A predictive study of metabolism reprogramming in cervical carcinoma
Introduction
Invasive cervical carcinoma (ICC) ranks as the fourth most common female malignancy and the fourth leading cause of cancer-related mortality in women worldwide. It is responsible for more than 7% of all malignancy-related deaths in women (1,2), and 90% of cancer-related deaths in low-and middle-income countries (3,4). Although cervical cancer screening and human papillomavirus (HPV) vaccination provides effective prevention, the lack of accessibility of cancer screening and vaccine in certain regional areas poses a serious health burden (5). Almost all cases of ICC are caused by infection of the high-risk HPV (6).
Intriguingly, not all HPV infections lead to carcinogenesis. There is some evidence to suggest that HPV mediates a variety of mechanisms to evade innate and adaptive immune responses, thereby creating a complex tumor microenvironment (TME) which is conducive to persistent infections and eventually, carcinogenesis (7-9). For patients with stage I–III ICC, 15–61% of women will experience metastatic disease within the first 2 years after completing treatment (10). Once the disease progresses, second-line and subsequent-line treatment options are limited, and patients often have a poor prognosis. Currently, there is a paucity of any effective biomarkers or scoring system for evaluating the prognosis and response to immunotherapy in patients with ICC.
Metabolic reprogramming is one of the emerging hallmarks of cancer (11) and has attracted much attention over the past decade. The abnormal metabolic functions of cancer cells were first proposed by Otto Warburg, who discovered that cancer cells consumed more glucose relative to normal cells. Since then, a number of researches have focused on the cancer-related metabolic reprogramming in the metabolic pathway, including metabolic changes in glucose, amino acids, and lipids, to explore potential therapeutic targets in cancer progression. More importantly, emerging evidence indicates that cancer cells are able to suppress anti-tumor immune response by competing for and depleting essential nutrients or otherwise reducing the metabolic fitness of tumor-infiltrating immune cells.
Although metabolic reprogramming has been recently analyzed in pan-cancer or cancer-specific settings (12), to date, there have been no studies examining metabolic reprogramming in ICC.
We speculate that ICC can be divided into subtypes with distinct metabolic states according to molecular patterns, and this may provide evidence for individualized patient prognosis. This study examined the common or distinct molecular features in metabolic reprogramming relating to EMT and immune cell infiltration to assess their clinical relevance and drug resistance. We present the following article in accordance with the TRIPOD reporting checklist (available at https://atm.amegroups.com/article/view/10.21037/atm-22-981/rc).
Methods
Datasets
The study was conducted in accordance with the Declaration of Helsinki (as revised in 2013). The following datasets were obtained for analysis: The Cancer Genome Atlas Cervical Squamous Cell Carcinoma and Endocervical Adenocarcinoma (TCGA-CESC) dataset including the expression data (https://tcga-xena-hub.s3.us-east-1.amazonaws.com/download/TCGA.CESC.sampleMap%2FHiSeqV2.gz), the phenotypic data (https://tcga-xena-hub.s3.us-east-1.amazonaws.com/download/TCGA.CESC.sampleMap%2FCESC_clinicalMatrix), and the survival data (https://tcga-xena-hub.s3.us-east-1.amazonaws.com/download/survival%2FCESC_survival.txt); the GSE44001 dataset from the Gene Expression Omnibus (GEO) database (https://www.ncbi.nlm.nih.gov/geo/query/acc.cgi); and the human GTF from the Ensembl database (Homo_sapiens. GRCh38.99. GTF. Gz) (http://www.ensembl.org/info/data/ftp/index.html). The gene set related to immune cells from the List of Pan-cancer Immune Metagenes was obtained from PubMed Identifier (PMID) 28052254; metabolism-related genes were derived from PMID 33917859; and hallmark gene sets (h.a ll. Version. Symbols. GMT) (http://www.gsea-msigdb.org/gsea/index.jsp), epithelial (EPI) related genes, and mesenchymal (MES) related genes were derived from PMID 25214461. The clinical characteristics of the cases from the TCGA-CESE dataset are shown in Table 1, and the characteristics from the GSE44001 dataset are listed in Table 2.
