Risk score model of autophagy-related genes in osteosarcoma
Introduction
Osteosarcoma (OS) is a common pediatric malignancy with high disability and mortality rates (1). With improved understanding of cancer pathogenesis and the updating of diagnostic methods, the 5-year survival rate of patients with OS has increased from <20% to 50–60% (2). So far, the prognosis still depends largely on tumor stage system and histopathological diagnosis. However, traditional methods are not enough to accurately evaluate the prognosis of OS patients (3). Therefore, it is necessary to develop accurate and reliable biological indicators to help doctors choose optional treatment methods. In recent years, oncology biology has made significant progress in the field of OS (4). One of the achievements is that macroautophagy (referred to thereafter as autophagy) are involved in the therapeutic response of tumor (5,6).
Autophagy is a highly conservative catabolic cellular event degrading aggregated proteins and damaged organelles. The dynamic process includes induction, nucleation of the autophagosome, growth of the double-membrane, sealing and merging with the lysosome, and the disintegration of engulfed materials (3). Typically, a basal level of autophagy exists everywhere in the cell to decompose and reuse non-functional cellular contents as a source of intracellular nutrition. In response to various stimuli and stresses, such as hunger, hypoxia and drugs, the extent of autophagy can increase dramatically to provide intracellular nutrients and remove harmful content (e.g., damaged mitochondria) (4). It suggests that autophagy is subject to highly orchestrated regulation. Several known signaling pathways for regulating key cellular events are also involved in autophagy, including AMPK/CaMKK, p53/DRAM, PI3K/AKT/mTOR, JAK-STAT and RAS signaling pathways (3).
Autophagy is a double-edged sword in the carcinogenesis process. It inhibits or promotes the development of tumors in a situation-dependent manner, which depends on the tumor type, clinical stage, genetic background, and even treatment strategies. More and more studies have shown the significance of autophagy in OS (3). Autophagy is a pre-survival pathway used by OS tumor cells to increase their proliferation and development, resist cancer therapy, and preserve the cancer stem cell (CSC) pool within the tumor. However, autophagy may also be anti-tumor in OS and lead to cell death (7). Prominently, in tumors with mutations in the RAS pathway genes, hyperactivity of autophagy is indispensable to meet extraordinarily high demands of tumor cell metabolism (8). Numerous studies have proven that autophagy participates in the occurrence and development of OS by inhibiting the signaling pathway PI3K/AKT/mTOR (9-11). Besides, autophagy-related genes (ARGs) (CCL2, EGFR and MYC) were associated with a different prognosis in OS (12).
During tumor development, cancer cells pathologically affect the tumor microenvironment (TME) by inducing various types of stress, including hypoxia, oxidative stress and acidosis (13). These effects cause abnormal reactions of adjacent immune cells and stromal cells that promote necrosis and metastasis (14). Therapeutic strategies targeting tumor-associated macrophages have been shown to significantly inhibit the metastasis of advanced OS (15,16). Although a large number of studies have been published, no effective immunotherapy has been developed to treat OS due to the rarity and heterogeneity of OS, the lack of specific tumor antigens, and the target effect of drugs (17-19).
These findings substantiate the involvement of autophagy in OS and suggest that ARGs may hold great promise as prognostic markers in OS. Hence, we considered that constructing and validating a risk score model of the ARGs of OS would benefit evaluation of both treatment and prognosis.
There were several similar reports about the establishment of risk score model of the ARGs of OS in PubMed, but their researchs were limited to a separate model. We have integrated the data of OS in TARGET (Therapeutically Applicable Research To Generate Effective Treatments) database (https://ocg.cancer.gov/programs/target) and GEO (Gene Expression Omnibus) database (www.ncbi.nlm.nih.gov/geo), whose sample size is more than these two studies. Secondly, the receiver operating characteristic (ROC) value of our model is 0.930, which is better than that of the models in these two studies, and can more accurately predict the survival rate of OS patients. We present the following article in accordance with the TRIPOD reporting checklist (available at https://atm.amegroups.com/article/view/10.21037/atm-22-166/rc).
