A new pyroptosis model can predict the immunotherapy response and immune microenvironment characteristics and prognosis of patients with cutaneous melanoma based on TCGA and GEO databases
Introduction
Pyroptosis is believed to be a form of caspase-mediated programmed cell death, which activates inflammatory caspase and eventually leads to the lysis of gasdermins (GSDMs) when the body is invaded by pathogens. N-terminally bound GSDMs (GSDMs-NT) perforate the cell membrane surface, thereby inducing lytic death of the pathogen and the infected host cell (1-3). Pyroptosis is not limited to infectious diseases, and new research suggests that it is also associated with cancer (4). In humans, GSDMs consist of GSDMA, GSDMB, GSDMC, GSDMD, GSDME (DFNA5), and DFNB59 (PJVK). Gasdermin D (GSDMD) was first discovered in 2015 and was identified as the key executive protein of downstream molecular signals after the activation of caspase (4,5). The classical pyroptosis pathway is mediated by the assembly of inflammasomes, including NLRC4, NLRP1, NLRP2, NLRP3, NLRP6, NLRP7, and AIM2 (6,7). Inflammasomes and their related cytokines are also associated with the tumor immune microenvironment and prognosis (8,9).
Early-stage cutaneous melanoma has a good prognosis, but advanced melanoma has a poor prognosis (10). Immunotherapy has achieved good results in the treatment of cutaneous melanoma and has been used for first-line treatment (11). Meanwhile, our previous study found that GSDMs were associated with the immune microenvironment (12). Therefore, we wanted to observe the relationship between the pyroptosis and the immune microenvironment of melanoma, as well as the relationship with the prognosis of melanoma.
Therefore, we performed a pan-cancer analysis of 13 genes associated with GSDMs and inflammasomes and found that they were closely related to the overall survival (OS) of skin cutaneous melanoma (SKCM). Then, we clustered the prognostic genes among all 33 pyroptosis-related genes and constructed a risk model, which could also divide SKCM into 2 clusters with significant differences in prognosis. The established model could be well verified by the data in the Gene Expression Omnibus (GEO) database. Previous studies in gastric (13) and colon (14) cancer have also modeled the risk associated with prognosis.
Further analysis also showed that the C2 cluster with better prognosis was predominantly the low-risk group, and compared with the C1 cluster or high-risk group, the C2 cluster or low-risk group tended to have high expression of pyroptosis-related genes. This group also had a lower exclusion score, suggesting that it had a more favorable microenvironment for T cell infiltration (15), along with higher immune scores, more infiltration of immune cells, especially T cells, and greater gene expression of chemokines and their receptors. However, we also found that the C2 cluster or low-risk group had a higher dysfunction score, indicating a stronger microenvironment that suppresses T cell immune function (15). Furthermore, our study showed that the C2 cluster or low-risk group was more sensitive to anti-PD1 and CTLA4 therapy.
Therefore, the pyroptosis status in SKCM is closely related to the prognosis of patients and the immune microenvironment. There is more immune cell infiltration and more immune escape factors in tumor tissue with high expression of pyroptosis-related factors, making these tumors more suitable for immunotherapy. The pyroptosis prognostic risk score can effectively predict the prognosis of patients with melanoma and predict the characteristics of the immune microenvironment and the sensitivity to immunotherapy. At the same time, we believe that in the future, changing the state of pyroptosis may turn cold tumors that are not suitable for immunotherapy into hot tumors that are suitable for immunotherapy. We present the following article in accordance with the TRIPOD reporting checklist (available at https://atm.amegroups.com/article/view/10.21037/atm-22-1095/rc).
Methods
Data sources
The gene expression data as well as mutation and survival data of SKCM was obtained from The Cancer Genome Atlas (TCGA) website (https://portal.gdc.cancer.gov/repository). The ESTIMATE (16) and CIBERSORT algorithms (17) were used to calculate the immune and stromal scores and the immune cell infiltration content of each tumor. We also downloaded gene expression data and survival data of cutaneous melanoma from the GEO database (https://www.ncbi.nlm.nih.gov/geo/) (GEO accession number: GSE19234). Microsatellite instability (MSI), exclusion, and dysfunction scores were downloaded from the Tumor Immune Dysfunction and Exclusion (TIDE) website (http://tide.dfci.harvard.edu). Immunophenoscores (IPS) were downloaded from The Cancer Immunome Database (TCIA) website (https://tcia.at/).
The study was conducted in accordance with the Declaration of Helsinki (as revised in 2013).
