Integrative bioinformatics analysis of potential therapeutic targets and immune infiltration characteristics in dilated cardiomyopathy
Introduction
Dilated cardiomyopathy (DCM) refers to a myocardial disease characterized by ventricular dilation and impaired heart contractility (systolic and diastolic function), but the dysfunction cannot be sufficiently explained by pressure or volume overload or coronary artery disease (1). DCM is currently the major cause of systolic heart failure and is a heart transplant indication (2). However, the mechanisms underlying DCM are complex and not fully understood. Many experimental studies have shown that the cell-mediated autoimmune mechanism triggered by viral infection plays an important role in the pathophysiological processes of DCM. The significance of inflammation to DCM development has also been recognized (3,4).
MicroRNA (miRNA) is an endogenous small non-coding RNA with a length of approximately 22 nucleotides. It plays an important role in RNA silencing and the post-transcriptional regulation of gene expression (5). Research has shown that miRNA has important functions in various pathophysiological processes of cardiovascular diseases such as angiogenesis, cardiac cell contractility, plaque formation, cardiac rhythm arrangement, and cardiac cell growth (6). It has been reported that miRNAs can be involved in left ventricular reverse remodeling (LVRR) and extracellular matrix (ECM) fibrosis in DCM (7,8). Therefore, miRNAs can provide useful information for the pathophysiology of DCM.
The immunological network also contributes to the pathogenesis of DCM (9). Viral myocarditis has been identified as a major cause of DCM (10). Previous studies have reported immune cell infiltration as a typical feature of the second stage of viral myocarditis. Natural killer (NK) cells and macrophages induce the initial host immune response, leading to the production of cytokines (tumor necrosis factor-α, interleukin-1, interleukin-2, and interferon-γ) and inflammatory cell infiltration. Antigen-specific T lymphocytes and antibody-producing B cells induce the secondary immune response. Immune-mediated myocardial injuries play an important role in the progression from myocarditis to DCM (3,4,11). Researchers have also focused on the function of specific immune cells. For example, macrophages and T-helper 17 cells were identified as immune cells that regulate the transition from myocarditis to DCM (12). However, there are still few studies on the specific changes of various immune cells in DCM.
Microarray technology and bioinformatics analysis are widely used in genome-wide gene expression analysis. They have been extensively employed to identify novel diagnostic and prognostic disease biomarkers and therapeutic targets in human diseases (13,14). However, there are few studies, to our knowledge, focused on miRNAs, their target genes, and immune cells in the cardiac tissue of patients with DCM. This study combined 3 DCM datasets (GSE3585, GSE9800, and GSE84796) from the Gene Expression Omnibus (GEO) to form an integrated dataset. Batch differences were then eliminated. After screening for differentially expressed genes (DEGs), we assessed the associations between gene co-expression modules and clinical traits using weighted gene co-expression network analysis (WGCNA). Enrichment analysis was performed, and a protein-protein interaction (PPI) network and miRNA-transcription factor-mRNA (miRNA-TF-mRNA) network were constructed successively to identify the key genes, miRNAs, and pathways associated with DCM. CIBERSORT (https://cibersort.stanford.edu/) is an algorithm for estimating immune cell abundance by a versatile computational method called support vector regression (15). Finally, cells were detected using the CIBERSORT method to investigate immune cell infiltration in DCM. The study was initiated to identify underlying mechanisms, potential biomarkers, or therapeutic targets in DCM. We present the following article in accordance with the STREGA reporting checklist (available at https://atm.amegroups.com/article/view/10.21037/atm-22-732/rc).
Methods
Gene expression dataset
RNAseq data are available on the Gene Expression Omnibus database (GEO, https://www.ncbi.nlm.nih.gov/geo/). A total of 1,452 microarray expression profile datasets for human DCM were retrieved from a search of the GEO database. After careful review, 3 gene expression profiles (GSE3585, GSE9800, and GSE84796) were collected. The GSE3585 profile was based on the GPL96 platform Affymetrix Human Genome U133A array (HG-U133A), the GSE9800 profile was based on the GPL887 platform Agilent-012097 Human 1A Microarray V2 (G4110B), and the GSE84796 profile was based on the GPL14550 Agilent-028004 SurePrint G3 Human GE 8x60K microarray. All data are freely available online, and this study did not involve any experiments conducted on humans or animals. The study was conducted in accordance with the Declaration of Helsinki (as revised in 2013).
Data processing and identification of DEGs
R is a free software environment for statistical computing and graphics. The R software (Version 4.0.5) was applied for data processing and DEGs identification. The Linear Models for Microarray Analysis (LIMMA) package and impute package were used to preprocess the 3 raw datasets, matching probe ID and gene names, removing missing values, normalizing data, and performing log2 conversions (16,17). The LIMMA package was used to merge the 3 datasets into an integrated dataset. And the surrogate variable analysis (SVA) package, installed from Bioconductor (https://bioconductor.org/), was used to remove batch effects and other unwanted variations from among the 3 datasets (18). The LIMMA package was used to screen for DEGs further, with the cutoff values set as a corrected P value <0.05 and |log2 Fold change (FC)| >0.5. The R-language ggplot2 and pheatmap packages were used to draw a volcano map and a heat map of the DEGs.