Methods
Data preparation
The TARGET database is a comprehensive database for pediatric tumors. It aims to identify the biological processes (BPs) in pediatric tumors using comprehensive genomics methods, using the data to help guide the development of less toxic and more effective therapies through the generation of useful drug targets and prognostic markers for researchers (20).
The gene sets information (85 patients: TARGET-OS) was contained as a training set from the TARGET database (21). Clinical information included survival time, survival state, sex, age, disease at diagnosis, primary tumor site, specific tumor region and definitive surgery. The autophagy information used for selecting the ARGs was collected from the Human Autophagy Database (HADb) (22). Another dataset (GSE16091) was downloaded from the GEO database as the validation set (23).
Model establishment
To establish our model, we combined univariate Cox-LASSO–multivariate Cox regression with consideration of the clinical factors, and finally selected the ARGs to establish the risk score model. Univariate and multivariate Cox regression analyses were performed using R’s “survival” package, and P<0.01 was used as the filtering condition of univariate Cox. To prevent large variance, we performed LASSO regression analysis using R’s “GLMNET” package, and determined K value by minimum lambda (21). The gene at the minimum of the Akaike information criterion (AIC) was calculated and used as the variable to be included in the model, and each patient’s gene expression level were used in the model to calculate the risk score, with the algorithm as follows:, where coefx is the regression coefficient obtained from multivariate Cox regression analysis, and Exprx represents the gene expression level of each variable (24,25). The median risk score of each patient was taken as the reference standard for dividing the high and low groups, and then the Kaplan-Meier method (K-M method) was used to analyze the survival of the two groups and draw the survival curves (26). In order to avoid mutual influence between risk factors, we conducted principal component analysis (PCA) and dimension reduction. According to the key parameters and scores of the model, and in combination with various clinical factors, we drew a clinically relevant nomogram for predicting the 1-, 3-, and 5-year survival rates. The scales on the nomogram represented the numerical ranges of each variable. The total scores calculated for each variable could be used to predict the survival rate (27).
Model validation
We used the “Survival ROC” package to draw ROC curves and used the “RMS” package to obtain the calibration curve to evaluate the accuracy of the predicted 1-, 3- and 5-year survival rates, and used the ROC curves to verify each grouping variable (28). The diagnostic value of key ARGs was validated by a dataset from the GEO database (34 patients), and the diagnostic value of each key ARG was evaluated by the area under the ROC.
Gene enrichment analysis (GO and KEGG)
We investigated the cellular components (CC), BP and molecular function (MF) of the ARGs in the gene ontology (GO) database. And selected ARGs were utilized in the functional pathway analysis of Kyoto Encyclopedia of Genes and Genomes (KEGG). R software and ClusterProfiler package were used to conduct the functional enrichment analysis. We then used the “corrplot” package to analyse the relationships between ARGs by Pearson’s correlation coefficient.
Gene set enrichment analysis (GSEA)
GSEA is a method used for enrichment of gene sets to determine the distribution differences between whole gene sets and phenotypes, thereby achieving enrichment. The grouping file of the ARGs expression differences and the downloaded expression matrix file of OS common transcription group were input into GSEA4.0.3 software (29). The data sets used for enrichment were C2 and C5 molecular sets from the Molecular Characteristic Database (MSigDB), and the output results were adjusted to 100 sheets (30). Finally, the enrichment gene sets were screened according to | Normalized Enrichment Score | (|NES|) >1, False-DiscoveryRate (FDR) <0.25 and P<0.05.
Immune cell infiltration
We visualized the proportions of immune cell signatures in the training set. The single-sample GSEA (ssGSEA) algorithm was used to access the immune cell infiltration level and the stromal content for each OS sample. The ConsensuClusterPlus R package was used to perform consensus clustering.
Prediction of response to targeted therapy
Half-maximal inhibitory concentrations (IC50) of targeted therapeutic drugs were plotted using R’s “ggplot2” and “pRRophetic” packages. The relationship between the IC50s and high- and low-risk groups were represented by box plots.
