Mechanism of Radix Scutellariae in the treatment of influenza A based on network pharmacology and molecular docking
Introduction
Influenza is an acute infectious disease caused by the influenza virus. Influenza virus is divided into the following 3 types: A, B, and C. Influenza A virus belongs to the Orthomyxoviridae family. It is a single-stranded, negative RNA virus that is prone to mutation (1). An influenza pandemic is usually caused by the emergence of new or old subtypes of influenza A virus. Acute respiratory infections caused by influenza A virus have the characteristics of acute onset and strong infectivity, characterized by high fever and cough, and are often associated with pneumonia and small airway dysfunction. A few patients might have severe complications, such as acute respiratory distress syndrome, sepsis, and even death. According to the World Health Organization, approximately 650,000 people die of influenza every year worldwide (2).
At present, the main control measures for influenza are prevention and antiviral treatment. Influenza vaccine has been proved to be effective in preventing infection, but due to the strong variability of influenza virus and poor economic conditions in some areas, large-scale vaccinations are not always possible, and the popularization and application of influenza vaccine are still very limited. Influenza has not been well controlled (3). For people infected with influenza virus, the current mainstream treatment measures are neuraminidase inhibitors, such as oseltamivir. A study has shown that oseltamivir can reduce the symptoms of influenza in adults for about 17 h, but it does not reduce the number of hospitalizations for influenza-related complications (4). It can also increase adverse reactions, such as nausea and vomiting (5,6). With the emergence of virus mutations and drug resistance, the clinical effective rate of neuraminidase inhibitors in some areas might not be accurate; consequently, it is important to further explore the therapeutic drugs for influenza (7).
In recent years, increasing attention has been directed treatment options for influenza A with traditional Chinese medicine, which has become a well-researched area (8-10). At present, some traditional Chinese medicines have been confirmed to have an effect on the treatment of influenza A, and are expected to be used as supplements to first-line drugs for the treatment of influenza A (11-13). Throughout history, Chinese medicine has been used in the treatment of influenza. The traditional Chinese medicine Radix Scutellariae (RS) of Scutellaria Labiatae is a perennial herb, and is considered a good antidote, mainly for febrile disease, upper respiratory tract infection, hyperactivity cough, jaundice with damp-heat pathogen pneumonia, and other diseases, and does not produce drug resistance (14,15). In the clinical practice of traditional Chinese medicine, RS as a common medicine plays a certain role in the treatment of colds caused by heat and external pathogens (16).
Here we used network pharmacology and molecular docking technology, as well as biological information research results published in Traditional Chinese Medicine Systems Pharmacology Database and Analysis Platform (TCMSP), Online Mendelian Inheritance in Man (OMIM), STRING, GENECARD (The Human Gene Database), UniProt (https://www.uniprot.org/), RCSB PDB (Research Collaboration for Structural Bioinformatics ProteinDateBank), PubChem, and other network databases, as well as language and software, such as Perl, R, Cytoscape, AutoDock Vina, and PyMOL, combined with Gene Ontology (GO) and Kyoto Encyclopedia of Genes and Genomes (KEGG) enrichment analysis, to explore the potential components and protein-gene interaction mechanism of RS on the treatment of influenza A at the microlevel. Our aim was to create a visual display to provide evidence for the clinical application of traditional Chinese medicine on influenza A. We present the following article in accordance with the STREGA reporting checklist (available at https://atm.amegroups.com/article/view/10.21037/atm-22-1176/rc).
Methods
Screening the effective components and the predicted target genes of RS
Using TCMSP, we screened molecular IDs in the active ingredients of RS according to herb name category, with the criteria of oral bioavailability (OB) >30% and drug likeness (DL) >0.18. Through the Perl editing program version 6.4, we further screened the known molecular IDs from the UniProt protein database, and finally selected the effective components of RS and the corresponding prediction target genes. We selected these molecular IDs and genes as the target genes for the study of effective components and predicted targets of RS, and expressed them as the corresponding gene abbreviation in the UniProt database for further research.
Screening the predictive targets of influenza A
We searched the disease prediction targets of influenza A and the corresponding human protein-gene information via the GeneCards website (https://www.genecards.org/). The study was conducted in accordance with the Declaration of Helsinki (as revised in 2013). In addition, we searched “influenza” in the gene map column to export the corresponding human protein-genes through the OMIM website (https://omim.org/), and then superimposed the 2 groups of search results and removed the duplicate genes to obtain gene information of disease prediction targets.
Finding target genes
The VennDiagram software package was downloaded from the Bioconductor website (http://bioconductor.org/). The program was run in R version 4.0.3 based on the previously screened predicted target genes of RS and pretarget genes of influenza A, and the Venn diagram was drawn. We further narrowed the target gene range to common protein-genes.
Analysis of the regulatory network relationship of active components of RS
Using Perl version 6.4, we obtained the target compound list from active components, which interacted with the target genes using Cytoscape version 3.6.1. The regulatory network diagram of active components of RS was then drawn.
