Combining PET with MRI to improve predictions of progression from mild cognitive impairment to Alzheimer’s disease: an exploratory radiomic analysis study
Introduction
Alzheimer’s disease (AD) is the most common neurodegenerative disease, and mild cognitive impairment (MCI) is a high-risk prodromal stage of AD (1,2). The neuropathological substrates of MCI can be heterogeneous: some patients with MCI may not experience disease progression, some may return to normal, while others may develop AD (3,4). This study aimed to make a significant contribution to the evaluation of risk factors used to predict MCI conversion to AD.
In recent years, neuroimaging modalities have been instrumental in solidifying our understanding of the clinical diagnosis of AD. These modalities include magnetic resonance imaging (MRI) and positron emission tomography (PET), which have attracted the attention of researchers focusing on MCI and AD (5,6). MRI-based structural biomarkers that target gray matter atrophy or shape alterations are those most commonly used in the early biomarker-based detection of AD (7,8) 18F-fluorodeoxyglucose PET (18F-FDG PET) imaging is relatively sensitive and can be used to assess brain glucose metabolism in patients with MCI (7).However, the need for dual-modality or even multimodality techniques to predict MCI conversion to AD has not been conclusively supported. While multimodality imaging can provide a more accurate evaluation of most brain diseases, false-positive results may hinder the implementation of hybrid techniques in the clinical setting, and thus dual-modality imaging for the prediction of MCI conversion to AD is a topic of ongoing research that has yet to be fully evaluated (9,10).
Radiomic analysis aims to extract a large number of quantitative features from medical imaging data and establish statistical models that assist in disease diagnosis, prognosis, and treatment monitoring, thus enhancing the clinical decision-making process (11,12). In recent years, this methodology has been applied in early AD diagnosis and MCI conversion prediction (8,13-17). According to previous research, the accuracy of radiomics-based classification is relatively stable but remains at an exploratory stage. For example, although different modalities provide complementary information for MCI prediction and classification (18,19), the predictive accuracy of a multimodality technique in hybrid imaging has only been partially investigated.
The primary objective of the present study was to explore the potential of dual-modality 18F-FDG PET and MRI scans to predict MCI conversion to AD. We compared the predictive performance of both single-modality imaging techniques with a dual-modality approach. We also investigated the degree to which imaging-derived biomarkers were comparable between these modalities. We present the following article in accordance with the MDAR (Materials Design Analysis Reporting) reporting checklist (available at https://atm.amegroups.com/article/view/10.21037/atm-21-4349/rc).
Methods
The experimental design framework used in this study is outlined in Figure 1. First, structural MRI and 18F-FDG PET scans were preprocessed. Eighty cortical regions from the automated anatomical labeling (AAL) atlas were used as regions of interest (ROIs). Radiomics features were extracted and selected using single or dual modalities, and the selected features were then used to construct a Cox proportional hazards model to compare the predictive performance of the different modalities. In addition, a correlation analysis was performed to assess the correlation between the features of the 18F-FDG PET and MRI models.
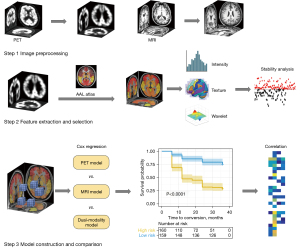
Participants and image preprocessing
Participants were recruited from 2 independent medical centers. Cohort A comprised 355 patients with MCI and 94 healthy control participants (HCs) whose data were collected from the Alzheimer’s Disease Neuroimaging Initiative (ADNI) database (http://adni.loni.usc.edu/). Cohort B comprised 22 patients with MCI whose data were collected from the Department of Neurology at the Huashan Hospital in Shanghai, China. Demographic and clinical information, including education, age, sex, Mini-Mental State Examination (MMSE) scores, time for conversion to AD, and brain scans, were collected for the two cohorts. Patients with MCI were categorized into two groups: an MCI non-converter (MCI-nc) group (n=187), which included participants whose MCI did not convert to AD during the 3-year follow-up, and an MCI converter (MCI-c) group (n=168), which included those whose MCI converted to AD within the 3-year follow-up. Participants who had a biphasic change in diagnosis during follow-up in which MCI converted to AD and returned to MCI were excluded from the analysis. Cohort A contained MRI and 18F-FDG PET scan data from the 94 HCs obtained at 2 points in time with an average interval of 2 years. These data were used to perform a stability analysis of the radiomic features. In total, 355 patients with MCI were also included in cohort A. Cohort B comprised 10 MCI-c and 12 MCI-nc participants. The participants had both MRI and 18F-FDG PET imaging data. During the follow-up period, participants in the MCI-nc group remained clinically stable, while those in the MCI-c group converted to AD during an average follow-up period of 24.5±9.6 months. Data inclusion criteria and acquisition protocol information are detailed further in the Supplementary file (Appendix 1).
