5-methylcytosine RNA methylation regulators affect prognosis and tumor microenvironment in lung adenocarcinoma
Introduction
Lung cancer is one of the leading causes of cancer-related morbidity and mortality worldwide (1). Non-small cell lung carcinoma (NSCLC), accounting for 85% of all lung cancers, mainly comprises lung squamous cell carcinoma (LUSC) and lung adenocarcinoma (LUAD), with LUAD being the most common NSCLC subtype (2). With advances in targeted therapy and immunotherapy (3,4), the 5-year survival rate of patients with NSCLC is still unsatisfactory, at 4–17% (5). Patients with the same clinical characteristics can have distinctly different prognoses because of molecular differences. Therefore, there is an urgent need to confirm new molecular targets to improve the clinical treatment outcome in patients with LUAD.
Methylation of RNA, an important epigenetic modification that includes 5-methylcytosine (m5C), N6-methyladenosine (m6A), N1-methyladenosine (m1A), pseudouridine (Ψ), and inosine (I), has been identified to decorate protein-coding messenger RNAs (mRNAs) and noncoding RNAs (ncRNAs) (6-10). Modifications of RNA play crucial roles in RNA translation, transcription, processing, stability, and splicing (11,12), and m5C is one of the most common RNA modifications (13). The m5C RNA methylation can be catalyzed dynamically by a series of significant mediator proteins known as “writers” [tRNA aspartic acid methyltransferase 1 (TRDMT1), NOP2 nucleolar protein (NSUN1), NOP2/Sun RNA methyltransferase 2 (NSUN2), NSUN3-7], “readers” [Aly/REF export factor (ALYREF) and Y-box binding protein 1 (YBX1)], and “erasers” [tetmethylcytosine dioxygenase 1 (TET1), TET2-3]” (13-16). Dysregulation and disorder of m5C are associated with the occurrence of human diseases, including malignancies (17-19).
In recent years, immune checkpoint blockade (ICB) has made great breakthroughs in clinical efficacy for patients with cancer (20). However, only a small number of patients benefit from ICB (21). Numerous studies have identified that the tumor microenvironment (TME), which contains immune cells (such as T and B lymphocytes, natural killer (NK) cells, macrophages, polymorphonuclear cells, dendritic cells, as well as mast cells) and stromal cells, plays a crucial role in tumor progression, immunotherapy response, and immune escape (22,23). However, the relationship among m5C, immunotherapy response, and the TME in LUAD remains unclear. Therefore, a comprehensive understanding of the effect of m5C regulators on the TME might provide new insights into the immune regulation of the TME.
In this study, we analyzed 14 m5C RNA methylation regulators in LUAD from The Cancer Genome Atlas (TCGA) and Gene Expression Omnibus (GEO) databases. We identified that m5C regulators were closely associated with LUAD prognosis, and then constructed an m5C signature (m5Csig) to predict the LUAD survival and evaluate the response to ICB. Overall, the results indicated that m5Csig could act as a biomarker to predict survival and the response of ICB in LUAD. We present the following article in accordance with the TRIPOD reporting checklist (available at https://atm.amegroups.com/article/view/10.21037/atm-22-500/rc).
Methods
Acquisition of data
The RNA sequencing data (n=594), somatic mutation information (n=561), copy number variation (CNV) information (n=555), and the corresponding clinicopathological features (n=522) of patients with LUAD were downloaded from TCGA (https://portal.gdc.cancer.gov/). To validate the findings in the TCGA database, the validation cohort GSE72094 (n=442) was downloaded from the Gene Expression Omnibus (GEO) database (https://www.ncbi.nlm.nih.gov/geo/). The study was conducted in accordance with the Declaration of Helsinki (as revised in 2013).
Protein-protein interaction analysis
The Search Tool for the Retrieval of Interacting Genes (STRING) database (https://string-db.org/) was used to analyze protein-protein interaction (PPI) information and detect the interactions of 14 m5C regulators. We then extracted PPI pairs with a combined score of 0.4.
Unsupervised clustering for 14 m5C regulators
Unsupervised clustering analysis was used to identify different m5C RNA modification patterns among patients with LUAD (n=535) from TCGA. The 14 m5C regulators included 8 writers (TRDMT1, NSUN1–7), 2 readers (ALYREF and YBX1), and 4 erasers [TET1–3, AlkB homolog 1, histone H2A dioxygenase (ALKBH1)]. The consensus clustering algorithm was employed to categorize patients with LUAD into different modification patterns (24). The consensus ClusterPlus package (https://bioconductor.org/packages/release/bioc/html/ConsensusClusterPlus.html) was used to perform the above steps with a cycle computation of 1,000 iterations to guarantee the stability and reliability of the results (25). The overall survival (OS) rates of patients with the 3 modification patterns were calculated using the Kaplan-Meier method.