Gene Expression Profiling Interactive Analysis (GEPIA)
Using the GEPIA2 (18) (http://gepia.cancer-pku.cn/) online analysis website, “Survival Map” was selected in the search analysis toolbar of GEPIA2. All gene names were input, “Overall Survival” was selected in methods, the P value was set to 0.05, and median for group cutoff was selected. Multiple genes were analyzed in relation to OS in all tumors. “Dimensionality Reduction” was selected in the analysis toolbar, and the tumor type was “Skin Cutaneous Melanoma, SKCM”. Genotype-tissue expression (GTEx) selected skin-not sun exposed (suprapubic) and skin-sun exposed (lower leg), and 13 pyroptosis-related genes were input for principal component analysis (PCA). “Survival Plots” was selected in the analysis toolbar, and the tumor type was “Skin Cutaneous Melanoma, SKCM”. Overall survival was selected in methods and median was selected for group cutoff to analyze the relationship between each gene and OS in SKCM patients.
R software analysis and plotting
Kaplan-Meier survival curves were drawn by R software with the survival and survminer packages. Cluster analysis was performed by the ConsensusClusterPlus package, and heat maps were drawn by the Pheatmap package. The GSEABase and GSVA packages were used to score ssGSEA. Prognosis-related genes were used to build a prognostic model, SKCM data in the TCGA database was used as a training set, and the melanoma data in the GEO database was used for validation, and the timeROC package was used to plot the receiver operating characteristic (ROC) curve of the survival model. The Ggalluvial package was used to plot the Sankey diagram. The Rtsne package was used to reduce the dimensionality, and then PCA was performed.
Gene Ontology (GO) and Kyoto Encyclopedia of Genes and Genomes (KEGG) analysis
The clusterProfiler, org.Hs.eg.db, enrichplot, and ggplot2 packages were applied for the GO and KEGG enrichment of differential genes with a P value of less than 0.001 between the C1 and C2 clusters or between the high- and low-risk groups. Output enrichment results with pvalueFilter <0.05 and qvalueFilter <0.05, Then the top 10 most statistically significant categories in biological process (BP), cellular component (CC) and molecular function (MF) in GO enrichment were plotted. The top 30 categories with the greatest statistical significance in the KEGG enrichment were mapped.
Statistical analysis
On the GEPIA2 online analysis website, P<0.05 was selected, which was considered as statistically significant. Pearson’s correlation coefficient was used for correlation analysis, and P<0.05 was statistically significant. Assess the predictive power of a model by comparing AUC. For difference analysis, GO, KEGG enrichment, univariate and multivariate Cox analysis, and prognostic analysis, P<0.05 was considered as statistically significant.
Results
Pan-cancer analysis of the relationship between the gene expression of GSDMs and inflammasomes and OS
Using the GEPIA2 online analysis tool, we performed a pan-cancer analysis of the relationship between GSDMs, inflammasome-related genes, and OS. It was found that GSDMB, GSDMD, NLRC4, NLRP1, NLRP3, NLRP6, NLRP7, and AIM2 were negatively correlated with the OS of SKCM (Figure 1A). It was also found that the expression of GSDMs and inflammasome-related genes was significantly different in cutaneous melanoma and skin tissue (Figure 1B). In addition, Kaplan-Meier survival curves of SKCM were plotted separately for each prognostic gene (Figure 1C-1J).
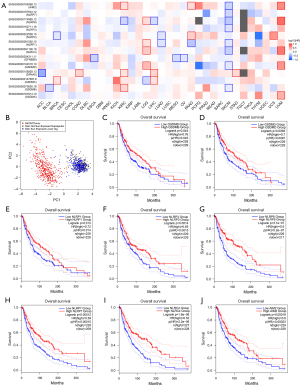
Two clusters of SKCM with significant differences in prognosis based on clustering of prognostic-related GSDMs and inflammasome genes
We used the prognostic-related GSDMs and inflammasome genes to cluster the skin melanoma samples. We found these genes could effectively divide SKCM into 2 clusters for melanoma data from TCGA database (Figure 2A-2C), and the 2 clusters had significant differences in prognosis (P<0.05; Figure 2D). Based on melanoma data from GEO database, it was also found that these genes could effectively divide melanoma data into 2 clusters (Figure 2E-2G), and there was a significant difference in prognosis between the 2 clusters (P<0.05; Figure 2H).
