Machine learning-based combined nomogram for predicting the risk of pulmonary invasive fungal infection in severely immunocompromised patients
Introduction
Invasive fungal infection (IFI) in the lung is a common complication in severely immunocompromised patients, such as those with hematologic malignancies who have undergone hematopoietic stem cell transplantation or those receiving high-dose chemotherapy (1). IFI is associated with substantial morbidity, having a mortality rate of 50% to 85% (2); given this, early and accurate diagnosis of IFI is vital to enable optimized treatment and improved patient outcomes. Clinicians now have various commercially available tools for IFI diagnosis, and each tool has its inherent advantages and disadvantages (3). Serum galactomannan testing may help with the diagnosis, but it has limited sensitivity, which ranges from 30% to 46%, and it frequently yields false-positive results (4,5). Recently, a consensus group of the European Organization for Research and Treatment of Cancer (EORTC) and the Mycoses Study Group (MSG) established diagnostic criteria for IFI (6). These updated guidelines are based on host factors, clinical information, and microbiological criteria, which allow classification of IFIs into various levels of probability as proven, probable, or possible IFIs. However, only a minority of cases are proven using invasive procedures because of the risk of fatal bleeding due to thrombocytopenia.
Computed tomography (CT) is an important imaging modality in the diagnosis and management of high-risk patients with pneumonia. Several studies have focused on describing early predictive CT features of pulmonary IFIs (7-9). Characteristic CT signs of invasive fungal pneumonia consist of multiple nodules with a surrounding “halo sign” and/or wedge-shaped areas of consolidation (8). However, these CT findings of IFIs are usually nonspecific and indistinguishable from other respiratory infections. In particular, when consolidation is present early during pneumonia, differential diagnosis is often difficult and only presumptive.
Radiomics, an emerging quantitative imaging method we proposed in 2012 (10), can extract mineable high-dimensional data from medical images at the voxel or pixel level. It serves as the bridge between imaging biomarkers and personalized medicine to reflect underlying structural and pathophysiologic information (11). A recent radiomics study indicated potential in the discrimination of progressive pulmonary tuberculosis from community-acquired pneumonia (12). More recently, the combination of radiomics and machine learning (ML) models has been demonstrated to potentially offer improved diagnostic, prognostic, and predictive accuracy (13). The correlation of imaging findings with clinical information is fundamental for rapid, accurate diagnosis (14). However, to the best of our knowledge, an ML-based approach for incorporating imaging biomarkers with clinical characteristics in the prediction of IFIs for clinical decision support has not been developed.
Therefore, the purpose of this study was to investigate whether an ML-based clinical radiomic nomogram integrating CT radiomic features and clinical factors can distinguish IFI from bacterial pneumonia in patients with hematologic malignancies. In addition, the benefit provided by 3-dimensional (3D) radiomic features compared with 2-dimensional (2D) texture analysis was explored. We present the following article in accordance with the TRIPOD reporting checklist (available at https://atm.amegroups.com/article/view/10.21037/atm-21-4980/rc).
Methods
The clinical trial was registered on https://www.chictr.org.cn (registration number: ChiCTR2000038557). The study was conducted in accordance with the Declaration of Helsinki (as revised in 2013). The study was approved by institutional review board of Nanfang Hospital (No. NFEC-2017-087), and individual consent for this retrospective analysis was waived.
Patient selection
Patients from the Department of Hematology who underwent chest CT between June 2017 and March 2021 were identified using our institution’s database. The inclusion criteria were as follows: (I) age 18 years and over and with host factors according to the EORTC and/or MSG criteria (6); (II) suspected of having a pulmonary infection and clinical symptoms and signs of persistent cough, pleural pain, or hemoptysis; (III) CT scan with thin section (slice thickness less than 1.5 mm); and (IV) CT findings of a consolidation measuring greater than 5 mm in diameter. The exclusion criteria were as follows: (I) patients who underwent CT scan within more than 5 days after initiation of symptoms (n=16); (II) patients with incomplete clinic and etiological data including basic information, comorbidities, symptoms, and laboratory findings (Table S1) available for re-evaluation (n=47); and (III) patients who received antibiotic therapy (n=23).
All patients with proven or probable pulmonary IFI were diagnosed according to the consensus EORTC and/or MSG criteria. Proven IFI was defined based on histopathologic evidence following percutaneous needle aspiration biopsy. Probable IFI was defined based on the presence of host factors, an area of consolidation on CT, and mycological evidence of fungal infection from culture analysis of bronchoalveolar lavage fluid or serum or microbiologic evidence of galactomannan positivity (8). Diagnosis of bacterial pneumonia was based on a positive culture of respiratory tract specimen for a bacterial organism, such as sputum or bronchoalveolar lavage fluid and CT evidence of pulmonary consolidation >5 mm in diameter (8). The pathway of patient enrollment is shown in Figure 1.
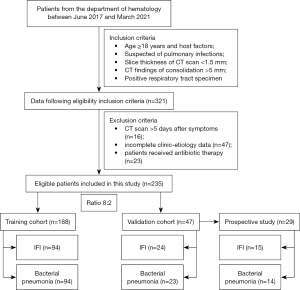
CT image acquisition
All patients were examined with the following CT scanners: Brilliance iCT (Philips Healthcare, Best, Netherlands), GE Discovery CT 750 HD (GE Medical Systems, Chicago, IL, USA), and Somatom Definition and Somatom Emotion (Siemens Medical Solutions, Erlangen, Germany). The CT scanning parameters included a tube voltage of 100 to 130 kV, automatic tube current modulation, a detector collimation of 192 × 0.6 mm or 128 × 0.625 mm, and a slice thickness of 1 to 1.5 mm. All images were reconstructed with a high kernel (b60) and a matrix of 512×512.