Immunohistochemistry
We obtained five OS and five paracancerous samples from The First Affiliated Hospital of Guangxi Medical University for immunohistochemistry. All patients were diagnosed as OS by pathology. Clinicopathological data, such as sex and age, were collected. The study was conducted in accordance with the Declaration of Helsinki (as revised in 2013). This study was approved by The First Affiliated Hospital of Guangxi Medical University Ethics Review Committee [approval No. 2021(KY-E-125)]. Because the information and privacy of the patients needed to be fully protected, the requirement for obtaining informed consent was waived.
The tissue slices were placed in xylene for 20 min, then fresh xylene was replaced and repeated once more. The dewaxed tissue slices were soaked in 100% ethanol for 5 min twice, then 95% ethanol, 80% ethanol and distilled water for 5 min respectively. The alkaline antigen repair solution (Tri-EDTA, pH=9) was heated to boiling in a pressure cooker, the tissue slices were placed in the solution for 2 min, then cooled to room temperature naturally (31). Next, they were incubated with 3% H2O2 at room temperature for 10 min in the dark, then blocked with normal sheep serum solution at room temperature for 30 min. The primary antibody RP215 was added at 4 ℃ overnight and then treated with HRP-labeled secondary antibody at room temperature for 30 min. Then, diaminobenzidine (DAB) coloration and hematoxylin counterstaining was carried (31).
Ten high-power (×400) visual fields were randomly selected, and two researchers independently read the images. Hematoxylin stained the cell nucleus blue, and positive expression of DAB is brownish yellow.
Statistical analysis
All data were statistically analyzed using SPSS22.0 (IBM) and R 3.6.2 (https://www.r project.org/). Hazard ratio (HR) and 95% confidence interval (CI) were used to represent the relative risk between each variable and the prognosis of OS patients. All results P<0.05 were considered statistically significant.
Results
Collation of ARGs
The expression files of 85 patients (from TARGET-OS, all diagnosed with OS) downloaded from TARGET and 232 autophagy genes in HADb were combined to find OS-related ARGs and their expression levels and clinical information. Expression files of 34 patients (from GSE16091, all diagnosed with OS) were used to validate the model’s stability. Based on univariate Cox analysis, 10 survival-related ARGs were obtained (Figure 1A). We then used multivariate Cox regression and the LASSO method to generate a classifier to forecast OS according to the expression of ARGs (Figure 1B,1C). Finally, a combination of five genes (CCL2, AMBRA1, VEGFA, MYC and EGFR) remained as predictors in the model (Figure 1D).
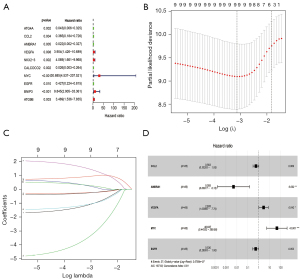
Data preprocessing and risk score model establishment
According to the median risk score of the training set, the patients in the training and validation sets were divided into low- and high-risk groups. Survival analysis between groups showed that a low-risk score significantly correlated with good prognosis of OS patients (Figure 2A,2B). A heatmap to demonstrate the expression level of the five genes from their signatures was plotted (Figure 2C). Similar results were observed in the validation set (Figure 2D-2F). According to the results, the expression levels of CCL2, AMBRA1 and EGFR were relatively lower in the high-risk group. On the other hand, patients in the high-risk group tended to have a higher VEGFA and MYC expression levels. The survival rates and gene expression levels of each hub ARG are shown in Figure 3.
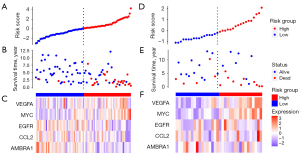
Nomogram development and verification
After PCA, a risk score combined with five independently related risk factors (sex, age, disease at diagnosis, definitive surgery and risk score) was used to form an OS risk estimation nomogram (Figure 4). K-M curves indicated that over time, the survival rate of the low-risk group was higher than that of the high-risk group (Figure 5A). The C-index (0.853 in the training set) and calibration curve were plotted to assess prediction accuracy (Figure 5B,5C). The validation group C index (0.879) and calibration curve also showed high prediction of actual survival rate (Figure 5D-5F).