Table 1
Clinical features | TCGA-CESC |
---|---|
HPV_types | |
HPV16 | 103 |
HPV18 | 27 |
HPV30 | 1 |
HPV31 | 3 |
HPV33 | 3 |
HPV35 | 1 |
HPV39 | 3 |
HPV45 | 9 |
HPV52 | 5 |
HPV58 | 5 |
HPV59 | 3 |
HPV68 | 1 |
HPV69 | 1 |
HPV70 | 1 |
HPV73 | 1 |
Negative | 10 |
NA | 115 |
Smoking_years | |
>40 | 3 |
≤40 | 81 |
NA | 208 |
Menopause_status | |
Peri | 25 |
Post | 81 |
Pre | 125 |
Indeterminate | 3 |
Unknown | 58 |
Grade | |
G1 | 19 |
G2 | 129 |
G3 | 117 |
G4 | 1 |
GX | 26 |
Pathologic_M | |
M0 | 107 |
M1 | 10 |
MX | 175 |
Pathologic_N | |
N0 | 129 |
N1 | 56 |
NX | 107 |
Pathologic_T | |
T1 | 137 |
T2 | 68 |
T3 | 17 |
T4 | 9 |
TX | 61 |
Radiation_therapy | |
Yes | 143 |
No | 56 |
NA | 93 |
BMI | |
>24 | 173 |
≤24 | 80 |
NA | 39 |
FIGO | |
I | 137 |
II | 68 |
III | 17 |
IV | 9 |
Unknown | 61 |
Cluster_cell | |
Cluster1 | 155 |
Cluster2 | 137 |
Cluster_metabolic | |
Cluster1 | 108 |
Cluster2 | 188 |
HPV, human papillomavirus; FIGO, International Federation of Gynecology; BMI, body mass index.
Table 2
GSE44001 | |
---|---|
OS | |
Alive | 262 |
Dead | 38 |
Stage | |
I | 258 |
II | 42 |
OS, overall survival.
Definition
Immune cell profiling
The R package GSVA (v1.34.0) software was used to calculate the enrichment score of each sample based on the collection of genes related to immune infiltrating cells. The R packet ConsensusClusterPlus (v1.50.0) was then applied to the samples based on the immune infiltration enrichment score. The unsupervised clustering algorithm used was pam and the distance was euclidean, and two immune infiltrating subgroups were obtained. Survival analysis of the two groups of samples was conducted using R package Survival (V3.2-7) and SurvMiner (V0.4.8). All data were displayed using Kaplan-Meier (KM) curves and heat maps. Clinical information such as HPV types, smoking years, menopause status, tumor grade, pathological M classification, and pathological N classification were compared between groups using Fisher’s test.
Metabolic signature
According to the list of genes related to the metabolic pathways obtained from PMID 33917859, the metabolic pathways of samples were examined. Kruskal-Wallis tests were used to detect the differences in the metabolic enrichment scores between immune infiltration groups. The related genes common to all metabolic pathways were further analyzed and the UpSet graph was constructed. The relationship between immune infiltration and metabolic enrichment was assessed using Pearson correlation coefficient and the correlation heat maps was drawn using corrplot (V0.84) software.
Metabolic subtype
Metabolic pathways with significant differences between immune infiltration groups were selected as important metabolic pathways. The expression matrix of related genes in the cleavage pathway was used for unsupervised clustering of the samples. The proportion of ambiguous clustering (PAC) algorithm was used to identify the optimal number of classifications and the metabolic subtypes were obtained. The R package ConsensusClusterPlus was used. The KMDIST clustering algorithm was applied, and the distance used was Canberra. The R package Survival and SURvMiner were then applied to analyze the survival of the subtypes and construct the KM curve. The distribution differences of menopause status, tumor grade, pathological M status, pathological N status, pathological T status, radiation therapy status, International Federation of Obstetrics and Gynecology (FIGO) grade, and cluster of immune cell within metabolic subtypes were detected by Fisher’s test, and histograms were drawn to show the significant differences. The hallmark gene set was used to perform Gene Set Enrichment Analysis (GSEA) of metabolic subtype samples. The enrichment score was obtained, plotted, and displayed using heat maps.