Identification of differentially expressed genes between m5C modification patterns
The empirical Bayesian approach in the limma R package (https://bioconductor.org/packages/release/bioc/html/limma.html) was applied to identify differentially expressed genes (DEGs) among the different m5C modification patterns in the standard comparison mode (26). The significance criteria for determining DEGs was set as an adjusted P value <0.001. To identify the potential functions and pathways enriched in the different modification patterns, gene ontology (GO) and Kyoto Encyclopedia of Genes and Genomes (KEGG) analyses were performed based on the DEGs identified among the different modification patterns (27).
Development of the m5C regulators-related prognostic signature
Univariate Cox regression analysis was performed to identify m5C RNA methylation regulators that were associated with the OS of patients with LUAD. A least absolute shrinkage and selection operator (LASSO) Cox regression algorithm was carried out to construct an optimal m5Csig to predict the prognosis of patients with LUAD. Then, the obtained prognosis-associated genes were used to construct the m5Cscore function to calculate the score for each patient. The m5Cscore formula we used as follows:
where m5Cscore is a prognostic risk score for patients with LUAD patients, coefi represents the coefficient, and expri represents the expression of each prognostic gene. According to the median m5Cscore, the patients with LUAD were classified into high- and low-risk groups. The Kaplan-Meier method with the log-rank test was used to evaluate the OS differences between the high- and low-risk groups, and a receiver operating characteristic curve (ROC) was used to evaluate the prediction accuracy of m5Csig. Univariate and multivariate Cox regression analyses were used to explore prognostic values of m5Csig and clinical characteristics.
Estimation of TME cell infiltration
According to the method used by Newman et al. (28), 22 types of tumor-infiltrating immune cells (TIICs) between the high- and low-risk groups were estimated using the Cell Type Identification By Estimating Relative Subsets Of RNA Transcripts (CIBERSORT) algorithm.
Building and validation of a nomogram
Clinical factors [age, gender, stage and tumor-node-metastasis (TNM) stage] and the m5Cscore were used to develop a prognostic nomogram to evaluate the probability of 1-, 2-, and 3-year OS for patients with LUAD (29). The C-index and a calibration plot were constructed to estimate the accuracy and consistency of the m5Cscore.
Statistical analysis
Spearman and distance correlation analyses were used to compute the correlation coefficients among the expression levels of m5C regulators. The Wilcoxon test was used to analyze the difference between 2 groups, and the Kruskal–Wallis test and one-way analysis of variance (ANOVA) were used among 3 or more groups. LASSO Cox regression and Kaplan-Meier analyses were performed to construct and evaluate the m5Cscore. The area under the receiver operating characteristic curve (AUC) was used to investigate the time-dependent prognostic value of the m5Cscore. Multivariate Cox regression and stratified analysis were used to verify the independence of the m5Cscore from other clinical factors. Statistical significance was set at P<0.05, and all statistical P values were 2-sided. All data were processed using R 4.0.3 software (R Foundation for Statistical Computing, Vienna, Austria).
Results
Landscape of genetic variation among m5C regulators in LUAD
The workflow of our study is shown in Figure S1. A total of 14 m5C regulators including 8 writers (TRDMT1, NSUN1–7), 2 readers (ALYREF and YBX1), and 4 erasers (TET1–3, ALKBH1) were included in our study (Table S1). First, the frequency of CNVs and somatic mutations of the 14 m5C regulators were investigated in LUAD. The CNV alteration frequency indicated that CNV alterations were ubiquitous among the 14 m5C regulators. As shown in Figure 1A and Table S2, NSUN2 (13.69% amplification vs. 1.80% deletion), ALYREF (10.81% amplification vs. 1.44% deletion), YBX1 (7.39% amplification vs. 1.80% deletion), and NSUN4 (6.13% amplification vs. 2.52% deletion) were associated with amplification of the copy number, while ALYBH1 (4.86% deletion vs. 1.80% amplification) and NSUN1 (5.95% deletion vs. 4.32% amplification) were frequently deleted. The distribution of CNV alteration of m5C regulators on chromosomes is shown in Figure 1B. The analysis showed that 13.19% of patients with LUAD (n=74) experienced mutations of m5C regulators. The highest mutation frequency was exhibited by TET1 (4%) followed by TET2 (2%) and TET3 (2%), while the genes including the writers (NSUN1, NSUN3–5 and NSUN7), readers (ALYREF and YBX1) had no mutations in the patients with LUAD (Figure 1C). To explore the relationship between CNV alteration and the expression of m5C regulators, we analyzed the mRNA expression levels of the regulators. The results indicated that the expression levels of NSUN1, NSUN2, NSUN4–7, ALKBH1, TET1, TET3, and ALYREF were significantly upregulated in LUAD (P<0.001), whereas the expression level of TRDMT1 was significantly downregulated in LUAD (P<0.001). No significant difference was found in the expression levels of TET2, YBX1, and NSUN3 (Figure 1D,1E). These analyses showed CNV might play a crucial role in the imbalanced expression of m5C regulators, which could affect the occurrence and progression of LUAD. The clinicopathological features of the patients with LUAD are summarized in Table S3.