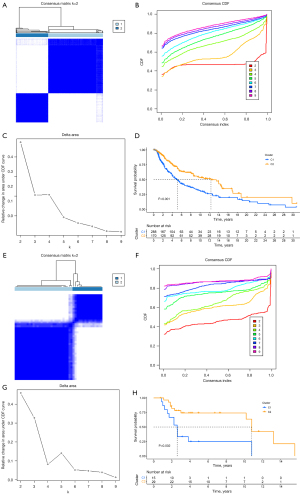
Two clusters of SKCM with significant differences in prognosis based on clustering of prognostic-related genes 33 pyroptosis-related genes via data from the TCGA database
Using the SKCM data in TCGA database, we further clustered the genes related to the prognosis of melanoma among 33 pyroptosis-related genes and found that these genes could also divide SKCM into 2 clusters (Figure 3A-3D), and the prognosis of the 2 clusters was significantly different (Figure 3E). Moreover, in the C2 cluster with a better prognosis, the expression of pyroptosis-related genes was higher than that in the C1 cluster (Figure 3F,3G).
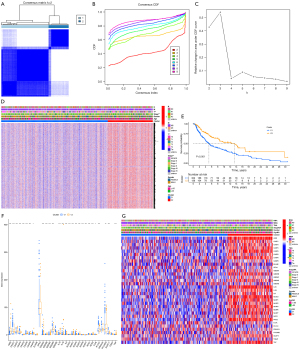
Two clusters of SKCM with significant differences in prognosis based on clustering of prognostic-related genes 33 pyroptosis-related genes via data from the GEO database
By analyzing the data of skin melanoma from the GSE19234 data set downloaded from the GEO database, we also found that the pyroptosis prognostic genes could divide melanoma into 2 clusters (Figure 4A-4C), and the prognosis of the 2 clusters was significantly different (Figure 4D). Moreover, in the C2 cluster with a better prognosis, the expression of pyroptosis-related genes was higher than that in the C1 cluster (Figure 4E).
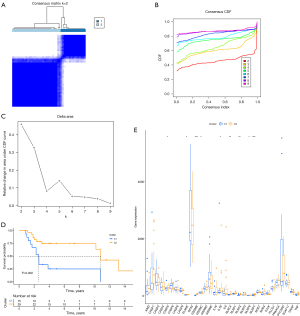
The C1 and C2 clusters had different immune cell infiltration profiles and different immunoinhibitor, MHC molecule, immunostimulator, chemokine, and chemokine receptor gene expression
Using the CIBERSORT algorithm to calculate the content of immune cells, it was found that C2 cluster had a higher proportion of infiltrating memory B cells, CD8+ T cells, activated memory CD4+ T cells, Tregs, and M1 macrophages, and a lower proportion of M2 macrophages, M0 macrophages, and resting mast cells compared with the C1 cluster. The proportions of most other cells in the C2 cluster were also higher than those in the C1 cluster, but this was not statistically significant (Figure 5A). Using the ssGSEA method to calculate immune cell scores, it was found that all immune cell scores in the C2 cluster were higher than those in the C1 cluster (Figure 5B). In addition, the C2 cluster had high gene expression of most immunoinhibitors (Figure 5C), MHC molecules (Figure 5D), immunostimulators (Figure 5E), and chemokines and their receptors (Figure 5F).
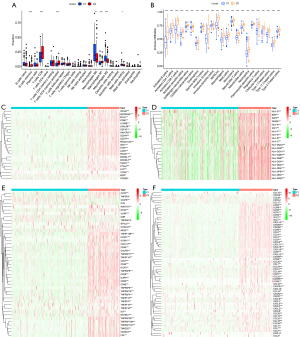
The risk model constructed by the genes related to the prognosis of pyroptosis could effectively predict the prognosis of patients
Based on SKCM data from TCGA database, prognostic risk models were constructed for SKCM using prognosis-related genes among 13 major pyroptosis genes or 33 pyroptosis genes. The prognostic risk models constructed using the 13 major pyroptosis genes could not be validated in melanoma data in the GEO database (Figure 6A). Finally, a prognostic risk model including AIM2, GSDMC, GSDMD, IL18, NLRP6, and PRKACA was constructed by using the prognosis-related genes among the 33 pyroptosis-related genes (Figure 6B), and it was found that the constructed prognostic risk model could be effectively verified by the melanoma data in the GEO database (Figure 6C). By analyzing the ROC curve, it was found that the prognostic risk model constructed by the prognosis-related genes among the 33 pyroptosis genes was better than that constructed by the prognosis-related genes among the 13 pyroptosis genes. Because the area under the curve (AUC) value of 1 year, 2 years and 3 years is higher than that of the latter model (Figure 6D,6E). The application of this model in the GEO database also had a higher AUC value (Figure 6F). The risk plot also indicated that the risk score was related to the prognosis of patients (Figure 6G-6I). Through PCA (Figure 6J-6L) and t-SNE (Figure 6M-6O) analysis, it was found that the prognostic genes involved in the construction of the model could divide the samples into 2 different groups.