Once acquired, all 18F-FDG PET and MRI scans were preprocessed as describedpreviously(17) (Figures S1,S2). This study was approved by the Research Ethics Committee of Huashan Hospital (No. KY2013-336) and was carried out in accordance with the Declaration of Helsinki (as revised in 2013). Informed consent was obtained from all participants in Cohort B or their legal guardians prior to participation.
Image preprocessing was performed using Statistical Parametric Mapping 12 (SPM 12, https://www.fil.ion.ucl.ac.uk/spm/). The original 18F-FDG PET scans were registered to corresponding structural MRI scans and corrected for the partial volume effect (PVE) with the voxel-wise Muller-Gartner method. The MRI images were segmented using a unified segmentation method. Next, the MRI images, gray matter masks, and 18F-FDG PET images were warped to the Montreal Neurological Institute (MNI) space. Finally, the normalized PET images were smoothed using an isotropic Gaussian kernel of 8 mm.
Radiomic feature extraction and selection
In the feature extraction process, 80 cortical regions from the AAL atlas were defined as the ROIs (20). A radiomics tool developed by Vallières (21) (https://github.com/mvallieres/radiomics) was used, and 430 radiomic features were extracted from each ROI for the MRI and 18F-FDG PET images. Finally, 68,800 features were extracted from the images of each participant. The extracted features included intensity and textural features, as described in the Supplementary file (Appendix 1).
Feature selection was preceded by 10-fold cross-validation, with 90% of the data included as the training dataset and 10% included as the test dataset in each fold, and by the elimination of unit restriction for each feature value through normalization to zero mean and unit standard deviation.
Feature selection was performed using the following steps: (I) feature reliability analysis; (II) statistical testing; and (III) top feature selection. The feature reliability analysis was based on the HCs from cohort A. Cronbach’s alpha coefficient was used to evaluate the stability of the features, and stable features with a coefficient greater than 0.75 were selected. Each participant in the HC group had feature sets evaluated at 2 points in time. The most discriminative features between the MCI-c and MCI-nc groups were selected for statistical testing. We used 2-sample t-tests and rank-sum tests to identify the features with significant differences between participants in the MCI-c and MCI-nc groups (P<0.01). Finally, the top features were selected, and an L1-penalized Cox model was constructed from the training dataset using least absolute shrinkage and selection operator (LASSO) regression. LASSO is a robust method that is especially suitable for the regression of high-dimensional features in a radiomic strategy, and patient features were selected based on the associations with the survival endpoints and time (22). After feature selection, a prediction model was constructed, and 10-fold cross-validation was performed with 200 repetitions in the feature selection stage.
Cox regression model construction
Our prediction model was an L1-penalized Cox regression model. Typical features were selected during the training phase and used to construct the final Cox model. Cox regression is a statistical analysis method that combines clinical outcomes and the time taken by an outcome to appear. In this study, the clinical outcome was conversion from MCI to AD. The time taken by the outcome to appear was the interval between baseline and endpoint. For each participant, the baseline was established as the date of their MRI and PET scans, and the endpoint was either the time of AD diagnosis (for the MCI-c group) or the last follow-up appointment (for the MCI-nc group).
The “glmnet” and “survival” packages (23-25) in R were used to construct the Cox model (http://www.R-project.org/). We used Harrell’s concordance index (C-index) to evaluate the model’s predictive performance. The C-index was calculated for the training and test datasets. The prognostic index (PI), a linear combination of the selected features and their coefficients, was calculated for each participant in the test dataset using the Cox model and used to calculate the C-index for that dataset. To evaluate the predictive performance of the Cox model in an unbiased manner, 10-fold cross-validation was repeated 20 times, and an average C-index (with the standard deviation values) was calculated. The number of times each feature was repeated in the model construction was counted, and those that repeated in the prediction model for more than two-thirds of the time were selected. These “conserved” features were used for further analyses.
To further compare the predictive performance of these features using single- and dual-modality PET and MRI, we calculated the PI of each participant according to the corresponding modality. Individuals were then stratified into high- and low-risk groups based on the median PI. Survival differences in the risk groups were examined using a log-rank test, and Kaplan-Meier survival curves were also plotted.