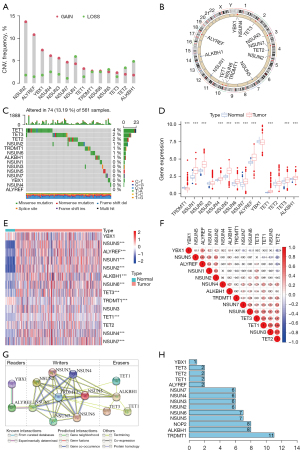
Correlation and interaction between m5C regulators
To determine the crosstalk among m5C regulators, correlation analysis was performed, which showed mainly positive correlations among the m5C regulators; however, several regulators exhibited a negative correlation among the m5C regulators. For example, TET2 and NSUN3 had the strongest positive correlation (r=0.7, P<0.001), whereas the correlation between TET2 and NSUN5 was negative (r=−0.30, P<0.001). Weak correlations were observed between TRDMT1 and other regulators (YBX1, NSUN5, ALYREF, NSUN1, NSUN2, NSUN4, and ALKBH1) (Figure 1F and Table S4). The PPI network analysis indicated that the 14 m5C regulators interacted with each other and TRDMT1 was one of the hub genes (Figure 1G,1H).
Network analyses of m5C regulators
Univariate Cox regression analysis showed the prognostic values of 14 m5C regulators in patients with LUAD, and each regulator had a different prognostic value (Figure 2A). Among the m5C regulators, TRDMT1, NSUN1, NSUN4, NSUN7, and ALYREF were related to the prognosis of patients with LUAD (P<0.05). The interactions, connections, and prognostic values of the m5C regulators are depicted in the m5C regulatory network (Figure 2B). The strongest positive correlation was observed between TET2 and NSUN3 (r=0.70, P<0.001), while the strongest negative correlation was observed between TET2 and NSUN5 (r=−0.30, P<0.001) (Tables S5,S6).
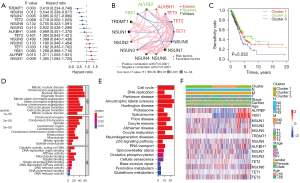
Consensus clustering of m5C regulators identified 3 clusters with different clinical outcomes
To explore whether the expression levels of m5C regulators were associated with prognosis, consensus clustering analysis was applied to classify patients with LUAD in TCGA cohort into subgroups based on their consensus expression of m5C regulators. It was found that K=3 had optimal clustering stability to classify the patients with LUAD into 3 clusters, namely m5C clusters 1–3 (Figure S2A-S2H). Patients in m5C cluster 3 had a significantly poorer OS than patients in cluster 1 and cluster 2 (Figure 2C, P=0.032). Furthermore, to identify enriched functions and pathways among the clusters, GO and KEGG analyses were conducted based on the DEGs identified among the m5C clusters. The results indicated that the DEGs were enriched in various processes, including RNA transport, spliceosome, mitotic nuclear division, and chromosome segregation (Figure 2D,2E). All significant (P<0.05) GO terms and KEGG pathways for the DEGs among 3 clusters are shown in Tables S7,S8. Furthermore, the associations among the 3 clusters, clinicopathological features, and expression levels of the 14 m5C regulators in the TCGA-LUAD cohort were evaluated. As shown in Figure 2F, the expression levels of the m5C regulators were higher in cluster 3, especially for ALYREF and YBX1.
Prognostic analysis of m5Csig in the TCGA-LUAD
As mentioned above, 5 m5C regulators, TRDMT1, NSUN1, NSUN4, NSUN7, and ALYREF, were associated with the prognosis of patients with LUAD according to the results of univariate Cox regression analysis (Figure 3A). The regulators TRDMT1, NSUN4, and NSUN7 act as protective factors, whereas NSUN1 and ALYREF are associated with risk of LUAD. The 5 m5C regulators were incorporated to build m5Csig according to the LASSO Cox regression algorithm. The regression coefficients of the 5 m5C regulatory factors are as follows: TRDMT1, −0.519056; NOP2, 0.166168; NSUN4, −0.376147; NSUN7, −0.246224; ALYREF, 0.163589. The patients with LUAD with complete clinical information (n=500) were classified into a high-risk group (n=250) and a low-risk group (n=250) according to the median m5Cscore, which was used as the cutoff point. As shown in Figure 3B, the expression levels of the risk-associated m5C regulators, NSUN1 and ALYREF, were upregulated in the high-risk group, and those of NSUN4, TRDMT1, and NSUN7 were downregulated in the high-risk group. With the increasing m5Cscore, the number of patients who died increased significantly (Figure 3C,3D). The Kaplan-Meier curve revealed that the patients in the high-risk group had a significantly poorer OS than those in the low-risk group (P=6.578e-05, Figure 3E). Principal component analysis (PCA) analysis showed that the high- and low-risk groups were stratified significantly in 2 different directions, indicating that the patients with LUAD in the high-risk group could be distinguished from those in the low-risk group (Figure 3F). The time-dependent ROC analysis indicated that the AUC values of m5Csig for 1-, 2-, and 3-year OS were 0.637, 0.615, and 0.658, respectively (Figure 3G). Next, univariate and multivariate Cox regression analyses were used to analyze whether the m5Cscore can be used as an independent prognostic factor. Univariate Cox regression analysis showed that T [hazard ratio (HR) =1.579, 95% confidence interval (CI): 1.296–1.923, P<0.001], N (HR =1.706, 95% CI: 1.405–2.072, P<0.001), M (HR =0.037, 95% CI: 1.038–3.272, P=0.037), stage (HR =1.577, 95% CI: 1.348–1.845, P<0.001) and m5Cscore (HR =2.800, 95% CI: 1.700–4.623, P<0.001) were significantly correlated with OS (Figure 3H). However, no significant correlation was found between age and gender and OS. Multivariate Cox regression analysis indicated that only m5Cscore (HR =2.263, 95% CI: 1.342–3.816, P=0.002) can be used as an independent prognostic factor for LUAD (Figure 3I). These results indicated that the m5Cscore has the potential to predict prognosis in LUAD patients.