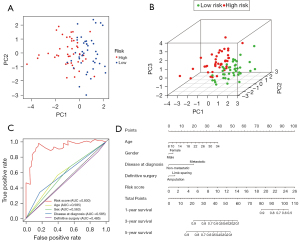
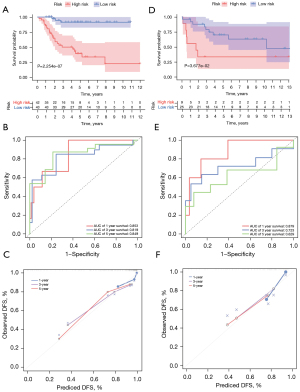
KEGG and GO analysis
To clarify the biological pathways and processes related to the five ARGs, we carried out KEGG signaling pathway and GO functional process analysis. The results indicated that these ARGs were functional in autophagy-related processes such as the PI3K-Akt signaling pathway and MAPK signaling pathway (Figure 6).
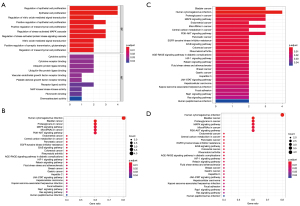
GSEA
The patients were stratified according to their median of risk scores. GSEA results revealed that the five ARGs were favorably enriched in the biocarta-TOB1 pathway, antigen-receptor mediated signaling pathway, inflammatory response to antigenic stimulus, membrane invagination, phagocytic vesicle membrane, regulation of lymphocyte activation, T cell receptor signaling pathway, reactome costimulation by the CD28 family and reactome signaling by the B cell receptor, and natural killer (NK) cell-mediated cytotoxicity (Figure 7).
Immune infiltrating
Except for actived dendritic cells (aDCs) and immature dendritic cells (iDCs), the numbers of other immune cells and immune functions in the low-risk group were significantly higher than in the high-risk group (Figure 8A-8C).
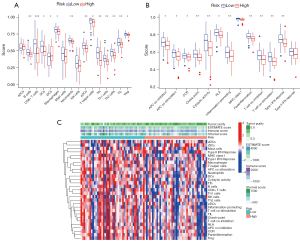
Response to targeted therapy
According to the predicted IC50s, the high- and low-risk groups had different responses to various targeted drugs, and the difference was statistically significant. The high-risk group had lower IC50s, which indicated that the group was more sensitive to targeted drugs (Figure 9).
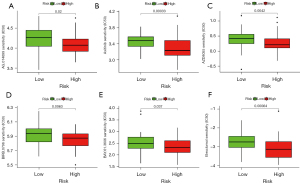
Immunohistochemistry
The expressions of the hub genes were more obvious in the OS group than in the paracancerous group (Figure 10).
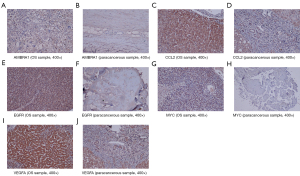
Discussion
Autophagy is a “self-digesting” metabolic process of cell renewal, which has been proven to continue the growth of tumor cells by maintaining cell energy production (32). In addition, inhibition of autophagy enhances the effectiveness of anticancer therapy (33). These findings substantiate the involvement of autophagy in OS and suggest that ARGs may hold great promise as prognostic markers in OS. Hence, we constructed and validated a risk score model of the ARGs of OS to benefit evaluation of both treatment and prognosis.
We screened OS-related ARGs to obtain five key genes that are potential targets for new molecular therapies. A risk prediction model was constructed for these five key ARGs, and it demonstrated that the survival curves of the high- and low-risk groups were significantly separated (31). In the time-dependent model, the risk score and number of deaths increased significantly with time, indicating that these five key ARGs have great significance in predicting OS prognosis. From the ROC prediction results of 1-, 3-, and 5-year survival rates, the five key ARGs could predict the prognosis of OS patients well. Similarly, the five key ARGs also showed satisfactory results in the study of clinical traits.
