Identification of molecular subtypes premised on the characteristics of immune infiltration of endometrial cancer
Introduction
Uterine corpus cancer has been ranked as the third commonly occurring gynecologic malignancy in the world, recording approximately 417,000 new incidents and contributing to 97,000 deaths in 2020 alone (1), with uterine corpus endometrial carcinoma (UCEC) accounting for the highest number of cases. UCEC is marked by considerable molecular variability and infiltration by a diverse population of stromal and immune cells (2,3). Despite improvements in radiotherapy, chemotherapy, and surgery, patients with recurrent or advanced endometrial cancer (EC) have a dismal prognosis. Targeted therapies are promising for advanced endometrial malignancies, particularly tumor progression after receiving standard treatment (4,5). Immunotherapy has good antineoplastic activity (6-8), but many questions remain about its safety and effectiveness. Different treatments are a new subject for medical research, but studies of treatment efficacy are limited by non-random sample populations. Hence, it is critical to research patients’ general immunological condition, to detect molecular subtypes of cancer, and to the enhance treatment efficacy of EC.
Rather than operating in isolation, cancer cells create their own tumor microenvironment (TME) via intimate interactions with stromal cells and the extracellular matrix (ECM) (9). Tumor-associated macrophages (TAMs) may be a valuable therapeutic target for EC. For instance, estrogen receptors in macrophages promote the invasion of EC cells by activating the extracellular signal-regulated kinase 1/2 pathway (10), and highly malignant and invasive tumors show increased expression of Ki-67 and p53 (11). PD-L1 specifically is found in 70–80% of malignant endometrial cells, as well as all instances of uterine sarcoma, indicating that PD-1/PD-L interactions might be an effectives target for immunotherapy in EC (12,13). Recently, a focus on molecular classification of EC and establishing molecular subtypes predicated on the mutational or transcriptomic landscape (14,15), coupled with the fact that the TME can cause drug resistance, highlighted significantly more complicated illness contrary to what was previously acknowledged. Studies have shown that immune cell infiltration (ICI) is associated with the development of various cancers (16-18), however, the vast majority of studies currently have focused on the mechanism of ICI involved in the occurrence and development of endometrial cancer. In this study, we focused the ICI landscape of EC rather than a specific ICI.
The development of genome-sequencing methods along with genomic databases has enabled the identification of a large number of tumor biomarkers. Using The Cancer Genome Atlas (TCGA) database (https://portal.gdc.cancer.gov/), we identified immune-related genes with promising prognostic significance for EC and categorized EC into five separate subtypes predicated on the infiltration patterns of immune cells. In addition, we also studied the relationship between ICI scores and clinical prognosis, tumor mutant genes, and immune gene expression, which could assist precision medical treatment, and provide clinical decision support. We present the following article in accordance with the STREGA reporting checklist (available at https://atm.amegroups.com/article/view/10.21037/atm-22-301/rc).
Methods
Data collection and preprocessing
The latest RNA-seq data along with accompanying clinical data were downloaded from the TCGA database. A total of 538 tumor specimens were preprocessed as follows: (I) removal of specimens lacking clinical data; (II) removal of samples without overall survival (OS) and status data; (III) include the probe that corresponds to multiple genes that were removed; (IV) remaining probes translated into gene symbols; and (V) expression of multiple gene symbols presented as median value. Table 1 presents a summary of the clinical information. The study was conducted in accordance with the Declaration of Helsinki (as revised in 2013).
Table 1
Survival | Status | TCGA-UCEC |
---|---|---|
OS | Status 0 | 448 |
Status 1 | 90 | |
DSS | Status 0 | 476 |
Status 1 | 60 | |
Unknown | 2 | |
DFI | Status 0 | 364 |
Status 1 | 55 | |
Unknown | 119 | |
PFI | Status 0 | 416 |
Status 1 | 122 | |
Age | >65 | 234 |
≤65 | 301 | |
Unknown | 3 | |
Stage | Stage I | 335 |
Stage II | 50 | |
Stage III | 124 | |
Stage IV | 29 |
EC, endometrial cancer; TCGA, The Cancer Genome Atlas; UCEC, uterine corpus endometrial carcinoma; OS, overall survival; DSS, disease specific survival; DFI, disease-free survival; PFI, progression-free interval.
Calculation of scores of tumor-infiltrating immune cells (TIICs)
A total of 22 different types of human immune cells were studied for their infiltration levels in EC utilizing the “CIBERSORT” R program (https://cibersort.stanford.edu/index.php) and the LM22 signature with 1,000 permutations. ESTIMATE assessed the purity of stromal and immune cells per sample (19). The “ConcensusClusterPlus” package was used to identify UCEC subgroups premised on the characteristics of TIICs (20). For classification stability, the process was done 1,000 times, and the results revealed two distinct ICI subtypes with significantly different survival rates.
Identification of differentially expressed genes related to the immunophenoscore (IPS_DEGs)
The IPS is calculated using a 0–10 scale depending on the gene expression z-scores of representative cell types, whereby higher scores are linked to higher immunogenicity (21). The Cancer Immunome Atlas (https://tcia.at/home) was used to retrieve EC patients’ IPS and to calculate the best threshold value based on the gradient density between the IPS and OS of the EC patients. Finally, the optimal threshold was set at an IPS score of 7.75. The grouping of the patients into low- and high-score groups was done via the “Survminer” R package. Finally, we utilized the “limma” package to examine the IPS_DEGs between distinct performance groups.