Lesion segmentation
The CT images were independently interpreted by 2 thoracic radiologists (G.W and C.Y, with 6 and 12 years of chest CT experience, respectively) who were blinded to the clinical information. The following semantic radiological features were assessed according to published definitions (8) by consensus: single/multiple lesions, consolidation (patchy, segmental, or wedge-shaped), halo sign, cavitation, nodule, and ground-glass opacity (GGO).
The volumes of interest (VOIs) were manually drawn on CT axial slices by 1 thoracic radiologist (C.Y) using ITK-SNAP (version 3.6.0, www.itksnap.org) and encompassed the entire lesion. The 2D-labeling regions of interest (ROIs) were manually delineated based on the single-axial image with the largest lesion area. If multiple lesions were present, only the largest cross-sectional lesion was segmented.
Image preprocessing and feature extraction
Image resampling and gray-level normalization were performed before feature extraction. All image data were resampled to a 1×1×1 mm3 voxel size, and the gray level was normalized to 64 levels. Images were filtered and radiomic features were extracted from VOIs and ROIs using Pyradiomics 2.1.2 (https://pyradiomics.readthedocs.io/en/latest/index.html) (15). The 3D (n=835) and 2D (n=815) radiomic features included (I) first-order statistic features; (II) shape-based features; (III) texture features; and (IV) wavelet-based features.
Dimension reduction with reproducibility and collinearity analysis
A high-dimensional feature reduction process was then performed to select the most relevant features for differential diagnosis. To verify the robustness of the radiomic features, CT images of 30 cases were randomly chosen and independently delineated by 2 radiologists (C.Y and S.W). To assess the intraobserver reproducibility, the same procedure was performed twice by 1 radiologist (C.Y) within 2 weeks of the first delineation. The intra- and interclass correlation coefficient (ICC) was calculated to evaluate the reproducibility of radiomic features. Features with good agreement (both ICCs >0.8) were selected for further analyses. Collinearity analysis with Pearson correlation coefficients was performed to evaluate the relevance and redundancy of the features. Features indicated by a Pearson correlation coefficient (r) >0.9 were eliminated.
Feature selection and model building
The ML models were built with different combinations of 4 common feature selection methods [analysis of variance (ANOVA), recursive feature elimination (RFE), Relief, and Kruskal-Wallis rank-sum test (KW)] and 9 classification methods (logistic regression (LR), least absolute shrinkage and selection operator (LASSO), support vector machine (SVM), linear discriminant analysis (LDA), decision tree, random forest, Adaboost, Gaussian process, and naïve Bayes). Patients were randomly divided into the training and validation group at a ratio of 8:2. All the classifiers were trained with a 5-fold cross-validation. The area under the receiver operator characteristic (ROC) curve (AUC) was used to evaluate the discriminative performance of different models. The model with the highest AUC value in the cross-validation cohort was considered the radiomic model. The radiomic signature (Radscore) was then calculated using a multivariable logistic regression model by linearly combining the most predictive features, which were weighted by their respective coefficients.
Clinical and radiological model building and evaluation
Considering the potential additional value of clinical characteristics, CT features, and laboratory results (Table S1) to improve the predictive performance, we first identified independent variables (P<0.1) using univariate analysis. In the training cohort, a clinical radiomic model incorporating the radiomic signature and clinical predictors was developed using multivariable logistic regression analysis. A collinearity diagnosis with a variance inflation factor was performed before the multivariate analysis. Backward stepwise selection was employed using the likelihood ratio test with Akaike’s information criterion (AIC) as the stopping rule. The model with the lowest AIC value was selected as the final model. A clinical model was also constructed separately using multivariable logistic regression analysis based on statistically significant clinical factors. The ROC curve and AUC were applied to evaluate the predictive accuracy of established models in the validation cohort. Differences in AUC between various prediction models were compared using the DeLong test.
Construction and validation of the radiomic nomogram
The radiomic nomogram was built based on proportionally converting regression coefficients of each predictor in the combined model to a 0- to 100-point scale. Calibration of the clinical radiomic nomogram was plotted using the calibration curve, which measures the consistency between the nomogram-predicted probability and the actual rate. The Hosmer-Lemeshow test was used to assess the goodness of fit of the nomogram. In addition, decision curve analysis (DCA) was conducted to estimate the clinical utility of the radiomic signature, clinical risk factors, and nomogram by quantifying net benefits at different threshold probabilities in the validation dataset. The decision curves of the treat-all strategy and the treat-none strategy were used as references in the DCA. The workflow of this study is shown in Figure 2.
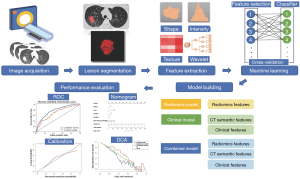
Comparison of the combined nomogram against practicing radiologists
A prospective pilot study was also conducted at our institution from April 2021 to August 2021. The inclusion and exclusion criteria and the definitions for bacterial pneumonia and pulmonary IFI mentioned above were used in the pilot study cohort. A total of 15 patients with IFI and 14 patients with bacterial pneumonia were enrolled to compare the performance of the combined nomogram with practicing radiologists in terms of classification. One junior and one senior radiologist (with 6 and 12 years of experience, respectively) were employed to participate in the study. Ground truth was established based on the enrollment criteria mentioned above.