Protein-gene interaction analysis
Target genes were input into the toolbar of Multiple Protein, and a protein-protein interaction (PPI) network diagram was drawn following the interaction relationship between proteins and genes, which was evaluated using STRING. The top 30 proteins and genes with the number of interactive chains among the genes were ranked using R language; more than 20 interactive chains were selected for further research.
GO and KEGG pathway enrichment analyses
GO and KEGG were used for the enrichment analyses. The “DOSE” and “clusterProfiler” software packages were download from the Bioconductor database, and human species genome H19 (org. Hs. eg. db) was selected as the reference. Multiple hypothesis testing was used (set at P<0.05 and Q<0.05), and GO and KEGG enrichment analyses were done using clusterProfiler.
Molecular docking
Molecular docking was carried out, and the binding of RS compounds with influenza A virus protein was calculated by AutoDock Vina software. The main RNA coding proteins of influenza A virus included hemagglutinin (HA), NeurAminidase (NA), nucleoprotein (NP), RNA polymerase [polymerase basic protein 1 (PB1), polymerase basic protein 2 (PB2)], matrix protein (M1, M2), and non-structural protein (NS). The 3D structures were screened out from the RCSB PDB database (https://www.rcsb.org/) for further study.
The 3D structures of the 8 selected proteins were used as receptors, respectively, and the AutoDock tool (MGLTools 1.5.6) was used to remove water, molecules in solvent, and unnecessary metals, and then treated with total hydrogenation and charge calculation, before being saved in PDBQT format.
We crossed these active genes in the influenza A signaling pathway with the target genes, and were used to locate key target genes which more than 20 chains hinteracting with PPI network. We consulted the previously obtained list of active components of RS, and selected compounds rich in anchored key target genes for further study.
The 3D molecular structures of the selected compounds were used as small molecule ligands from the PubChem database (https://pubchem.ncbi.nlm.nih.gov/). The SDF format was converted into PBD format by OpenBabel software. The water content was removed by the AutoDock tool (MGLTools 1.5.6), and polar hydrogens were added. After calculating the charge, the 3D molecular structures were saved in PDBQT format.
Using AutoDock Vina molecular docking software, we set the grid box coordinates and protein receptor size. To ensure the accuracy of molecular docking, we also set the automatic configuration docking of each receptor and ligand 30 times. The influenza A virus-related protein receptor and the core target compound of RS were then docked using AutoDock Vina. We completed the docking and calculation analysis under the default setting in AutoDock Vina. According to the degree of free binding energy of molecular docking, we intercepted the free binding energy data of the optimal conformation and the corrected conformation from the calculation results, and selected the optimal conformation in the simulated molecular docking for recording.
Statistical analysis
TCMSP was used for screening molecular IDs with the OB >30% and DL >0.18. Multiple hypothesis testing was carried out with P<0.05 and Q<0.05. The software or algorithms used in the study include Perl editing program version 6.4, VennDiagram software, Cytoscape version 3.6, “DOSE” and “clusterProfiler” software, AutoDock Vina software, AutoDock tool (MGLTools 1.5.6), OpenBabel software, AutoDock tool (MGLTools 1.5.6), STRING, and autoDock Vina molecular docking software. The used databases contain UniProt protein database, GeneCards, OMIM, GO, KEGG, and PubChem database.
Results
Effective components and predicted target genes of RS
There are 143 molecular IDs in the active ingredients of RS; DL was used for screening. Thirty-six molecular IDS were obtained that met the screening conditions using the Chinese medicine pharmacology database and analysis platform, TCMSP. Using the Perl software version 6.4 editing program, we further screened 36 known molecular IDs from the UniProt protein database, and finally selected 32 effective components of RS, corresponding to 95 predicted target genes.
Predictive targets of influenza A
We searched the disease prediction targets of influenza A through the GeneCards website and found 2,575 corresponding human proteins and genes. In addition, we searched “influenza” in the gene map column through OMIM, and found 5 corresponding human proteins and genes. We superimposed 2 sets of search results, and a total of 2,577 disease prediction target genes were obtained after removing duplicate genes.
Effective components and prediction target genes of RS
VennDiagram software was downloaded from the Bioconductor website. The program was run in R version 4.0.3 based on the previously screened predicted target genes of RS and pretarget genes of influenza A. The intersection Venn diagram of 2,577 disease genes and 95 target genes of RS was obtained (Figure 1). We further narrowed the target gene range to the following 40 common proteins and genes: NOS2, PTGS1, PTGS2, PRSS1, ADRB2, RELA, BCL2, BAX, CASP3, CASP8, FASN, FASLG, ESR1, MAPK14, AKT1, CASP9, JUN, IL6, AHSA1, CXCL8, CCL2, PRKCD, FN1, MCL1, CHRM1, SLC6A3, VEGFA, FOS, MMP9, HIF1A, MPO, AHR, CYCS, CYP1A2, CHRM2, PRKCA, PON1, ADRA2A, PLAU, and MAOA.
Regulatory network relationship of active components of RS
Using Perl version 6.4, 4 components without obvious interaction were excluded from 32 active components, and 28 target compound lists were obtained, which interacted with 40 target genes (Table 1). According to the defined interaction nodes and regulatory attributes, the regulatory network diagram of active components of RS was drawn and using Cytoscape version 3.6.1. The regulatory network diagram of RS and influenza A was constructed by 28 effective components and 40 target genes (Figure 2).
