Study on the effect of the ballistocardiography-based “Internet + Smart Bed” health management system on the quality of life of elderly users with chronic diseases
Introduction
According to the data released by the Population Division of the United Nations Department of Economic and Social Affairs (2020), the number of people over 65 years of age is nearly 730 million, and is expected to exceed 1.5 billion by 2050 worldwide (1). As the elderly population continues to grow, the prevalence of chronic diseases is gradually increasing (2). Changes in demographic structures and disease spectrums have inspired new research trends in the field of medicine and health, particularly in smart sensing devices that can be used to monitor the physical health status and vital signs of patients with chronic diseases. The application of these devices can bring great benefits to patients, their family members, and nursing staff, and can alleviate the economic burden of families, relieve the psychological burden of patients, and improve the quality of health care (3).
According to the above description, many researchers have focused on ballistocardiography (BCG). BCG signaling is a descriptive method for recording cardiac blood pumping activity and causing periodic vibration of the body without direct contact (4,5). This movement is transmitted by the ballistic forces related to cardiac contraction, blood ejection, and deceleration of blood flow in large vessels. These heart-related movements are picked up and recorded by sensors (6), and the details of cardiac physiology, such as the case of valve closure (mainly in the left atrium and ventricle), can be measured by using a special form of BCG [seismocardiography (SCG)] on the chest. The main applications are for qualitative and quantitative research and for clinical applications such as heart rate monitoring, sleep structure analysis, and cardiac function monitoring and evaluation, and certain research results have been achieved (7). At present, research on BCG technology is being further explored (8-12). In 2012, the Ahmanson-University of California, Los Angeles (UCLA) Center for Cardiomyopathy (13) published a paper on building a self-health management model in a home-based setting. For patients with heart failure, sensing technology is used to collect human hemodynamic data and transmit it to the cloud, and clinicians can evaluate human health status according to the changes in data to provide customized treatment plans for patients. However, this theoretical model has not yet been put into practice.
On the above basis, our team has developed a type of Smart Bed that can obtain the motion signals of human cardiopulmonary organs by means of BCG technology (Figure 1). The sensor is installed on the bed board under the mattress near the position of the user’s chest, which hardly affects the daily life of the subject, making it possible for BCG technology to measure the health status of the body for a long period of time (14). The team has also built the “Internet + Smart Bed” (IPBS for “Internet + Smart Bed”) health management system (called “IPBS system” for short), putting into practice a theoretical model in the literature (13), to carry out daily monitoring and management of vital signs of the human body (including both healthy and non-healthy people). In this study, the IPBS health management intervention was carried out for elderly users with chronic diseases only, in order to build a health management effect evaluation model with quality of life as the evaluation index (15). See Figure 2 for key process of IPBS system for the monitoring and management of the health status of people with chronic diseases, to study the effect of the IPBS system on the quality of life of elderly users with chronic diseases in health management, to provide a basis for carrying out effective health management in institutions for the elderly, and to explore the application value of the IPBS system in the health management of chronic diseases. We present the following article in accordance with the STROBE reporting checklist (available at https://atm.amegroups.com/article/view/10.21037/atm-22-998/rc).
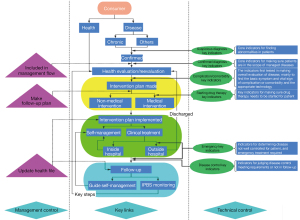
Methods
Data acquisition and research subjects
In this study, non-equivalent grouping was adopted to divide the subjects into the control group and treatment group. The control group received routine examinations and health risk management in nursing homes. The observation group, in addition to this, received services of the IPBS health management system developed by the team. The study was conducted in accordance with the Declaration of Helsinki (as revised in 2013). The study was approved by the Ethics Committee of the First Hospital of Jiaxing & The Affiliated Hospital of Jiaxing University (No. LS2019-191) and informed consent was taken from all the patients. A longitudinal design was used for data analysis. All subjects were required to complete a consent form and a questionnaire via an internet link, and were informed of the purpose of the study, the confidential nature of the study, and the requirement to complete the questionnaire anonymously.