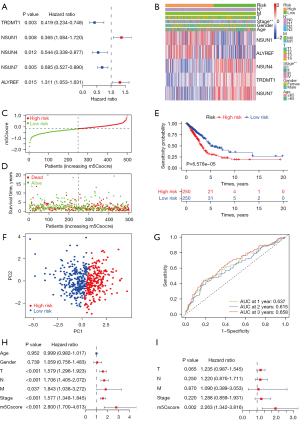
Validation of m5Csig in the GEO database
We validated m5Csig in the GSE72094 cohort. In total, 397 patients with complete clinical information were stratified into the high-risk group (n=198) and the low-risk group (n=199) using the median m5Cscore. As the m5Cscore increased, the number of deaths among the patients increased (Figure 4A,4B). Patients in the low-risk group had a better OS than those in the high-risk group (P=1.58e-03, Figure 4C). The PCA analysis suggested that patients were appropriately classified into high- and low-risk groups (Figure 4D). The ROC curves showed that the AUC values for 1-, 2-, and 3-year OS were 0.651, 0.615, and 0.59 respectively (Figure 4E). Univariate and multivariate Cox regression analyses also demonstrated that the m5Cscore can be used as an independent prognostic factor for LUAD patients (Figure 4F,4G).
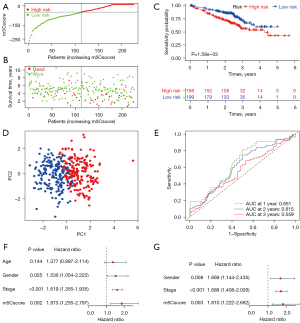
Clinical characteristics between the high- and low-risk groups
A stratification analysis was performed to evaluate whether the m5Cscore could predict survival with the same clinical factor subgroup. Patients were stratified based on clinical parameters, such as age (£65/>65 years), gender (female/male), T (T1+2/T3+4), N (N0/N1–3), M (M0/M1), and stage (I+II/III+IV). The results showed that the m5Cscore could classify patients of the same stratum of age, gender, and early stage (T1–2, N0, M0 and stage I+II) into high- and low-risk groups (P<0.05). Patients in the high-risk group had a poorer OS than those in the low-risk group in each stratum (Figure S3A-S3L). We further analyzed the differences of clinical characteristics between the high- and low-m5Cscore groups and the difference of m5Cscore among different clinical characteristics. No significant distribution difference was found in terms of age (£65/>65 years) (P=0.15, Figure S4A,S4B), gender (female/male) (P=0.37, Figure S4C,S4D), stage I and stage II (P=0.19), stage I and stage III (P=0.33), and stage II and stage III (P=0.87) (Figure S4E,S4F). However, significant clinical differences were observed in terms of stage I and stage IV (P=0.043), stage II and stage IV (P=0.018), stage III and stage IV (P=0.023) (Figure S4E,S4F), ever smoking and never smoking (P=0.046, Figure 5A,5B), EGFR mutation group and EGFR wild group (P=4.2e-05, Figure 5C,5D), KRAS mutation group and KRAS wild group (P=0.0032, Figure 5E,5F), and TP53 mutation group and TP53 wild group (P=0.006, Figure 5G,5H).
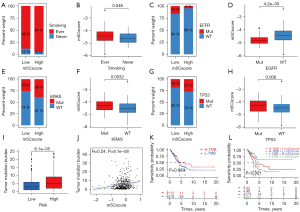
Tumor mutation burden in the high- and low- risk groups in the TCGA-LUAD database
The tumor mutation burden (TMB) quantification analyses indicated that the high-risk group correlated remarkably with a higher TMB (P<0.001, Figure 5I). The m5Cscore and TMB also exhibited a significant positive correlation (R=0.24, P<0.001, Figure 5J). There was no difference in OS between the high- and low-TMB groups (P=0.089, Figure 5K). As shown in Figure 5L, when combined with the m5Cscore, there were significant survival differences among the 4 groups. The high-TMB/low-m5Cscore group had better survival than the high-TMB/high-m5Cscore group, and the low-TMB/high-m5Cscore group had the least favorable OS.