Table 1
Sequence no. | Molecule ID | Molecule name | Target symbol |
---|---|---|---|
1 | MOL000228 | (2R)-7-hydroxy-5-methoxy-2-phenylchroman-4-one | ADRA1B, ADRB2, CHRM1, ESR1, PTGS1, PTGS2, SLC6A3 |
2 | MOL002917 | 5, 2', 6'-Trihydroxy-7, 8-dimethoxyflavone | NOS2, PRSS1, PTGS1, PTGS2 |
3 | MOL000552 | 5, 2'-Dihydroxy-6, 7, 8-trimethoxyflavone | NOS2, PRSS1, PTGS1, PTGS2 |
4 | MOL002909 | 5, 7, 2, 5-tetrahydroxy-8, 6-dimethoxyflavone | NOS2, PRSS1, PTGS2 |
5 | MOL002925 | 5, 7, 2', 6'-Tetrahydroxyflavone | PTGS1, PTGS2 |
6 | MOL012245 | 5, 7, 4'-trihydroxy-6-methoxyflavanone | PTGS1, PTGS2 |
7 | MOL002933 | 5, 7, 4'-Trihydroxy-8-methoxyflavone | ESR1, NOS2, PRSS1, PTGS1, PTGS2 |
8 | MOL001689 | Acacetin | ADRB2, BAX, BCL2, CASP3, CASP8, FASLG, FASN, NOS2, PRSS1, PTGS1, PTGS2, RELA |
9 | MOL002714 | Baicalein | AHR, AKT1, BAX, BCL2, CASP3, CYCS, FOS, HIF1A, MMP9, MPO, PRSS1, PTGS1, PTGS2, RELA, VEGFA |
10 | MOL000358 | Beta-sitosterol | ADRA1B, ADRB2, BAX, BCL2, CASP3, CASP8, CASP9, CHRM1, CHRM2, JUN, PON1, PRKCA, PTGS1, PTGS2 |
11 | MOL002910 | Carthamidin | PTGS1, PTGS2 |
12 | MOL001458 | Coptisine | ESR1, NOS2, PRSS1, PTGS1, PTGS2 |
13 | MOL002913 | Dihydrobaicalin_qt | PTGS1, PTGS2 |
14 | MOL002937 | Dihydrooroxylin | ADRA1B, ADRB2, PTGS1, PTGS2 |
15 | MOL002879 | Diop | ADRB2 |
16 | MOL000073 | Ent-Epicatechin | ESR1, PTGS1, PTGS2 |
17 | MOL002897 | Epiberberine | ESR1, NOS2, PRSS, 1PTGS2 |
18 | MOL002914 | Eriodyctiol (flavanone) | PTGS1, PTGS2 |
19 | MOL008206 | Moslosooflavone | ADRA1B, ADRB2, NOS2, PRSS1, PTGS1, PTGS2 |
20 | MOL002934 | Neobaicalein | ESR1, NOS2, PRSS1, PTGS2 |
21 | MOL000525 | Norwogonin | NOS2, PTGS1, PTGS2 |
22 | MOL002928 | Oroxylin A | ADRA1B, ADRB2, BCL2, CASP3, CYP1A2, IL6, NOS2, PRSS1, PTGS1, PTGS2 |
23 | MOL002932 | Panicolin | NOS2, PRSS1, PTGS1, PTGS2 |
24 | MOL012266 | Rivularin | NOS2, PRSS1, PTGS1, PTGS2 |
25 | MOL002915 | Salvigenin | ADRA1B, ADRB2, NOS2, PRSS1, PTGS1, PTGS2 |
26 | MOL002927 | Skullcapflavone II | NOS2, PRSS1, PTGS1, PTGS2 |
27 | MOL000449 | Stigmasterol | ADRA1B, ADRA2A, ADRB2, CHRM1, CHRM2, MAOA, PLAU, PTGS1, PTGS2, SLC6A3 |
28 | MOL000173 | Wogonin | ADRB2, AHSA1, AKT1, BAX, BCL2, CASP3, CASP9, CCL2, CXCL8, ESR1, FN1, IL6, JUN, MAPK14, MCL1, NOS2, PRKCD, PRSS1, PTGS1, PTGS2, RELA |
Construction of the regulatory network and PPI network of active components in RS
Using STRING, a total of 40 target genes were input into the toolbar of Multiple Protein page, then a PPI network diagram was drawn, and the interaction relationship between protein and genes was evaluated automatically. According to the analysis of connection density of RS components, 9 effective components, including acacetin, wogonin, moslosooflavone, and salvigenin, were highly expressed in RS. The 40 target genes were analyzed by PPI through STRING, and the network diagram was constructed (Figure 3). The top 30 genes with the number of interactive chains among genes were ranked using R language, as shown in Figure 4. The darker the color of the interaction chain, the thicker the line. Sixteen genes (AKT1, IL6, JUN, CXCL8, VEGFA, CASP3, FOS, CYCS, MMP9, PTGS2, CCL2, RELA, ESR1, CASP8, FN1, and MAPK14) had higher activity expression, and the 9 effective components and 16 target genes of RS had higher activity expression. For the KEGG enrichment analysis, 11 active genes were expressed in the influenza A signaling pathway. We crossed these 11 genes with 16 target genes, found more than 20 chains interacting with PPI network, and obtained the following 8 anchored key target genes: AKT1, IL6, CXCL8, CASP3, CYCS, CCL2, RELA, and CASP8.