The inclusion criteria for this study were as follows: (I) aged 60 or above; (II) elderly participants who live in pension institutions; (III) those with certain cognitive ability, able to express personal opinions clearly; (IV) those suffering from chronic diseases common in the elderly. The exclusion criteria were as follows: (I) having a serious psychological disorder or mental illness; (II) currently participating in or having participated in any other study project in the last 30 days.
According to the inclusion and exclusion criteria, 150 users who were staying in a pension institution in January 2020 were enrolled for the study. The treatment group was composed of 79 participants, and the control group comprised 71 participants. Table 1 shows the participant characteristics. The homogeneity of the subjects ensured the quality of the experiment. Among the 15 chronic diseases recorded, the disease with the highest prevalence in the treatment and control groups was hypertension (75.95% and 71.83%, respectively), followed by coronary heart disease (22.78% and 30.99%, respectively), and then diabetes (25.32% and 25.35%, respectively). The chronic diseases with a prevalence between 10% and 25% included senile cataracts and hyperlipidemia, while emphysema had the lowest prevalence. It can thus be seen that the subjects of this study were suffering mainly from elderly cardio-cerebrovascular and metabolic diseases.
Table 1
Subject | Treatment group (n=79) | Control group (n=71) | T test | P value |
---|---|---|---|---|
Sex, n (%) | 0.05924 | 0.47642 | ||
Male | 33 (41.77) | 30 (42.25) | ||
Female | 46 (58.23) | 41 (57.75) | ||
Age () | 83.84±6.49 | 82.09±5.88 | 1.72607 | 0.043218 |
Chronic diseases, n (%) | 0.14748 | 0.441477 | ||
One disease | 34 (43.04) | 28 (40.28) | ||
Two diseases or more | 45 (56.96) | 43 (59.72) | ||
Distribution of chronic diseases, n (%) | 0.1795 | 0.429418 | ||
Hypertension | 60 (75.95) | 51 (71.83) | ||
Coronary heart disease | 18 (22.78) | 22 (30.99) | ||
Diabetes | 20 (25.32) | 18 (25.35) | ||
Senile cataract | 13 (16.46) | 15 (21.13) | ||
Hyperlipidemia | 12 (15.19) | 10 (14.08) | ||
Arteriosclerosis | 8 (10.13) | 4 (5.63) | ||
Cerebral apoplexy | 4 (5.06) | 7 (9.86) | ||
Hyperplasia of the prostate | 3 (3.80) | 8 (11.27) | ||
Chronic gastritis | 4 (5.06) | 3 (4.23) | ||
Degenerative osteoarthritis | 4 (5.06) | 2 (2.82) | ||
Deafness | 6 (7.59) | 0 (0.0) | ||
Malignant tumor | 3 (3.80) | 0 (0.0) | ||
Senile pneumonia | 1 (1.27) | 1 (1.41) | ||
Dyspepsia | 1 (1.27) | 1 (1.41) | ||
Emphysema | 0 (0.0) | 1 (1.41) |
Quality of life scale
In this study, the IPBS health management system was used to monitor and follow up the health-related quality of life indicators of the elderly with chronic diseases such as hypertension, diabetes, and coronary heart disease. The quality of life scale (16) used in this study was validated for reliability based on a large sample size in the elderly population in the community, and the results showed that the scale had good reliability and validity. The structural validity, discriminant validity, internal reliability and split-half reliability are tested respectively. The results show that the reliability and validity of the scale are good. The specific reliability and validity indicators are as follows: the reliability coefficient (Cronbach α) is 0.789, and the reliability coefficient of each dimension is between 0.684 and 0.903. The correlation coefficient of the two semi-scales was 0.521. The Equal-length Spearman was 0.873 and Guttman Split-half was 0.865. Factor analysis of construct validity showed that Kaiser Meyer Olkin (KMO) was 0.761, Bartlett’s χ2=14,231.9, P<0.001; the extracted factors can explain 67.37 % of the total variance. The above indicators show that the scale has high reliability and validity for the measurement of health-related quality of life of the elderly in China. Based on this, the team pretested and optimized the scale structure to adapt it to the experimental environment of this study and ensure the effectiveness of the structure. There was a total of 47 items in this questionnaire. The dimensions of body function, activities in daily life, memory function, emotional personality, and social