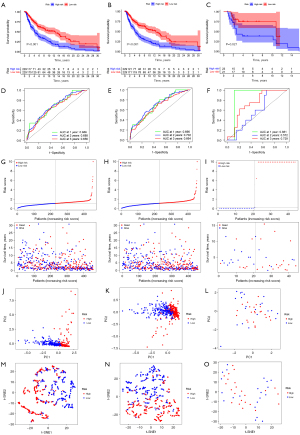
Risk score was an independent prognostic factor for SKCM
The risk score was significantly associated with prognosis in both univariate (Figure 7A) and multivariate (Figure 7B) Cox analyses. This suggests that the risk score can be used as a prognostic indicator of SKCM and is an independent prognostic factor.
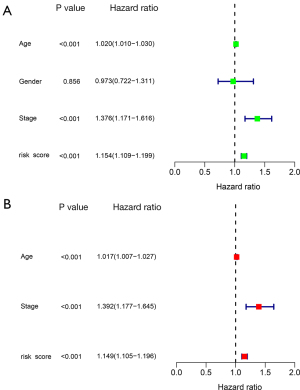
High- and low-risk groups had different immune cell infiltration and immune scores
Through the Sankey diagram (Figure 8A), we found that the C2 cluster was predominantly the low-risk group, and the C1 cluster was predominantly the high-risk group. Our analysis also showed that the C2 cluster had a better prognosis than the C1 cluster. In the low-risk group, pyroptosis-related genes were also highly expressed (Figure S1A). The CIBERSORT method was used to calculate the infiltration content of immune cells in the tumor microenvironment (Figure 8B). It was found that the low-risk group had a higher proportion of memory B cells, plasma cells, CD8+ T cells, activated memory CD4+ T cells, Tregs, and M1 macrophages than the high-risk group, along with a lower proportion of resting gamma delta natural killer (NK) cells, M2 macrophages, M0 macrophages, and resting mast cells. The proportions of most other cells in the low-risk group were also higher than those in the high-risk group, but there was no statistical significance. Using ssGSEA to score immune cells (Figure S1B), it was also found that almost all immune cells in the low-risk group scored higher than those in the high-risk group. Immune function analysis showed that except for mast cells, which were lower in the low-risk group, other immune functions were higher in the low-risk group (Figure 8C), which was consistent with the low proportion of resting mast cells in the low-risk group. The immune score is often correlated with the content of immune cells, and it was found that the risk score and immune score were highly correlated and statistically significant (Figure 8D). In addition, the risk score was not significantly associated with TMB (Figure 8E) and DNA methylation based Stemness Scores (DNAss) (Figure 8F), although it was significantly associated with RNA based Stemness Scores (RNAss) (Figure 8G) and stromal score (Figure 8H), but the correlation coefficient was too low.
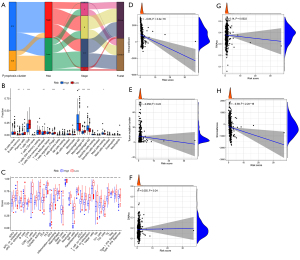
High- and low-risk groups had different gene expression profiles of immunoinhibitors, MHC molecules, immunostimulators, and chemokines and their receptors
Our analysis showed that genes such as immunoinhibitors (Figure 9A), MHC molecules (Figure 9B), immunostimulators (Figure 9C), and chemokines and their receptors (Figure 9D) were highly expressed in the low-risk group.
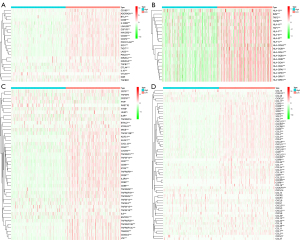
The correlation between risk score and the expression of genes such as immunoinhibitors, MHC molecules, immunostimulators, and chemokines and their receptors
It was found that the risk score was negatively correlated with the gene expression of most of the immunoinhibitors (Figure 10A), MHC molecules (Figure 10B), immunostimulators (Figure 10C), and chemokines and their receptors (Figure 10D). Analysis of common immune checkpoint-related genes found that the correlation coefficients with PD1, PDL1, PDL2, TIGIT, CTLA-4, LAG3, LGALS9, HAVCR2, and BTLA were greater than 0.5, and the P values were less than 0.001 (Figure 10E-10M).