Four of the five key ARGs have been previously identified. Chen et al. reported that high-grade OS cells highly express CCL2, and that OS cells with high expression of CCL2 are more closely related to proliferation and invasion (34). Another study found that microRNA (miR)-150-5p weakens the proliferative and invasive potential of OS cells by downregulating the VEGFA level, and knockdown of VEGFA remarkably weakened the proliferative and invasive capacities of OS cells (35).
Moreover, researchers have applied multi-region whole-genome sequencing to identify that amplification of the MYC oncogene is the main cause of paediatric OS (36). A previous study also revealed that the miR-7/EGFR pathway is significant in the metastasis of OS cells and indicated that EGFR has the potential to become a prognostic marker and a promising therapeutic target for OS (37). To date, AMBRA1 has not been directly related to OS, but it has been related to prostatic cancer (38).
According to our enrichment analyses, OS autophagy was associated with the PI3K–Akt and MAPK signaling pathways. Jin et al. demonstrated that miR-1224-5p targeted PLK1 and mediated autophagy by blocking the PI3K/Akt/mTOR signaling pathway (39). Previous studies demonstrated that PEITC could induce autophagy in K7M2 OS cells and eventually activated the ROS-related MAPK signaling pathway (40); moreover, escin was proved to be an activator of the ROS/p38 MAPK signaling pathway to counteract OS by inducing autophagy (41). These studies show that our results were credible. The GSEA results suggested that autophagy in OS is favorably related to immune and inflammatory responses. Related pathways including B cells, T cells and NK cells may be the potential direction of targeted therapy.
The predicted IC50s indicated that the high-risk group was more sensitive to targeted drugs. Previous research has proved the importance of these targeted drugs in biological tumor cytology. For example, axitinib (AG-013736) is a potent and selective inhibitor of VEGFR-1–3. In transfected or endogenous RTK-expressing cells, axitinib potently blocked growth factor-stimulated phosphorylation of VEGFR-2 and VEGFR-3 (42,43). AZD8055 showed low activity against all PI3K subtypes (α, β, γ, δ) and other near-PI3K kinase families (ATM and DNA-PK). AZD8055 inhibits the phosphorylation of mTORC1 (p70S6K and 4E-BP1), mTORC2 (AKT), and downstream proteins (44). BIRB 796 is one of the most effective and slowest-separating inhibitors of human p38 MAPK (45). The combination of BIRB0796 with p38 MAPKs or JNK1/2 decreased phosphorylation of upstream kinase MKK6 or MKK4, but did not enhance dephosphorylation (46). The results of these drug sensitivity analyses coincided with those for the pathway analyses mentioned above.
The tumor-associated immune response is integral in the TME, whereas autophagy is of great significance in regulating tumor-related immune responses (47). Immune cell infiltration analyses showed that both immune cells and immune functional processes were obviously significant in the low-risk group, demonstrating that the high-risk group had less autophagy-related immune response.
Some limitations exist in this study. For example, the sample size is relatively small. Further research with more samples is needed to better evaluate the performance of the model and elucidate the underlying mechanism in the future.
Conclusions
In summary, we identified a 5-autophagy-gene-based prognostic signature in OS. We created a risk score model according to five ARGs associated with OS and verified its accuracy and stability. This risk score model shows commendable performance to predict the prognosis of OS at 1, 3, and 5 years for OS patients independently, which will provides potential guidance of OS targeted therapy.
Acknowledgments
The authors thank The First Affiliated Hospital of Guangxi Medical University for the assistance with data collection.
Funding: This work was supported by Guangxi Natural Science Foundation (No. 2017JJA10088).