Statistical analysis
All statistical analyses were performed using R software (version 3.5.2; The R Foundation for Statistical Computing, Vienna, Austria) and SPSS software (version 22.0, IBM Corp., Armonk, NY, USA). Continuous and categorical data were compared using independent t- (or Mann-Whitney U) tests and chi-square (or Fisher’s exact) statistics, respectively, as appropriate. The ML algorithms were programmed with Python scikit-learn environment (version 0.19.1). A 2-sided P value <0.05 was considered to be significant.
The “glmnet” package was used to perform LASSO logistic regression, the ROC was plotted using the “pROC” package, nomogram construction and calibration plotting were performed using the “rms” package, the Hosmer-Lemeshow test was performed using the “generalhoslem” package, and the “rmda” package was used to construct the DCA curve.
Results
Clinical and CT imaging characteristics
A total of 235 patients consisting of 118 individuals with IFI and 117 with bacterial pneumonia were enrolled in the study. Clinical characteristics including CT semantic features, clinical variables, and laboratory parameters of patients with IFI and bacterial pneumonia in both the training and validation datasets are shown in Tables 1,2. There were no differences in sex, age, or most clinical and CT features between the 2 groups. The halo sign and nodule were more common in patients with IFI than in those with bacterial pneumonia, while patchy consolidation and GGO were more frequent in those with bacterial pneumonia (each P<0.05) in the training set. Significant differences between the 2 groups were observed in the incidence of sputum, neutrophil percentage, and lymphocyte percentage.
Table 1
CT features | Training set (n=188), n (%) | Validation set (n=47), n (%) | |||||
---|---|---|---|---|---|---|---|
IFI (n=94) | Bacterial pneumonia (n=94) | P value | IFI (n=24) | Bacterial pneumonia (n=23) | P value | ||
Number of lesions | 0.631 | 0.238 | |||||
Single | 29 (30.9) | 26 (27.7) | 9 (37.5) | 5 (21.7) | |||
Multiple | 65 (69.1) | 68 (72.3) | 15 (62.5) | 18 (78.3) | |||
Consolidation | |||||||
Patchy | 71 (75.5) | 82 (87.2) | 0.039* | 16 (66.7) | 19 (82.6) | 0.210 | |
Segmental | 46 (48.9) | 34 (36.2) | 0.077 | 10 (41.7) | 9 (39.1) | 0.859 | |
Wedge-shaped | 10 (10.6) | 12 (12.8) | 0.650 | 2 (8.3) | 3 (13.0) | 0.601 | |
Halo sign | 0.013* | 0.172 | |||||
Absence | 33 (35.1) | 50 (53.2) | 12 (50.0) | 16 (69.6) | |||
Presence | 61 (64.9) | 44 (46.8) | 12 (50.0) | 7 (30.4) | |||
Cavitation | 0.470 | 0.157 | |||||
Absence | 89 (94.7) | 91 (96.8) | 22 (91.7) | 23 (100.0) | |||
Presence | 5 (5.3) | 3 (3.2) | 2 (8.3) | 0 (0.0) | |||
Nodule | 0.011* | 0.298 | |||||
Absence | 72 (76.6) | 85 (90.4) | 18 (75.0) | 20 (87.0) | |||
Presence | 22 (23.4) | 9 (9.6) | 6 (25.0) | 3 (13.0) | |||
GGO | 0.005* | 0.955 | |||||
Absence | 91 (96.8) | 80 (85.1) | 21 (87.5) | 20 (87.0) | |||
Presence | 3 (3.2) | 14 (14.9) | 3 (12.5) | 3 (13.0) |
*, P<0.05. CT, computed tomography; IFI, invasive fungal infection; GGO, ground-glass opacity.
Table 2
Clinical factors | Training set (n=188) | Validation set (n=47) | |||||
---|---|---|---|---|---|---|---|
IFI (n=94) | Bacterial pneumonia (n=94) | P value | IFI (n=24) | Bacterial pneumonia (n=23) | P value | ||
Age (years) | 43.3±15.6 | 40.5±13.7 | 0.188 | 39.3±11.9 | 43.7±16.2 | 0.293 | |
Sex | 0.766 | 0.671 | |||||
Male | 57 (60.6) | 55 (58.5) | 14 (58.3) | 12 (52.2) | |||
Female | 37 (39.4) | 39 (41.5) | 10 (41.7) | 11 (47.8) | |||
Hematologic malignancy | 0.379 | 0.859 | |||||
AML | 55 (58.5) | 49 (52.1) | 14 (58.3) | 14 (60.9) | |||
Non-AML | 39 (41.5) | 45 (47.9) | 10 (41.7) | 9 (39.1) | |||
Infection history | 43 (45.7) | 35 (37.2) | 0.236 | 11 (45.8) | 6 (26.1) | 0.024* | |
Chemotherapy | 75 (79.8) | 65 (69.1) | 0.094 | 20 (83.3) | 11 (47.8) | 0.010* | |
Immunosuppressant | 5 (5.3) | 7 (7.4) | 0.551 | 2 (8.3) | 1 (4.3) | 0.576 | |
HSCT | 30 (31.9) | 27 (28.7) | 0.634 | 8 (33.3) | 8 (34.8) | 0.917 | |
GVHD | 13 (13.8) | 8 (8.5) | 0.247 | 4 (16.7) | 4 (17.4) | 0.947 | |
Remission | 7 (7.4) | 6 (6.4) | 0.774 | 4 (16.7) | 0 | 0.041* | |