Comparison classification
To compare the predictive performances of the different modalities, a single-modality Cox model was constructed using features solely from the MRI or 18F-FDG PET scans, while a dual-modality Cox model was constructed using their combined features. The traditional PET model was constructed by calculating the average FDG standardized uptake value ratio (SUVR), and the MRI model was constructed by calculating the global gray matter volume (GMV). In addition, we used the participants’ demographic information (age, sex, number of years of education) and MMSE scores to construct a clinical Cox model to compare the effects imaging and basic clinical factors may have on the risk of MCI conversion to AD.
Correlation verification of crucial image signatures between different modalities
In this study, conserved features from the 18F-FDG PET and MRI models were considered crucial image signatures, and the relationship between the image signatures of different modalities was further explored. Correlation coefficients between the crucial PET and MRI features were calculated.
Statistical analysis
Descriptive statistics of the continuous variables are expressed as the mean ± standard deviation, and a 2-sample t-test was used to compare the differences between the two groups. A chi-squared test was used to illustrate the differences in qualitative variables. Partial correlation coefficients were used to evaluate the correlation between radiomic 18F-FDG PET and MRI features to adjust for age and sex effects. Survival differences in the different risk groups were evaluated using a log-rank test. P values were 2-tailed, and statistical significance was set at P<0.01. MATLAB 2016b (MathWorks Inc., Natick, MA, USA) was used to perform all the statistical tests.
Results
Participants
Table 1 shows the demographic and clinical details of the two cohorts. Significant differences between the MCI-c and MCI-nc groups in cohort A were observed in age (P=0.018) and MMSE scores (P<0.001), while significant differences were observed only with regard to age in cohort B (P<0.001). There were no significant differences in sex or education levels among the different groups.
Table 1
Information | MCI | HCs | |||||||||||
---|---|---|---|---|---|---|---|---|---|---|---|---|---|
MCI-c (n=168) | MCI-nc (n=187) | P value | Time_1 (n=94) | Time_2 (n=94) | P value | ||||||||
Median (IQR) | Mean (SD) | Median (IQR) | Mean (SD) | Median (IQR) | Mean (SD) | Median (IQR) | Mean (SD) | ||||||
Cohort A: ADNI | |||||||||||||
N | 168 | 187 | 94 | 94 | |||||||||
Sex (M/F) | 95/73 | 109/78 | 0.740a | 48/46 | 48/46 | 1a | |||||||
Age (years) | 74.4 (9.2) | 74.0 (7.1) | 72.3 (10.7) | 72.1 (7.5) | 0.018b* | 72.5 (8.6) | 72.8 (5.9) | 74.5 (8.6) | 74.8 (5.9) | 0.022b* | |||
Education (years) | 16.0 (4.0) | 16.0 (2.6) | 16.0 (4.0) | 16.0 (2.6) | 0.910b | 17.0 (3.0) | 16.9 (2.4) | 17.0 (3.0) | 16.9 (2.4) | 1b | |||
MMSE | 27.0 (3.0) | 26.5 (2.2) | 28.0 (2.0) | 28.0 (1.6) | <0.001b* | 30.0 (1.0) | 29.2 (1.2) | 30.0 (1.0) | 29.1 (1.3) | 0.766b | |||
MoCA | 21.0 (3.7) | 21.0 (2.8) | 21.0 (4.3) | 21.1 (2.7) | 0.870b | 26.0 (3.0) | 25.9 (2.1) | 26.0 (3.0) | 25.8 (2.0) | 0.873b | |||
ADAS-Cog 13 | 20.3 (7.7) | 20.7 (6.5) | 20.5 (6.8) | 20.9 (6.3) | 0.641b | 9.0 (6.0) | 8.6 (3.9) | 9.0 (6.0) | 8.5 (3.7) | 0.732b | |||
Conversion time (months) | 12.0 (18.0) | 14.1 (8.9) | – | – | – | ||||||||
Cohort B: Huashan Hospital | |||||||||||||
N | 10 | 12 | |||||||||||
Sex (M/F) | 6/4 | 5/7 | 0.392a | ||||||||||
Age (years) | 72.7 (7.6) | 73.5 (4.1) | 65.0 (10.0) | 64.3 (5.7) | <0.001b* | ||||||||
Education (years) | 13.5 (4.0) | 13.7 (2.3) | 12.0 (4.0) | 11.9 (2.9) | 0.132b | ||||||||
MMSE | 26.0 (1.0) | 25.5 (2.2) | 27.0 (2.0) | 26.9 (1.6) | 0.100b | ||||||||
MoCA | 22.0 (4.0) | 21.3 (3.2) | 23.0 (3.0) | 22.5 (2.4) | 0.743b | ||||||||
Conversion time (months) | 23.3 (11.9) | 24.5 (9.6) | – | – | – |
a, Chi-square; b, 2-sample t-tests; *, P<0.05. MCI, mild cognitive impairment; ADNI, Alzheimer’s Disease Neuroimaging Initiative; MCI-c, MCI converters; MCI-nc, MCI non-converters; HCs, healthy control participants; IQR, interquartile range; SD, standard deviation; MMSE, Mini-Mental State Examination; MoCA, Montreal Cognitive Assessment; ADAS-Cog 13, Alzheimer’s Disease Assessment Scale-Cognitive 13.