Calculation of the ICI score and dimension reduction
The “ConsensusClusterPlus” package of R software categorized the IPS_DEGs to acquire reliable ICI gene signatures. Specifically, positive correlations between IPS_DEGs and the gene cluster resulted in the designation of an “ICI Gene Signature A”, and the remainder was designated as an “ICI Gene Signature B”. Utilizing R software “ClusterProfiler”, functional annotation of the prognostic ICI gene signatures was carried out to gain insights into their bio-functions. Enrichment analysis based on gene ontology (GO) terms was categorized into three types: cellular components, molecular functions, and biological processes (BP). Subsequently, the dimension in the above ICI genes with different signatures was reduced utilizing the Boruta algorithm (22). In addition, principal-component analysis (PCA) was performed on the signature score from ICI gene signatures A and B. The following is the signature score equation for the ICI gene in the sample:
Somatic variants data analysis
The TCGA database was also used to retrieve EC patients’ mutation data. In this research, we computed the tumor mutation burden (TMB), which is the sum of each sample’s nonsynonymous mutations. Mutation status was evaluated and displayed by the “maftools” package (23). The somatic mutations in the EC driver genes were examined in order to determine if they had lower or higher ICI scores. We then merged the TMB data with the clinical information and evaluated the top-ranked 30 driver genes that had the greatest mutation frequency.
Association between ICI score and clinical application
Using the IMvigor210CoreBiologies package (http://research-pub.gene.com/IMvigor210CoreBiologies/), the microarray data of the IMvigor210 study were retrieved. IMvigor210 was selected for external validation because it comprised patients with metastatic urothelial cancer who had undergone immunotherapy (anti-PD-L1 agent, specifically, atezolizumab) (24). We constructed the ICI score signature, similarly as for the GSE78220 cohort that was retrieved from the GEO database, and our model was able to substantially differentiate prognosis between low- and high-ICI scores in an external independent cohort.
Other statistical methods
In this study, the Wilcoxon test and the Kruskal-Wallis test were used to contrast two or multiple groups. The chi-square test was utilized for the connection between the ICI score groupings and the frequency of somatic mutations, and the Spearman correlation coefficient was calculated using Spearman analysis. For the purpose of comparing the OS rates of EC patients with various molecular subtypes, the log-rank and Kaplan-Meier tests were conducted. A two-sided P value <0.05 was deemed significant.
Results
Identification of different TIICs involved in EC
We measured the proportions of 22 different TME-tumor-infiltrating lymphocytes (TILs) in 538 EC patients who had a CIBERSORT P<0.05 (https://cdn.amegroups.cn/static/public/10.21037atm-22-301-1.xlsx). In the TCGA-UCEC cohort, the scores for immune, stromal, and ESTIMATE were utilized to determine the distribution of ICI (https://cdn.amegroups.cn/static/public/10.21037atm-22-301-2.xlsx). With respect to the TIICs characteristics, unsupervised cluster analysis of the TCGA-UCEC cohort showed high similarity within groups, but the low similarity between groups whenever k=2. Equally significant, the survival time was markedly diverse. Therefore, we selected k=2 as the ideal number of clusters and obtained two molecular subtypes (Figure 1).
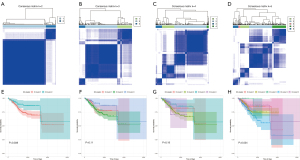
The TILs subgroups exhibited substantially varied levels of distinct EC immunogenomic subtypes (Figure 2A), and a considerable variation was discovered in prognosis between the two subtype samples (P=0.038, log-rank test, Figure 2B). The correlation coefficient heat-map reflected precise communication modes among the immune cells in the TME (Figure 2C). Moreover, we discovered that patients in ICI cluster II possessed greater concentrations of TILs, which included resting natural killer (NK) cells, activated memory CD4 T cells, T regulatory cells, CD8 T cells, and activated NK cells (Figure 2D). In a similar manner, we computed the variation in PD1 and PD-L1 expression levels between the two ICI subtypes, which revealed that ICI cluster II exhibited greater levels of PD1 and PD-L1 expression, whereas ICI cluster I exhibited reduced levels (Figure 2E,2F).
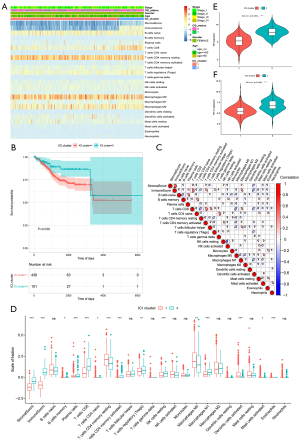
Identifying DEGs associated with IPS (IPS_DEGs)
The IPS is a novel approach based on RNA-seq for the prediction of responders to immune checkpoint inhibitor treatment in melanoma patients (21). It ascertains the optimum threshold based on the distribution of IPS scoring systems with related OS calculated during the grouping process. We then divided the EC samples into low- and high-score groups via the optimal threshold (value =7.75) (Figure 3A,3B). There was an OS-related substantial variation between the low- and high-score groups (Figure 3C).