Weighted gene co-expression network analysis (WGCNA)
WGCNA was performed with the R-language WGCNA package (19). The variance of each gene expression value was calculated and ranked. The top 25% were selected to build the network for WGCNA. The flashClust package was used for optimal hierarchical clustering (19). Outlier samples were removed to construct a reliable network. The soft threshold power was calculated using the pick-Soft-Threshold function in R. The adjacency matrix was created using the soft threshold. Then we transformed the adjacency matrix into a topological overlap matrix (TOM). The TOM was then applied to compute the corresponding dissimilarity (1-TOM). The gene co-expression modules were detected by using the dynamic tree cut approach. Modules were merged if the distance between them was less than 0.2. After relating modules to clinical traits, module membership (MM) and gene significance (GS) were calculated. Correlation networks between the module eigengenes and the clinical traits were then visualized. Common genes (CGs) were identified as DEGs from the integrated dataset and significant module genes that overlapped.
Gene ontology (GO) and Kyoto Encyclopedia of Genes and Genomes (KEGG) pathway enrichment analysis of CGs
GO analysis is a common, useful method for pathway-enrichment analyses and biological interpretation. GO terms can be classified into 3 categories: biological process (BP), molecular function (MF), and cellular component (CC). KEGG (https://www.genome.jp/kegg/) is a widely used pathway-related database. GO analysis and KEGG pathway enrichment analysis of CGs in this study were performed using the database for annotation, visualization, and integrated discovery (DAVID) 6.8 (https://david.ncifcrf.gov/). A corrected P value ≤0.05 and gene counts ≥2 were considered significant.
PPI network construction and hub gene identification
The search tool for the retrieval of interacting genes (STRING) database (www.string-db.org) primarily realizes the function of predicting PPI through comprehensive data from experimental results, abstracts from literature reports, and other databases. In this study, the STRING database was used to analyze the PPI network of the CGs, and a minimum interaction score ≥0.4 was set as the cutoff value. Subsequently, the PPI network was visualized using Cytoscape 3.8.2 software (20). CytoHubba, a plugin provided by Cytoscape, was used to identify the core genes in the network with 12 algorithms and provide accurate and comprehensive analyses of disease-related genes in DCM.
Construction of the miRNA-TF-mRNA regulatory network
The miRTarBase (21), Starbase (22), and Targetscan (23) databases were used to predict the target miRNAs of the CGs. A Venn diagram was created to detect overlapping miRNA lists from all 3 databases. Enrichr (http://amp.pharm.mssm.edu/Enrichr/) is a comprehensive gene set enrichment analysis web server that contains a large collection of diverse gene-set libraries available for analysis. The transcription factor database (TRANSFAC) and JASPAR position weight matrices (PWMs) sections in Enrichr were used to identify the transcription factors (TFs) that regulate the CGs. A P value ≤0.05 was selected as the cutoff point. After evaluating the miRNA-TF-mRNA regulatory relationship, Cytoscape 3.8.2 software was used to visualize the regulatory network.
Immune cell infiltration analysis
CIBERSORT is an analytical tool that estimates the abundances of member cell types in a mixed cell population using gene expression data. We downloaded the LM22 signature matrix from the CIBERSORT website (https://cibersort.stanford.edu/) and obtained the expression profiles of 22 types of immune cells. We used the CIBERSORT algorithm to quantify the relative proportion of infiltrating immune cells in DCM. A P value ≤0.05 was set as the cutoff point. Percentages for each type of immune cell in the samples were calculated and displayed in bar plots. The R-language pheatmap package was used to draw the heat map of 22 different types of immune cells in the samples, and the corrplot package was used to draw the correlation heat map, showing the correlation of 22 infiltrating immune cells. The vioplot package was used to visualize and compare the levels of immune cells in the DCM and control samples.
Statistical analysis
R software (version 4.0.5) was used for statistical analyses. The R packages applied in each step were mentioned above and could be downloaded from Bioconductor (https://bioconductor.org/). All statistical tests were two-sided, and P value <0.05 was considered statistically significant.
Results
Identification of DEGs
Three gene expression profiles (GSE3585, GSE9800, and GSE84796) were selected for this study. The GSE3585 dataset included 7 heart biopsy samples of DCM patients and 5 normal cardiac samples, the GSE9800 dataset contained 12 DCM heart tissue samples and 6 normal cardiac tissue samples, and the GSE84796 consisted of 10 DCM cardiac samples and 7 normal heart tissue samples. Specific information about the 3 datasets is presented in Table 1. A total of 53 DEGs were significantly differentially expressed, of which 34 were significantly upregulated genes and 19 were downregulated (Table S1). The top 5 upregulated genes were frizzled-related protein (FRZB/FRP), asporin (ASPN), pleckstrin homology-like domain family A member 1 (PHLDA1), complement factor H related 2 (CFH), and ornithine decarboxylase 1 (ODC1). The top 5 downregulated genes were protein phosphatase 1 regulatory inhibitor subunit 1A (PPP1R1A), chromosome 1 open reading frame 105 (C1orf105), isocitrate dehydrogenase 2 (IDH2), endonuclease G (ENDOG), and coiled-coil domain-containing 69 (CCDC69). The heatmap and volcano plot of the DEGs are shown in Figure 1A,1B, respectively.