Conserved features in different modalities
Features included in the prediction model for more than two-thirds of the time were selected. The numbers of conserved features identified in the single-modality PET and MRI models and the dual-modality model were 13, 12, and 14, respectively. The conserved features of the 18F-FDG PET model were primarily derived from the textural features of the cingulate cortex, hippocampus, parahippocampal gyrus, precuneus, and other temporoparietal regions. For the MRI model, the conserved features were mainly derived from the hippocampus, parahippocampal gyrus, and inferior parietal lobe. Overlapping regions of the conserved features included the bilateral hippocampus and the parahippocampal gyrus, indicating that these regions showed structural changes and metabolic abnormalities.
Most of the conserved features in the dual-modality model overlapped with those in the single-modality models. The conserved features from the MRI images were mainly distributed in the inferior temporoparietal regions. The repeated appearance of these features suggested that they could effectively predict MCI conversion with excellent reproducibility. Figure 2 shows the distribution of conserved features across the brain regions in the 3 models. All conserved features from the MRI, 18F-FDG PET, and dual-modality models are listed in Table S1, with the crucial image signatures in Table S2. In addition, we have explained the meaning of these conserved features and the direction of changes in the Supplementary file (Appendix 1).
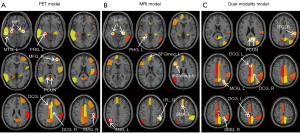
Radiomic analysis predicted MCI conversion to AD
Six prediction models, namely the SUVR_PET model, the GMV_MRI model, the radiomic PET model, the radiomic MRI model, the radiomic dual-modality model, and the clinical model, were constructed. Overall, in cohort A, image-based models (SUVR_PET, GMV_MRI, and the radiomic single- and dual-modality models) were superior to the clinical model, while the radiomic dual-modality model was superior to the radiomic single-modality imaging models. Conversely, the GMV_MRI model and SUVR_PET model had similar predictive performances. Similar results were also found in cohort B. Table 2 summarizes the evaluations of predictive performance for each model.
Table 2
Model | Cohort A training | Cohort A testing | Cohort B |
---|---|---|---|
Clinical | 0.692 (0.0004) | 0.684 (0.006) | 0.685 (0.006) |
SUVR_PET | 0.791 (0.002) | 0.751 (0.007) | 0.700 (0.004) |
GMV_MRI | 0.803 (0.003) | 0.749 (0.008) | 0.702 (0.007) |
Radiomic PET | 0.871 (0.004) | 0.753 (0.008) | 0.734 (0.011) |
Radiomic MRI | 0.807 (0.004) | 0.741 (0.007) | 0.760 (0.009) |
Radiomic dual-modality | 0.884 (0.004) | 0.766 (0.009) | 0.798 (0.008) |
C-indices are expressed as the mean (standard deviation) as derived from multiple 10-fold cross-validation in each model. SUVR, standardized uptake value ratio; PET, positron emission tomography; GMV, gray matter volume; MRI, magnetic resonance imaging.
As a result, some of the conserved features from the 18F-FDG PET and MRI models overlapped in the hippocampus and parahippocampal gyrus. To further evaluate the predictive performance of these crucial image signatures, they were used as predictors to construct an independent conventional Cox model for each of the following imaging modalities. Four crucial image signatures distributed in the hippocampus and parahippocampal gyrus on the 18F-FDG PET images were included, as were four crucial image signatures from the MRI images (Table S2). The results indicated that these conserved features could effectively predict the risk of conversion to AD in individuals with MCI.