Footnote
Reporting Checklist: The authors have completed the TRIPOD reporting checklist. Available at https://atm.amegroups.com/article/view/10.21037/atm-22-166/rc
Data Sharing Statement: Available at https://atm.amegroups.com/article/view/10.21037/atm-22-166/dss
Conflicts of Interest: All authors have completed the ICMJE uniform disclosure form (available at https://atm.amegroups.com/article/view/10.21037/atm-22-166/coif). All authors report that this work was supported by Guangxi Natural Science Foundation (No. 2017JJA10088). The authors have no other conflicts of interest to declare.
Ethical Statement: The authors are accountable for all aspects of the work in ensuring that questions related to the accuracy or integrity of any part of the work are appropriately investigated and resolved. This study was approved by The First Affiliated Hospital of Guangxi Medical University Ethics Review Committee [approval No. 2021(KY-E-125)]. The study was conducted in accordance with the Declaration of Helsinki (as revised in 2013). Because the information and privacy of the patients needed to be fully protected, the requirement for obtaining informed consent was waived.
Open Access Statement: This is an Open Access article distributed in accordance with the Creative Commons Attribution-NonCommercial-NoDerivs 4.0 International License (CC BY-NC-ND 4.0), which permits the non-commercial replication and distribution of the article with the strict proviso that no changes or edits are made and the original work is properly cited (including links to both the formal publication through the relevant DOI and the license). See: https://creativecommons.org/licenses/by-nc-nd/4.0/.
References
- Pingping B, Yuhong Z, Weiqi L, et al. Incidence and Mortality of Sarcomas in Shanghai, China, During 2002-2014. Front Oncol 2019;9:662. [Crossref] [PubMed]
- Sayles LC, Breese MR, Koehne AL, et al. Genome-Informed Targeted Therapy for Osteosarcoma. Cancer Discov 2019;9:46-63. [PubMed]
- Levy JMM, Towers CG, Thorburn A. Targeting autophagy in cancer. Nat Rev Cancer 2017;17:528-42. [Crossref] [PubMed]
- Marinković M, Šprung M, Buljubašić M, et al. Autophagy Modulation in Cancer: Current Knowledge on Action and Therapy. Oxid Med Cell Longev 2018;2018:8023821. [Crossref] [PubMed]
- Sharma K, Le N, Alotaibi M, et al. Cytotoxic autophagy in cancer therapy. Int J Mol Sci 2014;15:10034-51. [Crossref] [PubMed]
- Guo JY, White E. Autophagy, Metabolism, and Cancer. Cold Spring Harb Symp Quant Biol 2016;81:73-8. [Crossref] [PubMed]
- Camuzard O, Santucci-Darmanin S, Carle GF, et al. Role of autophagy in osteosarcoma. J Bone Oncol 2019;16:100235. [Crossref] [PubMed]
- Guo JY, White E. Autophagy is required for mitochondrial function, lipid metabolism, growth, and fate of KRAS(G12D)-driven lung tumors. Autophagy 2013;9:1636-8. [Crossref] [PubMed]
- Yang Z, Yu W, Liu B, et al. Estrogen receptor β induces autophagy of osteosarcoma through the mTOR signaling pathway. J Orthop Surg Res 2020;15:50. [Crossref] [PubMed]
- Meng CY, Zhao ZQ, Bai R, et al. MicroRNA-22 mediates the cisplatin resistance of osteosarcoma cells by inhibiting autophagy via the PI3K/Akt/mTOR pathway. Oncol Rep 2020;43:1169-86. [Crossref] [PubMed]
- Pan Z, Cheng DD, Wei XJ, et al. Chitooligosaccharides inhibit tumor progression and induce autophagy through the activation of the p53/mTOR pathway in osteosarcoma. Carbohydr Polym 2021;258:117596. [Crossref] [PubMed]