Table 1
Dataset ID | Platform | Sample size (DCM/control) | Region |
---|---|---|---|
GSE3585 | GPL96: [HG-U133A] Affymetrix Human Genome U133A Array | 7/5 | Germany |
GSE9800 | GPL887: Agilent-012097 Human 1A Microarray (V2) G4110B | 12/6 | Japan |
GSE84796 | GPL14550: Agilent-028004 SurePrint G3 Human GE 8x60K Microarray | 10/7 | France |
GEO, Gene Expression Omnibus; DCM, dilated cardiomyopathy.
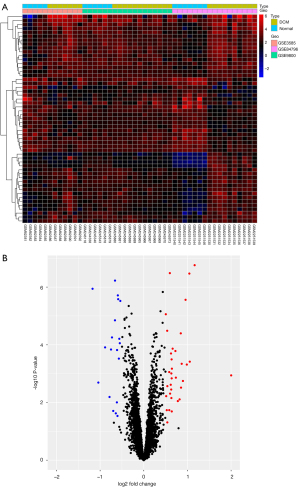
Identification of gene co-expression networks and modules
The genes with variance ranked in the top 25% (n=1,578) were selected for cluster analysis. The outlier identification threshold was set to 30, and 1 outlier sample was identified and excluded. The soft-threshold power beta value was set to 4, and the R-squared value was set to 0.8. Modules were detected using the dynamic tree cut approach, and modules with highly correlated eigengenes were merged with a merge height set to 0.2. A total of 5 modules were obtained. Genes in the same module had high connectivity and similar function (Figure 2A). Each module was assigned a unique color identifier and expression profile summarized by a module eigengene (ME). The ME in the yellow module (r=0.52, P=2E-04) exhibited the highest significant positive correlation with DCM, compared with other modules (Figure 2B). Therefore, the yellow module was considered the key module in this study. A correlation plot was drawn up to show correlations between MM and GS for the modules associated with DCM. There was a significant positive correlation (r=0.72, P=1.6E-34) between a gene’s MM and GS in the yellow module (Figure 2C). Overlap between the DEGs from the integrated dataset and significant module genes identified 41 CGs (Figure 2D).
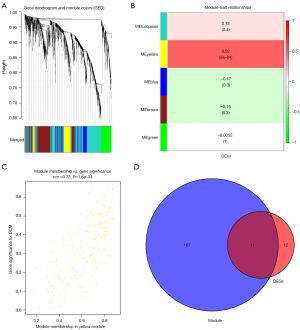
Functional enrichment analyses of CGs
The results of GO analysis indicated that CGs were mainly enriched in BPs, including collagen fibril organization, regulation of blood pressure, keratan sulfate catabolic process, and skeletal system development. In the CC ontology, the CGs were significantly enriched in the extracellular space, proteinaceous ECM, ECM, and extracellular region. MF analysis showed that the CGs were significantly enriched in heparin binding, platelet-derived growth factor binding, ECM structural constituents, and virus receptor activity. The results of KEGG pathway analysis showed that CGs were primarily enriched in protein digestion and absorption, ECM-receptor interaction, amoebiasis, and platelet activation (Figure 3, Table S2).
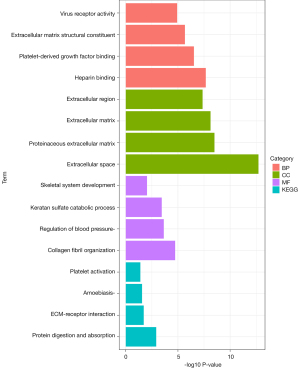
PPI network construction and hub gene identification
After removing disconnected nodes, we used the STRING tool to establish a PPI network that contained 24 nodes and 73 edges. The network included 21 upregulated and 3 downregulated genes (Figure 4). Next, we used the CytoHubba plugin to identify the highly connected genes (hub genes) in this network. The results showed that collagen type III alpha 1 chain (COL3A1) had the highest degrees of connectivity, followed by periostin (POSTN), collagen type I alpha 1 chain (COL1A1), ASPN, lumican (LUM), fibromodulin (FMOD), connective tissue growth factor (CTGF), osteoglycin (OGN), collagen type I alpha 2 chain (COL1A2), and dermatopontin (DPT) (Table S3). All these hub genes were upregulated in DCM.
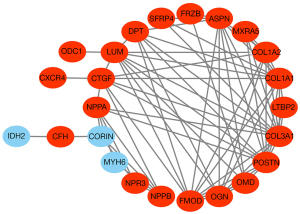
MiRNA-TF-mRNA regulatory network analysis
After the miRNA-mRNA network and TF-mRNA network were predicted, the miRNA-TF-mRNA regulatory relationships were assessed. The regulatory network included 99 miRNAs, 9 transcription factors, and 39 genes. Cytoscape software was used to visualize the integrative miRNA-TF-mRNA co-expression network (Figure 5). We discovered that FRZB and ASPN were targeted by paired related homeobox 2 (Prrx2) within the network. We also found that miR-129-5p could regulate ASPN, PHLDA1, and IDH2 at the same time.