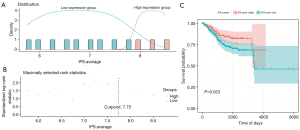
Identification of five immune molecular subtypes of EC
We conducted differential analyses to screen out the DEGs in both groups, and predicated on the |log2 (fold change)| >1 and adjusted P value <0.05, an aggregate of 508 DEGs were discovered (https://cdn.amegroups.cn/static/public/10.21037atm-22-301-3.xlsx): 8 of these genes were up-modulated in the low-score group and 500 were up-modulated in the high-score group.
We conducted unsupervised clustering of the 508 DEGs, which was obtained by subsequent observation of the heat-map and Kaplan-Meier plotter (Figure 4A-4J). The clustering results were stable when k=5, so five molecular subtypes were obtained.
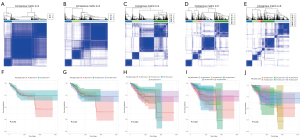
The TCGA cohort was classified into five genomic clusters, designated as gene cluster 1 (GenC1), gene cluster 2 (GenC2), gene cluster 3 (GenC3), gene cluster 4 (GenC4), and gene cluster 5 (GenC5). The GeneType A designation was given to the 275 DEGs that were shown to be positively connected to the gene cluster, whereas the GeneType B designation was given to the remaining 233 DEGs.
The “ClusterProfiler” package in R was used to perform cluster analysis of gene expression (Figure 5A) (25). Survival analysis showed significant differences between different gene clusters (P=0.021; log-rank test; Figure 5B). The BPs that were highly enriched are shown in Figure 5C,5D. It can be seen that the majority of the enriched pathways were associated with immunobiological activities.
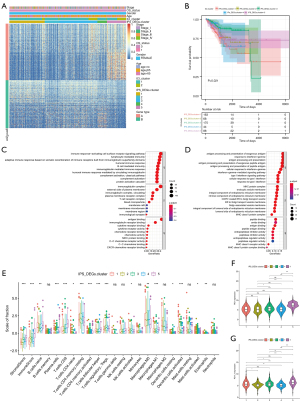
To analyze the relationship between the five gene clusters and immunity, we estimated the ICI scores in the five ICI gene clusters. The findings showed that the stromal and immune scores of GenC5 were significantly higher than those for the other gene clusters, and CD8 T cells, plasma cells, and naive B cells scores in GenC5 were also markedly greater than in the other clusters (Figure 5E). Similarly, the expression levels of PD1/PD-L1 in the gene clusters showed significant differences, as shown in Figure 5F,5G.
Establishment of the ICI score
An ICI score model predicated on the GeneType A and GeneType B immune gene subtypes was developed for quantification of ICI in tumors via gene expression. First, we needed to reduce noise or redundant genes and further used the Boruta algorithm to minimize the size of GeneType A and B. Following this reduction, 138 genes were retained as factors for model construction, of which 71 belonged to GeneType A and 67 belonged to GeneType B (https://cdn.amegroups.cn/static/public/10.21037atm-22-301-4.xlsx). Finally, we used PCA to compute the expression profiles of the 138 characteristic genes: (I) the ICI score A-derived ICI signature gene A; and (II) the ICI score B-derived ICI signature gene B. We calculated the optimal density gradient threshold value to determine the ICI scores that OS was most related to, and a score of −6.14 was set as the cut-off value. The TCGA-UCEC cohort was categorized according to the best threshold value: those with high- or low-ICI scores. As shown in Figure 6A-6C, the difference between the two groups was significant.
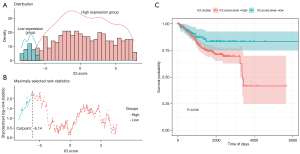
Figure 7A depicts the patients’ distribution across the five gene clusters. The immune checkpoints expressions, including PD-L1 or PD-1, are often used to predict immunotherapeutic benefits in multiple malignancies. To examine this, we began by selecting immune checkpoint-related candidate genes, including PDCD1 (PD-1), IDO1, HAVCR2, CTLA4, LAG3, CD274 (PD-L1), and immune-activity-related signatures, including TNF, CXCL9, PRF1, GZMB, GZMA, IFNG, CXCL10, TBX2 and CD8A, to assess their relationships with our ICI scores. We found that these immune checkpoints and immune-activity-relevant genes were all up-modulated in the high ICI score group with the exception of TNF, TBX2, and IDO1 (Figure 7B). Moreover, the gene set enrichment analysis showed that the T cell receptor, B cell receptor, and NK cell-mediated cytotoxicity signaling pathways were highly enriched in the high ICI score group (Figure 7C).
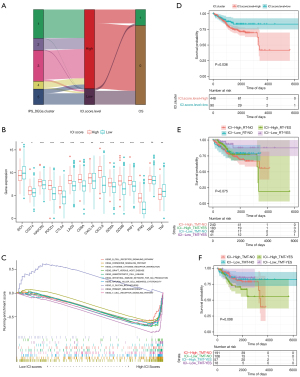
Kaplan-Meier survival analysis demonstrated that patients with a low-ICI score had a considerably longer OS as opposed to those with a high-ICI score (log-rank test, P=0.036, Figure 7D). Additionally, we evaluated the influence of adjuvant treatment on the prognosis per each subgroup of ICI. The results of survival analysis among patients who received adjuvant therapy were similar to the above (Figure 7E,7F).