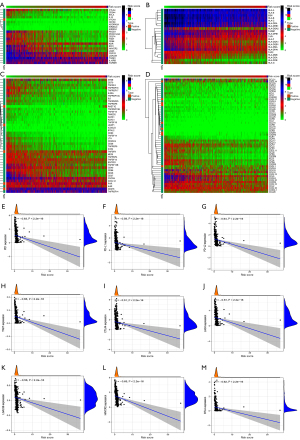
Exclusion, dysfunction, and IPS differences between the C1 and C2 clusters or the high- and low-risk groups
Through the Sankey diagram (Figure 11A) or difference analysis, it was found that the low-risk group or C2 cluster tended to have more MSI patients, lower exclusion scores, but higher dysfunction scores (Figure 11B-11G). At the same time, the low-risk group or C2 cluster had higher IPS, including ips_ctla4_neg_pd1_pos, ips_ctla4_pos_pd1_neg, and ips_ctla4_pos_pd1_pos. These findings suggest that the low-risk group or C2 cluster is more suitable for anti-PD1 or CTLA4 immunotherapy (Figure 11H-11O).
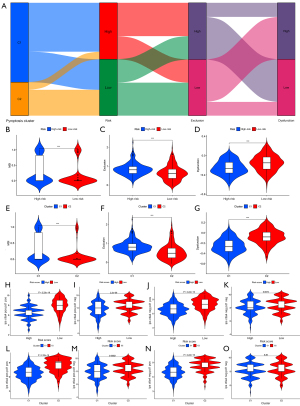
GO and KEGG enrichment
GO and KEGG enrichment analysis was performed on the differential genes between the C1 and C2 clusters (Figure 12A,12B) or between the high- and low-risk groups (Figure 12C,12D). It was found that GO enrichment was mainly involved in immune-related functions. KEGG enrichment was also mainly involved in immune cell-related pathways.
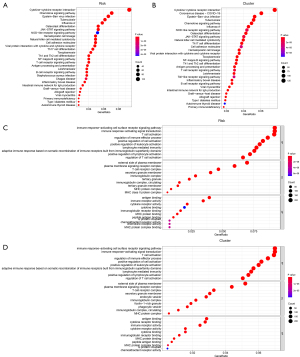
Discussion
The classical pyroptosis pathway is mediated by inflammasomes and caspase-1. When the body is invaded by pathogens, inflammatory caspase is activated, which eventually leads to the lysis of GSDMs. GSDM-NTs perforate the cell membrane surface, thereby inducing lytic death of pathogens and infected host cells (1,2,6,7). In addition to PJVK, almost all N-terminal domains of GSDMs can exert pore-forming activity in the plasma membrane (4,19). It has now been found that they can also be cleaved by other proteases, such as caspase-4/5/11, caspase-3, caspase-8, and granzymes (20).
Using GEPIA2 online analysis tools, “Median” was selected for group cutoff to analyze the prognosis of GSDM family genes and inflammasome-related genes. We found that most of the GSDMs and inflammasome genes were negatively correlated with the prognosis of SKCM. We used prognosis-related genes to cluster SKCM data downloaded from TCGA database and found that SKCM could be effectively divided into 2 clusters, and the prognosis of the C1 and C2 clusters was significantly different. Melanoma data downloaded from the GEO database also found the same phenomenon. However, the prognostic risk model constructed by these genes could not be validated in the GEO database.
In order to better cluster the tumors and construct a good prognostic risk model, we clustered the prognostic-related genes among 33 pyroptosis genes. Both SKCM data downloaded from TCGA database and melanoma data downloaded from the GEO database could effectively divide melanoma into 2 clusters, and the prognosis of the 2 clusters was statistically different. The results were similar to the clustering of prognosis-related genes in GSDMs and inflammasomes. Furthermore, we constructed a prognostic risk model by using prognosis-related genes, and finally constructed a prognostic risk model including AIM2, GSDMC, GSDMD, IL18, NLRP6, and PRKACA. We found that the constructed prognostic risk model could be effectively validated by the melanoma data in the GEO database. At the same time, the prognostic risk model constructed by prognostic-related genes among 33 pyroptosis genes was better than that constructed by prognostic-related genes among 13 pyroptosis genes. Additionally, the risk score was an independent prognostic factor of SKCM.