adaptation were retained from the original scale, while the dimensions of dietary health, sports, behavior compliance (BC), health knowledge (HK), and self-health management were added, and thus 10 dimensions were formed. The questionnaire, based on the actual situation, also added user evaluation, and then the optimized scale was modeled by means of Bayesian methods to evaluate the effect of the IPBS system on the quality of life of the subjects. In addition, this study examined the Cronbach α value of the questionnaire to determine the reliability of the study structure. Fornell and Larcker (17) suggested adopting a Correspondence analysis value greater than 0.7. In this study, the Cronbach α coefficient was calculated as 0.729, which supported the convergence reliability of this scale. We also used the KMO value to evaluate the discriminant validity of the scale, which was 0.884, indicating that the questionnaire had good validity and that our model met the recommended quality criteria.
Statistical processing
In this study, Student’s t-test (referred to as t-test hereinafter) and the Bayesian generalized linear regression model (referred to as BGLM hereinafter) were used to evaluate the improvement of the quality of life of the measured subjects. In the t-test, the t-value was used to determine whether there was any difference between the treatment and control group and between the samples before and after intervention. In the BGLM, the specific value of the mixed effects of group-time was estimated through the probability distribution of the fitted parameter coefficients. All hypothesis tests were performed using two-sided tests, and the test level α was set at 0.05. R 4.1.0 was used for statistical analysis. The results of the t-test were shown in charts, and the results of the BGLM were shown in distribution diagrams.
Results
The results of the intervention management of the subjects using IPBS system monitoring
In this study, on the basis of the vital signs calculated by the IPBS system, such as heart rate, heart rate variability, respiratory rate, body fatigue index, and the change trend of BCG’s original waveform, the physiological indicators of different subjects were distinguished, classified, and interpreted. According to the amplitude of BCG signals, the degree and proportion of abnormalities of the original waveform and their close relationship with cardiovascular disease were predicted. Through the trend of long-term changes in data, the mean value and fluctuation range of the index specific for each individual were calculated, and the long-term change in quality of life and health status were assessed (18-21).
The relevant staff, through remote access to online health information, provided advice on lifestyle changes, medication intake, physical activity adjustment, and more, and tracked the health status of the elderly in critical situations and provided timely help, so that health managers and patients themselves clearly understood the risk factors affecting health and the main daily precautions, and made personal health plans to guide health behavior.
Through a 15-month intervention of the observation group by the IPBS system, as can be seen in Figure 3, the monitoring results mainly included events such as changes in sleep phase, changes in cardiac function, mood, stress, fatigue status, being off or on the bed, and early detection of unknown diseases, which is also an important direction of the application of BCG signaling in clinical medicine.
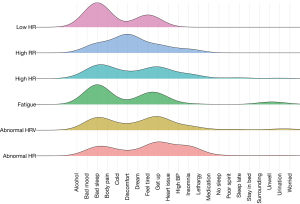
Analysis of questionnaire survey results
The experiment began in January 2020 and ended in March 2021, with the data collected in the form of questionnaires twice before the start of the experiment and in March 2021, at an interval of 15 months. The results in this section were based on a survey of quality of life for 150 subjects undergoing intervention before the start of the experiment and 15 months after the intervention (a total of 300 questionnaires were distributed, including 150 for pre-experimental baseline data and another 150 for post-intervention data). Finally, 300 copies of experimental data were received, and all 300 copies had valid data, with an efficiency of 100%.