Relationship between somatic mutations and ICI scores
Due to the TMB’s reflection of the total neo-antigen load, it shows great promise as a prognostic biomarker for immunotherapy (26,27). Hence, we evaluated patients’ TMB in the low- and high-ICI score subgroups with somatic mutation data and determined their distribution. TMB was substantially greater in the low-ICI score subgroup compared with the high-ICI score cohort (Figure 8A). Although the results were not statistically significant in the model, we could clearly see a negative link between ICI score and TMB (Spearman coefficient: R=–0.32, Figure 8B). TMB was associated with good OS and low-ICI score group patients had elevated TMB levels (log-rank test, P=0.0011, Figure 8C). Following that, we conducted a stratified survival study to determine the prospective clinical value of ICI scores in prognosis classification (Figure 8D), and the ICI score subsets demonstrated considerable survival variance in both the low- and high-TMB subgroups, which could be a potential independent indicator and increase the predicted accuracy for OS.
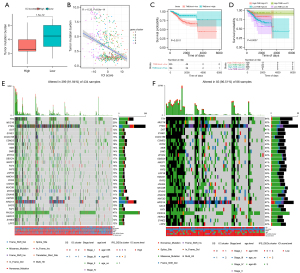
The top 30 highly mutated driver genes were chosen to ascertain the distribution of mutation predicated on ICI score; TTN, MUC16, DST, SYNE1, CCDC168, CSMD3, RYR2, NEB, DMD, ZFHX4, OBSCN, MACF1, FAT4, FAT3, LRP-1B, HMCN1, RYR3, USH2A, MUC5B, ZFHX3, DNAH8, KMT2D, ARID1A, SACS, FAT1, HERC2, SYNE2, and LRP2 were always mutated and acted as drivers in EC (Figure 8E,8F). Our analyses suggest a novel perspective for the exploration of the mechanism of TIICs in immunotherapy and the relationship with tumor mutation (Table 2).
Table 2
Gene symbol | High ICi score (n=434) | Low ICI score (n=86) | P value | |||
---|---|---|---|---|---|---|
n | % | n | % | |||
TTN | 143 | 33 | 55 | 64 | 1.00E-03 | |
MUC16 | 87 | 20 | 45 | 52 | 1.00E-05 | |
PTEN | 265 | 61 | 69 | 80 | 2.00E-01 | |
DST | 56 | 13 | 35 | 41 | 3.00E-06 | |
SYNE1 | 65 | 15 | 37 | 43 | 9.00E-06 | |
CCDC168 | 56 | 13 | 33 | 38 | 1.00E-05 | |
CSMD3 | 82 | 19 | 37 | 43 | 5.00E-04 | |
RYR2 | 78 | 18 | 34 | 40 | 1.00E-03 | |
NEB | 61 | 14 | 39 | 45 | 6.00E-07 | |
DMD | 61 | 14 | 37 | 43 | 3.00E-06 | |
ZFHX4 | 65 | 15 | 38 | 44 | 4.00E-06 | |
OBSCN | 74 | 17 | 39 | 45 | 2.00E-05 | |
MACF1 | 61 | 14 | 35 | 41 | 1.00E-05 | |
FAT4 | 65 | 15 | 40 | 47 | 1.00E-06 | |
FAT3 | 52 | 12 | 39 | 45 | 2.00E-08 | |
LRP1B | 56 | 13 | 33 | 38 | 1.00E-05 | |
HMCN1 | 56 | 13 | 32 | 37 | 3.00E-05 | |
RYR3 | 61 | 14 | 34 | 40 | 3.00E-05 | |
USH2A | 56 | 13 | 36 | 42 | 1.00E-06 | |
MUC5B | 69 | 16 | 43 | 50 | 4.00E-07 | |
ZFHX3 | 82 | 19 | 40 | 47 | 8.00E-05 | |
DNAH8 | 48 | 11 | 37 | 43 | 2.00E-08 | |
KMT2D | 95 | 22 | 43 | 50 | 2.00E-04 | |
ARID1A | 174 | 40 | 58 | 67 | 9.00E-03 | |
SACS | 43 | 10 | 33 | 38 | 1.00E-07 | |
FAT1 | 61 | 14 | 38 | 44 | 1.00E-06 | |
PIK3CA | 204 | 47 | 47 | 55 | 5.00E-01 | |
HERC2 | 56 | 13 | 30 | 35 | 1.00E-04 | |
SYNE2 | 52 | 12 | 33 | 38 | 3.00E-06 | |
LRP2 | 52 | 12 | 38 | 44 | 5.00E-08 |
Role of ICI scores in the independent validation cohort
Additionally, we sought to determine if the ICI score might be used to forecast immunotherapeutic efficacy in EC patients. In the IMvigor210 cohort, we chose patients who had undergone anti-PD-L1 immunotherapy for external validation of our findings. We discovered that the proportion of anti-PD-L1 therapy responders was increased in the low-ICI group as opposed to the high-ICI group (Wilcoxon test, P=3.318e-5, Figure 9A), and a high-ICI score was linked to disease progression when undergoing immunotherapy, whereas a low ICI score was linked to improved outcomes (log-rank test, P=0.033, Figure 9B,9C). We obtained similar results in the GSE78220 cohort, which received different immunotherapies (Wilcoxon test, P=9.219e-2; log-rank test, P=0.046, Figure 9D-9F). Accordingly, ICI ratings have possible clinical relevance in EC patients, because they can be used to categorize immunotherapy susceptibility.