Expression of immune checkpoints and TME cell infiltration characteristics between the high- and low-risk groups in TCGA database
To determine the tumor immune infiltration characteristics, we evaluated the expression of 24 immune checkpoints between the high- and low-risk groups. We found a substantial difference in the expression of 24 immune checkpoints, among which LAG3, PDCD1 (PD-1), TNFRSF4, CD274 (PD-L1), CD276, TNFRSF8, TMIGD2, TNFRSF9, TNFSF4, TNFSF9, KIR3DL1, TNFRSF18, and CD70 were upregulated significantly in the high-risk group (Figure 6A). We further analyzed the proportion of immune cells between the high- and low- risk groups in the TCGA-LUAD database. Heterogeneity of LUAD was indicated by the different ratios of each cell type (Figure 6B). Furthermore, we compared the infiltration of immune cells between the high- and low- risk groups. As shown in Figure 6C, the high-risk group had a higher fraction of CD8 T cells, activated CD4 memory T cells, follicular helper T cells, resting NK cells, and M0 macrophages compared with those in the low-risk group (P<0.05). However, naive B cells, resting CD4 memory T cells, resting dendritic cells, and resting mast cells were markedly downregulated in the high-risk group (P<0.05).
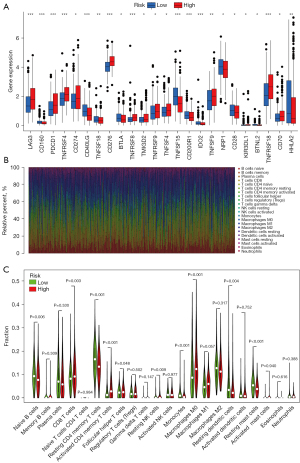
Construction of a prognostic nomogram for LUAD in the TCGA data
To establish a clinically applicable method to evaluate the prognosis of patients with LUAD, we constructed a prognostic nomogram by integrating clinical factors (age, gender, stage) with the m5Cscore (Figure S5A). Using the bootstrap method, calibration plots showed no significant deviation from the ideal for 1-, 3- and 5-year OS (Figure S5B). These results indicated that the prognostic nomogram could be used to predict the prognosis of patients with LUAD.
Discussion
Abnormalities of m5C modifications have been shown to influence RNA stability, gene expression, and protein synthesis, and thus have an essential role in various cellular, biological, and pathological processes (20-32). The RNA m5C modification and its regulators have been shown to be involved in the progression of various cancers, including hepatocellular carcinoma (33), bladder cancer (34), glioblastoma multiforme (35), breast cancer (36), and head and neck carcinoma (37), indicating that RNA m5C might play an important role in tumorigenesis and progression.
However, the biological functions and mechanism by which m5C modifications affect the TME were previously unknown.
In this study, we found that m5C regulators were significantly differently expressed in LUAD. By analyzing the expression profiles of m5C regulators, we identified 3 m5C clusters associated with different prognoses. Moreover, GO and KEGG function analysis indicated that DEGs among the 3 clusters were closely correlated with biological processes and signaling pathways, such as RNA transport, spliceosome, mitotic nuclear division, and chromosome segregation. We also identified 4 writers (TRDMT1, NSUN1, NSUN4, and NSUN7) and 1 eraser (ALYREF) were correlated with the prognosis of LUAD using LASSO Cox regression. Among the 5 m5C regulators, TRDMT1 mainly mediates tRNA stability and regulates cell metabolism of the m5C modification (30,38,39). Loss of TRDMT1 promoted homologous recombination and increased cellular sensitivity to DNA double-strand breaks (40). The NSUN1 protein (also known as NOP2) is a nucleolar-specific protein that plays a crucial role in RNA modification (41), cell cycle progression (41), chromatin organization (42), and HIV-1 latency (43). NSUN4, which forms a complex with MTERF4, is not essential in mitochondrial ribosome biogenesis and mitochondrial translation termination in conditional Nsun4 mouse knockout mutants (44,45). High expression of NSUN7 has been associated with shorter survival in low-grade gliomas (46), and a deletion mutation of NSUN7 has been associated with reduced sperm motility in asthenospermic men (47). The mRNA export adaptor, ALYREF, serves as a specific m5C-binding protein and functions in promoting mRNA export (48,49). An ALYREF-MYCN coactivator complex might be involved in neuroblastoma tumorigenesis (50).
An m5Csig was constructed, which divided patients with LUAD into high- and low-risk groups. Patients in the high-risk group had a significantly poorer OS than those in the low-risk group. Univariate and multivariate Cox regression analyses demonstrated that the m5Cscore was an independent prognostic factor for patients with LUAD. Accumulated evidence has demonstrated that patients overexpressing PD-1/PD-L1 and with a high TMB status are associated with an improved and durable ICB response (51-53). The TMB quantification analyses indicated that the high-risk group correlated markedly with a higher TMB. The m5Cscore and TMB also exhibited a significant positive correlation. The high-risk group displayed significantly higher expression levels of PD-1 and PD-L1 than the low-risk group. The above results demonstrated that LUAD with a high m5Cscore might show a better response to ICB therapy.