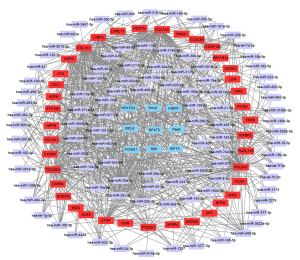
Immune cell infiltration analysis
Bar plots and heat maps showed the percentages of different immune cells in each sample (Figure 6). The immune cell infiltration violin plot showed that samples with DCM had higher levels of M1 macrophages than those in the control group (Figure 7A). The immune cell correlation relationships showed that eosinophils positively correlated with naïve CD4 T cells (r=0.9), activated memory CD4 T cells positively correlated with neutrophils (r=0.78), and resting mast cells positively correlated with M0 macrophages (r=0.75). Monocytes and resting NK cells positively correlated (r=0.74), and there was also a positive correlation between resting NK cells and activated dendritic cells (r=0.73). Neutrophils positively correlated with CD8 T cells (r=0.72). On the other hand, resting mast cells and activated mast cells negatively correlated (r=−0.66), as did naïve CD4 T cells and resting memory CD4 T cells (r=−0.53) and CD8 T cells and activated NK cells (r=−0.51) (Figure 7B).
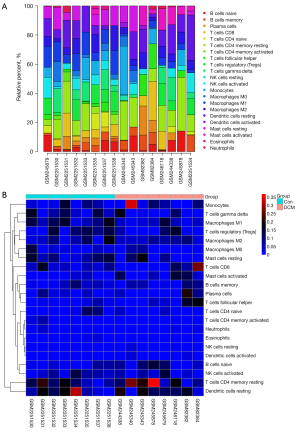
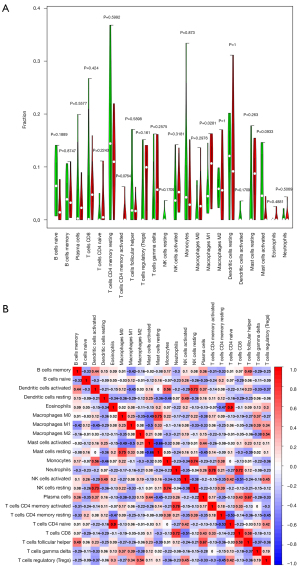
Discussion
DCM is the most common cardiomyopathy globally, yet its pathogenesis has not been fully elucidated to date. In the past, research on gene expression files was limited by small sample sizes and research skills. This study identified 53 differential genes between the DCM group and the control group of the integrated datasets, of which 34 genes were upregulated, and 19 genes were downregulated. Genes such as FRZB, ASPN, and PHLDA1 were significantly upregulated, whereas IDH2 and ENDOG were significantly downregulated.
DCM characteristics such as ventricular dilatation, hypertrophy, deterioration of cardiac function, and cardiac fibrosis have previously been observed in secreted frizzled-related protein-1 (sFRP-1 or sFRZB-1) knockout mice (24). It was reported that sFRP-1 was involved in cardiomyocyte stiffness in DCM (25), and secreted frizzled-related protein 2 (sFRP2) has been found to prevent inflammatory precursors and cardiac fibroblasts from transforming into pathogenic myofibroblasts, a critical process in DCM (26). In addition, ASPN has been found to increase significantly in DCM left ventricle samples (27), while the PHLDA1 gene mutation is differentially expressed in DCM and control groups (28). In 1 study, the conditional mutant IDH2 mice exhibited DCM, characterized by severely reduced wall motion and increased dilatation (29). Similar effects have also been described in humans, and patients with IDH2 mutations have been proven more likely to get DCM (30). One retrospective study showed that patients with acute myeloid leukemia and IDH2 mutations were more prone to cardiac dysfunction (31). In other studies, dimethyl α-ketoglutarate inhibited maladaptive autophagy IDH2-dependently in DCM (32), and ENDOG reportedly impacted canine DCM development through the apoptotic pathway (33).
The present study used WGCNA to identify 5 gene modules, among which the yellow module, including 208 genes, exhibited the highest significant positive correlation with DCM. We selected CGs from the intersection of genes identified in the WGCNA significant module and DEGs from the LIMMA analysis to make our results more rigorous. Functional enrichment analyses of the CGs revealed that DCM was primarily related to collagen fibril organization and the ECM.
The ECM provides structural support and participates in most basic cell behaviors, such as proliferation, adhesion, and migration, and is a major component of the cellular microenvironment (34). The myocardial ECM mainly comprises type I collagen, type III collagen, and elastin. Type I collagen is associated with thicker and stiffer fibers, while type III collagen is associated with thinner and more compliant fibers. Both type I and type III collagen expressions increase in DCM, and these fibrous collagens assemble into stable fibrillar networks by cross-linking, which is an essential process for ECM stabilization (35). However, newly produced collagen lacks stable cross-links and may cause ventricular dilatation. The ratio of type I to type III collagen can be considered an indicator of myocardial dilation. This ratio increases in DCM and is associated with increased stiffness and reduced elasticity in the cardiac wall (36,37). The results of the functional enrichment analyses of CGs in this study were concordant with previous studies.