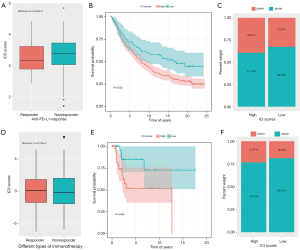
Discussion
The incidence of EC is comparable to that of breast cancer and cervical cancer among the gynecological malignant tumors, and its global morbidity and mortality are increasing annually (1,28). Its carcinogenesis and progression are driven by multiple mechanisms, many of which are closely related to the TME (29,30). TME was composed of tumour cells, stromal cells and immune cells including TAMs, NK cells, T regulatory cells, activated memory CD4 T cells, resting NK cells, CD8 T cells, etc. Thus, a new treatment strategy is required to restore host antitumor immunity for EC treatment. Regarding immunotherapy, only after confirming the functional mechanism and performance and rightly appraising the clinical effects and suitable recipients, can it be deemed a good practical solution. In this research, we examined the clinical characteristics and prognosis of EC patients and reinforced the evaluation model of the ICI scoring method by quantifying the comprehensive tumor immune milieu of EC. Through the ICI score, we can provide a personalized therapeutic schedule for patients with the “best fit” characteristics.
There are numerous ICIs in UCEC samples, which suggests that immune cells perform a function in the development and cell transformation of malignancy (3). Based on the TME-TILs score, we discovered that elevated immune score and stromal score were significantly linked to OS and showed that the immune scores of activated NK cells, T regulatory cells, activated memory CD4 T cells, resting NK cells, and CD8 T cells may be associated with improved prognosis, similar to previous studies demonstrating a link between ICI and survival time (31). EC was traditionally classified into two groups (type I and type II). However, with the development of high-throughput sequencing technology, molecular classification of EC based on genomic characterization provided possibility for individualized treatment (32-34). TCGA molecular classification identified EC into four classes: (I) ultramutated EC; (II) microsatellite instability-high (MSI-H) genotype (hypermutated); (III) copy number low tumors; (IV) copy number high tumors (35). Since the TCGA molecular classification was recommended by the National Comprehensive Cancer Network as a new clinical standard as of March 2020 and was recently incorporated into the ESGO recommendations (36), immunotherapy for different EC subtypes (especially POLE and MSI-H) has gradually attracted attention (37-39).
More and more evidence has shown that a large amount of immune cells and cytokines can be found in EC tissue, which can stimulate endogenous anti-tumor immune response (40). Compared with other gynecological malignancies, the expression level of PD-1 and PD-L1 in EC is the highest. In the TME of EC, tumor cells and infiltrating immune cells express immune checkpoints inhibitors such as CTLA4, PD-1 and PD-L1 and PD-L2 ligands, in order to inhibit the activation of T cells and to promote tumor immune escape (41). Immune checkpoint inhibitors, such as the PD-1 antibody pembrolizumab, can block the function of immune checkpoints and inhibit the immune escape of tumor cells, thereby mobilizing the autoimmune system to eliminate tumors. This study aimed to present an effective classification model based on ICI profiles and the patterns of immune-related gene expression that can achieve precision medicine for EC. Depending on the IPS_DEGs in the EC cohort, we were able to identify five distinct gene expression subtypes, of which the total immune profiles of GenC5 were significantly higher than those of the other clusters, and the percentages of CD8 T cells, plasma cells, and naive B cells were higher in GenC5 than in the other four clusters. These data suggested that the immunological milieu of GenC5 has been bolstered in some way. Notably, GeneC3 had the second-highest immune scores. When comparing OS, GeneC3 exhibited the poorest prognosis, whereas GenC5 exhibited a considerably improved prognosis in contrast to the other clusters. TAM infiltration into GenC3 correlated with a higher stromal score. Considerable infiltration of CD45RO_T cells and CD8 T cells in tumors is associated with a favorable prognosis, but UCEC patients with a high degree of TAM infiltration have a poor prognosis (42). Macrophages are one of the key regulators in the TME and perform an integral function in the process of tumor angiogenesis, progression, and metastasis (43,44). We concluded that the distribution of TILs in EC directly reflects the immune response character and functional condition of EC. In this study, we depicted the ICI landscape of EC using a TCGA-UCEC cohort. Collectively, our findings corroborated those from prior studies, demonstrating that gene clusters might soon become a useful prognostic tool for EC.
Predicated on the ICI landscape, we constructed a score that quantified the ICI pattern per patient. Anomalous expression of molecules on the immune checkpoint is among the most significant mechanisms of immune evasion by tumors (45,46). Study also suggests that infiltration of stromal cells may deplete immune cells and effectively counteract the immune infiltration survival benefit by up-modulating immune checkpoints (47).
During the evolution of EC, studies have found that gene mutations also affect TME, for example, MUC16 mutations could enhance the infiltration and antitumor immunity of cytotoxic T lymphocytes in the TME of EC patients (48-50).