GO and KEGG pathway enrichment analysis
A histogram of the GO analysis was obtained, which summarizes the enrichment results of the top 20 genes of BP, MF, and CC. The darker the color of the horizontal column, the less P value, the longer the column, the higher the degree of aggregation, and the better the enrichment and expression of the both. Seventy-one bioinformatic expressions were obtained by GO enrichment analysis, and the p value were all <0.05. We took the enriched expression of the top 20 comprehensive permutations (Figure 5), including cytokine receptor binding, DNA binding transcription factor binding, heme binding, tetrapyrrole binding, RNA polymerase II-specific DNA-binding transcription factor binding, protein heterodimerization activity were highly expressed.
KEGG enrichment analysis was performed (Figure 6). The deeper the red color of the bubble diagram, the larger the bubble and the greater the effect. A total of 124 signaling pathways were screened by KEGG enrichment analysis. KEGG database no. hsa05164 (https://www.kegg.jp/kegg-bin/show_pathway?hsa05164) is the analysis pathway of influenza A. The 13 gene protease (AKT1, IL6, CXCL8, CASP3, CYCS, CCL2, RELA, CASP8, PRSS1, FASLG, PRKCA, CASP9, and BAX) contained in 5 active compounds rich in RS, the upregulation of which can produce specific effects on RIG-I-like receptor signaling pathway, NOD-like receptor signaling pathway, Toll-like receptor signaling pathway, MAPK signaling pathways.
Molecular docking results
Sixteen genes in the influenza A signaling pathway with more than 20 interaction chains between 11 active genes and the PPI network were selected, and the following 8 anchored key target genes were obtained: AKT1, IL6, CXCL8, CASP3, CYCS, CCL2, RELA, and CASP8. Compared with the list of active components of RS in Table 1, these eight anchoring key target genes are rich in five compounds in RS, they are acacetin, wogonin, baicalein, oroxylin A, beta-sitosterol.
Using AutoDock Vina molecular docking software, we first set the grid box coordinates and protein receptor size according to the parameters in Table 2. At the same time, to ensure the accuracy of molecular docking, we also set the automatic configuration docking of each receptor and ligand 30 times. Eight influenza A virus related protein receptors were docked with 5 core target compounds of RS in AutoDock Vina, and completed 1,200 docking and calculation analysis by default.
Table 2
Macromolecular receptor | Grid box at the center with coordinates | Grid box with the size |
---|---|---|
HA: 4wst | x=39.165, y=–117.699, z=13.968 | 126.00Å × 126.00Å × 126.00Å |
NA: 5hun | x=113.804, y=28.484, z=–125.911 | 67.550Å × 64.333Å × 67.550Å |
NP: 3zdp | x=1.960, y=–47.375, z=–5.075 | 108.85Å × 108.85Å × 108.85Å |
PB1–PB2: 3a1g | x=–20.897, y=–2.761, z=12.645 | 37.917Å × 60.667Å × 68.250Å |
PA: 4iuj | x=–14.82, y=–24.836, z=50.209 | 70.617Å × 69.378Å × 73.094Å |
M1: 4pus | x=2.953, y=–3.758, z=26.557 | 50.794Å × 47.078Å × 73.094Å |
M2: 3bkd | x=3.529, y=13.528, z=–14.351 | 51.133Å × 49.822Å × 70.800Å |
NS: 6dgk | x=4.308, y=–16.074, z=–26.318 | 51.567Å × 56.622Å × 63.700Å |
According to the degree of free binding energy of molecular docking, we intercepted 9 free binding energy data, including the optimal structure and the corrected structure from the calculation results, and selected the optimal structure in the simulation of molecular docking for recording, as shown in Table 3. It should be noted that all the results of the above docking simulation data are calculated under the default settings of AutoDock Vina, and recorded with no human intervention and adjustment.