Previous studies have also found that clustering genes related to pyroptosis can classify ovarian cancer, gastric cancer, and colon cancer into 2 clusters with different prognosis, and the risk scores constructed for gastric cancer, lung cancer, and colon cancer can effectively predict patient prognosis (13,14,21,22). But except in lung cancer, the establishment of risk model directly applies pyroptosis-related genes (22), Models in stomach cancer (13) and colon cancer (14) is mainly based on DEGs identified based on the 2 pyroptosis subtypes. This time, we constructed a prognostic risk model directly using pyroptosis genes.
Because the C2 cluster had a better prognosis, further analysis also showed that the C2 cluster was predominantly the low-risk group and the C1 cluster was predominantly the high-risk group. Moreover, the C2 cluster or low-risk group was showed high expression of pyroptosis-related genes. Previous researchers have found that GSDMA is associated with the development of gastric cancer and esophageal cancer, and GSDMB and GSDMC are associated with the development of breast cancer and melanoma, respectively (23). Moreover, PD-L1 can induce pyroptosis by promoting the expression of GSDMC (24). In a study of GSDMD, it was also found that the pyroptosis of nasopharyngeal carcinoma cells induced by paclitaxel was mediated by GSDMD, and the pyroptosis induced by paclitaxel was inhibited after knocking out GSDMD (25). Low expression of GSDME may favor melanoma growth (26). Therefore, the good prognosis of the C2 cluster or low-risk group may be due to the high expression of pyroptosis genes, which increases the incidence of pyroptosis of tumor cells or increases the sensitivity of tumor cells to drug-induced pyroptosis.
High immune scores tend to indicate more immune cell infiltration in the tumor microenvironment (16). Our analysis found a negative correlation between the risk score and immune score. Subsequent analysis revealed that the C2 cluster or low-risk group tended to have more immune cell infiltration, especially effector T cell infiltration. This may be due to cell pyroptosis inducing the release of large amounts of inflammatory factors and cell contents. This results in the recruitment of immune cells, which further induces the inflammatory response and causes inflammatory death of the cell (27).
TIDE module can model 2 primary mechanisms of tumor immune evasion: the induction of T cell dysfunction in tumors with high infiltration of cytotoxic T lymphocytes (CTLs) and the prevention of T cell infiltration in tumors with low CTL levels, and the exclusion score and dysfunction score can represent the strength of the 2 tumor immune evasion mechanisms, respectively (15). Our analysis found that the C2 cluster or low-risk group had a lower exclusion score, indicating that the ability to prevent T cell infiltration in tumors was weak. However, the induction of T cell dysfunction in tumors was stronger because the C2 cluster or low-risk group had a higher dysfunction score. This suggests that the low-risk group has a more favorable microenvironment for T cell infiltration than the high-risk group, but has a stronger ability to induce T cell dysfunction. Our analysis also showed that the C2 cluster or low-risk group had more T cell infiltration and greater expression of chemokines and their receptors, which may favor immune cell infiltration. However, at the same time, this was accompanied by high expression of immunoinhibitors and MHC-related genes, as well as more immunosuppressive Tregs. These factors may induce T cell dysfunction. The IPS can predict response to immunotherapy with CTLA-4 and PD-1 blockers (28). Our analysis showed that patients in the C2 cluster or low-risk group had a higher IPS, indicating that they were more sensitive to treatment against PD1 or CTLA4. This may also be related to the fact that there is more infiltration of immune cells but greater expression of immunoinhibitors such as PD1 and CTLA4. When immunosuppression is relieved, there are more immune cells in tumors to play an anti-tumor role. Previous study has also shown that the immune response induced by pyroptosis activation is a double-edged sword that affects all stages of tumorigenesis (27). On the one hand, the activation of inflammasome-mediated pyroptosis and the release of pyroptosis-produced cytokines alter the immune microenvironment and promote the development of tumors by evading immune surveillance (27). On the other hand, pyroptosis-produced cytokines can also collect immune cells and induce the immune system to improve the efficiency of tumor immunotherapies (27). Therefore, the C2 cluster or low-risk group tended to have more immune cell infiltration, but also more immune escape factors, such as immunosuppressive cells and immunoinhibitors, along with greater MHC gene expression, leading to T cell dysfunction. This type of tumor may be a hot tumor that is more suitable for immunotherapy (29).