Analysis of the effect of the IPBS system on the quality of life of patients with chronic diseases
The subjects of this study were elderly patients with chronic diseases. The experiment targeted 150 elderly people suffering from one or more chronic diseases, among whom 79 were in the treatment group which used the IPBS system and 71 were in the control group with no IPBS system. The statistical distribution of the scores of the subjects with chronic diseases before and after IPBS system intervention is shown in Figure 4, with the control group in red and the treatment group in blue, and before represents the pre-intervention score distribution and after represents the post-intervention score distribution. The statistical distribution of scores tended to be basically normal, with the scores all distributed in the interval (0, 5.0), consistent with the theoretical interval of the measurement model. Some of the data were concentrated between (4.5, 5.0), which was caused by the interference of inertial bias and social approval bias on the Likert scale (22).
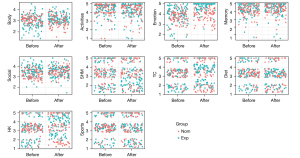
In view of the fact that the BCG technology used in the IPBS system was mainly applied for the detection of cardiovascular function and the diagnosis of cardiovascular disease, much of the work was focused on the extraction of signal features for disease-specific diagnosis, but its actual impact on quality of life was not studied. This study investigated the effect of the actual application of BCG technology, after transformation into a product, on the quality of life of the subjects. In order to assess the effect of the IPBS intervention on the management of chronic diseases in the elderly, and to evaluate the differences between the roles played by the subjects in the system in different management modes, we used the t-test for qualitative analysis and BGLM for quantitative analysis, combined with the two-sided method for analytical verification.
Chi-square test
In the evaluation model in Figure 5, we used the Chi-square test (referred to as the t-test hereinafter) to conduct a qualitative analysis on the differences between the treatment group and control group and the samples before and after intervention. In this model, it was assumed that all samples were random ones independent of each other. The degree of deviation between the actual observed value and the theoretically inferred value is shown by the t value. The actual error values between the samples were measured by means of a two-sided test, with the test level α set to 0.05.
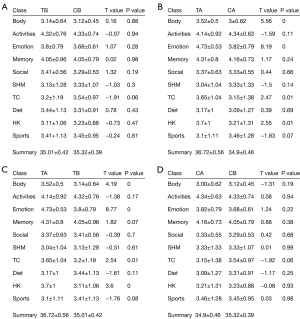
T-test (Figure 5A) analysis showed that P>0.05, indicating homogeneity and no statistical difference between the 2 groups, meeting the prerequisites of the experiment. Figure 5B shows the analysis results after health management intervention on the subjects. For body function, emotion, BC, and HK, there were significant differences (P<0.05), and the values of the t value indicators were 5.56, 8.19, 2.47, and 2.55, respectively. It can be seen that the score of the treatment group was better than that of the control group after intervention. In Figure 5C, body, emotion, TC, and HK in the treatment group were significantly different (P<0.05), and the indicators of the treatment group increased significantly after intervention. As shown in Figure 5D for the control group, P>0.05 for all indicators, demonstrating no significant differences, indicating that all indicators in the control group were basically unchanged before and after intervention.
Bayesian generalized linear regression model
In the evaluation model shown in Figure 6, the Bayesian generalized linear regression model (referred to as BGLM hereinafter) was adopted to quantify the mixed effect of group-time. In order to avoid over-fitting of the model, the hypothetical scores were made linear with the mixed effect of group-time, and the errors followed a normal distribution. Time and group were set as binary variables: 0 represented pre-intervention/control group, and 1 represented post-intervention/treatment group. By means of Bayesian posterior distribution, the probability distribution of each parameter coefficient could be calculated effectively, so as to calculate the effect of each dimension. The Markov Chain Monte Carlo method (referred to as MCMC hereinafter) was used in the sampling fitting part, and 4 chains were used in the simulation of this model. Iteration was 2,000 times, in which the first 1,000 times were used for warmup to eliminate the effect of the initial value. By comparing the historical recursion of the MCMC of each chain, it could be learned whether the iteration converged to determine whether the fitting was successful. Due to the small number of samples (N=150), compared with other analysis methods based on big data, the Bayesian method could achieve a more accurate fitting level through repeated sampling by MCMC and give a good description of the distribution of parameter coefficients. For the probability distribution of parameter coefficients, a two-sided test was used, with the test level α set to 0.05, and a distribution plot was drawn to visually display the results.