Survival differences in the low-risk and the high-risk groups were significant in the training and test datasets (log-rank tests, P<0.01). Figure 3 shows the characteristics of each Cox model and the corresponding Kaplan-Meier survival curves. The Akaike information criterion (AIC) test was used to evaluate the quality of the Cox models (radiomic PET: 1,584.59; radiomic MRI: 1,603.48; dual-modality: 1,572.27). For the validation dataset (cohort B), we evaluated whether the risk groups were better classified according to the PI derived from each model. The 3 models demonstrated good performance in differentiating between the groups with high and low risks of conversion to AD (PET model: P<0.001; MRI model: P=0.007; dual-modality model: P<0.001).
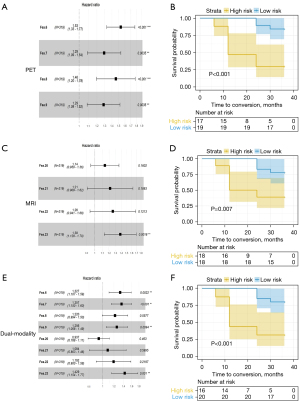
Correlation between different model feature signatures
In this study, we obtained 13 conserved features in the radiomic PET model [Table S1 (a)] and 12 conserved features in the MRI model [Table S1 (b)]. We calculated the correlation coefficients and obtained a 13×12 correlation matrix. Of these paired correlation matrices, 83 showed significant correlations in cohort A (P<0.001; Figure 4A), and 4 showed significant correlations in cohort B (P<0.001; Figure 4B). Among them, there were 4 pairs of features that were related in all cohorts and modalities, as shown in the red box in Figure 4. On the 18F-FDG PET images, these features were in the parahippocampal (busyness), cingulum_mid [zone percentage (ZP)], large zone low gray-level emphasis (LZLGE), and cingulum_mid [large zone emphasis (LZE)]. On the MRI images, they were in the angular gyrus [run percentage (RP)], hippocampus (coarseness), and olfactory cortex (coarseness).
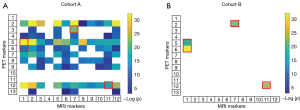
Discussion
This study used radiomic analysis to compare the capabilities of single-modality MRI and 18F-FDG PET and dual-modality MRI to predict the MCI conversion to AD. One strength of this study was that we used two separate cohorts from Western (ADNI) and Chinese (Huashan) populations. Although the sample size of the Huashan dataset was somewhat small as an external test dataset whose inclusion might have therefore led to overfitting and inconsistent results among the different modalities, our findings verified the feature stability across different ethnic cohorts.
We showed that dual-modality imaging is useful but not necessary for the prediction of MCI conversion to AD. We constructed 6 LASSO models based on the sources of the features. Image-based prediction models showed superior performance to the clinical model. Notably, the radiomic PET/MRI models had better predictive performance than did the traditional PET/MRI models. This may be due to the lower sensitivity and higher subjectivity of the neuropsychological scales (26).The C-indices of the dual-modality model constructed using data from PET and MRI images were 0.766 for the ADNI participants (cohort A) and 0.798 for the Huashan participants (cohort B) (Table 2). These results demonstrate the reliability of the radiomic models for predicting MCI conversion to AD. However, comparison of the single- and dual-modality MRI and PET models revealed that MRI outperformed PET in cohort B (C-indices of 0.760 and 0.734, respectively; P<0.001), while the dual-modality model resulted in only a modest improvement over the single-modality models (C-index of 0.798 for dual-modality vs. 0.760 for MRI, and 0.734 for PET; both P<0.001). The cohort A test dataset also showed a modest improvement with the dual-modality model, with PET marginally outperforming MRI in the single-modality models (Table 2). The small discrepancies between these results may be attributable to ethnic differences between the Western and Chinese study populations or the uncertainty in the C-index values of cohort B because of the small sample size. Larger studies are required, and no clear recommendation can be made regarding the choice of which single-modality imaging technique to use. Nevertheless, our findings suggest only a modest improvement in predictive capacity can be expected when dual-modality imaging is performed.
Consistent with our hypothesis, most of the conserved features were identified in regions significantly associated with AD, specifically, the medial temporal areas, inferior parietal lobe, precuneus, and cingulate gyrus, which are all brain regions that experience early pathological protein (amyloid-β and hyperphosphorylated tau) deposition (27). They also experience early atrophy, thickness reduction, and metabolic reduction (28-30). Our correlation analyses revealed that some features from different modalities have obvious correlations, indicating the importance of certain regions in the early diagnosis of AD. For example, there was a correlation between the hippocampal features in the 2 modalities, and this region was also correlated with other areas, including the precuneus and the medial cingulate gyrus, which are typical areas of interest in patients with AD.