Fever | 56 (59.6) | 56 (59.6) | 1.000 | 13 (54.2) | 18 (78.3) | 0.081 | |
Body temperature (℃) | 37.4±4.1 | 37.9±1.3 | 0.248 | 37.31.3 | 38.21.4 | 0.233 | |
Cough | 34 (36.2) | 44 (46.8) | 0.139 | 10 (41.7) | 14 (60.9) | 0.188 | |
Sputum | 24 (25.5) | 38 (40.4) | 0.030* | 6 (25.0) | 13 (56.5) | 0.028* | |
Chest pain | 6 (6.4) | 7 (7.4) | 0.774 | 1 (4.2) | 2 (8.7) | 0.525 | |
Hemoptysis | 2 (2.1) | 3 (3.2) | 0.65 | 0 | 0 | 1.000 | |
Dyspnea | 2 (2.1) | 4 (4.3) | 0.407 | 0 | 0 | 1.000 | |
Chill | 10 (10.6) | 7 (7.4) | 0.446 | 4 (16.7) | 4 (17.4) | 0.947 | |
Headache | 12 (12.8) | 12 (12.8) | 1.000 | 6 (25.0) | 6 (26.1) | 0.932 | |
WBC (×109/L) | 10.0±46.8 | 8.8±28.2 | 0.831 | 7.8±10.4 | 8.3±15.8 | 0.893 | |
Neutrophil (×109/L) | 3.5±10.9 | 2.6±5.1 | 0.505 | 7.6±15.6 | 2.5±3.2 | 0.137 | |
Neutrophil percentage (%) | 48.5±29.2 | 40.1±27.0 | 0.043* | 55.9±26.6 | 42.1±26.4 | 0.088 | |
Lymphocyte (×109/L) | 5.1±31.1 | 2.6±7.3 | 0.449 | 1.4±1.6 | 1.7±2.0 | 0.543 | |
Lymphocyte percentage (%) | 36.9±27.9 | 44.9±27.2 | 0.049* | 31.0±24.4 | 34.5±23.5 | 0.635 | |
Monocyte (×109/L) | 1.3±5.6 | 3.5±17.0 | 0.251 | 1.3±3.3 | 4.1±11.3 | 0.257 | |
Monocyte percentage (%) | 10.6±11.3 | 14.8±17.1 | 0.052 | 11.9±14.3 | 21.3±24.7 | 0.127 | |
CRP (mg/dL) | 72.8±65.5 | 72.2±70.8 | 0.954 | 62.1±67.5 | 70.4±65.5 | 0.690 | |
G test (%) | 12/31 (38.7) | 8/41 (19.5) | 0.072 | 2/9 (22.2) | 3/9 (33.3) | 0.599 | |
PCT (pg/mL) | 1.2±3.9 | 1.5±5.7 | 0.746 | 0.6±1.2 | 0.3±0.3 | 0.405 |
*, P<0.05. IFI, invasive fungal infection; AML, acute myelogenous leukemia; HSCT, hematopoietic stem cell transplant; GVHD, graft-versus-host disease; WBC, white blood count; CRP, C-reactive protein; PCT, procalcitonin.
Model assessment
A total of 36 predictive radiomic models were built through the combination of 4 selection methods and 9 classifiers. The heatmaps of mean AUC values from various ML models in the training, cross-validation, and validation cohorts are shown in Figure 3 and Figure S1. Generally, the 3D-based models represented better performance than did the 2D-based models. Moreover, LR classifiers outperformed other classification methods in terms of discrimination. Among models using parameters from 3D and 2D images, the selection method KW with LR classifier yielded the highest AUC of 0.644 and 0.641, respectively in the cross-validation group.
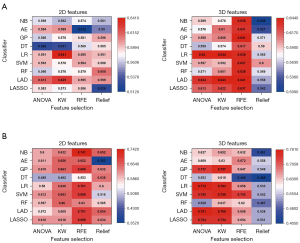
The selected radiomic features to discriminate IFI and bacterial pneumonia in the training cohort are summarized in Table 3, including 1 shape feature, 2 texture features, and 9 wavelet features. A radiomic signature (Radscore) was then constructed using the features and their respective coefficients. There was a significant difference in Radscore between the IFI and bacterial pneumonia patients in the training cohort (0.683 vs. −0.724; P<0.001), which was confirmed in the validation cohort (0.353 vs. −0.717, P=0.002). The AUC of the radiomic signature performance was 0.803 (95% CI: 0.739–0.866) in the training cohort and 0.767 (95% CI: 0.624–0.910) in the validation cohort.
Table 3
Radiomics model | Coefficient | Clinical model | Coefficient | Combined model | Coefficient |
---|---|---|---|---|---|
original_shape_Sphericity | 4.327 | Halo sign | 0.726 | Radscore | 0.959 |
original_glcm_Imc1 | 2.942 | Patchy consolidation | −1.112 | Patchy consolidation | −0.763 |
original_glcm_Imc2 | −1.747 | GGO | −1.748 | GGO | −1.087 |
wavelet-LLH_firstorder_Kurtosis | 4.45 | Nodule | 1.035 | Nodule | 1.087 |
wavelet-LLL_firstorder_Robust Mean Absolute Deviation | 2.004 | Lymphocyte percentage | −0.013 | Sputum | −0.599 |
wavelet-LLL_glcm_Correlation | 3.919 | Monocyte percentage | −0.029 | Monocyte percentage | −0.024 |
wavelet-LLL_glcm_Joint Energy | 0.501 | intercept | 1.352 | intercept | 1.096 |
wavelet-LLL_glcm_Imc1 | −0.978 | ||||
wavelet-LLL_glcm_Imc2 | 3.403 | ||||
wavelet-LLL_glcm_Maximum Probability | 1.908 | ||||
wavelet-LLL_glszm_Large Area High Gray Level Emphasis | −2.48 | ||||
wavelet-LLL_gldm_Large Dependence High Gray Level Emphasis | 0.109 | ||||
Intercept | −0.021 |
GGO, ground-glass opacity.
