated that patients with a higher number of iCAF infiltrations had a longer OS with statistical difference (P<0.05), which was consistent with the results of our preceding analysis (Figure 5E). We also compared the clinical features, such as tumor size, TNM stage, CA125 and CA199 levels, between iCAF-high and iCAF-low, or myCAF-high and myCAF-low subgroups in the external validation group. We found that iCAF was related to a lower TNM stage (P=0.0367) (Tables 1,2). Collectively, we discovered and validated the diverse prognostic impact of iCAF and myCAF in PDAC patients.
Table 1
Clinical features | iCAF-high, mean ± SD | iCAF-low, mean ± SD | P/χ2 |
---|---|---|---|
Age, years | 60.9±10.3 | 61.3±8.7 | P=0.875008 |
Size, cm | 2.9±1.1 | 3.5±1.3 | P=0.071009 |
Weight, kg | 66.4±9.1 | 63.3±10.52 | P=0.262188 |
Gender, n | χ2=0.000514 | ||
Male | 14 | 28 | |
Female | 7 | 16 | |
TNM stage, n | χ2=0.00367 | ||
1a | 3 | 4 | |
1b | 6 | 19 | |
2a | 3 | 8 | |
2b | 9 | 13 | |
CA125, U/mL | 23.5±16.9 | 37.4±43.1 | P=0.164621 |
CA199, U/mL | 1,108.7±4,476.2 | 233.5±477 | P=0.211595 |
iCAFs, inflammatory cancer-associated fibroblasts; PDAC, pancreatic ductal adenocarcinoma; TNM, tumor node metastasis.
Table 2
Clinical features | myCAF-high, mean ± SD | myCAF-low, mean ± SD | P/χ2 |
---|---|---|---|
Age, years | 60.8±9.5 | 61.3±9.0 | P=0.782 |
Size, cm | 3±1.1 | 3.5±1.4 | P=0.112 |
Weight, kg | 64.7±9.4 | 64±10.7 | P=0.78 |
Gender, n | χ2=0.04653 | ||
Male | 19 | 23 | |
Female | 8 | 15 | |
TNM stage, n | χ2=0.069276 | ||
1a | 4 | 3 | |
1b | 8 | 17 | |
2a | 6 | 5 | |
2b | 9 | 14 | |
CA125, U/mL | 29.6±35.6 | 35.5±38.3 | P=0.553 |
CA199, U/mL | 192.2±425.5 | 743.6±3371.9 | P=0.412 |
myCAF, myofibroblast cancer-associated fibroblast; PDAC, pancreatic ductal adenocarcinoma; TNM, tumor node metastasis.
Association of higher abundance of iCAF with an inflammatory phenotype
Preceding findings suggested that the iCAF signature in PDAC is associated with more active metabolism. We further investigated how iCAFs influence cancer biology in PDAC. PDAC samples were divided into 2 groups based on the abundance of iCAFs (low or high). PCA plot showed a difference between the 2 groups (Figure 6A). Gene analysis of the 2 groups was performed, showing the ~6,000 genes were differently expressed between groups. KEGG annotation of the upregulated genes showed that for the iCAF-high group, inflammatory pathways, such as the JAK-STAT and PI3K-AKT signaling pathways, or metabolic pathways, such as the Wnt and PPAR signal pathways, were enriched (Figure 6B). For the iCAF-low group, bile secretion and ECM-receptor interaction were enriched (Figure 6C). We evaluated the metabolic activity in the iCAF-high or low groups using a ssGSEA algorithm, which, in line with the previous finding (16), showed higher metabolic activity, including lipid metabolism and glucose metabolism, was enriched in the iCAF-high group (Figure 6D, left). Moreover, we compared the immune cell specific chemotaxis genes between groups, and annotated them by the immune cell they attracted. Interestingly, the iCAF-high group was more attractive to immune cells, such as leukocytes and monocytes (Figure 6D, right). The tumor inflammation signature (TIS) is a widely applied gene signature to evaluate inflammatory state based on antigen-presented cell abundance, T/natural killer cell abundance, interferon activity and T cell exhaustion (22). Higher TIS score is related to benefit from PD1 treatment. We found a significantly higher expression of TIS signature genes in the iCAF-high group compared with the iCAF-low group (Figure 6E). Taken together, these findings suggested that higher iCAF abundance in PDAC is related to more reprogramming of metabolism and higher inflammatory state, and may predict a better response to immune therapy.