GO and KEGG enrichment analysis showed that the DEGs between the high- and low-risk groups or between the C1 and C2 clusters were mainly enriched in immune-related functions or immune-related signaling pathways. This is consistent with our analysis of the immune microenvironment.
In conclusion, pyroptosis is closely related to the prognosis of SKCM, the immune microenvironment, and the response to immunotherapy. The prognostic model or risk score constructed by prognostic-related genes can effectively predict the prognosis of SKCM At the same time, the C2 cluster or low-risk group had a more favorable microenvironment for immune cell infiltration, resulting in more immune cell infiltration, especially T cells. However, at the same time, this group had more immune escape factors, such as more immunoinhibitors, greater MHC gene expression, and more infiltration of immunosuppressive Tregs. These tumors are more suitable for immunotherapy. Therefore, the risk score of pyroptosis can distinguish different immune microenvironments and predict the sensitivity to immunotherapy. This model can be used to guide the prognosis of patients and the choice of immunotherapy. At the same time, we believe that further studies should try to change the pyroptosis status of SKCM, which may improve the prognosis of patients and change cold tumors without immune cell infiltration into hot tumors rich in immune cells (29), thereby making them suitable for immunotherapy. This may also be a treatment for SKCM.
Acknowledgments
Funding: None.
Footnote
Reporting Checklist: The authors have completed the TRIPOD reporting checklist. Available at https://atm.amegroups.com/article/view/10.21037/atm-22-1095/rc
Conflicts of Interest: All authors have completed the ICMJE uniform disclosure form (available at https://atm.amegroups.com/article/view/10.21037/atm-22-1095/coif). The authors have no conflicts of interest to declare.
Ethical Statement: The authors are accountable for all aspects of the work in ensuring that questions related to the accuracy or integrity of any part of the work are appropriately investigated and resolved. The study was conducted in accordance with the Declaration of Helsinki (as revised in 2013).
Open Access Statement: This is an Open Access article distributed in accordance with the Creative Commons Attribution-NonCommercial-NoDerivs 4.0 International License (CC BY-NC-ND 4.0), which permits the non-commercial replication and distribution of the article with the strict proviso that no changes or edits are made and the original work is properly cited (including links to both the formal publication through the relevant DOI and the license). See: https://creativecommons.org/licenses/by-nc-nd/4.0/.
References
- Yuan YY, Xie KX, Wang SL, et al. Inflammatory caspase-related pyroptosis: mechanism, regulation and therapeutic potential for inflammatory bowel disease. Gastroenterol Rep (Oxf) 2018;6:167-76. [Crossref] [PubMed]
- Gao YL, Zhai JH, Chai YF. Recent Advances in the Molecular Mechanisms Underlying Pyroptosis in Sepsis. Mediators Inflamm 2018;2018:5823823. [Crossref] [PubMed]
- Kuriakose T, Kanneganti TD. Pyroptosis in Antiviral Immunity. Curr Top Microbiol Immunol 2019; [Epub ahead of print]. [Crossref] [PubMed]
- Fang Y, Tian S, Pan Y, et al. Pyroptosis: A new frontier in cancer. Biomed Pharmacother 2020;121:109595. [Crossref] [PubMed]
- Shi J, Zhao Y, Wang K, et al. Cleavage of GSDMD by inflammatory caspases determines pyroptotic cell death. Nature 2015;526:660-5. [Crossref] [PubMed]
- Morimoto N, Kono T, Sakai M, et al. Inflammasomes in Teleosts: Structures and Mechanisms That Induce Pyroptosis during Bacterial Infection. Int J Mol Sci 2021;22:4389. [Crossref] [PubMed]
- Fann DY, Lee SY, Manzanero S, et al. Pathogenesis of acute stroke and the role of inflammasomes. Ageing Res Rev 2013;12:941-66. [Crossref] [PubMed]