TMB, as a biomarker of responsiveness to immune checkpoint inhibitors, is intimately linked to the number of gene mutations and neo-antigens, which helps the immune system to identify a tumor and promotes the activation of the antitumor immune response (26,27). The TMB of POLE and MSI-H is higher, which indicated that EC patients with POLE and MSI-H are most likely to benefit from PD-1/PD-L1 blockade therapy. In UCEC, high TMB is associated with longer OS (51), but higher TMB plays different roles in other cancers, which may be attributed to different driver mutations or other factors. With regard to the potential prognostic value of the mutated genes in EC, many studies have found that patients with mutations in PTEN, KRAS, TNN, CTNNB1, and MUC16 had a more favorable prognosis than patients with the wild-type genes (52-54). In our study, a favorable prognosis was seen in the EC patients in the low-ICI score group, as opposed to those who had a higher ICI score. TMB, MUC16, and PTEN are associated with a low-ICI score, and mutations in the low-ICI score group may affect immune infiltration, which may explain why the group with a low-ICI score and the higher TMB mutation rate exhibited a favorable survival. Furthermore, the ICI score continued to independently stand as a prognostic predictor even after stratification.
Considering its clinical application, the prognostic significance for immunotherapy efficacy of the ICI score on its own or when combined with PD-1/PD-L1 inhibition therapy was evaluated in IMvigor210 and GSE78220 cohorts. These results were additionally corroborated by cohorts of patients undergoing anti-PD-1 immunotherapy and other immunological treatments. Although the degree of improvement in both groups was not found to vary significantly, and higher immunotherapeutic responsiveness was observed in the low-ICI groups as opposed to the high-ICI groups, these data support the potential utility of the ICI score in clinical practice as a biomarker of the effectiveness of the immunotherapy response. According to our results, the clinician can perform ICI- related genes sequencing on EC patients in clinical practice, and classify patients into low/high-ICI score subgroups based on gene expression profiles. The low-ICI score patients can be recommended for immunotherapy and may have a better prognosis.
Based on this discussion, it can be seen that we identified important immune-related genes in EC and developed a prognostic ICI model. We investigated the possible involvement of TME molecules in the EC using a model that was founded on immune-related genes. These findings, coupled with future clinical trials, may result in a new categorization for targeted therapy in EC, which might lead to improved patient survival and longer lives. The discovery of ICI patterns may pave the way for new clinical antitumor exploration and medication development that harnesses the body’s immune system to fight cancer.
Acknowledgments
Funding: This research was funded by “Construction of a breast cancer immunogenicity identification model based on artificial intelligence imaging and genomics and its clinical application (No. J202106)”.
Footnote
Reporting Checklist: The authors have completed the STREGA reporting checklist. Available at https://atm.amegroups.com/article/view/10.21037/atm-22-301/rc
Conflicts of Interest: All authors have completed the ICMJE uniform disclosure form (available at https://atm.amegroups.com/article/view/10.21037/atm-22-301/coif). The authors have no conflicts of interest to declare.
Ethical Statement: The authors are accountable for all aspects of the work in ensuring that questions related to the accuracy or integrity of any part of the work are appropriately investigated and resolved. The study was conducted in accordance with the Declaration of Helsinki (as revised in 2013).
Open Access Statement: This is an Open Access article distributed in accordance with the Creative Commons Attribution-NonCommercial-NoDerivs 4.0 International License (CC BY-NC-ND 4.0), which permits the non-commercial replication and distribution of the article with the strict proviso that no changes or edits are made and the original work is properly cited (including links to both the formal publication through the relevant DOI and the license). See: https://creativecommons.org/licenses/by-nc-nd/4.0/.
References
- Sung H, Ferlay J, Siegel RL, et al. Global Cancer Statistics 2020: GLOBOCAN Estimates of Incidence and Mortality Worldwide for 36 Cancers in 185 Countries. CA Cancer J Clin 2021;71:209-49. [Crossref] [PubMed]
- Morice P, Leary A, Creutzberg C, et al. Endometrial cancer. Lancet 2016;387:1094-108. [Crossref] [PubMed]