ASPN is an ECM protein that belongs to the class I small leucine-rich repeat proteoglycan (SLRP) family. ASPN can bind to type I collagen and may induce collagen mineralization (38). Among its related pathways are the degradation of the ECM and ECM proteoglycans. GO annotations related to this gene include calcium ion binding and collagen binding. The present study found ASPN to be significantly upregulated in the DCM group. FRZB functions as modulators of Wnt signaling through direct interaction with Wnts (39). The Wnt signaling pathway works as an important modulator of ECM expression and regulates ECM assembly (40). PHLDA1 has been reported as involved in the regulation of the cell cycle (41), and the physical forces at the cell-ECM interfaces can regulate the cell cycle balance (42). IDH2 plays an important role in intermediary metabolism and energy production, while it has been reported that ECM can regulate energy metabolism and act as potential therapeutic targets for metabolic diseases (43). ENDOG is a protein-coding gene related to apoptosis modulation and signaling. Apoptosis plays an important role in the synthesis, deposition, and remodeling of ECM proteins (44). Based on these findings, we conjectured that FRZB, ASPN, PHLDA1, IDH2, and ENDOG could regulate DCM through the ECM signaling pathway.
Myocardial fibrosis is a major feature of DCM. Prrx2 is a protein-coding gene and belongs to the paired family of homeobox proteins. The expression patterns of Prrx2 provide evidence consistent with a role in cellular proliferation (45). The upregulation of Prrx2 has been found to promote cardiac fibrosis in myocardial infarction mice by targeting Wnt5a (46), and Prrx2 has been reported to function in the downstream activity in embryonic fibroblasts (47). Within the miRNA-TF-mRNA regulatory network, we discovered that Prrx2 could regulate both FRZB and ASPN. Our results also suggested that the miR-129-5p can act as the bridge to connect ASPN, PHLDA1, and IDH2. Inhibition of miR-129-5p can promote the synthesis of type I collagen and thus drive cell fibrogenesis (48), and downregulated miR-129-5p contributes to increased collagen expression in scleroderma fibroblasts (49). We speculated that miR-129-5p might target ASPN in regulating DCM through the ECM signaling pathway. And miR-129-5p/ASPN might be viewed as a potential therapeutic target for DCM.
The virus-induced autoimmune response plays a key role in the pathophysiologic process of DCM (50). Various immune cells trigger the anti-myocardial autoimmune process and continuously attack the myocardial tissues with specific immunopathogenic features by mediating innate and adaptive responses. However, previous immune infiltration analysis for DCM has not been very informative (51). We used CIBERSORT to evaluate the expression levels of 22 immune cells in DCM. The results showed that there was a significant difference in immune cell infiltration between DCM cardiac samples and normal heart tissue samples. The expression of M1 macrophages increased significantly in the DCM group. Therefore, M1 macrophages may be potential core immune cells, involved in the progression of DCM. Macrophages can be categorized into 2 types: M1 (proinflammatory) or M2 (anti-inflammatory). M1 macrophages can produce proinflammatory chemokines such as IL-6, IL-12 and tumor necrosis factor (TNF). In infected tissues, macrophages are first differentiated into the M1 subtype to resist pathogens (52). Viral myocarditis is an important cause of DCM and has been linked to Coxsackievirus B (CVB) infection. Even if the pathogen has been cleared, the virus may induce an immune response that causes inflammation (53). Recent studies have confirmed that macrophages play an important role in the host immune response in myocarditis. One study found the DCM myocardium infiltrated by CD4(+) and CD8(+) T lymphocytes and macrophages (54). Increased osteopontin levels in macrophages have also been reported to be a determinant of fibrosis and cardiac remodeling in a CVB3-induced myocarditis model (55). Another study detected a significantly elevated number of infiltrates in the experimental autoimmune myocarditis (EAM) model myocardium. It was indicated that the activation of macrophages was crucial to the EAM induction (56). Research has shown that high collagen expression in fibroblasts can be regulated by collagen degradation by M1 macrophage proteinases (57). Macrophages and other immune cells can directly stimulate fibrosis by activating cardiac fibroblasts and can indirectly stimulate fibrosis by synthesizing various profibrotic molecules (58). Therefore, macrophage infiltration may induce ECM remodeling by regulating fibrosis, thus functioning in DCM. There was a close association between the potential genes and miRNAs identified in our study and the immune cell infiltration. ASPN has been reported to inhibit the TLR2- and TLR4-induced proinflammatory cytokine expression in macrophages (59). PHLDA1 expression was reported to be a negative regulator of LPS-induced proinflammatory cytokine in macrophages (60). And miR-129-5p was identified to be involved in the autophagy and apoptosis of macrophages (61,62). Our findings provided a potential theory of DCM development, and suggested that M1 macrophages could possibly be used as a potential therapeutic target for DCM.
There were some limitations to our study. Firstly, we only focused on the significantly expressed genes and pathways of the enrichment analysis. Additionally, the association of immune cell infiltration and miRNAs should be further explored. Finally, the results of this study are yet to be verified through relevant in vivo and in vitro experiments. We will focus on the functional research on key genes such as ASPN, FRZB and IDH2 subsequently. The problems of the limitations will be investigated in future studies.