Statistical analysis
The differentially expressed genes (DEG) between samples of different subtypes were obtained using the R-package limma. The call threshold was |logFC| >1 and the adjusted P value was set to <0.05 two sided. The enrichment pathways were obtained and bubble maps were constructed. Combined with the OS data, batch Cox univariate regression analysis was performed on the DEGs. Genes with the greatest correlation were selected for KM analysis and subsequent analyses. Least Absolute Shrinkage and Selection Operator (LASSO) regression dimension reduction was performed on the univariate Cox regression results, and a risk scoring model was constructed. This process utilized the R package GLMNET (V4.0-2), where Y was Surv (time, event) and family was Cox. To build a more accurate regression model, lambda screening was firstly carried out by cross-validation, and then the model corresponding to Lambaa. min was selected to further extract the expression matrix of related genes in the model, and to calculate the expression matrix of each sample based on the following formula: . where exp represents the expression level of the corresponding gene, β represents the regression coefficient (COEF) of the corresponding gene in the LASSO regression results, the RScore represents the sum of the expression level of the significantly related gene in each sample multiplied by the COEF of the corresponding gene, I represents the sample, and J represents the gene. Based on the risk score of the sample, the median was used as the node to divide the high- and low-risk groups. Combined with the OS data, KM curves were drawn and the P values were calculated. A P value <0.05 was considered statistically significant. The sample risk score was further used as the model prediction result, and the area under the curve (AUC) value of the model was calculated based on the survival data, and the receiver operating characteristic (ROC) curve was drawn.
Independent data set validation
The GSE44001 dataset was downloaded from the GEO database and samples with missing survival data were omitted. Using the median as the cut-off value, the samples were divided into a high-risk and a low-risk group. By combining the survival data, KM curves were constructed and the P value was calculated, where P<0.05 was considered statistically significant. The risk score was further used as the prediction result. ROC curves were constructed and the AUC was calculated in combination with the survival data. The AUC values for 1-, 3-, and 5-year survival were greater than 0.6.
Results
The immune infiltrating subtypes and the endothelial-mesenchymal transition (EMT) scores were closely related to metabolic pathways
The relevant CESC expression data and clinical data were downloaded from the UCSC Xena database, and samples with missing OS data and those with an OS of 0 months were removed. Finally, 292 cancer samples were obtained. The expression information of 16,515 protein-coding genes was extracted from the expression data, and subsequent analyses were completed with the expression matrix.
Based on the genes associated with 28 immune infiltrating cells, ssGSEA was used to calculate the infiltrating scores in each sample. Two subtypes were obtained by unsupervised clustering, with 155 samples in cluster 1 and 137 samples in cluster 2. The KM curve showed a significant difference in survival between the two groups, and the decline of the survival curve of cluster 2 was significantly slower than that of cluster 1 (P=0.028; Figure 1A). There were significant differences in HPV type, pathological M status, and pathological N status between the immune subtypes. Based on the 28 immune infiltrating cell scores, cluster 2 samples generally had high immune infiltrating cell enrichment scores, while most cells in cluster 1 samples had low immune infiltrating cell enrichment scores. The enrichment scores of macrophages, regulatory T cell, central memory CD4 T cell, and activated CD4 T cell in cluster 2 samples were significantly higher than those in cluster1 (Figure 1B).
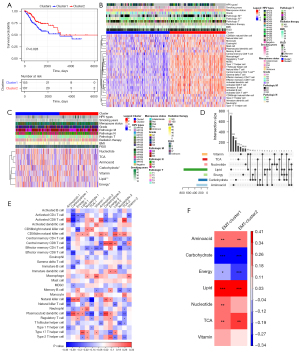
Seven genes related to metabolic pathways were obtained and the enrichment scores of seven metabolic pathways in all samples were calculated. There were significant differences between immune subtypes in the carbohydrate pathway, as well as the lipid and energy pathway (Figure 1C). Further analyses demonstrated that the lipid and vitamin pathway shared the most characteristic genes (n=26), followed by the vitamin and vitamin pathway (n=14) (Figure 1D).