A recent multicenter study suggested that the hippocampal radiomic features can serve as robust biomarkers for clinical application in predicting MCI conversion to AD (8). The brain regions identified in the present study are in concordance with the regions associated with AD development (13,14,31,32).Several studies have described changes in the hippocampal function as having an impact on the cingulate and precuneus gyrus (33,34). Our study showed a correlation between the textural features of the two regions, indicative of a corresponding pathological correlation. As a result, textural features extracted from these regions were more useful in differentiating between the MCI-c and MCI-nc groups. However, the large degree of overlap identified between the two modalities suggests a large degree of redundancy, with dual-modality imaging providing only a slight gain in information. This fact and the minor improvements in predictive ability indicate that only limited benefits can be expected from dual-modality PET and MRI imaging. We believe that in resource-poor settings, where patients do not have access to more than 1 imaging modality, or in cases of contraindication or concern about the additional radiation burden encountered in nuclear medicine imaging, single-modality imaging would be acceptable.
This study had some limitations. First, we only used radiomic analysis to verify whether dual-modality imaging is necessary for predicting MCI conversion to AD. Exploration of other methodologies is required to confirm our findings. Second, the radiomic method indicated individual differences between the features. Although the stability of the radiomic features was studied using HCs, the stability of the radiomic features in patients with MCI could be the topic of an ongoing study. Third, the C-index values of cohort B may contain uncertainty in the external test dataset owing to the small sample size. Research must be furthered using external test data with a larger sample size and greater heterogeneity to confirm our findings. Lastly, considering that the ADNI data were used as the training dataset, differences in ethnicity between the training and testing groups should not be ignored.
Conclusions
Comparison of a radiomic model for the prediction of MCI conversion to AD identified a large overlap between 18F-FDG PET and MRI, with much redundancy in dual-modality imaging. The present study showed that the incremental benefit of combining 18F-FDG PET and MRI was limited and that in radiomic models for predicting MCI conversion, single-modality imaging may be sufficient.
Acknowledgments
Funding: This article was supported by grants from the National Natural Science Foundation of China (Nos. 61603236, 81830059, 81971641, 81671239, and 81361120393), the Shanghai Municipal Science and Technology Major Project (Nos. 2017SHZDZX01 and 2018SHZDZX03), and the 111 Project (No. D20031). Data collection and sharing for this project were funded by the Alzheimer’s Disease Neuroimaging Initiative (ADNI; National Institutes of Health Grant U01 AG024904) and DODADNI (Department of Defense; No. W81XWH-12-2-0012). ADNI is funded by the National Institute of Aging and the National Institute of Biomedical Imaging and Bioengineering and through generous contributions from the following: AbbVie, Alzheimer’s Association; Alzheimer’s Drug Discovery Foundation; Araclon Biotech; BioClinica, Inc.; Biogen; Bristol-Myers Squibb Company; CereSpir, Inc.; Eisai, Inc.; Elan Pharmaceuticals, Inc.; Eli Lilly and Company; EuroImmun; F.Hoffmann-La Roche Ltd. and its affiliated company Genentech, Inc.; Fujirebio; GE Healthcare; IXICO Ltd.; Janssen Alzheimer Immunotherapy Research & Development, LLC.; Johnson & Johnson Pharmaceutical Research & Development LLC.; Lumosity; Lundbeck; Merck & Co., Inc.; Meso Scale Diagnostics, LLC.; NeuroRx Research; Neurotrack Technologies; Novartis Pharmaceuticals Corporation; Pfizer, Inc.; Piramal Imaging; Servier; Takeda Pharmaceutical Company; and Transition Therapeutics. The Canadian Institutes of Health Research is providing funds to support ADNI clinical sites in Canada. Private sector contributions are facilitated by the Foundation for the National Institutes of Health (www.fnih.org). The grantee organization is the Northern California Institute for Research and Education, and the study is coordinated by the Alzheimer’s Disease Cooperative Study at the University of California, San Diego, CA, USA. ADNI data are disseminated by the Laboratory for Neuro Imaging at the University of Southern California, CA, USA.
Footnote
Reporting Checklist: The authors have completed the MDAR reporting checklist. Available at https://atm.amegroups.com/article/view/10.21037/atm-21-4349/rc
Data Sharing Statement: Available at https://atm.amegroups.com/article/view/10.21037/atm-21-4349/dss
Peer Review File: Available at https://atm.amegroups.com/article/view/10.21037/atm-21-4349/prf
Conflicts of Interest: All authors have completed the ICMJE uniform disclosure form (available at https://atm.amegroups.com/article/view/10.21037/atm-21-4349/coif). The authors have no conflicts of interest to declare.