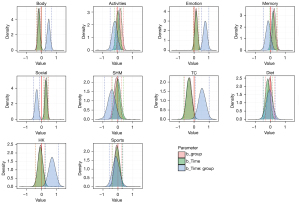
In Figure 6, a Bayesian generalized linear regression model was used to verify the interaction process between the subjects, and it could be seen by viewing the historical recursive graph of MCMC that the model had converged and the density function graph of each parameter coefficient presented a quasi-normal distribution. Therefore, the mean value of parameter coefficients could be taken as the estimated value of the parameter coefficient. The results showed that for the time parameter coefficient of body, ß1 (95% CI) =–0.12 (–0.30, 0.06), including 0, and it could be concluded that the simple effect of the time parameter was not statistically significant. The simple effect of the group parameter was ß2 (95% CI) =0.01 (–0.16, 0.19), including 0, and it could be concluded that the simple effect of group parameter was not statistically significant. The mixed effect of time-group was ß3 (95% CI) = 0.50 (0.25, 0.75), excluding 0, with significant differences from other effects, so the time-group effect was considered to be statistically significant. According to the regression coefficient ß3 of all dimensions, it could be inferred that after unit time, the subjects using the IPBS system would score 1.50 times higher in body function than before use. Similarly, emotion would be 1.79 times higher, TC 1.84 times higher, and HK 1.61 times higher than before intervention.
It can be seen that the Bayesian generalized linear regression model tended to be consistent with the analytical results of t-test effects. This can be explained by the following facts: the indicators of the elderly as the subjects in this study were improved in the dimensions of body function, emotional personality, BC, and health knowledge, because the BCG technology used in the IPBS system was very sensitive to the monitoring of changes in these indicators, and it could intervene in advance and guide the subjects to make timely changes. Other indicators such as activities in daily life, memory function, social adaptation, and sports are significantly related to the natural decline of the body of the elderly, in line with the decline of normal physiological functioning during ageing.
Discussion
This study, in which the IPBS system built with BCG technology was applied to clients, is the first in the world with innovative application value. Although previous BCG related studies conducted by other teams made the first attempt to provide an appropriate user interface to visualize real-time BCG and SCG data for future medical diagnosis (23), the field application value of the signals has not been further explored to bring practical benefits to medical or health management. The research of our team has realized the practical application of the IPBS system by conducting quantitative data analysis from horizontal and vertical aspects on the outcome factors after monitoring the physical health status of the users of IPBS system. The results showed that the user’s mood, pressure control, and heart function changes, among other factors, could be monitored through the health management mode of the IPBS system in a timely manner, in order to perceive the user’s physical condition in advance for timely intervention, so as to improve their quality of life. By evaluating the quality of life of patients with chronic diseases in the 2 groups, it could be seen from the results that the various quality of life scores of patients in the observation group were significantly higher than those in the control group, and also significantly improved compared with scores at enrollment, indicating that the implementation of the IPBS system could comprehensively improve the quality of life of patients with chronic diseases. It also makes patients pay more attention to their own physical condition, change their previous bad living habits and dietary habits, and have higher medication and BC, thus reducing the incidence of disease and reducing the incidence of complications. IPBS system can monitor human vital signs data for a long time, judge human health status, and automatically push health reminders and suggestions through APP. Give human intervention and health management according to health risk level. The health management mode based on IPBS system, even without professionals and service teams, can also play a certain role in the management of their own health through APP interaction.