The correlation between the enrichment scores of the 28 immune infiltrating cells and metabolic enrichment scores was further calculated. The heat maps demonstrated that activated CD4 T cells, activated CD8 T cells, central memory CD8 T cells, natural killer cells, and plasmacytoid dendritic cells were significantly correlated with the metabolic enrichment scores, suggesting that immune infiltrating subtypes are closely related to metabolic pathways (Figure 1E).
The correlation between EMT score and metabolic enrichment score was assessed. The results revealed that the EMT cluster 1 of the immune infiltrating subtype cluster 1 was significantly positively correlated with amino acids, lipids, TCA, and nucleotides, and significantly negatively correlated with carbohydrate and energy. The EMT cluster 2 showed significant positive correlation with amino acids, lipids, and TCA, and significant negative correlation with carbohydrate and energy. Furthermore, the correlation between EMT score and different metabolic pathways also differed between the two immune subtypes (Figure 1F).
The two metabolic subtypes contained clusters of genes that were involved in the carbohydrate pathway, as well as the lipid and energy metabolic pathway
There were significant differences in the immunoassay subtypes in the carbohydrate pathway and the lipid and energy pathway, consisting of 1103 characteristic genes. Two metabolic subtypes were obtained by unsupervised clustering (Figure 2A). The KM curve showed a significant differences in survival between the 2 metabolic subtypes (Figure 2B). The heat map and principal component analysis (PCA) both demonstrated that the expression of related genes in three important metabolic pathways differed between the two metabolic subtypes (Figure 2C,2D).
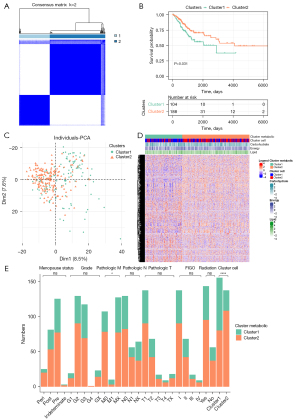
The correlation between metabolic subtypes and clinical characteristics was examined, including menopause, pathology grade, pathological M status, pathological N status, pathological T status, radiation therapy status, FIGO, and immune cell clusters The results revealed that the distribution of metabolic subtypes differed significantly between samples with different pathological M status and immune cell clusters (Figure 2E).
Gene set variation analysis (GSVA) between the two metabolic subtypes
As shown in Figure 3A, the enrichment scores of 15 hallmark pathways were significantly different between the two subtypes. Further analysis of the differentially expressed genes between subtypes revealed 1,257 differentially expressed genes between cluster 1 and cluster 2, including 237 upregulated and 1,020 downregulated genes in cluster 2 (Figure 3B).
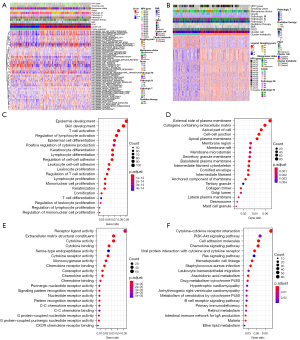
The differentially expressed genes were analyzed for functional enrichment. The Gene Ontology (GO) enrichment analysis can be divided into three parts, namely, biological process (BP), cell component (CC), and molecular function (MF). Epidermis development, skin development, and T cell activation were the main pathways enriched in the BP for cluster 1 upregulated genes. The pathways of CC enrichment mainly included external side of plasma membrane and collagen-containing extracellular matrix. MF enrichment pathways included receptor ligand activity and extracellular matrix structural constituent. Kyoto Encyclopedia of Genes and Genomes (KEGG) analysis revealed that the main pathways that were enriched included cytokine-cytokine receptor interaction and the PI3K-Akt signaling pathway (Figure 3C-3F).