Accumulating evidence suggested that m5C is closely related to TME. Schoeler et al. demonstrated that TET enzymes control antibody production and shape the mutational landscape in germinal centre B cells. TET2 and TET3 guide the transition of germinal centre B cells to antibody-secreting plasma cells (54). Yue et al. revealed Tet2/3-deficiency in Treg cells leads to T cell activation and results in an activated phenotype and dysregulated expression of multiple Treg activation and phenotypic molecules in healthy mice (55). In our study, the CIBERSORT results showed that the high-risk group had stronger immune cell invasion compared with that of the low-risk group, for example, the numbers of CD8 T cells and activated CD4 memory T cells were significantly increased. These results suggested that the m5C regulators might be involved in the progression and prognosis of LUAD by modulating TIIC infiltration of the TME. Targeting m5C-related regulators might provide a novel way to improve the efficiency of ICB in LUAD.
However, there were several limitations to our study. First, this was a bioinformatic study based on a public database; therefore, further in vivo and in vitro experimental studies are needed to explore the potential effect and mechanism of m5C regulators in LUAD. Second, more potential m5C regulators have yet to be discovered. Last, the regulatory mechanism of m5C regulators in the TME was not determined, which requires further investigation to provide a better understanding.
Conclusions
In summary, we comprehensively analyzed the relationship between m5C methylation regulators and TME immune modulation. Based on the characteristics of m5C regulators, m5Csig was constructed to predict the prognosis of patients with LUAD, which might provide novel strategies for ICB therapy.
Acknowledgments
Funding: This study was supported by grants from the Science and Technology Tackling Program of Guangzhou for People’s Livelihood (No. 201903010003), the National Natural Science Foundation of China (No. 82003212), the Discipline Construction Project of Guangzhou Medical University During the 14th Five-Year Plan (No. 06-410-2107181), the Guangzhou Key Medical Discipline Construction Project Fund (No. 02-412-B205002-1004042), and the Medical and Health Technology Projects of Guangzhou, China (No. 2015A011086). The funding bodies played no role in the design of the study and collection, analysis, and interpretation of data and in writing the manuscript.
Footnote
Reporting Checklist: The authors have completed the TRIPOD reporting checklist. Available at https://atm.amegroups.com/article/view/10.21037/atm-22-500/rc
Conflicts of Interest: All authors have completed the ICMJE uniform disclosure form (available at https://atm.amegroups.com/article/view/10.21037/atm-22-500/coif). The authors have no conflicts of interest to declare.
Ethical Statement: The authors are accountable for all aspects of the work in ensuring that questions related to the accuracy or integrity of any part of the work are appropriately investigated and resolved. The study was conducted in accordance with the Declaration of Helsinki (as revised in 2013).
Open Access Statement: This is an Open Access article distributed in accordance with the Creative Commons Attribution-NonCommercial-NoDerivs 4.0 International License (CC BY-NC-ND 4.0), which permits the non-commercial replication and distribution of the article with the strict proviso that no changes or edits are made and the original work is properly cited (including links to both the formal publication through the relevant DOI and the license). See: https://creativecommons.org/licenses/by-nc-nd/4.0/.
References
- Sung H, Ferlay J, Siegel RL, et al. Global Cancer Statistics 2020: GLOBOCAN Estimates of Incidence and Mortality Worldwide for 36 Cancers in 185 Countries. CA Cancer J Clin 2021;71:209-49. [Crossref] [PubMed]
- Chen Z, Fillmore CM, Hammerman PS, et al. Non-small-cell lung cancers: a heterogeneous set of diseases. Nat Rev Cancer 2014;14:535-46. [Crossref] [PubMed]
- Peters S, Reck M, Smit EF, et al. How to make the best use of immunotherapy as first-line treatment of advanced/metastatic non-small-cell lung cancer. Ann Oncol 2019;30:884-96. [Crossref] [PubMed]
- Hirsch FR, Suda K, Wiens J, et al. New and emerging targeted treatments in advanced non-small-cell lung cancer. Lancet 2016;388:1012-24. [Crossref] [PubMed]