Effect of combining clinical parameters and radiomics in prediction
In multivariable logistic regression analysis, significant risk factors for IFI included a higher Radscore, lower monocyte percentage, the absence of sputum, and the presence of halo sign, nodule, patchy consolidation, or GGO (all P<0.05). There was no multicollinearity between the significant factors and the Radscore. The list of the selected factors and their associated coefficients in the clinical and combined model are illustrated in Table 3. Our combined model integrating clinical and radiological variables with a radiomic signature achieved an AUC of 0.844 in the validation set, which indicated improved prediction performance compared to the clinical model (AUC =0.696) and radiomic model (AUC =0.767) according to the Delong test (both P<0.01). The predictive performance results of different models are presented in Figure 4 and Table 4.
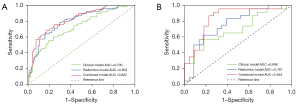
Table 4
Model | Training cohort | Validation cohort | |||||
---|---|---|---|---|---|---|---|
Clinical model | Radiomics model | Combined model | Clinical model | Radiomics model | Combined model | ||
AUC (95% CI) | 0.730 (0.658, 0.802) | 0.803 (0.739, 0.866) | 0.822 (0.762, 0.881) | 0.696 (0.542, 0.850) | 0.767 (0.624, 0.910) | 0.844 (0.723, 0.965) | |
Accuracy | 0.681 | 0.748 | 0.755 | 0.689 | 0.734 | 0.822 | |
Sensitivity | 0.556 | 0.656 | 0.756 | 0.565 | 0.609 | 0.913 | |
Specificity | 0.806 | 0.839 | 0.753 | 0.818 | 0.864 | 0.727 | |
Positive predictive value | 0.557 | 0.657 | 0.756 | 0.592 | 0.638 | 0.952 | |
Negative predictive value | 0.804 | 0.837 | 0.753 | 0.781 | 0.825 | 0.697 |
AUC, area under the receiver operator characteristic curve; CI, confidence interval.
Nomogram apparent performance and clinical use of DCA
The clinical radiomic nomogram was constructed based on the combined model (Figure 5A). The calibration curves demonstrated good consistency between the prediction and actual observation of IFI and bacterial pneumonia in both cohorts (Figure 5B,5C). The Hosmer-Lemeshow test yielded nonsignificant statistics in the training cohort (P=0.619) and validation cohort (P=0.446), suggesting a perfect fit of the nomogram.
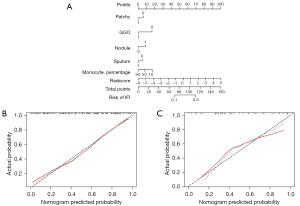
The clinical DCA was used to demonstrate clinical decision utility of the combined nomogram (Figure 6). The nomogram showed higher net benefit than did that using the radiomic signature or clinical features alone, which were better than the treat-all or treat-none strategies.
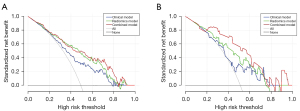
Comparison of the combined nomogram with practicing radiologists
The performances on differentiating IFI from bacterial pneumonia of the developed nomogram and radiologists were then compared. The diagnostic system achieved an accuracy of 79.3%, a sensitivity of 80.0%, and a specificity of 84.6%, which was overall superior to the values of the junior and senior radiologist (Figure 7). Representative cases are shown in Figure 8.
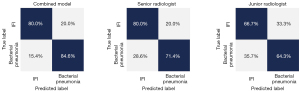
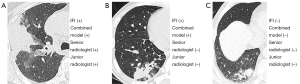
Discussion
In the current study, we developed and validated an ML-based nomogram that incorporates the radiomic signature and clinical characteristics of noninvasive, individualized diagnoses of IFI in patients with hematologic malignancies. The highest performance was achieved with the combination of the selection method KW with LR classifier. The proposed clinical radiomic nomogram demonstrated favorable discrimination in the validation cohort (AUC =0.844), outperforming the radiomic-only and clinical prediction model. The diagnostic performance of the combined model was demonstrated to be superior to that of practicing radiologists in a prospective fashion. ML-based radiomic analysis could potentially serve as a quantitative imaging biomarker to aid discrimination of IFI from bacterial pneumonia.
Pulmonary IFI is life-threatening condition with a considerable rate of mortality in immunocompromised patients (16). Patients with hematologic malignancies have an elevated risk of fungal infection due to hypofunction of the immune system and frequent use of immunosuppressive agents in clinical treatment, and symptoms of pulmonary IFI are often nonspecific (17). In clinical practice, the most challenging task is to distinguish IFI from bacterial pneumonia. Similarly, the more conventional bacterial pathogens are present in opportunistic infections in immunocompetent individuals, with fever, chest pain, hemoptysis, focal consolidation, and rapid increases in inflammatory markers (18). As outlined by the EORTC and MSG in 2002 and updated in 2008, the classification of proven, probable, or possible IFIs is a helpful tool for diagnostic purposes (6). However, the number of proven cases is quite low because fine-needle aspiration is invasive and limited to thrombocytopenia patients.