Six genes were selected as prognostic indicators and 29 genes were selected for the risk scoring model
Univariate Cox regression analysis identified 178 genes with significant correlation, and the 6 genes with the greatest correlation were selected for KM analysis. The results showed that the survival curve of samples with high ELK3 (ETS Transcription Factor ELK3) expression decreased faster than that of samples with low ELK3 expression, suggesting that high expression of ELK3 may adversely affect the prognosis of patients. Conversely, the survival curve of samples with low expression of BIN2 (Bridging Integrator 2), MEI1 (Meiotic Double-Stranded Break Formation Protein 1), CCR7 (C-C Motif Chemokine Receptor 7), CYP4F12 (Cytochrome P450 Family 4 Subfamily F Member 12), and DUOX1 (Dual Oxidase 1) decreased faster than that of samples with high expression of these genes, suggesting that low expression of these genes may adversely affect the prognosis of patients (Figure 4A-4G). LASSO regression analysis was performed on the genes identified in the univariate Cox regression, Lambda varies with the coefficient of the variable, Lambda. Min obtained by cross validation and the regression coefficient corresponding to the screened variables are shown in figure 4H-4J respectively. A total of 29 genes were obtained for construction of the risk scoring model, and the following formula was obtained: Risk Score =MAK * (−0.1933) + RASSF5 * (−0.1157) + DES * (-0.0770) + MEI1 * (-0.0770) + LHX2 * (−0.0721) + CD177 * (−0.0655) + ALOX12B * (−0.0546) + SGK1 * (-0.0536) + C3orf70 * (−0.0509) + CH25H * (-0.0463) + ZNF831 * (−0.0366) + CHIT1 * (−0.0310) + LILRA4 * (−0.0248) + CCL17 * (-0.0223) + EPHB6 * (−0.0209) + KLHL6 * (−0.0035) + BIN2 * (−0.0011) +SLC24A3*0.0082 + DNAJC12 * 0.0227 + PCOLCE2 * 0.0229 + SCN2A * 0.0331 + CRABP1 * 0.0362 + CA12 * 0.0481 + PRKAA2 * 0.0515 + GPR157 * 0.0614 + GJB2 * 0.0782 + POSTN * 0.0949 + CXCL3 * 0.1150 + ELK3 * 0.2073. The samples were divided into a high- and low-risk group based on the median risk score. Combined with the OS data, the KM analysis demonstrated that the survival curves of the two groups were significantly different.
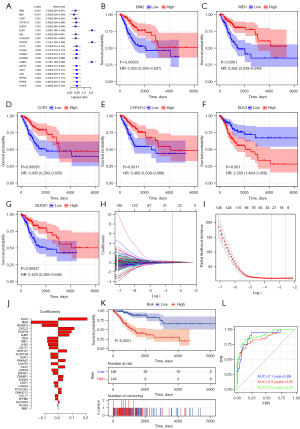
The sample risk score was then used for the prediction model, and the AUC was calculated based on the survival data. The AUC of the 1-, 3-, and 5-year survival were all greater than 0.8, indicating that the model had good performance (Figure 4K,4L).
Univariable and multivariable analysis revealed that the risk-score is an independent indicative factor of prognosis, however, neither FIGO stage nor pathological stage were risk factors for prognosis (Table 3).
Table 3
Parameter | Univariable analysis | Multivariable analysis | |||
---|---|---|---|---|---|
HR (95% CI) | P value | HR (95% CI) | P value | ||
Risk (high vs. low) | 7.233 (3.922−13.339) | <0.001 | 6.802 (3.637−12.721) | <0.001 | |
grade (G3-4 vs. G1-2) | 0.837 (0.560−1.251) | 0.467 | 0.843 (0.562−1.265) | 0.488 | |
FIGO (III–IV vs. I–II) | 1.968 (1.468−2.639) | <0.001 | 1.717 (1.286−2.292) | 0.002 |
FIGO, International Federation of Gynecology.