Ethical Statement: The authors are accountable for all aspects of the work in ensuring that questions related to the accuracy or integrity of any part of the work are appropriately investigated and resolved. This study was approved by the Research Ethics Committee of Huashan Hospital (No. KY2013-336) and was carried out in accordance with the Declaration of Helsinki (as revised in 2013). Informed consent was obtained from all participants in Cohort B or their legal guardians prior to participation.
Open Access Statement: This is an Open Access article distributed in accordance with the Creative Commons Attribution-NonCommercial-NoDerivs 4.0 International License (CC BY-NC-ND 4.0), which permits the non-commercial replication and distribution of the article with the strict proviso that no changes or edits are made and the original work is properly cited (including links to both the formal publication through the relevant DOI and the license). See: https://creativecommons.org/licenses/by-nc-nd/4.0/.
References
- Petersen RC, Doody R, Kurz A, et al. Current concepts in mild cognitive impairment. Arch Neurol 2001;58:1985-92. [Crossref] [PubMed]
- Li JQ, Tan L, Wang HF, et al. Risk factors for predicting progression from mild cognitive impairment to Alzheimer's disease: a systematic review and meta-analysis of cohort studies. J Neurol Neurosurg Psychiatry 2016;87:476-84. [Crossref] [PubMed]
- Petersen RC, Roberts RO, Knopman DS, et al. Mild cognitive impairment: ten years later. Arch Neurol 2009;66:1447-55. [Crossref] [PubMed]
- Schneider JA, Arvanitakis Z, Leurgans SE, et al. The neuropathology of probable Alzheimer disease and mild cognitive impairment. Ann Neurol 2009;66:200-8. [Crossref] [PubMed]
- Sanchez-Catasus CA, Stormezand GN, van Laar PJ, et al. FDG-PET for Prediction of AD Dementia in Mild Cognitive Impairment. A Review of the State of the Art with Particular Emphasis on the Comparison with Other Neuroimaging Modalities (MRI and Perfusion SPECT). Curr Alzheimer Res 2017;14:127-42. [Crossref] [PubMed]
- Blazhenets G, Ma Y, Sörensen A, et al. Principal Components Analysis of Brain Metabolism Predicts Development of Alzheimer Dementia. J Nucl Med 2019;60:837-43. [Crossref] [PubMed]
- Ten Kate M, Ingala S, Schwarz AJ, et al. Secondary prevention of Alzheimer's dementia: neuroimaging contributions. Alzheimers Res Ther 2018;10:112. [Crossref] [PubMed]
- Zhao K, Ding Y, Han Y, et al. Independent and reproducible hippocampal radiomic biomarkers for multisite Alzheimer’s disease: diagnosis, longitudinal progress and biological basis. Science Bulletin 2020;65:1103-13. [Crossref]
- Suk HI, Shen D, editors. Deep Learning-Based Feature Representation for AD/MCI Classification 2013; Berlin, Heidelberg: Springer Berlin Heidelberg.