This study had some limitations. Firstly, the physiological indicators based on the IPBS system had limitations and issues of accuracy in prediction, so more physiological indicators need to be included and integrated to predict the health status of the body to improve accuracy, thus improving satisfaction with the technology. Secondly, since the measurement method of effect indicators used in the experiment was mainly based on self-reported questionnaire information, there might be reporting bias in the study. The inability to blind the subjects in the treatment group might also lead the subjects to exaggerate the benefits of health management. Finally, the single-center study design was also one of the limitations of this study. It is hoped that analysis can be performed on multi-center pension institutions and various application scenarios after the IPBS system is promoted to the market in the future, so as to contribute to the establishment of an effective service model based on BCG. Therefore, future studies will learn Quality of life theory from the IPBS system to further explore whether it will affect other patient variables unrelated to compliance, such as ethics and information security.
Conclusions
This study used the IPBS system established with BCG, a revolutionary technology, to break through the restrictions of equipment and environment for the collection of human life parameters, integrate the health management service system into daily family health care services, and promote the IPBS system to become the core component of the health management approach. The effect of the IPBS system on the quality of life of elderly users with chronic diseases highlights the substantive role the IPBS system can play in the management of chronic diseases and the improvement of human life quality, and provides new ideas for the effective management of chronic diseases. This study has some practical significance. The disease monitoring and early warning functions in the IPBS system and the indicators obtained through measurement and calculation with BCG technology can be used for medical analysis, in order to achieve real-time monitoring and evaluation of the health status of the service users and provide early warning of potential diseases as an effective analysis method for the prevention and control of diseases of the elderly. This system can effectively relieve the pressure of shortages in medical resources, make up for the disconnection between daily care and professional medical care, and provide certain help for the resolution of social issues of elderly care.
Acknowledgments
Funding: This research was funded by Zhejiang Provincial Department of Science and Technology Zhejiang Industry-University-Research Institute Collaboration Association (grant No. [2019] 48), Zhejiang Primary Health Research Center (grant No. 2020JC07), the Key Discipline of Jiaxing Respiratory Medicine Construction Project (grant No.2019-zc-040), Jiaxing Key Laboratory of Precision Treatment for Lung Cancer, and the Research on Theory and Software Application of Chronic Disease Management Based on Smart Bed.
Footnote
Reporting Checklist: The authors have completed the STROBE reporting checklist. Available at https://atm.amegroups.com/article/view/10.21037/atm-22-998/rc
Data Sharing Statement: Available at https://atm.amegroups.com/article/view/10.21037/atm-22-998/dss
Conflicts of Interest: All authors have completed the ICMJE uniform disclosure form (available at https://atm.amegroups.com/article/view/10.21037/atm-22-998/coif). HC is from Keeson Technology Corporation Limited. The other authors have no conflicts of interest to declare.
Ethical Statement: The authors are accountable for all aspects of the work in ensuring that questions related to the accuracy or integrity of any part of the work are appropriately investigated and resolved. The study was conducted in accordance with the Declaration of Helsinki (as revised in 2013). The study was approved by the Ethics Committee of the First Hospital of Jiaxing &The Affiliated Hospital of Jiaxing University (No. LS2019-191) and informed consent was taken from all the patients.
Open Access Statement: This is an Open Access article distributed in accordance with the Creative Commons Attribution-NonCommercial-NoDerivs 4.0 International License (CC BY-NC-ND 4.0), which permits the non-commercial replication and distribution of the article with the strict proviso that no changes or edits are made and the original work is properly cited (including links to both the formal publication through the relevant DOI and the license). See: https://creativecommons.org/licenses/by-nc-nd/4.0/.
References
- United Nations. Department of Economic and Social Affairs, Population Division (2020). World Population Ageing 2020 Highlights: Living arrangements of older persons. United Nations Publication, 2020.