Independent data set validation demonstrated that the risk scoring model had good performance
The GSE44001 data was downloaded from the GEO database, scores were calculated according to the model, and KM curves were drawn. The results demonstrated significant differences in the KM curves between the high- and low-risk groups (P<0.0001; Figure 5A). The sample risk scores were then used for the model prediction, and the AUC of the model was calculated based on survival data. The AUC of 1-, 3-, and 5-year survival were all greater than 0.6, indicating that the model performance was good (Figure 5B). Figure 5C shows the curves of risk scores of all the samples in the model, and a scatter plot of the survival time by Figure 5D. A heat map of the gene expression was drawn between high and low risk groups in the risk model (Figure 5E).
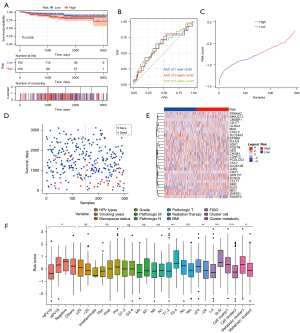
The relationship between risk and bedside characteristics was assessed. The results showed that the risk scores varied significantly with different HPV types, pathological T status, FIGO score, body mass index (BMI), immune cell clusters, and metabolic groups, suggesting that the correlating traits were consistent with our risk model (Figure 5F).
Resistance to chemotherapy and immune cell infiltration among the different risk groups
The response of samples in the low-risk group to 138 drugs was predicted (Table S1). The results revealed that the high-risk group had better resistance to nilotinib, methotrexate, cisplatin, AICAR, BIRB.0796, and lenalidomide (Figure 6A-6F).
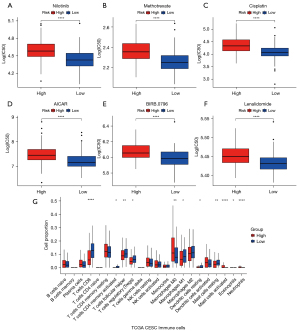
Furthermore, there were significantly greater numbers of M0 macrophages, activated mast cells, and neutrophils in the high-risk group compared to the low-risk group (P<0.05). Conversely, the low-risk group showed significantly higher numbers of CD8 T cells, follicular helper T cells, regulatory T cells (Tregs), M1 macrophages, resting dendritic cells, and mast cells compared to the high-risk group (P<0.05; Figure 6G).
Discussion
Metabolic reprogramming is an essential pathway for events that mediate malignant transformation, and it therefore plays a crucial role in the prognosis of ICC (13). This current study demonstrated that immune infiltrating subtypes is closely related to metabolic pathways, especially the carbohydrate pathway, as well as the lipid and energy metabolic pathway. Furthermore, the EMT scores demonstrated a relationship between metabolic reprogramming, TME, and EMT (14). There were also significant differences in HPV types, pathological M status, and pathological N status between the immune subtypes, suggesting that the TME is affected by both cervical carcinoma and HPV infection.
Based on the specific metabolic pathways, two clusters of metabolic subtypes with significant difference were identified. The differentially expressed genes in cluster 1 were enriched in cytokine-cytokine receptor interaction and the PI3K-Akt signaling pathway, with the latter being the most commonly activated pathway in human cancers (14). Oncogenic activation of the PI3K-Akt pathway reprograms cellular metabolism by augmenting the activity of nutrient transporters and metabolic enzymes, thereby supporting the anabolic demands of aberrantly growing cells (15). Metabolism-related prognostic factors based on immune typing were used to construct a risk proportional regression model to predict prognosis. Six genes, including ELK3, BIN2, MEI1, CCR7, CYP4F12, and DUOX1 were selected as indicators of prognosis, among which DUOX-1 as a member of NADPH oxidase family, is recognized as a special functional enzyme that can regulate the production of reactive oxygen species, has been indicated to mediates the host defense system of epithelial cells, and influences the infiltration state of immune cells in microenvironment.