- Vanderstraeten A, Tuyaerts S, Amant F. The immune system in the normal endometrium and implications for endometrial cancer development. J Reprod Immunol 2015;109:7-16. [Crossref] [PubMed]
- Colombo N, Preti E, Landoni F, et al. Endometrial cancer: ESMO Clinical Practice Guidelines for diagnosis, treatment and follow-up. Ann Oncol 2013;24:vi33-8. [Crossref] [PubMed]
- Njoku K, Chiasserini D, Whetton AD, et al. Proteomic Biomarkers for the Detection of Endometrial Cancer. Cancers (Basel) 2019;11:1572. [Crossref] [PubMed]
- O'Hara MH, O'Reilly EM, Varadhachary G, et al. CD40 agonistic monoclonal antibody APX005M (sotigalimab) and chemotherapy, with or without nivolumab, for the treatment of metastatic pancreatic adenocarcinoma: an open-label, multicentre, phase 1b study. Lancet Oncol 2021;22:118-31. [Crossref] [PubMed]
- Demetri GD, Luke JJ, Hollebecque A, et al. First-in-Human Phase I Study of ABBV-085, an Antibody-Drug Conjugate Targeting LRRC15, in Sarcomas and Other Advanced Solid Tumors. Clin Cancer Res 2021;27:3556-66. [Crossref] [PubMed]
- Guida A, Sabbatini R, Gibellini L, et al. Finding predictive factors for immunotherapy in metastatic renal-cell carcinoma: What are we looking for? Cancer Treat Rev 2021;94:102157. [Crossref] [PubMed]
- Hanahan D, Coussens LM. Accessories to the crime: functions of cells recruited to the tumor microenvironment. Cancer Cell 2012;21:309-22. [Crossref] [PubMed]
- Jing X, Peng J, Dou Y, et al. Macrophage ERα promoted invasion of endometrial cancer cell by mTOR/KIF5B-mediated epithelial to mesenchymal transition. Immunol Cell Biol 2019;97:563-76. [Crossref] [PubMed]
- Gupta V, Yull F, Khabele D. Bipolar Tumor-Associated Macrophages in Ovarian Cancer as Targets for Therapy. Cancers (Basel) 2018;10:366. [Crossref] [PubMed]
- De Felice F, Marchetti C, Tombolini V, et al. Immune check-point in endometrial cancer. Int J Clin Oncol 2019;24:910-6. [Crossref] [PubMed]
- Gatalica Z, Snyder C, Maney T, et al. Programmed cell death 1 (PD-1) and its ligand (PD-L1) in common cancers and their correlation with molecular cancer type. Cancer Epidemiol Biomarkers Prev 2014;23:2965-70. [Crossref] [PubMed]
- Liu J, Nie S, Wu Z, et al. Exploration of a novel prognostic risk signatures and immune checkpoint molecules in endometrial carcinoma microenvironment. Genomics 2020;112:3117-34. [Crossref] [PubMed]
- Wang G, Wang D, Sun M, et al. Identification of prognostic and immune-related gene signatures in the tumor microenvironment of endometrial cancer. Int Immunopharmacol 2020;88:106931. [Crossref] [PubMed]
- Steidl C, Lee T, Shah SP, et al. Tumor-associated macrophages and survival in classic Hodgkin's lymphoma. N Engl J Med 2010;362:875-85. [Crossref] [PubMed]
- Li YW, Qiu SJ, Fan J, et al. Intratumoral neutrophils: a poor prognostic factor for hepatocellular carcinoma following resection. J Hepatol 2011;54:497-505. [Crossref] [PubMed]
- Nishikawa H, Sakaguchi S. Regulatory T cells in cancer immunotherapy. Curr Opin Immunol 2014;27:1-7. [Crossref] [PubMed]
- Newman AM, Liu CL, Green MR, et al. Robust enumeration of cell subsets from tissue expression profiles. Nat Methods 2015;12:453-7. [Crossref] [PubMed]
- Sørlie T, Perou CM, Tibshirani R, et al. Gene expression patterns of breast carcinomas distinguish tumor subclasses with clinical implications. Proc Natl Acad Sci U S A 2001;98:10869-74. [Crossref] [PubMed]
- Charoentong P, Finotello F, Angelova M, et al. Pan-cancer Immunogenomic Analyses Reveal Genotype-Immunophenotype Relationships and Predictors of Response to Checkpoint Blockade. Cell Rep 2017;18:248-62. [Crossref] [PubMed]
- Kursa MB. Rudnicki WRJJoSS. Feature Selection with Boruta Package. J Stat Softw 2010;36:1-13. [Crossref]
- Mayakonda A, Lin DC, Assenov Y, et al. Maftools: efficient and comprehensive analysis of somatic variants in cancer. Genome Res 2018;28:1747-56. [Crossref] [PubMed]
- Mariathasan S, Turley SJ, Nickles D, et al. TGFβ attenuates tumour response to PD-L1 blockade by contributing to exclusion of T cells. Nature 2018;554:544-8. [Crossref] [PubMed]
- Yu G, Wang LG, Han Y, et al. clusterProfiler: an R package for comparing biological themes among gene clusters. OMICS 2012;16:284-7. [Crossref] [PubMed]
- Gibney GT, Weiner LM, Atkins MB. Predictive biomarkers for checkpoint inhibitor-based immunotherapy. Lancet Oncol 2016;17:e542-51. [Crossref] [PubMed]
- Rizvi NA, Hellmann MD, Snyder A, et al. Cancer immunology. Mutational landscape determines sensitivity to PD-1 blockade in non-small cell lung cancer. Science 2015;348:124-8. [Crossref] [PubMed]
- Lortet-Tieulent J, Ferlay J, Bray F, et al. International patterns and trends in endometrial cancer incidence, 1978-2013. J Natl Cancer Inst 2018;110:354-61. [Crossref] [PubMed]