Conclusions
We speculate that miR-129-5p might target ASPN in regulating DCM through the ECM signaling pathway. Macrophage infiltration may lead to ECM remodeling and ultimately induce DCM.
Acknowledgments
Funding: The study was supported by National Clinical Research Center for Orthopedics, Sports Medicine & Rehabilitation and Jiangsu China-Israel Industrial Technical Research Institute Foundation (No. 2021-NCRC-CXJJ-PY-33).
Footnote
Reporting Checklist: The authors have completed the STREGA reporting checklist. Available at https://atm.amegroups.com/article/view/10.21037/atm-22-732/rc
Conflicts of Interest: All authors have completed the ICMJE uniform disclosure form (available at https://atm.amegroups.com/article/view/10.21037/atm-22-732/coif). All authors report that the study was supported by National Clinical Research Center for Orthopedics, Sports Medicine & Rehabilitation and Jiangsu China-Israel Industrial Technical Research Institute Foundation (No. 2021-NCRC-CXJJ-PY-33). The authors have no other conflicts of interest to declare.
Ethical Statement: The authors are accountable for all aspects of the work in ensuring that questions related to the accuracy or integrity of any part of the work are appropriately investigated and resolved. The study was conducted in accordance with the Declaration of Helsinki (as revised in 2013).
Open Access Statement: This is an Open Access article distributed in accordance with the Creative Commons Attribution-NonCommercial-NoDerivs 4.0 International License (CC BY-NC-ND 4.0), which permits the non-commercial replication and distribution of the article with the strict proviso that no changes or edits are made and the original work is properly cited (including links to both the formal publication through the relevant DOI and the license). See: https://creativecommons.org/licenses/by-nc-nd/4.0/.
References
- Merlo M, Cannatà A, Gobbo M, et al. Evolving concepts in dilated cardiomyopathy. Eur J Heart Fail 2018;20:228-39. [Crossref] [PubMed]
- Sinagra G, Elliott PM, Merlo M. Dilated cardiomyopathy: so many cardiomyopathies! Eur Heart J 2020;41:3784-6. [Crossref] [PubMed]
- Imanaka-Yoshida K. Inflammation in myocardial disease: From myocarditis to dilated cardiomyopathy. Pathol Int 2020;70:1-11. [Crossref] [PubMed]
- Pankuweit S, Ruppert V, Maisch B. Inflammation in dilated cardiomyopathy. Herz 2004;29:788-93. [Crossref] [PubMed]
- Lu TX, Rothenberg ME. MicroRNA. J Allergy Clin Immunol 2018;141:1202-7. [Crossref] [PubMed]
- Çakmak HA, Demir M. MicroRNA and Cardiovascular Diseases Balkan Med J 2020;37:60-71. [PubMed]
- Dziewięcka E, Totoń-Żurańska J, Wołkow P, et al. Relations between circulating and myocardial fibrosis-linked microRNAs with left ventricular reverse remodeling in dilated cardiomyopathy. Adv Clin Exp Med 2020;29:285-93. [Crossref] [PubMed]
- Rubiś P, Totoń-Żurańska J, Wiśniowska-Śmiałek S, et al. The relationship between myocardial fibrosis and myocardial microRNAs in dilated cardiomyopathy: A link between mir-133a and cardiovascular events. J Cell Mol Med 2018;22:2514-7. [Crossref] [PubMed]
- Carthy CM, Yang D, Anderson DR, et al. Myocarditis as systemic disease: new perspectives on pathogenesis. Clin Exp Pharmacol Physiol 1997;24:997-1003. [Crossref] [PubMed]
- Lasrado N, Reddy J. An overview of the immune mechanisms of viral myocarditis. Rev Med Virol 2020;30:1-14. [Crossref] [PubMed]
- Luo H, Wong J, Wong B. Protein degradation systems in viral myocarditis leading to dilated cardiomyopathy. Cardiovasc Res 2010;85:347-56. [Crossref] [PubMed]
- Hua X, Hu G, Hu Q, et al. Single-Cell RNA Sequencing to Dissect the Immunological Network of Autoimmune Myocarditis. Circulation 2020;142:384-400. [Crossref] [PubMed]
- Templin MF, Stoll D, Schrenk M, et al. Protein microarray technology. Trends Biotechnol 2002;20:160-6. [Crossref] [PubMed]
- Zhang Y, Szustakowski J, Schinke M. Bioinformatics analysis of microarray data. Methods Mol Biol 2009;573:259-84. [Crossref] [PubMed]
- Chen B, Khodadoust MS, Liu CL, et al. Profiling Tumor Infiltrating Immune Cells with CIBERSORT. Methods Mol Biol 2018;1711:243-59. [Crossref] [PubMed]
- Ritchie ME, Phipson B, Wu D, et al. limma powers differential expression analyses for RNA-sequencing and microarray studies. Nucleic Acids Res 2015;43:e47. [Crossref] [PubMed]