Table 3
Compound | Molecular formula | CAS No. | Target gene symbol | HA, 4wst, affinity score (kJ/mol) | NA, 5hun, affinity score (kJ/mol) | NP, 3zdp, affinity score (kJ/mol) | PB1–PB2 3a1g, affinity score (kJ/mol) | PA, 4iuj, affinity score (kJ/mol) | M1, 4pus, affinity score (kJ/mol) | M2, 3bkd, affinity score (kJ/mol) | NS, 6dgk, affinity score (kJ/mol) |
---|---|---|---|---|---|---|---|---|---|---|---|
Baicalein | C15H10O5 | 491-67-8 | AKT1, CASP3, CYCS, RELA | –8.4 | –7.2 | –8.2 | –7.1 | –8.2 | –9.1 | –8.9 | –7.0 |
Acacetin | C16H12O5 | 480-44-4 | CASP3, RELA, CASP8 | –7.4 | –6.7 | –7.6 | –6.8 | –8.0 | –8.6 | –8.9 | –7.0 |
Oroxylin A | C16H12O5 | 480-11-5 | IL6, CASP3 | –8.1 | –7.1 | –8.8 | –6.8 | –8.0 | –9.1 | –8.8 | –6.7 |
Wogonin | C16H12O5 | 632-85-9 | AKT1, IL6, CASP3, CXCL8, CCL2, RELA | –7.5 | –6.9 | –8.1 | –6.9 | –8.0 | –8.1 | –8.1 | –6.4 |
Beta-sitosterol | C29H50O | 83-46-5 | CASP3, CASP8 | –6.8 | –6.1 | –6.9 | –5.8 | –7.1 | –8.1 | –6.9 | –6.0 |
CAS No., Chemical Abstracts Service Registry Number; HA, hemagglutinin; NA, NeurAminidase; NP, nucleoprotein; PB1, polymerase basic protein 1; PB2, polymerase basic protein 2; PA, polymerase acidic; M1, matrix protein 1; M2, matrix protein 2; NS, non-structural protein.
Combined with the analysis of the influenza A signaling pathway, 5 compounds-acacetin, wogonin, baicalein, oroxylin A, and beta-sitosterol-which are rich in RS, were closely related to the main RNA coding proteins of influenza A virus, such as HA, Na, NP, RNA polymerase (PB1, PB2 and PA), matrix protein (M1 and M2), and NS. The 9 binding energy data of each result after molecular docking showed that the docking binding energy was less than –5 kJ/mol, indicating that the ligands and receptor proteins had strong affinity, and that the 5 core target compounds of RS could have high therapeutic benefit for influenza A. We recorded the expression of binding links with receptor amino acid residues in molecular docking results, as shown in Table 4. Taking the binding energy less than –8 kJ/mol as the screening condition, the optimal interaction results of 5 core targets (acacetin, wogonin, baicalein, oroxylin a, beta-sitosterol) of components of RS with M2, PA, NP, and HA, are shown as examples (Figure 7).
Table 4
Compound | HA, 4wst | NA, 5hun | NP, 3zdp | PB1–PB2, 3a1g | PA, 4iuj | M1, 4pus | M2, 3bkd | NS, 6dgk |
---|---|---|---|---|---|---|---|---|
Baicalein | ASP-158/3.0Å, TYR-157/3.2Å; 3.3Å | TRP-177/3.4Å, ARG-166/3.1Å; 3.1Å, ARG-154/3.0Å; 3.1Å; 3.4Å | ARG-65/3.0Å, GLN-364/3.1Å, HIS-140/2.2Å, TYR-496/2.7Å | ARG-721/3.1Å; 3.4Å, ASP-725/2.5Å, SER-0/3.1Å, ASP-729/2.0Å; 2.5Å | CYS-584/3.1Å, SER-648/3.2Å | GLN-75/2.0Å, LYS-47/3.3Å | VAL-27/2.3Å, SER-31/2.2Å, SER-31/2.1Å | LYS-175/2.7Å, TRP-203/2.1Å |
Acacetin | ASN-79/3.1Å, HIS-68/3.1Å, YLS-26/3.1Å | ARG-154/3.2Å, VAL-147/2.4Å, GLY-154/3.1Å | GLN-364/3.1Å, ARG-65/3.2Å, HIS-140/2.2Å, TYR-496/3.0Å | ARG-721/3.1Å, SER-0/2.3Å; 2.9Å | SER-648/2.8Å | GLN-75/2.1Å:2.1Å, LEU-46/3.1Å | ALA-30/3.3Å, SER-31/2.1Å | GLN-199/2.7Å; 2.8Å, VAL-194/2.2Å; 2.7Å |
Oroxylin A | GLY-155/2.3Å | ARG-116/3.3Å | ARG-152/2.9Å; 3.3Å, ASP-455/3.3Å, GLN-149/2.6Å | ASP-725/2.6Å, ARG-721/3.1Å; 3.2Å, SER-0/2.9Å | ASN-466/3.2Å, SER-648/2.9Å | LYS-47/3.3Å | SER-31/3.3Å | ARG-193/2.8Å, ARG-200/2.8Å, VAL-192/2.4Å |
Wogonin | ASP-158/3.0Å; 3.2Å, TYR-159/2.9Å, TYR-157/2.0Å | ARG-368/3.3Å, THR-366/3.1Å | GLN-149/3.3Å, GLU-495/2.0Å, ARG-152/2.9Å;3.0Å | SER-0/2.0Å; 3.1Å, ARG-721/3.1Å; 3.4Å | SER-648/2.8Å | GLN-75/2.4Å; 3.0Å, TRY-132/2.6Å | GLY-34/3.6Å, HIS-37/3.1Å | VAL-194/2.8Å; 3.3Å, ARG-200/2.9Å |
Beta-sitosterol | GLU-85/2.2Å | – | THR-151/2.4Å | – | SER-291/2.0Å; 3.2Å | ASN-85/3.0Å, ASN-87/2.0Å;3.4Å | PEG-803/ 2.1Å | ARG-200/2.8Å |
HA, hemagglutinin; NA, NeurAminidase; NP, nucleoprotein; PB1, polymerase basic protein 1; PB2, polymerase basic protein 2; PA, polymerase acidic; M1, matrix protein 1; M2, matrix protein 2; NS, non-structural protein.