- Pasanen A, Ahvenainen T, Pellinen T, et al. PD-L1 Expression in Endometrial Carcinoma Cells and Intratumoral Immune Cells: Differences Across Histologic and TCGA-based Molecular Subgroups. Am J Surg Pathol 2020;44:174-81. [Crossref] [PubMed]
- Felix AS, Weissfeld J, Edwards R, et al. Future directions in the field of endometrial cancer research: the need to investigate the tumor microenvironment. Eur J Gynaecol Oncol 2010;31:139-44. [PubMed]
- de Jong RA, Leffers N, Boezen HM, et al. Presence of tumor-infiltrating lymphocytes is an independent prognostic factor in type I and II endometrial cancer. Gynecol Oncol 2009;114:105-10. [Crossref] [PubMed]
- Rubio-Pérez J, Hernández R, Hernández T, et al. Dostarlimab for the treatment of endometrium cancer and other solid tumors. Drugs Today (Barc) 2021;57:187-97. [Crossref] [PubMed]
- Gómez-Raposo C, Merino Salvador M, Aguayo Zamora C, et al. Immune checkpoint inhibitors in endometrial cancer. Crit Rev Oncol Hematol 2021;161:103306. [Crossref] [PubMed]
- Dong D, Lei H, Liu D, et al. POLE and mismatch repair status, checkpoint proteins and tumor-infiltrating lymphocytes in combination, and tumor differentiation: identify endometrial cancers for immunotherapy. Front Oncol 2021;11:640018. [Crossref] [PubMed]
- Cancer Genome Atlas Research Network. Integrated genomic characterization of endometrial carcinoma. Nature 2013;497:67-73. [Crossref] [PubMed]
- Concin N, Matias-Guiu X, Vergote I, et al. ESGO/ESTRO/ESP guidelines for the management of patients with endometrial carcinoma. Radiother Oncol 2021;154:327-53. [Crossref] [PubMed]
- Brahmer JR, Tykodi SS, Chow LQ, et al. Safety and activity of anti-PD-L1 antibody in patients with advanced cancer. N Engl J Med 2012;366:2455-65. [Crossref] [PubMed]
- Makker V, Taylor MH, Aghajanian C, et al. Lenvatinib plus pembrolizumab in patients with advanced endometrial cancer. J Clin Oncol 2020;38:2981-92. [Crossref] [PubMed]
- Mitchell TC, Hamid O, Smith DC, et al. Epacadostat Plus Pembrolizumab in Patients With Advanced Solid Tumors: Phase I Results From a Multicenter, Open-Label Phase I/II Trial (ECHO-202/KEYNOTE-037). J Clin Oncol 2018;36:3223-30. [Crossref] [PubMed]
- Cao W, Ma X, Fischer JV, et al. Immunotherapy in endometrial cancer: rationale, practice and perspectives. Biomark Res 2021;9:49. [Crossref] [PubMed]
- Wei SC, Duffy CR, Allison JP. Fundamental mechanisms of immune checkpoint blockade therapy. Cancer Discov 2018;8:1069-86. [Crossref] [PubMed]
- Guo F, Dong Y, Tan Q, et al. Tissue Infiltrating Immune Cells as Prognostic Biomarkers in Endometrial Cancer: A Meta-Analysis. Dis Markers 2020;2020:1805764. [Crossref] [PubMed]
- Kitamura T, Qian BZ, Pollard JW. Immune cell promotion of metastasis. Nat Rev Immunol 2015;15:73-86. [Crossref] [PubMed]
- Qian BZ, Pollard JW. Macrophage diversity enhances tumor progression and metastasis. Cell 2010;141:39-51. [Crossref] [PubMed]
- Spranger S, Spaapen RM, Zha Y, et al. Up-regulation of PD-L1, IDO, and T(regs) in the melanoma tumor microenvironment is driven by CD8(+) T cells. Sci Transl Med 2013;5:200ra116. [Crossref] [PubMed]
- Taube JM, Anders RA, Young GD, et al. Colocalization of inflammatory response with B7-h1 expression in human melanocytic lesions supports an adaptive resistance mechanism of immune escape. Sci Transl Med 2012;4:127ra37. [Crossref] [PubMed]
- Thommen DS, Schumacher TN. T Cell Dysfunction in Cancer. Cancer Cell 2018;33:547-62. [Crossref] [PubMed]
- Hu J, Sun J. MUC16 mutations improve patients' prognosis by enhancing the infiltration and antitumor immunity of cytotoxic T lymphocytes in the endometrial cancer microenvironment. Oncoimmunology 2018;7:e1487914. [Crossref] [PubMed]
- Zhou H, Chen L, Lei Y, et al. Integrated analysis of tumor mutation burden and immune infiltrates in endometrial cancer. Curr Probl Cancer 2021;45:100660. [Crossref] [PubMed]
- van Gool IC, Eggink FA, Freeman-Mills L, et al. POLE Proofreading Mutations Elicit an Antitumor Immune Response in Endometrial Cancer. Clin Cancer Res 2015;21:3347-55. [Crossref] [PubMed]
- Ding H, Zhao J, Zhang Y, et al. Tumor mutational burden and prognosis across pan-cancers. Ann Oncol 2018. Available online: https://www.annalsofoncology.org/article/S0923-7534(19)48483-4/pdf
- Althubiti MA. Mutation Frequencies in Endometrial Cancer Patients of Different Ethnicities and Tumor Grades: An Analytical Study. Saudi J Med Med Sci 2019;7:16-21. [Crossref] [PubMed]
- Westin SN, Ju Z, Broaddus RR, et al. PTEN loss is a context-dependent outcome determinant in obese and non-obese endometrioid endometrial cancer patients. Mol Oncol 2015;9:1694-703. [Crossref] [PubMed]
- Sideris M, Emin EI, Abdullah Z, et al. The role of KRAS in endometrial cancer: a mini-review. Anticancer Res 2019;39:533-9. [Crossref] [PubMed]
(English Language Editor: K. Brown)