- Khvorykh GV, Khrunin AV. imputeqc: an R package for assessing imputation quality of genotypes and optimizing imputation parameters. BMC Bioinformatics 2020;21:304. [Crossref] [PubMed]
- Lawlor N, Marquez EJ, Lee D, et al. V-SVA: an R Shiny application for detecting and annotating hidden sources of variation in single-cell RNA-seq data. Bioinformatics 2020;36:3582-4. [Crossref] [PubMed]
- Langfelder P, Horvath S. WGCNA: an R package for weighted correlation network analysis. BMC Bioinformatics 2008;9:559. [Crossref] [PubMed]
- Shannon P, Markiel A, Ozier O, et al. Cytoscape: a software environment for integrated models of biomolecular interaction networks. Genome Res 2003;13:2498-504. [Crossref] [PubMed]
- Huang HY, Lin YC, Li J, et al. miRTarBase 2020: updates to the experimentally validated microRNA-target interaction database. Nucleic Acids Res 2020;48:D148-54. [PubMed]
- Yang JH, Li JH, Shao P, et al. starBase: a database for exploring microRNA-mRNA interaction maps from Argonaute CLIP-Seq and Degradome-Seq data. Nucleic Acids Res 2011;39:D202-9. [Crossref] [PubMed]
- Agarwal V, Bell GW, Nam JW, et al. Predicting effective microRNA target sites in mammalian mRNAs. Elife 2015;4:e05005. [Crossref] [PubMed]
- Sklepkiewicz P, Shiomi T, Kaur R, et al. Loss of secreted frizzled-related protein-1 leads to deterioration of cardiac function in mice and plays a role in human cardiomyopathy. Circ Heart Fail 2015;8:362-72. [Crossref] [PubMed]
- Jeffrey DA, Pires Da Silva J, Garcia AM, et al. Serum circulating proteins from pediatric patients with dilated cardiomyopathy cause pathologic remodeling and cardiomyocyte stiffness. JCI Insight 2021;6:e148637. [Crossref] [PubMed]
- Blyszczuk P, Müller-Edenborn B, Valenta T, et al. Transforming growth factor-β-dependent Wnt secretion controls myofibroblast formation and myocardial fibrosis progression in experimental autoimmune myocarditis. Eur Heart J 2017;38:1413-25. [PubMed]
- Zhang K, Wu M, Qin X, et al. Asporin is a Potential Promising Biomarker for Common Heart Failure. DNA Cell Biol 2021;40:303-15. [Crossref] [PubMed]
- Liu L, Huang J. Multiomics Analysis of Transcriptome, Epigenome, and Genome Uncovers Putative Mechanisms for Dilated Cardiomyopathy. Biomed Res Int 2021;2021:6653802. [Crossref] [PubMed]
- Akbay EA, Moslehi J, Christensen CL, et al. D-2-hydroxyglutarate produced by mutant IDH2 causes cardiomyopathy and neurodegeneration in mice. Genes Dev 2014;28:479-90. [Crossref] [PubMed]
- Kranendijk M, Struys EA, Gibson KM, et al. Evidence for genetic heterogeneity in D-2-hydroxyglutaric aciduria. Hum Mutat 2010;31:279-83. [Crossref] [PubMed]
- Kattih B, Shirvani A, Klement P, et al. IDH1/2 mutations in acute myeloid leukemia patients and risk of coronary artery disease and cardiac dysfunction-a retrospective propensity score analysis. Leukemia 2021;35:1301-16. [Crossref] [PubMed]
- Mariño G, Pietrocola F, Kong Y, et al. Dimethyl α-ketoglutarate inhibits maladaptive autophagy in pressure overload-induced cardiomyopathy. Autophagy 2014;10:930-2. [Crossref] [PubMed]
- Lopes R, Solter PF, Sisson DD, et al. Characterization of canine mitochondrial protein expression in natural and induced forms of idiopathic dilated cardiomyopathy. Am J Vet Res 2006;67:963-70. [Crossref] [PubMed]
- Bosman FT, Stamenkovic I. Functional structure and composition of the extracellular matrix. J Pathol 2003;200:423-8. [Crossref] [PubMed]
- Barison A, Grigoratos C, Todiere G, et al. Myocardial interstitial remodelling in non-ischaemic dilated cardiomyopathy: insights from cardiovascular magnetic resonance. Heart Fail Rev 2015;20:731-49. [Crossref] [PubMed]
- Louzao-Martinez L, Vink A, Harakalova M, et al. Characteristic adaptations of the extracellular matrix in dilated cardiomyopathy. Int J Cardiol 2016;220:634-46. [Crossref] [PubMed]
- Wittig C, Szulcek R. Extracellular Matrix Protein Ratios in the Human Heart and Vessels: How to Distinguish Pathological From Physiological Changes? Front Physiol 2021;12:708656. [Crossref] [PubMed]
- Henry SP, Takanosu M, Boyd TC, et al. Expression pattern and gene characterization of asporin. a newly discovered member of the leucine-rich repeat protein family. J Biol Chem 2001;276:12212-21. [Crossref] [PubMed]