- Qi W, Yan Q, Lv M, et al. Prognostic Signature of Osteosarcoma Based on 14 Autophagy-Related Genes. Pathol Oncol Res 2021;27:1609782. [Crossref] [PubMed]
- Chen JL, Lucas JE, Schroeder T, et al. The genomic analysis of lactic acidosis and acidosis response in human cancers. PLoS Genet 2008;4:e1000293. [Crossref] [PubMed]
- Roma-Rodrigues C, Mendes R, Baptista PV, et al. Targeting Tumor Microenvironment for Cancer Therapy. Int J Mol Sci 2019;20:840. [Crossref] [PubMed]
- Buddingh EP, Kuijjer ML, Duim RA, et al. Tumor-infiltrating macrophages are associated with metastasis suppression in high-grade osteosarcoma: a rationale for treatment with macrophage activating agents. Clin Cancer Res 2011;17:2110-9. [Crossref] [PubMed]
- Ratti C, Botti L, Cancila V, et al. Trabectedin Overrides Osteosarcoma Differentiative Block and Reprograms the Tumor Immune Environment Enabling Effective Combination with Immune Checkpoint Inhibitors. Clin Cancer Res 2017;23:5149-61. [Crossref] [PubMed]
- Saraf AJ, Fenger JM, Roberts RD. Osteosarcoma: Accelerating Progress Makes for a Hopeful Future. Front Oncol 2018;8:4. [Crossref] [PubMed]
- Wang Z, Li B, Ren Y, et al. T-Cell-Based Immunotherapy for Osteosarcoma: Challenges and Opportunities. Front Immunol 2016;7:353. [Crossref] [PubMed]
- Miwa S, Yamamoto N, Hayashi K, et al. Therapeutic Targets for Bone and Soft-Tissue Sarcomas. Int J Mol Sci 2019;20:170. [Crossref] [PubMed]
- Nagarajan R, Weigel BJ, Thompson RC, et al. Osteosarcoma in the first decade of life. Med Pediatr Oncol 2003;41:480-3. [Crossref] [PubMed]
- Dong S, Huo H, Mao Y, et al. A risk score model for the prediction of osteosarcoma metastasis. FEBS Open Bio 2019;9:519-26. [Crossref] [PubMed]
- Homma K, Suzuki K, Sugawara H. The Autophagy Database: an all-inclusive information resource on autophagy that provides nourishment for research. Nucleic Acids Res 2011;39:D986-90. [Crossref] [PubMed]
- Zhong X, Liu Y, Liu H, et al. Identification of Potential Prognostic Genes for Neuroblastoma. Front Genet 2018;9:589. [Crossref] [PubMed]
- Birnbaum DJ, Finetti P, Lopresti A, et al. A 25-gene classifier predicts overall survival in resectable pancreatic cancer. BMC Med 2017;15:170. [Crossref] [PubMed]
- Mao Y, Fu Z, Zhang Y, et al. A six-microRNA risk score model predicts prognosis in esophageal squamous cell carcinoma. J Cell Physiol 2019;234:6810-9. [Crossref] [PubMed]
- Han B, Zhang H, Zhu Y, et al. Subtype-specific risk models for accurately predicting the prognosis of breast cancer using differentially expressed autophagy-related genes. Aging (Albany NY) 2020;12:13318-37. [Crossref] [PubMed]
- Liao S, He J, Liu C, et al. Construction of autophagy prognostic signature and analysis of prospective molecular mechanisms in skin cutaneous melanoma patients. Medicine (Baltimore) 2021;100:e26219. [Crossref] [PubMed]
- Jiao Y, Fu Z, Li Y, et al. High EIF2B5 mRNA expression and its prognostic significance in liver cancer: a study based on the TCGA and GEO database. Cancer Manag Res 2018;10:6003-14. [Crossref] [PubMed]
- Subramanian A, Tamayo P, Mootha VK, et al. Gene set enrichment analysis: a knowledge-based approach for interpreting genome-wide expression profiles. Proc Natl Acad Sci U S A 2005;102:15545-50. [Crossref] [PubMed]