- Hirsch FR, Scagliotti GV, Mulshine JL, et al. Lung cancer: current therapies and new targeted treatments. Lancet 2017;389:299-311. [Crossref] [PubMed]
- Roundtree IA, Evans ME, Pan T, et al. Dynamic RNA Modifications in Gene Expression Regulation. Cell 2017;169:1187-200. [Crossref] [PubMed]
- Carlile TM, Rojas-Duran MF, Zinshteyn B, et al. Pseudouridine profiling reveals regulated mRNA pseudouridylation in yeast and human cells. Nature 2014;515:143-6. [Crossref] [PubMed]
- Boccaletto P, Machnicka MA, Purta E, et al. MODOMICS: a database of RNA modification pathways. 2017 update. Nucleic Acids Res 2018;46:D303-7. [Crossref] [PubMed]
- Li X, Xiong X, Yi C. Epitranscriptome sequencing technologies: decoding RNA modifications. Nat Methods 2016;14:23-31. [Crossref] [PubMed]
- Gilbert WV, Bell TA, Schaening C. Messenger RNA modifications: Form, distribution, and function. Science 2016;352:1408-12. [Crossref] [PubMed]
- Oerum S, Dégut C, Barraud P, et al. m1A Post-Transcriptional Modification in tRNAs. Biomolecules 2017;7:20. [Crossref] [PubMed]
- Liu J, Jia G. Methylation modifications in eukaryotic messenger RNA. J Genet Genomics 2014;41:21-33. [Crossref] [PubMed]
- Hussain S, Aleksic J, Blanco S, et al. Characterizing 5-methylcytosine in the mammalian epitranscriptome. Genome Biol 2013;14:215. [Crossref] [PubMed]
- Squires JE, Patel HR, Nousch M, et al. Widespread occurrence of 5-methylcytosine in human coding and non-coding RNA. Nucleic Acids Res 2012;40:5023-33. [Crossref] [PubMed]
- Khoddami V, Cairns BR. Identification of direct targets and modified bases of RNA cytosine methyltransferases. Nat Biotechnol 2013;31:458-64. [Crossref] [PubMed]
- Nombela P, Miguel-López B, Blanco S. The role of m6A, m5C and Ψ RNA modifications in cancer: Novel therapeutic opportunities. Mol Cancer 2021;20:18. [Crossref] [PubMed]
- Jonkhout N, Tran J, Smith MA, et al. The RNA modification landscape in human disease. RNA 2017;23:1754-69. [Crossref] [PubMed]
- Xue C, Zhao Y, Li L. Advances in RNA cytosine-5 methylation: detection, regulatory mechanisms, biological functions and links to cancer. Biomark Res 2020;8:43. [Crossref] [PubMed]
- Pan J, Huang Z, Xu Y. m5C RNA Methylation Regulators Predict Prognosis and Regulate the Immune Microenvironment in Lung Squamous Cell Carcinoma. Front Oncol 2021;11:657466. [Crossref] [PubMed]
- Garon EB, Rizvi NA, Hui R, et al. Pembrolizumab for the treatment of non-small-cell lung cancer. N Engl J Med 2015;372:2018-28. [Crossref] [PubMed]
- Topalian SL, Hodi FS, Brahmer JR, et al. Safety, activity, and immune correlates of anti-PD-1 antibody in cancer. N Engl J Med 2012;366:2443-54. [Crossref] [PubMed]
- Fridman WH, Zitvogel L, Sautès-Fridman C, et al. The immune contexture in cancer prognosis and treatment. Nat Rev Clin Oncol 2017;14:717-34. [Crossref] [PubMed]
- Dunn GP, Old LJ, Schreiber RD. The three Es of cancer immunoediting. Annu Rev Immunol 2004;22:329-60. [Crossref] [PubMed]
- Hartigan JA, Wong MA. Algorithm AS 136: A K-Means Clustering Algorithm. Journal of the Royal Statistical Society 1979;28:100-8.
- Wilkerson MD, Hayes DN. ConsensusClusterPlus: a class discovery tool with confidence assessments and item tracking. Bioinformatics 2010;26:1572-3. [Crossref] [PubMed]
- Ritchie ME, Phipson B, Wu D, et al. limma powers differential expression analyses for RNA-sequencing and microarray studies. Nucleic Acids Res 2015;43:e47. [Crossref] [PubMed]
- Yu G, Wang LG, Han Y, et al. clusterProfiler: an R package for comparing biological themes among gene clusters. OMICS 2012;16:284-7. [Crossref] [PubMed]
- Newman AM, Liu CL, Green MR, et al. Robust enumeration of cell subsets from tissue expression profiles. Nat Methods 2015;12:453-7. [Crossref] [PubMed]
- Iasonos A, Schrag D, Raj GV, et al. How to build and interpret a nomogram for cancer prognosis. J Clin Oncol 2008;26:1364-70. [Crossref] [PubMed]
- David R, Burgess A, Parker B, et al. Transcriptome-Wide Mapping of RNA 5-Methylcytosine in Arabidopsis mRNAs and Noncoding RNAs. Plant Cell 2017;29:445-60. [Crossref] [PubMed]
- King MY, Redman KL. RNA methyltransferases utilize two cysteine residues in the formation of 5-methylcytosine. Biochemistry 2002;41:11218-25. [Crossref] [PubMed]
- Cheng JX, Chen L, Li Y, et al. RNA cytosine methylation and methyltransferases mediate chromatin organization and 5-azacytidine response and resistance in leukaemia. Nat Commun 2018;9:1163. [Crossref] [PubMed]