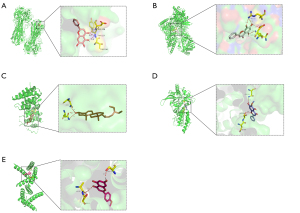
Discussion
Network pharmacology is an exemplification in drug discovery because it can provide the information of systems biology and network theory. The complexity between drug components and biological systems in a given disease scenario can be described by network pharmacology. Until now, network pharmacology is widely used to study traditional Chinese medicines. Network pharmacology-based computer simulation and experimental confirmation are designed to detect the activity of substances and therapeutic mechanisms (17-19).
In the present study, the following 5 active compounds were screened out: acacetin, wogonin, baicalein, oroxylin A, and beta-sitosterol. The results of molecular docking showed that the 5 compounds had strong affinity with the main RNA coding proteins of influenza A virus. Acacetin and the amino acid residues ala-30, ser-31 in M2 protein, wogonin and gln-149, glu-495, and arg-152 in NP protein, baicalein and asp-158/and tyr-157 in HA protein, oroxylin A and asn-466, ser-648 in PA protein, beta-sitosterol and asn-85, Asn-87 in M1 protein has stable molecular docking configuration and strong affinity, which indicates that the 5 active compounds in RS play an important role in the treatment of influenza A. Baicalein, wogonin, and oroxylin A are flavonoids, which are natural antioxidants and therapeutic agents with the properties of vitamin P, and can reduce the barrier dysfunction caused by influenza A virus, mainly acting on HA, Na, M1, M2, NS1, and NS2. Baicalein and wogonin have the highest content in RS, and could be the main treatment option for the prevention and treatment of influenza A and pulmonary endothelial barrier dysfunction (14,20).
KEGG enrichment analysis showed that the key targets were mainly concentrated in the apoptosis pathway, mitogen-activated protein kinase (MAPK) signaling pathway, tumor necrosis factor (TNF) signaling pathway, PI3K-AKT signaling pathway and nucleotide-binding and oligomerization domain-like receptor signaling pathway. After integrating the PPI network, GO enrichment analysis, and molecular docking results, we found that RS has a therapeutic effect on influenza A through the following ways: (I) the apoptosis pathway and TNF signaling pathway. As seen in KEGG database no. hsa05164 (https://www.kegg.jp/kegg-bin/show_pathway?hsa05164), upregulating AKT1 (marked by PKB in the pathway diagram) can indirectly inhibit the premature apoptosis of virus parasitic cells; CASP3 and CYCS (marked by cytc in the pathway map) were activated to regulate the apoptosis of virus parasite cells by upregulating CASP8, BAX, and CASP9.
The TNF signaling pathway is an important pathway of inflammatory response, in which related factor receptors can induce apoptosis (21); IL6 is an important inflammatory cytokine. It participates in the TNF signaling pathway and the process of inflammation and oxidative stress by upregulating RELA (NF-κB) to promotes IL6 to intervene acute respiratory distress syndrome (ARDS) caused by influenza A virus (22) (II) the MAPK signaling pathway. Many inflammatory factors, such as IL-1β, TNF-α, and IL-6, are produced through the MAPK signaling pathway (23-26). Some anti-inflammatory drugs, such as Adezmapimod, losmapimod, the p38 MAPK inhibitors, play a role in targeting the MAPK signaling pathway (27-29); (III) cellular effects. The upregulation of CCL2 (labeled with MCP1 in the pathway map) and CXCL8 (labeled with IL8 in the pathway map) can regulate the chemotaxis of monocytes and macrophages, and interfere with viral myocarditis possibly caused by influenza A virus. The translation of messenger RNA (mRNA) is indirectly affected, and then the output of viral ribonucleoprotein (RNP) and the expression of viral protein is affected by upregulating PPKCA (labeled by PKCa in the pathway map); and (IV) the PI3K-AKT signaling pathway regulates the activation of inflammatory cells and the release of inflammatory mediators, and plays a role in the chronic inflammatory response of lung and airway (22). Baicalein and wogonin can silence the anti-apoptotic effect of pulmonary fibroblasts by inhibiting the activation of AKT1 in the PI3K-AKT signaling pathway to treat pulmonary fibrosis (21,30,31). Baicalein also exerts antipulmonary fibrosis effects through the PI3K-AKT pathway (21,32).
Network pharmacology has rapidly developed as a new approach for drug development and mechanism exploration. A wide range of databases, algorithms, and visualization software facilitate the process of network pharmacology study. However, the pharmacological effects of a specific herb could be not accurately confirmed because the uncertainty of the compounds and the corresponding targets. Besides, the public databases provide limited information causing the pharmacological effects are incompletely predicted. The present study has some other limitations. For example, the results are simplified on the basis of single traditional Chinese medicine-SR. Even combined with the results of network pharmacology and molecular docking, we still do not fully understand the exact treatment mechanism of action of influenza A.