CT plays a crucial role in clinical practice for the noninvasive diagnosis and management of pulmonary infections (7). The updated guidelines have enlarged the diagnostic role of CT scans, but imaging signs such as macronodules or patchy consolidation with or without the halo sign are still not specific, particularly in the early stages of pulmonary infections. Thus, in most cases, differentiation of fungal and other pneumonias using CT with the traditional practice of visual interpretation remains challenging. Consolidation is the most common CT finding in both diseases. Chen et al. (8) reported that multifocal nonsegmental consolidation was found significantly more often in patients with IFI (48.0%) than in those with bacterial pneumonia (22.6%). By contrast, we found a significantly higher frequency of focal patchy consolidation in patients with bacterial pneumonia than in those with IFI (87.2% vs. 75.5%; P<0.05), although similar definitions were used for bacterial pneumonia and pulmonary IFI. This can be attributed to the distribution bias relating to different patient populations. Moreover, the presence of nodules has been proven to be a significant risk predictor, which has long been considered to increase the risk of IFI (19).
Radiomics is a relatively new technique and has received much attention in cancer research (20). This approach allows high-throughput quantitative extraction of numerous invisible features (21). Radiomic signatures have succeeded in a variety of tumor-related analyses (22-26) but have rarely been used to investigate infectious diseases. It is known that IFI and bacterial pneumonia are completely different in terms of angiogenesis and inflammation. The heterogeneity, therefore, can be revealed by radiomic features. In this study, we found that the radiomic features of pulmonary lesions could be used to facilitate IFI diagnosis, with an accuracy of 0.767 in the validation set. Similar to our results, a recent study used texture analysis from 970 CT imaging features and demonstrated that radiomic features could be used as noninvasive biomarkers for the differential diagnosis of primary progressive pulmonary tuberculosis from community-acquired pneumonia in children (12). This study also demonstrated that 3D radiomic features showed better reproducibility and discrimination efficacy than did the 2D features, although 3D features are more time-consuming and require heavy-load computation. This is probably because the VOIs derived from 3D segmentation can provide the entire volumetric information of the lesions and be less vulnerable to hand-related artifacts (27).
Recent research has suggested that radiomics combined with ML algorithms showed promising potential in various fields, such as the differential diagnosis of pulmonary lesions (28) as well as the prediction of hospital stays in patients with SARS-CoV-2 infection (29) and disease-specific survival outcomes (30). Compared with previous studies, more selection methods and classifiers were assessed in this work to identify the optimal model with the best diagnostic performance. Among the classifiers showing feasible discriminative ability, LR is a representative of the linear classifiers, which enables the combination of selected radiomic features into a radiomic signature (31). By contrast, SVM is a nonlinear classifier, which uses support vectors to develop a polynomic hyperplane to separate classes. In this study, LR-based models achieved satisfactory performance with the highest AUC in the cross-validation group. Nonetheless, the suitable ML methods may vary in different clinical applications. For example, Zhang et al. (32) reported that RFE with SVM showed the best performance in molecular subtype stratification of lower-grade glioma.
According to multiple logistic regression analysis, this study identified lower monocyte percentage as an independent clinical variable associated with IFI. In particular, patients with impaired cellular function of phagocytes are susceptible to fungal infection, with an incidence of up to 25% being reported in the literature (33). The clinical radiomic model achieved better predictive efficacy than did the radiomic signature or clinical features alone, with a higher AUC and net benefit in DCA. Based on our results in this prospective pilot study, the combined model performed equally well (12/15, 80.0%) in the diagnosis of pulmonary IFI to the senior radiologist and outperformed a junior radiologist in discriminating IFI and bacterial pneumonia. The constructed nomogram in our study was conveniently used to predict the individualized probability of IFI.
This study has several limitations. First, the patients were assessed retrospectively in the training and validation phase, and thus selection bias was inevitable. Second, the sample size was relatively small, which partly led to the similar diagnostic performance across most of the tested models. More samples from different independent centers are needed to validate the robustness and generalization of the prediction model. Third, the boundary delineation of the 3D lesions is time-consuming and complicated. Potential subjectivity during the process of manual segmentation may hamper reproducibility of the results. Fourth, although multifold cross-validation was performed when constructing the ML classifiers, overfitting still occurred. This computational approach requires improvement in further studies. Finally, the gold standard for investigating IFI was based on the respiratory tract specimen and not on the histologic examination undertaken in this study.
Conclusions
A noninvasive ML-based radiomic nomogram was developed through integrating CT radiomic signatures and clinical risk factors, which exhibited favorable accuracy for the differentiation of IFI and bacterial pneumonia in patients with hematologic malignancies. This quantitative radiomic diagnostic model may be useful for precision medicine and improving medical decision support.