- Nijhof N, van Gemert-Pijnen LJ, Woolrych R, et al. An evaluation of preventive sensor technology for dementia care. J Telemed Telecare 2013;19:95-100. [Crossref] [PubMed]
- Kivimäki M, Steptoe A. Effects of stress on the development and progression of cardiovascular disease. Nat Rev Cardiol 2018;15:215-29. [Crossref] [PubMed]
- Inan OT, Etemadi M, Wiard RM, et al. Robust ballistocardiogram acquisition for home monitoring. Physiol Meas 2009;30:169-85. [Crossref] [PubMed]
- González-Landaeta R, Casas O, Pallàs-Areny R. Heart rate detection from an electronic weighing scale. Physiol Meas 2008;29:979-88. [Crossref] [PubMed]
- Giovangrandi L, Inan OT, Wiard RM, et al. Ballistocardiography--a method worth revisiting. Annu Int Conf IEEE Eng Med Biol Soc 2011;2011:4279-82. [PubMed]
- Xia J, Zhu W, Yang T. Research Progress of Ballistocardiogram Signal and Its Application in Medicine. China Medical Devices 2021;36:5.
- Wang Z, Zhou X, Zhao W, et al. Assessing the severity of sleep apnea syndrome based on ballistocardiogram. PLoS One 2017;12:e0175351. [Crossref] [PubMed]
- Kim CS, Carek AM, Inan OT, et al. Ballistocardiogram-Based Approach to Cuffless Blood Pressure Monitoring: Proof of Concept and Potential Challenges. IEEE Trans Biomed Eng 2018;65:2384-91. [Crossref] [PubMed]
- Liu M, Chen H. Pre-research for long-term driving monitoring system based on physiological signals. Proceedings of the 2016 6th International Conference on Advanced Design and Manufacturing Engineering (ICADME 2016), Zhuhai, China. 2016;96:685-91.
- Enayati M, Su BY, Despins L, et al. Investigating the interaction between ballistocardiogram and cardiac age, European Medical and Biomedical Engineering Conference, Tampere, Finland, June 11-15, 2017.
- Hui L. A study on the correlation between medical syndrome type and heart attack pattern and coronary artery nar-rowness in coronary heart disease and strangulation. Beijing University of Chinese Medicine, 2017.
- Bui AL, Fonarow GC. Home monitoring for heart failure management. J Am Coll Cardiol 2012;59:97-104. [Crossref] [PubMed]
- Armanfard N, Komeili M, Mihailidis A. Development of a Smart Home-Based Package for Unobtrusive Physiological Monitoring. Annu Int Conf IEEE Eng Med Biol Soc 2018;2018:4444-7. [Crossref] [PubMed]
- Nemcb KF, Němcové B, Budějovice Č. The SF-36 questionnaire: a tool to assess health-related quality of life. Retrieved on May, 2005.
- Chao J, Li Y, Xu H, et al. Health status and associated factors among the community-dwelling elderly in China. Arch Gerontol Geriatr 2013;56:199-204. [Crossref] [PubMed]
- Fornell C, Larcker DF. Structural equation models with unobservable variables and measurement error: algebra and statistics. J Mark Res 1981;18:382-8. [Crossref]
- Wang X. Thoughts on strengthening universal health management under the background of Health China Strategy. Chinese Journal of Health Management 2020;14:105-9.
- Meng Qun YX, Yu Chen. Explore a New Mode of Chronic Disease Management Via the Internet. Chinese Journal of Health Informatics & Management 2016;13:119-23.
- Inan OT, Migeotte PF, Park KS, et al. Ballistocardiography and seismocardiography: a review of recent advances. IEEE J Biomed Health Inform 2015;19:1414-27. [Crossref] [PubMed]
- Møller V, Schlemmer L, Toit S. Quality of life in South Africa: measurement and analysis. Human Sciences Research Council, Pretoria, 1987.
- Kreitchmann RS, Abad FJ, Ponsoda V, et al. Controlling for Response Biases in Self-Report Scales: Forced-Choice vs. Psychometric Modeling of Likert Items. Front Psychol 2019;10:2309. [Crossref] [PubMed]
- Jähne-Raden N, Wolf MC, Sigg S, et al. Development of a Presentation Interface for Seismo- and Ballistocardiographic Data. Stud Health Technol Inform 2020;272:103-6. [PubMed]