Conclusions
Based on network pharmacology and molecular docking analysis, RS is rich in core target compounds, and its mechanism of action is clearly expressed. It has a good effect on influenza A virus infection, and immunology- and inflammation-related targets and pathways through multi-component and multi-target regulation and comprehensive action, which is beneficial in the treatment of influenza A.
Acknowledgments
Funding: The study was supported by the Constructional Project of Lingnan Zhen’s Miscellaneous Disease Genre Inheritance Studio (No. [2013]233) and the Constructional Project of Zhang Zhongde Guangdong Famous Traditional Chinese Doctor Inheritance Studio (No. [2021]129); the Project of Guangdong Medical Science and Technology Research (No. C2021064); the Project of Traditional Chinese Medicine Bureau of Guangdong Province (No. 20212083); Collaborative Innovation Team Project of Guangzhou University of Chinese Medicine (Nos. 2021XK06 and 2021XK49); the Project of Guangdong Natural Science Foundation (No. 2021A1515011507).
Footnote
Reporting Checklist: The authors have completed the STREGA reporting checklist. Available at https://atm.amegroups.com/article/view/10.21037/atm-22-1176/rc
Conflicts of Interest: All authors have completed the ICMJE uniform disclosure form (available at https://atm.amegroups.com/article/view/10.21037/atm-22-1176/coif). The authors have no conflicts of interest to declare.
Ethical Statement: The authors are accountable for all aspects of the work in ensuring that questions related to the accuracy or integrity of any part of the work are appropriately investigated and resolved. The study was conducted in accordance with the Declaration of Helsinki (as revised in 2013).
Open Access Statement: This is an Open Access article distributed in accordance with the Creative Commons Attribution-NonCommercial-NoDerivs 4.0 International License (CC BY-NC-ND 4.0), which permits the non-commercial replication and distribution of the article with the strict proviso that no changes or edits are made and the original work is properly cited (including links to both the formal publication through the relevant DOI and the license). See: https://creativecommons.org/licenses/by-nc-nd/4.0/.
References
- Barberis I, Myles P, Ault SK, et al. History and evolution of influenza control through vaccination: from the first monovalent vaccine to universal vaccines. J Prev Med Hyg 2016;57:E115-20. [PubMed]
- World Health Organization. E. coli. Available online: https://www.who.int/news-room/fact-sheets/detail/e-coli [Accessed March 15, 2018]. 2018.
- Newall AT, Chaiyakunapruk N, Lambach P, et al. WHO guide on the economic evaluation of influenza vaccination. Influenza Other Respir Viruses 2018;12:211-9. [Crossref] [PubMed]
- Hurt AC, Kelly H. Debate Regarding Oseltamivir Use for Seasonal and Pandemic Influenza. Emerg Infect Dis 2016;22:949-55. [Crossref] [PubMed]
- Tejada S, Jansson M, Solé-Lleonart C, et al. Neuraminidase inhibitors are effective and safe in reducing influenza complications: meta-analysis of randomized controlled trials. Eur J Intern Med 2021;86:54-65. [Crossref] [PubMed]
- Dobson J, Whitley RJ, Pocock S, et al. Oseltamivir treatment for influenza in adults: a meta-analysis of randomised controlled trials. Lancet 2015;385:1729-37. [Crossref] [PubMed]
- Abed Y, Schibler M, Checkmahomed L, et al. Molecular pathway of influenza pan-neuraminidase inhibitor resistance in an immunocompromised patient. Antivir Ther 2019;24:581-7. [PubMed]
- He J, Li Z, Huang W, et al. Efficacy and safety of Chou-Ling-Dan granules in the treatment of seasonal influenza via combining Western and traditional Chinese medicine: protocol for a multicentre, randomised controlled clinical trial. BMJ Open 2019;9:e024800. [Crossref] [PubMed]
- Liu Z, Zhao J, Li W, et al. Computational screen and experimental validation of anti-influenza effects of quercetin and chlorogenic acid from traditional Chinese medicine. Sci Rep 2016;6:19095. [Crossref] [PubMed]
- Zhou X, Li H, Shi Z, et al. Inhibition activity of a traditional Chinese herbal formula Huang-Lian-Jie-Du-Tang and its major components found in its plasma profile on neuraminidase-1. Sci Rep 2017;7:15549. [Crossref] [PubMed]
- Xiong Y, Li NX, Duan N, et al. Traditional Chinese Medicine in Treating Influenza: From Basic Science to Clinical Applications. Front Pharmacol 2020;11:575803. [Crossref] [PubMed]