Acknowledgments
Funding: This work was supported by the Natural Science Foundation of Guangdong Province, China (Nos. 2017A030310102, 2018030310343, and 2020B1515020008) and the Medical Scientific Research Foundation of Guangdong Province (No. A2018014). The authors also acknowledge financial support from ERC-ADG-2015 (No. 694812-Hypoximmuno) and ERC-2020-PoC (No. 957565-AUTO.DIST ERC advanced grant INCT). The authors furthermore acknowledge financial support from EUROSTARS (COMPACT-12053), the European Union’s Horizon 2020 research and innovation program (grant agreement: ImmunoSABR No. 733008), MSCA-ITN-PREDICT (No. 766276), CHAIMELEON (No. 952172), EuCanImage (No. 952103), TRANSCAN Joint Transnational Call 2016 (JTC2016 CLEARLY; No. UM 2017-8295), and Interreg V-A Euregio Meuse-Rhine (EURADIOMICS No. EMR4). This work was also supported by the Dutch Cancer Society (KWF Kankerbestrijding; No. 12085/2018-2).
Footnote
Reporting Checklist: The authors have completed the TRIPOD reporting checklist. Available at https://atm.amegroups.com/article/view/10.21037/atm-21-4980/rc
Data Sharing Statement: Available at https://atm.amegroups.com/article/view/10.21037/atm-21-4980/dss
Peer Review File: Available at https://atm.amegroups.com/article/view/10.21037/atm-21-4980/prf
Conflicts of Interest: All authors have completed the ICMJE uniform disclosure form (available at https://atm.amegroups.com/article/view/10.21037/atm-21-4980/coif). TZ and HL have associations with Philips Healthcare, and each provided technical support for data analysis. HCW owns (minority) shares in Oncoradiomics. PL reports, within and outside the submitted work, grants/sponsored research agreements from Varian Medical, Oncoradiomics, ptTheragnostic, Health Innovation Ventures, and DualTpharma. He received an advisor/presenter fee and/or reimbursement of travel costs/external grant writing fee and/or in-kind manpower contribution from Oncoradiomics, BHV, Merck, and Convert Pharmaceuticals. He owns shares in Oncoradiomics SA and Convert Pharmaceuticals SA. He is a coinventor of 2 issued patents with royalties on radiomics (PCT/NL2014/050248, PCT/NL2014/050728) licensed to Oncoradiomics, 1 issued patent on mtDNA (PCT/EP2014/059089) licensed to ptTheragnostic/DNAmito, and 3 nonpatentable inventions (software) licensed to ptTheragnostic/DNAmito, Oncoradiomics, and Health Innovation Ventures. None of the authors had control of the data in a manner that would present a conflict of interest for the other employees or consultant authors. The other authors have no conflicts of interest to declare.
Ethical Statement: The authors are accountable for all aspects of the work in ensuring that questions related to the accuracy or integrity of any part of the work are appropriately investigated and resolved. The study was conducted in accordance with the Declaration of Helsinki (as revised in 2013). The study was approved by institutional review board of Nanfang Hospital (No. NFEC-2017-087), and individual consent for this retrospective analysis was waived.
Open Access Statement: This is an Open Access article distributed in accordance with the Creative Commons Attribution-NonCommercial-NoDerivs 4.0 International License (CC BY-NC-ND 4.0), which permits the non-commercial replication and distribution of the article with the strict proviso that no changes or edits are made and the original work is properly cited (including links to both the formal publication through the relevant DOI and the license). See: https://creativecommons.org/licenses/by-nc-nd/4.0/.
References
- Miceli MH, Díaz JA, Lee SA. Emerging opportunistic yeast infections. Lancet Infect Dis 2011;11:142-51. [Crossref] [PubMed]
- Wisplinghoff H, Bischoff T, Tallent SM, et al. Nosocomial bloodstream infections in US hospitals: analysis of 24,179 cases from a prospective nationwide surveillance study. Clin Infect Dis 2004;39:309-17. [Crossref] [PubMed]
- Azoulay E, Russell L, Van de Louw A, et al. Diagnosis of severe respiratory infections in immunocompromised patients. Intensive Care Med 2020;46:298-314. [Crossref] [PubMed]
- Ullmann AJ, Aguado JM, Arikan-Akdagli S, et al. Diagnosis and management of Aspergillus diseases: executive summary of the 2017 ESCMID-ECMM-ERS guideline. Clin Microbiol Infect 2018;24:e1-e38. [Crossref] [PubMed]
- Rogers TR, Morton CO, Springer J, et al. Combined real-time PCR and galactomannan surveillance improves diagnosis of invasive aspergillosis in high risk patients with haematological malignancies. Br J Haematol 2013;161:517-24. [Crossref] [PubMed]
- Donnelly JP, Chen SC, Kauffman CARevision and Update of the Consensus Definitions of Invasive Fungal Disease From the European Organization for Research and Treatment of Cancer and the Mycoses Study Group Education and Research Consortium, et al. Clin Infect Dis 2020;71:1367-76. [Crossref] [PubMed]
- Kuhlman JE, Fishman EK, Burch PA, et al. Invasive pulmonary aspergillosis in acute leukemia. The contribution of CT to early diagnosis and aggressive management. Chest 1987;92:95-9. [Crossref] [PubMed]