- Lin K, Wang S, Julius MA, et al. The cysteine-rich frizzled domain of Frzb-1 is required and sufficient for modulation of Wnt signaling. Proc Natl Acad Sci U S A 1997;94:11196-200. [Crossref] [PubMed]
- Astudillo P, Larraín J. Wnt signaling and cell-matrix adhesion. Curr Mol Med 2014;14:209-20. [Crossref] [PubMed]
- Neef R, Kuske MA, Pröls E, et al. Identification of the human PHLDA1/TDAG51 gene: down-regulation in metastatic melanoma contributes to apoptosis resistance and growth deregulation. Cancer Res 2002;62:5920-9. [PubMed]
- Uroz M, Wistorf S, Serra-Picamal X, et al. Regulation of cell cycle progression by cell-cell and cell-matrix forces. Nat Cell Biol 2018;20:646-54. [Crossref] [PubMed]
- Craft CS. MAGP1, the extracellular matrix, and metabolism. Adipocyte 2015;4:60-4. [Crossref] [PubMed]
- Hinz B, Lagares D. Evasion of apoptosis by myofibroblasts: a hallmark of fibrotic diseases. Nat Rev Rheumatol 2020;16:11-31. [Crossref] [PubMed]
- Higuchi M, Yoshida S, Ueharu H, et al. PRRX1 and PRRX2 distinctively participate in pituitary organogenesis and a cell-supply system. Cell Tissue Res 2014;357:323-35. [Crossref] [PubMed]
- Bai WW, Tang ZY, Shan TC, et al. Up-regulation of paired-related homeobox 2 promotes cardiac fibrosis in mice following myocardial infarction by targeting of Wnt5a. J Cell Mol Med 2020;24:2319-29. [Crossref] [PubMed]
- Mátés L, Korpos E, Déak F, et al. Comparative analysis of the mouse and human genes (Matn2 and MATN2) for matrilin-2, a filament-forming protein widely distributed in extracellular matrices. Matrix Biol 2002;21:163-74. [Crossref] [PubMed]
- Chen Y, Ou Y, Dong J, et al. Osteopontin promotes collagen I synthesis in hepatic stellate cells by miRNA-129-5p inhibition. Exp Cell Res 2018;362:343-8. [Crossref] [PubMed]
- Nakashima T, Jinnin M, Yamane K, et al. Impaired IL-17 signaling pathway contributes to the increased collagen expression in scleroderma fibroblasts. J Immunol 2012;188:3573-83. [Crossref] [PubMed]
- Biesbroek PS, Beek AM, Germans T, et al. Diagnosis of myocarditis: Current state and future perspectives. Int J Cardiol 2015;191:211-9. [Crossref] [PubMed]
- Bracamonte-Baran W, Čiháková D. Cardiac Autoimmunity: Myocarditis. Adv Exp Med Biol 2017;1003:187-221. [Crossref] [PubMed]
- Yunna C, Mengru H, Lei W, et al. Macrophage M1/M2 polarization. Eur J Pharmacol 2020;877:173090. [Crossref] [PubMed]
- Knowlton KU. CVB infection and mechanisms of viral cardiomyopathy. Curr Top Microbiol Immunol 2008;323:315-35. [Crossref] [PubMed]
- Luppi P, Rudert W, Licata A, et al. Expansion of specific alphabeta+ T-cell subsets in the myocardium of patients with myocarditis and idiopathic dilated cardiomyopathy associated with Coxsackievirus B infection. Hum Immunol 2003;64:194-210. [Crossref] [PubMed]
- Klingel K, Kandolf R. Osteopontin: a biomarker to predict the outcome of inflammatory heart disease. Semin Thromb Hemost 2010;36:195-202. [Crossref] [PubMed]
- Barcena ML, Jeuthe S, Niehues MH, et al. Sex-Specific Differences of the Inflammatory State in Experimental Autoimmune Myocarditis. Front Immunol 2021;12:686384. [Crossref] [PubMed]
- Farris SD, Don C, Helterline D, et al. Cell-Specific Pathways Supporting Persistent Fibrosis in Heart Failure. J Am Coll Cardiol 2017;70:344-54. [Crossref] [PubMed]
- Cojan-Minzat BO, Zlibut A, Agoston-Coldea L. Non-ischemic dilated cardiomyopathy and cardiac fibrosis. Heart Fail Rev 2021;26:1081-101. [Crossref] [PubMed]
- Yamaba S, Yamada S, Kajikawa T, et al. PLAP-1/Asporin Regulates TLR2- and TLR4-induced Inflammatory Responses. J Dent Res 2015;94:1706-14. [Crossref] [PubMed]
- Peng H, Wang J, Song X, et al. PHLDA1 Suppresses TLR4-Triggered Proinflammatory Cytokine Production by Interaction With Tollip. Front Immunol 2022;13:731500. [Crossref] [PubMed]
- Frank B, Marcu A, de Oliveira Almeida Petersen AL, et al. Autophagic digestion of Leishmania major by host macrophages is associated with differential expression of BNIP3, CTSE, and the miRNAs miR-101c, miR-129, and miR-210. Parasit Vectors 2015;8:404. [Crossref] [PubMed]
- Li N, Gao Q, Zhou W, et al. MicroRNA-129-5p affects immune privilege and apoptosis of nucleus pulposus cells via regulating FADD in intervertebral disc degeneration. Cell Cycle 2020;19:933-48. [Crossref] [PubMed]
(English Language Editor: L. Roberts)