- Huang K, Zhang P, Zhang Z, et al. Traditional Chinese Medicine (TCM) in the treatment of COVID-19 and other viral infections: Efficacies and mechanisms. Pharmacol Ther 2021;225:107843. [Crossref] [PubMed]
- Ti H. Phytochemical Profiles and their Anti-inflammatory Responses Against Influenza from Traditional Chinese Medicine or Herbs. Mini Rev Med Chem 2020;20:2153-64. [Crossref] [PubMed]
- Zhi H, Jin X, Zhu H, et al. Exploring the effective materials of flavonoids-enriched extract from Scutellaria baicalensis roots based on the metabolic activation in influenza A virus induced acute lung injury. J Pharm Biomed Anal 2020;177:112876. [Crossref] [PubMed]
- Zhang Y, Lyu C, Fong SYK, et al. Evaluation of potential herb-drug interactions between oseltamivir and commonly used anti-influenza Chinese medicinal herbs. J Ethnopharmacol 2019;243:112097. [Crossref] [PubMed]
- Wang ZL, Wang S, Kuang Y, et al. A comprehensive review on phytochemistry, pharmacology, and flavonoid biosynthesis of Scutellaria baicalensis. Pharm Biol 2018;56:465-84. [Crossref] [PubMed]
- Zhang R, Zhu X, Bai H, et al. Network Pharmacology Databases for Traditional Chinese Medicine: Review and Assessment. Front Pharmacol 2019;10:123. [Crossref] [PubMed]
- Wang X, Wang ZY, Zheng JH, et al. TCM network pharmacology: A new trend towards combining computational, experimental and clinical approaches. Chin J Nat Med 2021;19:1-11. [Crossref] [PubMed]
- Zhou Z, Chen B, Chen S, et al. Applications of Network Pharmacology in Traditional Chinese Medicine Research. Evid Based Complement Alternat Med 2020;2020:1646905. [Crossref] [PubMed]
- Zhu G, Zhang J, Yang Y, et al. The Key Target and Molecular Mechanism of the Volatile Component of Scutellaria baicalensis Georgi in Acute Lung Injury Based on Network Pharmacology. Front Pharmacol 2021;12:650780. [Crossref] [PubMed]
- Ueda K, Nishimoto Y, Kimura G, et al. Repeated lipopolysaccharide exposure causes corticosteroid insensitive airway inflammation via activation of phosphoinositide-3-kinase δ pathway. Biochem Biophys Rep 2016;7:367-73. [Crossref] [PubMed]
- Wang Y, Che M, Xin J, Zheng Z, Li J, Zhang S. The role of IL-1β and TNF-α in intervertebral disc degeneration. Biomed Pharmacother 2020;131:110660. [Crossref] [PubMed]
- Ming GF, Ma XH, Xu DM, et al. PBEF promotes the apoptosis of pulmonary microvascular endothelial cells and regulates the expression of inflammatory factors and AQP1 through the MAPK pathways. Int J Mol Med 2015;36:890-6. [Crossref] [PubMed]
- Lin LP, Niu GH, Zhang XQ. Influence of lncRNA MALAT1 on septic lung injury in mice through p38 MAPK/p65 NF-κB pathway. Eur Rev Med Pharmacol Sci 2019;23:1296-304. [PubMed]
- Li J, Zheng M, Wang C, et al. Cryptotanshinone attenuates allergic airway inflammation through negative regulation of NF-κB and p38 MAPK. Biosci Biotechnol Biochem 2020;84:268-78. [Crossref] [PubMed]
- Li M, Ye J, Zhao G, et al. Gas6 attenuates lipopolysaccharide-induced TNF-α expression and apoptosis in H9C2 cells through NF-κB and MAPK inhibition via the Axl/PI3K/Akt pathway. Int J Mol Med 2019;44:982-94. [Crossref] [PubMed]
- Yeung YT, Aziz F, Guerrero-Castilla A, et al. Signaling Pathways in Inflammation and Anti-inflammatory Therapies. Curr Pharm Des 2018;24:1449-84. [Crossref] [PubMed]
- Grimes JM, Grimes KV. p38 MAPK inhibition: A promising therapeutic approach for COVID-19. J Mol Cell Cardiol 2020;144:63-5. [Crossref] [PubMed]
- Xie C, Li X, Zhu J, et al. Magnesium isoglycyrrhizinate suppresses LPS-induced inflammation and oxidative stress through inhibiting NF-kappaB and MAPK pathways in RAW264.7 cells. Bioorg Med Chem 2019;27:516-24. [Crossref] [PubMed]
- Liu K, Li D, Zheng W, et al. Discovery, Optimization, and Evaluation of Quinazolinone Derivatives with Novel Linkers as Orally Efficacious Phosphoinositide-3-Kinase Delta Inhibitors for Treatment of Inflammatory Diseases. J Med Chem 2021;64:8951-70. [Crossref] [PubMed]
- Yang Y, Song Y, Nie Q, et al. Baicalein inhibits invasion and promotes apoptosis in glioma cells through the PI3K/Akt pathway. J BUON 2021;26:395-401. [PubMed]
- Down K, Amour A, Baldwin IR, et al. Optimization of Novel Indazoles as Highly Potent and Selective Inhibitors of Phosphoinositide 3-Kinase delta for the Treatment of Respiratory Disease. J Med Chem 2015;58:7381-99. [Crossref] [PubMed]
(English Language Editor: R. Scott)