- Chen W, Xiong X, Xie B, et al. Pulmonary invasive fungal disease and bacterial pneumonia: a comparative study with high-resolution CT. Am J Transl Res 2019;11:4542-51. [PubMed]
- Yan C, Xu J, Liang C, et al. Radiation Dose Reduction by Using CT with Iterative Model Reconstruction in Patients with Pulmonary Invasive Fungal Infection. Radiology 2018;288:285-92. [Crossref] [PubMed]
- Lambin P, Rios-Velazquez E, Leijenaar R, et al. Radiomics: extracting more information from medical images using advanced feature analysis. Eur J Cancer 2012;48:441-6. [Crossref] [PubMed]
- Lambin P, Leijenaar RTH, Deist TM, et al. Radiomics: the bridge between medical imaging and personalized medicine. Nat Rev Clin Oncol 2017;14:749-62. [Crossref] [PubMed]
- Wang B, Li M, Ma H, et al. Computed tomography-based predictive nomogram for differentiating primary progressive pulmonary tuberculosis from community-acquired pneumonia in children. BMC Med Imaging 2019;19:63. [Crossref] [PubMed]
- Chen B, Yang L, Zhang R, et al. Radiomics: an overview in lung cancer management-a narrative review. Ann Transl Med 2020;8:1191. [Crossref] [PubMed]
- Shen C, Liu Z, Wang Z, et al. Building CT Radiomics Based Nomogram for Preoperative Esophageal Cancer Patients Lymph Node Metastasis Prediction. Transl Oncol 2018;11:815-24. [Crossref] [PubMed]
- van Griethuysen JJM, Fedorov A, Parmar C, et al. Computational Radiomics System to Decode the Radiographic Phenotype. Cancer Res 2017;77:e104-7. [Crossref] [PubMed]
- Bow EJ. Considerations in the approach to invasive fungal infection in patients with haematological malignancies. Br J Haematol 2008;140:133-52. [Crossref] [PubMed]
- Kosmidis C, Denning DW. The clinical spectrum of pulmonary aspergillosis. Thorax 2015;70:270-7. [Crossref] [PubMed]
- Maschmeyer G, Haas A, Cornely OA. Invasive aspergillosis: epidemiology, diagnosis and management in immunocompromised patients. Drugs 2007;67:1567-601. [Crossref] [PubMed]
- Yan C, Tan X, Wei Q, et al. Lung MRI of invasive fungal infection at 3 Tesla: evaluation of five different pulse sequences and comparison with multidetector computed tomography (MDCT). Eur Radiol 2015;25:550-7. [Crossref] [PubMed]
- Gillies RJ, Kinahan PE, Hricak H. Radiomics: Images Are More than Pictures, They Are Data. Radiology 2016;278:563-77. [Crossref] [PubMed]
- Aerts HJ, Velazquez ER, Leijenaar RT, et al. Decoding tumour phenotype by noninvasive imaging using a quantitative radiomics approach. Nat Commun 2014;5:4006. [Crossref] [PubMed]
- Liu Z, Meng X, Zhang H, et al. Predicting distant metastasis and chemotherapy benefit in locally advanced rectal cancer. Nat Commun 2020;11:4308. [Crossref] [PubMed]
- Xu X, Zhang HL, Liu QP, et al. Radiomic analysis of contrast-enhanced CT predicts microvascular invasion and outcome in hepatocellular carcinoma. J Hepatol 2019;70:1133-44. [Crossref] [PubMed]
- Zhang J, Huang Z, Cao L, et al. Differentiation combined hepatocellular and cholangiocarcinoma from intrahepatic cholangiocarcinoma based on radiomics machine learning. Ann Transl Med 2020;8:119. [Crossref] [PubMed]
- Huang Y, Liu Z, He L, et al. Radiomics Signature: A Potential Biomarker for the Prediction of Disease-Free Survival in Early-Stage (I or II) Non-Small Cell Lung Cancer. Radiology 2016;281:947-57. [Crossref] [PubMed]
- Wu G, Woodruff HC, Shen J, et al. Diagnosis of Invasive Lung Adenocarcinoma Based on Chest CT Radiomic Features of Part-Solid Pulmonary Nodules: A Multicenter Study. Radiology 2020;297:451-8. [Crossref] [PubMed]
- Yang L, Yang J, Zhou X, et al. Development of a radiomics nomogram based on the 2D and 3D CT features to predict the survival of non-small cell lung cancer patients. Eur Radiol 2019;29:2196-206. [Crossref] [PubMed]
- Wang X, Wan Q, Chen H, et al. Classification of pulmonary lesion based on multiparametric MRI: utility of radiomics and comparison of machine learning methods. Eur Radiol 2020;30:4595-605. [Crossref] [PubMed]
- Yue H, Yu Q, Liu C, et al. Machine learning-based CT radiomics method for predicting hospital stay in patients with pneumonia associated with SARS-CoV-2 infection: a multicenter study. Ann Transl Med 2020;8:859. [Crossref] [PubMed]
- Yuan M, Zhang YD, Pu XH, et al. Comparison of a radiomic biomarker with volumetric analysis for decoding tumour phenotypes of lung adenocarcinoma with different disease-specific survival. Eur Radiol 2017;27:4857-65. [Crossref] [PubMed]
- Fan Y, Chen C, Zhao F, et al. Radiomics-Based Machine Learning Technology Enables Better Differentiation Between Glioblastoma and Anaplastic Oligodendroglioma. Front Oncol 2019;9:1164. [Crossref] [PubMed]
- Zhang X, Tian Q, Wang L, et al. Radiomics Strategy for Molecular Subtype Stratification of Lower-Grade Glioma: Detecting IDH and TP53 Mutations Based on Multimodal MRI. J Magn Reson Imaging 2018;48:916-26. [Crossref] [PubMed]
- Reichenberger F, Habicht JM, Gratwohl A, et al. Diagnosis and treatment of invasive pulmonary aspergillosis in neutropenic patients. Eur Respir J 2002;19:743-55. [Crossref] [PubMed]
(English Language Editors: B. Meiser and J. Gray)