- Segovia M, Russo S, Girotti MR, et al. Role of inflammasome activation in tumor immunity triggered by immune checkpoint blockers. Clin Exp Immunol 2020;200:155-62. [Crossref] [PubMed]
- Thi HTH, Hong S. Inflammasome as a Therapeutic Target for Cancer Prevention and Treatment. J Cancer Prev 2017;22:62-73. [Crossref] [PubMed]
- Gurzu S, Beleaua MA, Jung I. The role of tumor microenvironment in development and progression of malignant melanomas - a systematic review. Rom J Morphol Embryol 2018;59:23-28. [PubMed]
- Sullivan RJ, Flaherty KT. Immunotherapy: Anti-PD-1 therapies-a new first-line option in advanced melanoma. Nat Rev Clin Oncol 2015;12:625-6. [Crossref] [PubMed]
- Wang YY, Shi LY, Xu MH, et al. A pan-cancer analysis of the expression of gasdermin genes in tumors and their relationship with the immune microenvironment. Transl Cancer Res 2021;10:4125-47. [Crossref] [PubMed]
- Shao W, Yang Z, Fu Y, et al. The Pyroptosis-Related Signature Predicts Prognosis and Indicates Immune Microenvironment Infiltration in Gastric Cancer. Front Cell Dev Biol 2021;9:676485. [Crossref] [PubMed]
- Song W, Ren J, Xiang R, et al. Identification of pyroptosis-related subtypes, the development of a prognosis model, and characterization of tumor microenvironment infiltration in colorectal cancer. Oncoimmunology 2021;10:1987636. [Crossref] [PubMed]
- Jiang P, Gu S, Pan D, et al. Signatures of T cell dysfunction and exclusion predict cancer immunotherapy response. Nat Med 2018;24:1550-8. [Crossref] [PubMed]
- Yoshihara K, Shahmoradgoli M, Martínez E, et al. Inferring tumour purity and stromal and immune cell admixture from expression data. Nat Commun 2013;4:2612. [Crossref] [PubMed]
- Chen B, Khodadoust MS, Liu CL, et al. Profiling Tumor Infiltrating Immune Cells with CIBERSORT. Methods Mol Biol 2018;1711:243-59. [Crossref] [PubMed]
- Tang Z, Kang B, Li C, et al. GEPIA2: an enhanced web server for large-scale expression profiling and interactive analysis. Nucleic Acids Res 2019;47:W556-60. [Crossref] [PubMed]
- Feng S, Fox D, Man SM. Mechanisms of Gasdermin Family Members in Inflammasome Signaling and Cell Death. J Mol Biol 2018;430:3068-80. [Crossref] [PubMed]
- Fischer FA, Chen KW, Bezbradica JS. Posttranslational and Therapeutic Control of Gasdermin-Mediated Pyroptosis and Inflammation. Front Immunol 2021;12:661162. [Crossref] [PubMed]
- Ye Y, Dai Q, Qi H. A novel defined pyroptosis-related gene signature for predicting the prognosis of ovarian cancer. Cell Death Discov 2021;7:71. [Crossref] [PubMed]
- Lin W, Chen Y, Wu B, et al. Identification of the pyroptosis related prognostic gene signature and the associated regulation axis in lung adenocarcinoma. Cell Death Discov 2021;7:161. [Crossref] [PubMed]
- Aglietti RA, Dueber EC. Recent Insights into the Molecular Mechanisms Underlying Pyroptosis and Gasdermin Family Functions. Trends Immunol 2017;38:261-71. [Crossref] [PubMed]
- Hou J, Zhao R, Xia W, et al. PD-L1-mediated gasdermin C expression switches apoptosis to pyroptosis in cancer cells and facilitates tumour necrosis. Nat Cell Biol 2020;22:1264-75. [Crossref] [PubMed]
- Wang X, Li H, Li W, et al. The role of Caspase-1/GSDMD-mediated pyroptosis in Taxol-induced cell death and a Taxol-resistant phenotype in nasopharyngeal carcinoma regulated by autophagy. Cell Biol Toxicol 2020;36:437-57. [Crossref] [PubMed]
- Rogers C, Erkes DA, Nardone A, et al. Gasdermin pores permeabilize mitochondria to augment caspase-3 activation during apoptosis and inflammasome activation. Nat Commun 2019;10:1689. [Crossref] [PubMed]
- Li L, Jiang M, Qi L, et al. Pyroptosis, a new bridge to tumor immunity. Cancer Sci 2021;112:3979-94. [Crossref] [PubMed]
- Charoentong P, Finotello F, Angelova M, et al. Pan-cancer Immunogenomic Analyses Reveal Genotype-Immunophenotype Relationships and Predictors of Response to Checkpoint Blockade. Cell Rep 2017;18:248-62. [Crossref] [PubMed]
- Liu YT, Sun ZJ. Turning cold tumors into hot tumors by improving T-cell infiltration. Theranostics 2021;11:5365-86. [Crossref] [PubMed]