- Hojjati SH, Ebrahimzadeh A, Khazaee A, et al. Predicting conversion from MCI to AD by integrating rs-fMRI and structural MRI. Comput Biol Med 2018;102:30-9. [Crossref] [PubMed]
- Lambin P, Leijenaar RTH, Deist TM, et al. Radiomics: the bridge between medical imaging and personalized medicine. Nat Rev Clin Oncol 2017;14:749-62. [Crossref] [PubMed]
- Rizzo S, Botta F, Raimondi S, et al. Radiomics: the facts and the challenges of image analysis. Eur Radiol Exp 2018;2:36. [Crossref] [PubMed]
- Feng F, Wang P, Zhao K, et al. Radiomic features of hippocampal subregions in Alzheimer's disease and amnestic mild cognitive impairment. Front Aging Neurosci 2018;10:290. [Crossref] [PubMed]
- Feng Q, Chen Y, Liao Z, et al. Corpus Callosum Radiomics-Based Classification Model in Alzheimer's Disease: A Case-Control Study. Front Neurol 2018;9:618. [Crossref] [PubMed]
- Feng Q, Song Q, Wang M, et al. Hippocampus Radiomic Biomarkers for the Diagnosis of Amnestic Mild Cognitive Impairment: A Machine Learning Method. Front Aging Neurosci 2019;11:323. [Crossref] [PubMed]
- Feng Q, Wang M, Song Q, et al. Correlation between hippocampus MRI radiomic features and resting-state intrahippocampal functional connectivity in Alzheimer's disease. Front Neurosci 2019;13:435. [Crossref] [PubMed]
- Li Y, Jiang J, Lu J, et al. Radiomics: a novel feature extraction method for brain neuron degeneration disease using 18F-FDG PET imaging and its implementation for Alzheimer's disease and mild cognitive impairment. Ther Adv Neurol Disord 2019;12:1756286419838682. [Crossref] [PubMed]
- Wang P, Chen K, Yao L, et al. Multimodal Classification of Mild Cognitive Impairment Based on Partial Least Squares. J Alzheimers Dis 2016;54:359-71. [Crossref] [PubMed]
- Liu K, Chen K, Yao L, et al. Prediction of Mild Cognitive Impairment Conversion Using a Combination of Independent Component Analysis and the Cox Model. Front Hum Neurosci 2017;11:33. [Crossref] [PubMed]
- Seitzman BA, Gratton C, Marek S, et al. A set of functionally-defined brain regions with improved representation of the subcortex and cerebellum. Neuroimage 2020;206:116290. [Crossref] [PubMed]
- Vallières M, Freeman CR, Skamene SR, et al. A radiomics model from joint FDG-PET and MRI texture features for the prediction of lung metastases in soft-tissue sarcomas of the extremities. Phys Med Biol 2015;60:5471-96. [Crossref] [PubMed]
- Gui J, Li H. Penalized Cox regression analysis in the high-dimensional and low-sample size settings, with applications to microarray gene expression data. Bioinformatics 2005;21:3001-8. [Crossref] [PubMed]
- Simon N, Friedman J, Hastie T, et al. Regularization Paths for Cox's Proportional Hazards Model via Coordinate Descent. J Stat Softw 2011;39:1-13. [Crossref] [PubMed]
- Friedman J, Hastie T, Tibshirani R. Regularization Paths for Generalized Linear Models via Coordinate Descent. J Stat Softw 2010;33:1-22. [Crossref] [PubMed]
- Therneau TM, Grambsch PM. Modeling Survival Data: Extending the Cox Model. Modeling Survival Data: Extending the Cox Model, 2013.
- Huang K, Lin Y, Yang L, et al. A multipredictor model to predict the conversion of mild cognitive impairment to Alzheimer's disease by using a predictive nomogram. Neuropsychopharmacology 2020;45:358-66. [Crossref] [PubMed]
- Long JM, Holtzman DM. Alzheimer Disease: An Update on Pathobiology and Treatment Strategies. Cell 2019;179:312-39. [Crossref] [PubMed]
- Kato T, Inui Y, Nakamura A, et al. Brain fluorodeoxyglucose (FDG) PET in dementia. Ageing Res Rev 2016;30:73-84. [Crossref] [PubMed]
- Pini L, Pievani M, Bocchetta M, et al. Brain atrophy in Alzheimer's Disease and aging. Ageing Res Rev 2016;30:25-48. [Crossref] [PubMed]
- Risacher SL, Saykin AJ. Neuroimaging in aging and neurologic diseases. Handb Clin Neurol 2019;167:191-227. [Crossref] [PubMed]
- Bailly M, Destrieux C, Hommet C, et al. Precuneus and Cingulate Cortex Atrophy and Hypometabolism in Patients with Alzheimer's Disease and Mild Cognitive Impairment: MRI and (18)F-FDG PET Quantitative Analysis Using FreeSurfer. Biomed Res Int 2015;2015:583931. [Crossref] [PubMed]
- Zhou H, Jiang J, Lu J, et al. Dual-Model Radiomic Biomarkers Predict Development of Mild Cognitive Impairment Progression to Alzheimer's Disease. Front Neurosci 2019;12:1045. [Crossref] [PubMed]
- Papma JM, Smits M, de Groot M, et al. The effect of hippocampal function, volume and connectivity on posterior cingulate cortex functioning during episodic memory fMRI in mild cognitive impairment. Eur Radiol 2017;27:3716-24. [Crossref] [PubMed]
- Xue J, Guo H, Gao Y, et al. Altered directed functional connectivity of the hippocampus in mild cognitive impairment and Alzheimer's disease: a resting-state fMRI study. Front Aging Neurosci 2019;11:326. [Crossref] [PubMed]
(English Language Editors: L. Roberts and J. Gray)