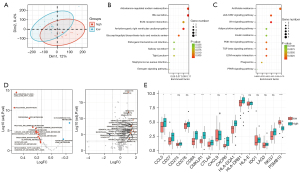
Discussion
PDAC patients are often diagnosed at a late stage, when curative surgical approaches are not feasible. Rarely do patients benefit from chemotherapy, due to the mesenchymal-rich character of PDAC. The existence of heterogeneous and distinct subgroups of CAFs has been widely recognized and traditionally divided into myCAFs and iCAFs, but recently a new subgroup, apCAF with antigen-presenting function, has been reported, enriching our understanding of CAFs (12). Moreover, a study based on single-cell sequencing showed that CAFs were categorized as CD105+ or CD105− CAFs; CD105+ pancreatic CAFs were permissive for tumor development in vivo, whereas CD105− fibroblasts were strongly tumor suppressive, providing a novel perspective on CAF types (23).
Previously, it has been considered that abundant CAFs and deposited collagen affected the perfusion of blood vessels through compression, preventing drugs and immune cells from entering the tumor and limiting the therapeutic effect (16). However, elimination of CAFs and the ECM does not prolong patient survival, which reflects the complexity and plasticity of CAFs. In this study, we found 3 spatially and functionally distinct CAF populations in human and murine PDAC and also plot the metabolism profile of CAF, indicated a potential association between metabolic rewiring and CAF subgroups. We introduced a novel algorism, scFrac, allowing calculation of enrichment score of all subgroups of CAFs in bulk RNAseq of PDAC, providing convenience for exploring how CAF subgroups dysregulate cancer biology. Interestingly, higher iCAF related to a better prognosis, but higher myCAF related to a worse one. A recent study showed that myCAF composition was a poor prognostic factor in 9 cancer types, including PDAC (24). Another single-cell sequencing study based on melanoma, head and neck squamous cell carcinoma and lung cancer revealed iCAFs were potentially associated with a molecular microenvironment of enhanced immune cell activation, whereas myCAFs were associated with a molecular microenvironment that favored ECM remodeling (25). When we cannot obliterate CAFs, perhaps reshaping and altering their phenotype, that is, turning foes into pals, would be a promising endeavor. Thus, it is crucial to clarify their features and functions as well as the principles of their mutual transformation.
Although there is mounting evidence of interconversion among distinct CAF subtypes, the mechanisms by which the CAF composition varies during cancer development and which CAF subtype is associated with prognosis and therapeutic sensitivity remain unknown. In comparison to normal pancreatic fibroblasts, human PDAC CAFs exhibited low levels of lipid storage genes such as fatty acid-binding proteins, but human and mouse CAFs showed high levels of the vitamin D receptor protein (26). In vitro treatment of human CAFs with the strong vitamin D analog calcipotriol resulted in the production of lipid droplets and a reduction in α-SMA expression in the majority of samples. CAFs isolated from murine PDAC may be shifted between the α-SMA-high and IL-6-producing states through TGF-β and IL-1 signaling, implying substantial fibroblast plasticity (27). Additionally, the fact that fibroblasts and the α-SMA promoter are sensitive to a variety of extracellular stimuli, including substrate stiffness, supports the notion that the α-SMA-high, ECM-producing-high state is reversible (28-32). Another research discovered that inhibiting the hedgehog pathway decreased myCAF and raised iCAF numbers, which coincides with a drop in cytotoxic T cells and an increase in regulatory T cells, associated with greater immunological suppression (33). Overall, these results suggest the potential for interconversion of the subtypes of CAFs. Our results revealed that CAFs were metabolically heterogenic, notably iCAF trending in glycolysis, while myCAF trended in TCA cycle and TCA derivatives such as glycine and serine metabolism. Targeting and reprogramming these metabolic pathways may provide novel therapeutic approaches for better clinical outcomes.
Patient outcomes will be improved through multidisciplinary advancements in imaging, surgery, radiation, and systemic therapies. Although clinical progress has been modest, our understanding of the molecular biology of PDAC and the TME continues to expand and will eventually inform rational therapeutic approaches that will result in clinical benefit.
Acknowledgments
We sincerely thank Dr. Xiaotian Shen (Huashan Hospital, Fudan University) for his assistance in downloading data and providing advice on manuscript preparation and submission.
Funding: This work was supported by the National Key Research and Development Program of China (No. 2017YFC1308604), the International Science and Technology Cooperation Project of Shanghai (No. 21490713700), the National Natural Science Foundation of China (No. 81871940), the Shanghai Natural Science Foundation (No. 21ZR1451600), the Medical Engineering Project of Fudan University (No. yg2021-017), and the “Fuqing Scholar” Student Scientific Research Program of Shanghai Medical College (FQXZ202115B).