- Liberzon A, Birger C, Thorvaldsdóttir H, et al. The Molecular Signatures Database (MSigDB) hallmark gene set collection. Cell Syst 2015;1:417-25. [Crossref] [PubMed]
- Xu J, Nie H, He J, et al. Using Machine Learning Modeling to Explore New Immune-Related Prognostic Markers in Non-Small Cell Lung Cancer. Front Oncol 2020;10:550002. [Crossref] [PubMed]
- Choi AM, Ryter SW, Levine B. Autophagy in human health and disease. N Engl J Med 2013;368:651-62. [Crossref] [PubMed]
- Giampieri F, Afrin S, Forbes-Hernandez TY, et al. Autophagy in Human Health and Disease: Novel Therapeutic Opportunities. Antioxid Redox Signal 2019;30:577-634. [Crossref] [PubMed]
- Chen Q, Sun W, Liao Y, et al. Monocyte chemotactic protein-1 promotes the proliferation and invasion of osteosarcoma cells and upregulates the expression of AKT. Mol Med Rep 2015;12:219-25. [Crossref] [PubMed]
- Qin Y, Zhang B, Ge BJ. MicroRNA-150-5p inhibits proliferation and invasion of osteosarcoma cells by down-regulating VEGFA. Eur Rev Med Pharmacol Sci 2020;24:9265-73. [PubMed]
- De Noon S, Ijaz J, Coorens TH, et al. MYC amplifications are common events in childhood osteosarcoma. J Pathol Clin Res 2021;7:425-31. [Crossref] [PubMed]
- Li H, Lan M, Liao X, et al. Circular RNA cir-ITCH Promotes Osteosarcoma Migration and Invasion through cir-ITCH/miR-7/EGFR Pathway. Technol Cancer Res Treat 2020;19:1533033819898728. [Crossref] [PubMed]
- Liu J, Chen Z, Guo J, et al. Ambra1 induces autophagy and desensitizes human prostate cancer cells to cisplatin. Biosci Rep 2019;39:BSR20170770. [Crossref] [PubMed]
- Jin B, Jin D, Zhuo Z, et al. MiR-1224-5p Activates Autophagy, Cell Invasion and Inhibits Epithelial-to-Mesenchymal Transition in Osteosarcoma Cells by Directly Targeting PLK1 Through PI3K/AKT/mTOR Signaling Pathway. Onco Targets Ther 2020;13:11807-18. [Crossref] [PubMed]
- Lv HH, Zhen CX, Liu JY, et al. PEITC triggers multiple forms of cell death by GSH-iron-ROS regulation in K7M2 murine osteosarcoma cells. Acta Pharmacol Sin 2020;41:1119-32. [Crossref] [PubMed]
- Zhu J, Yu W, Liu B, et al. Escin induces caspase-dependent apoptosis and autophagy through the ROS/p38 MAPK signalling pathway in human osteosarcoma cells in vitro and in vivo. Cell Death Dis 2017;8:e3113. [Crossref] [PubMed]
- Hu-Lowe DD, Zou HY, Grazzini ML, et al. Nonclinical antiangiogenesis and antitumor activities of axitinib (AG-013736), an oral, potent, and selective inhibitor of vascular endothelial growth factor receptor tyrosine kinases 1, 2, 3. Clin Cancer Res 2008;14:7272-83. [Crossref] [PubMed]
- Fenton BM, Paoni SF. The addition of AG-013736 to fractionated radiation improves tumor response without functionally normalizing the tumor vasculature. Cancer Res 2007;67:9921-8. [Crossref] [PubMed]
- Chresta CM, Davies BR, Hickson I, et al. AZD8055 is a potent, selective, and orally bioavailable ATP-competitive mammalian target of rapamycin kinase inhibitor with in vitro and in vivo antitumor activity. Cancer Res 2010;70:288-98. [Crossref] [PubMed]
- Pargellis C, Tong L, Churchill L, et al. Inhibition of p38 MAP kinase by utilizing a novel allosteric binding site. Nat Struct Biol 2002;9:268-72. [Crossref] [PubMed]
- Kuma Y, Sabio G, Bain J, et al. BIRB796 inhibits all p38 MAPK isoforms in vitro and in vivo. J Biol Chem 2005;280:19472-9. [Crossref] [PubMed]
- Marar C, Starich B, Wirtz D. Extracellular vesicles in immunomodulation and tumor progression. Nat Immunol 2021;22:560-70. [Crossref] [PubMed]