- He Y, Yu X, Li J, et al. Role of m5C-related regulatory genes in the diagnosis and prognosis of hepatocellular carcinoma. Am J Transl Res 2020;12:912-22. [PubMed]
- Chen X, Li A, Sun BF, et al. 5-methylcytosine promotes pathogenesis of bladder cancer through stabilizing mRNAs. Nat Cell Biol 2019;21:978-90. [Crossref] [PubMed]
- Cheray M, Etcheverry A, Jacques C, et al. Cytosine methylation of mature microRNAs inhibits their functions and is associated with poor prognosis in glioblastoma multiforme. Mol Cancer 2020;19:36. [Crossref] [PubMed]
- Huang Z, Pan J, Wang H, et al. Prognostic Significance and Tumor Immune Microenvironment Heterogenicity of m5C RNA Methylation Regulators in Triple-Negative Breast Cancer. Front Cell Dev Biol 2021;9:657547. [Crossref] [PubMed]
- Xue M, Shi Q, Zheng L, et al. Gene signatures of m5C regulators may predict prognoses of patients with head and neck squamous cell carcinoma. Am J Transl Res 2020;12:6841-52. [PubMed]
- Tuorto F, Liebers R, Musch T, et al. RNA cytosine methylation by Dnmt2 and NSun2 promotes tRNA stability and protein synthesis. Nat Struct Mol Biol 2012;19:900-5. [Crossref] [PubMed]
- Huang T, Chen W, Liu J, et al. Genome-wide identification of mRNA 5-methylcytosine in mammals. Nat Struct Mol Biol 2019;26:380-8. [Crossref] [PubMed]
- Chen H, Yang H, Zhu X, et al. m5C modification of mRNA serves a DNA damage code to promote homologous recombination. Nat Commun 2020;11:2834. [Crossref] [PubMed]
- Kosi N, Alić I, Kolačević M, et al. Nop2 is expressed during proliferation of neural stem cells and in adult mouse and human brain. Brain Res 2015;1597:65-76. [Crossref] [PubMed]
- Hong J, Lee JH, Chung IK. Telomerase activates transcription of cyclin D1 gene through an interaction with NOL1. J Cell Sci 2016;129:1566-79. [PubMed]
- Kong W, Biswas A, Zhou D, et al. Nucleolar protein NOP2/NSUN1 suppresses HIV-1 transcription and promotes viral latency by competing with Tat for TAR binding and methylation. PLoS Pathog 2020;16:e1008430. [Crossref] [PubMed]
- Metodiev MD, Spåhr H, Loguercio Polosa P, et al. NSUN4 is a dual function mitochondrial protein required for both methylation of 12S rRNA and coordination of mitoribosomal assembly. PLoS Genet 2014;10:e1004110. [Crossref] [PubMed]
- Cámara Y, Asin-Cayuela J, Park CB, et al. MTERF4 regulates translation by targeting the methyltransferase NSUN4 to the mammalian mitochondrial ribosome. Cell Metab 2011;13:527-39. [Crossref] [PubMed]
- Sato K, Tahata K, Akimoto K. Five Genes Associated With Survival in Patients With Lower-grade Gliomas Were Identified by Information-theoretical Analysis. Anticancer Res 2020;40:2777-85. [Crossref] [PubMed]
- Khosronezhad N, Hosseinzadeh Colagar A, Mortazavi SM. The Nsun7 (A11337)-deletion mutation, causes reduction of its protein rate and associated with sperm motility defect in infertile men. J Assist Reprod Genet 2015;32:807-15. [Crossref] [PubMed]
- Zhou Z, Luo MJ, Straesser K, et al. The protein Aly links pre-messenger-RNA splicing to nuclear export in metazoans. Nature 2000;407:401-5. [Crossref] [PubMed]
- Yang X, Yang Y, Sun BF, et al. 5-methylcytosine promotes mRNA export - NSUN2 as the methyltransferase and ALYREF as an m5C reader. Cell Res 2017;27:606-25. [Crossref] [PubMed]
- Nagy Z, Seneviratne JA, Kanikevich M, et al. An ALYREF-MYCN coactivator complex drives neuroblastoma tumorigenesis through effects on USP3 and MYCN stability. Nat Commun 2021;12:1881. [Crossref] [PubMed]
- Cohen EEW, Soulières D, Le Tourneau C, et al. Pembrolizumab versus methotrexate, docetaxel, or cetuximab for recurrent or metastatic head-and-neck squamous cell carcinoma (KEYNOTE-040): a randomised, open-label, phase 3 study. Lancet 2019;393:156-67. [Crossref] [PubMed]
- Fumet JD, Truntzer C, Yarchoan M, et al. Tumour mutational burden as a biomarker for immunotherapy: Current data and emerging concepts. Eur J Cancer 2020;131:40-50. [Crossref] [PubMed]
- Ahmed ME, Falasiri S, Hajiran A, et al. The Immune Microenvironment in Penile Cancer and Rationale for Immunotherapy. J Clin Med 2020;9:3334. [Crossref] [PubMed]
- Schoeler K, Aufschnaiter A, Messner S, et al. TET enzymes control antibody production and shape the mutational landscape in germinal centre B cells. The FEBS journal 2019;286:3566-81. [Crossref] [PubMed]
- Yue X, Lio CJ, Samaniego-Castruita D, Li X, et al. Loss of TET2 and TET3 in regulatory T cells unleashes effector function. Nat Commun 2019;10:2011. [Crossref] [PubMed]
(English Language Editor: J. Jones)