Footnote
Reporting Checklist: The authors have completed the REMARK reporting checklist. Available at https://atm.amegroups.com/article/view/10.21037/atm-22-407/rc
Data Sharing Statement: Available at https://atm.amegroups.com/article/view/10.21037/atm-22-407/dss
Conflicts of Interest: All authors have completed the ICMJE uniform disclosure form (available at https://atm.amegroups.com/article/view/10.21037/atm-22-407/coif). The authors have no conflicts of interest to declare.
Ethical Statement: The authors are accountable for all aspects of the work in ensuring that questions related to the accuracy or integrity of any part of the work are appropriately investigated and resolved. All procedures performed in this study involving human participants were in accordance with the Declaration of Helsinki (as revised in 2013). All clinical samples were collected with informed consent from the patients, and the research was approved by the ethics committees of Shanghai General Hospital, Shanghai Jiao Tong University School of Medicine (China) (No. 2021-130) and Minhang Hospital, Fudan University (China) (No. 2021-066-01K).
Open Access Statement: This is an Open Access article distributed in accordance with the Creative Commons Attribution-NonCommercial-NoDerivs 4.0 International License (CC BY-NC-ND 4.0), which permits the non-commercial replication and distribution of the article with the strict proviso that no changes or edits are made and the original work is properly cited (including links to both the formal publication through the relevant DOI and the license). See: https://creativecommons.org/licenses/by-nc-nd/4.0/.
References
- Siegel RL, Miller KD, Jemal A. Cancer statistics, 2016. CA Cancer J Clin 2016;66:7-30. [Crossref] [PubMed]
- Schnittert J, Bansal R, Prakash J. Targeting Pancreatic Stellate Cells in Cancer. Trends Cancer 2019;5:128-42. [Crossref] [PubMed]
- Wang S, Zheng Y, Yang F, et al. The molecular biology of pancreatic adenocarcinoma: translational challenges and clinical perspectives. Signal Transduct Target Ther 2021;6:249. [Crossref] [PubMed]
- Paszek MJ, Zahir N, Johnson KR, et al. Tensional homeostasis and the malignant phenotype. Cancer Cell 2005;8:241-54. [Crossref] [PubMed]
- DuFort CC, DelGiorno KE, Hingorani SR. Mounting Pressure in the Microenvironment: Fluids, Solids, and Cells in Pancreatic Ductal Adenocarcinoma. Gastroenterology 2016;150:1545-1557.e2. [Crossref] [PubMed]
- Mohammadi H, Sahai E. Mechanisms and impact of altered tumour mechanics. Nat Cell Biol 2018;20:766-74. [Crossref] [PubMed]
- Provenzano PP, Cuevas C, Chang AE, et al. Enzymatic targeting of the stroma ablates physical barriers to treatment of pancreatic ductal adenocarcinoma. Cancer Cell 2012;21:418-29. [Crossref] [PubMed]
- Sahai E, Astsaturov I, Cukierman E, et al. A framework for advancing our understanding of cancer-associated fibroblasts. Nat Rev Cancer 2020;20:174-86. [Crossref] [PubMed]
- Özdemir BC, Pentcheva-Hoang T, Carstens JL, et al. Depletion of carcinoma-associated fibroblasts and fibrosis induces immunosuppression and accelerates pancreas cancer with reduced survival. Cancer Cell 2014;25:719-34. [Crossref] [PubMed]
- Rhim AD, Oberstein PE, Thomas DH, et al. Stromal elements act to restrain, rather than support, pancreatic ductal adenocarcinoma. Cancer Cell 2014;25:735-47. [Crossref] [PubMed]
- Öhlund D, Handly-Santana A, Biffi G, et al. Distinct populations of inflammatory fibroblasts and myofibroblasts in pancreatic cancer. J Exp Med 2017;214:579-96. [Crossref] [PubMed]
- Elyada E, Bolisetty M, Laise P, et al. Cross-Species Single-Cell Analysis of Pancreatic Ductal Adenocarcinoma Reveals Antigen-Presenting Cancer-Associated Fibroblasts. Cancer Discov 2019;9:1102-23. [Crossref] [PubMed]
- Chen Z, Quan L, Huang A, et al. seq-ImmuCC: Cell-Centric View of Tissue Transcriptome Measuring Cellular Compositions of Immune Microenvironment From Mouse RNA-Seq Data. Front Immunol 2018;9:1286. [Crossref] [PubMed]
- Newman AM, Steen CB, Liu CL, et al. Determining cell type abundance and expression from bulk tissues with digital cytometry. Nat Biotechnol 2019;37:773-82. [Crossref] [PubMed]
- Peng J, Sun BF, Chen CY, et al. Single-cell RNA-seq highlights intra-tumoral heterogeneity and malignant progression in pancreatic ductal adenocarcinoma. Cell Res 2019;29:725-38. [Crossref] [PubMed]
- Wang Y, Liang Y, Xu H, et al. Single-cell analysis of pancreatic ductal adenocarcinoma identifies a novel fibroblast subtype associated with poor prognosis but better immunotherapy response. Cell Discov 2021;7:36. [Crossref] [PubMed]
- Chi JY, Hsiao YW, Liu HL, et al. Fibroblast CEBPD/SDF4 axis in response to chemotherapy-induced angiogenesis through CXCR4. Cell Death Discov 2021;7:94. [Crossref] [PubMed]
- Yu CH, Fang CY, Yu CC, et al. LINC00312/YBX1 Axis Regulates Myofibroblast Activities in Oral Submucous Fibrosis. Int J Mol Sci 2020;21:2979. [Crossref] [PubMed]
- Mellor P, Deibert L, Calvert B, et al. CREB3L1 is a metastasis suppressor that represses expression of genes regulating metastasis, invasion, and angiogenesis. Mol Cell Biol 2013;33:4985-95. [Crossref] [PubMed]
- Sun J, Xiong Y, Jiang K, et al. Hypoxia-sensitive long noncoding RNA CASC15 promotes lung tumorigenesis by regulating the SOX4/β-catenin axis. J Exp Clin Cancer Res 2021;40:12. [Crossref] [PubMed]
- Hosein AN, Brekken RA, Maitra A. Pancreatic cancer stroma: an update on therapeutic targeting strategies. Nat Rev Gastroenterol Hepatol 2020;17:487-505. [Crossref] [PubMed]
- Danaher P, Warren S, Lu R, et al. Pan-cancer adaptive immune resistance as defined by the Tumor Inflammation Signature (TIS): results from The Cancer Genome Atlas (TCGA). J Immunother Cancer 2018;6:63. [Crossref] [PubMed]
- Hutton C, Heider F, Blanco-Gomez A, et al. Single-cell analysis defines a pancreatic fibroblast lineage that supports anti-tumor immunity. Cancer Cell 2021;39:1227-44.e20. [Crossref] [PubMed]
- Li B, Pei G, Yao J, et al. Cell-type deconvolution analysis identifies cancer-associated myofibroblast component as a poor prognostic factor in multiple cancer types. Oncogene 2021;40:4686-94. [Crossref] [PubMed]
- Galbo PM Jr, Zang X, Zheng D. Molecular Features of Cancer-associated Fibroblast Subtypes and their Implication on Cancer Pathogenesis, Prognosis, and Immunotherapy Resistance. Clin Cancer Res 2021;27:2636-47. [Crossref] [PubMed]
- Sherman MH, Yu RT, Engle DD, et al. Vitamin D receptor-mediated stromal reprogramming suppresses pancreatitis and enhances pancreatic cancer therapy. Cell 2014;159:80-93. [Crossref] [PubMed]
- Biffi G, Oni TE, Spielman B, et al. IL1-Induced JAK/STAT Signaling Is Antagonized by TGFβ to Shape CAF Heterogeneity in Pancreatic Ductal Adenocarcinoma. Cancer Discov 2019;9:282-301. [Crossref] [PubMed]
- Malik R, Luong T, Cao X, et al. Rigidity controls human desmoplastic matrix anisotropy to enable pancreatic cancer cell spread via extracellular signal-regulated kinase 2. Matrix Biol 2019;81:50-69. [Crossref] [PubMed]
- Avery D, Govindaraju P, Jacob M, et al. Extracellular matrix directs phenotypic heterogeneity of activated fibroblasts. Matrix Biol 2018;67:90-106. [Crossref] [PubMed]
- Hinz B, Celetta G, Tomasek JJ, et al. Alpha-smooth muscle actin expression upregulates fibroblast contractile activity. Mol Biol Cell 2001;12:2730-41. [Crossref] [PubMed]
- Avgustinova A, Iravani M, Robertson D, et al. Tumour cell-derived Wnt7a recruits and activates fibroblasts to promote tumour aggressiveness. Nat Commun 2016;7:10305. [Crossref] [PubMed]
- Casey TM, Eneman J, Crocker A, et al. Cancer associated fibroblasts stimulated by transforming growth factor beta1 (TGF-beta 1) increase invasion rate of tumor cells: a population study. Breast Cancer Res Treat 2008;110:39-49. [Crossref] [PubMed]
- Steele NG, Biffi G, Kemp SB, et al. Inhibition of Hedgehog Signaling Alters Fibroblast Composition in Pancreatic Cancer. Clin Cancer Res 2021;27:2023-37. [Crossref] [PubMed]
(English Language Editor: K. Brown)