The high-risk group suffered lower survival rates compared to the low-risk group, with high probability of resistance to chemotherapeutic agents including nilotinib, methotrexate, cisplatin, AICAR, BIRB.0796, and lenalidomide. It should be noted that cisplatin plays an important role in auxiliary treatment in advanced cervical carcinoma before or after surgery or in conjunction with radiation therapy. Therefore, this study may provide some clues on the choice of chemotherapy for clinicians. The risk model also provided some insights regarding immune cell infiltration in the TME. The low-risk group featured high immune cell infiltration such as CD8 T cells, M1 macrophages, and resting dendritic cells. Indeed, previous studies have suggested that immune cell infiltration in the microenvironment can influence the efficacy of immunotherapy, and cells such as CD8 T cells and M1 macrophages in the microenvironment can support immunotherapy (16,17). Therefore, the risk score model may provide insights into the potential efficacy of immunotherapy in patients with ICC.
Our study figured out the connection of metabolic reprogramming beyond the Warburg effect for cancer cells and their microenvironment, providing potential key genes of metabolic pathways in regulating the immunity of TME, however, there is still need to conduct more molecular and cellular examinations to explore the specific mechanism of metabolites regulating the immunity of TME in ICC.
Acknowledgments
Funding: None.
Footnote
Reporting Checklist: The authors have completed the TRIPOD reporting checklist. Available at https://atm.amegroups.com/article/view/10.21037/atm-22-981/rc
Conflicts of Interest: All authors have completed the ICMJE uniform disclosure form (available at https://atm.amegroups.com/article/view/10.21037/atm-22-981/coif). The authors have no conflicts of interest to declare.
Ethical Statement: The authors are accountable for all aspects of the work in ensuring that questions related to the accuracy or integrity of any part of the work are appropriately investigated and resolved. The study was conducted in accordance with the Declaration of Helsinki (as revised in 2013).
Open Access Statement: This is an Open Access article distributed in accordance with the Creative Commons Attribution-NonCommercial-NoDerivs 4.0 International License (CC BY-NC-ND 4.0), which permits the non-commercial replication and distribution of the article with the strict proviso that no changes or edits are made and the original work is properly cited (including links to both the formal publication through the relevant DOI and the license). See: https://creativecommons.org/licenses/by-nc-nd/4.0/.
References
- Bray F, Ferlay J, Soerjomataram I, et al. Global cancer statistics 2018: GLOBOCAN estimates of incidence and mortality worldwide for 36 cancers in 185 countries. CA Cancer J Clin 2018;68:394-424. [Crossref] [PubMed]
- World Health Organization: Data visualization tools for exploring the global cancer burden in 2020. Available online: https://gco.iarc.fr/today/home (accessed January 22 2021.
- Cohen PA, Jhingran A, Oaknin A, et al. Cervical cancer. Lancet 2019;393:169-82. [Crossref] [PubMed]
- UICC. Cervical cancer elimination. Available online: https://www.uicc.org/what-we-do/thematic-areas-work/cervical-cancer-elimination (accessed January 22 2021).
- Siegel RL, Miller KD, Jemal A. Cancer statistics, 2020. CA Cancer J Clin 2020;70:7-30. [Crossref] [PubMed]
- Crosbie EJ, Einstein MH, Franceschi S, et al. Human papillomavi- rus and cervical cancer. Lancet 2013;382:889-99. [Crossref] [PubMed]
- Shah W, Yan X, Jing L, et al. A reversed CD4/CD8 ratio of tumor-infiltrating lymphocytes and a high percentage of CD4(+)FOXP3(+) regulatory T cells are significantly associated with clinical outcome in squamous cell carcinoma of the cervix. Cell Mol Immunol 2011;8:59-66. [Crossref] [PubMed]
- Shibata T, Lieblong BJ, Sasagawa T, et al. The promise of combining cancer vaccine and checkpoint blockade for treating HPV-related cancer. Cancer Treat Rev 2019;78:8-16. [Crossref] [PubMed]
- Che Y, Yang Y, Suo J, et al. Induction of systemic immune responses and reversion of immunosuppression in the tumor micro- environment by a therapeutic vaccine for cervical cancer. Cancer Immunol Immunother 2020;69:2651-64. [Crossref] [PubMed]
- Pfaendler KS, Tewari KS. Changing paradigms in the systemic treatment of advanced cervical cancer. Am J Obstet Gynecol 2016;214:22-30. [Crossref] [PubMed]
- Hanahan D, Weinberg RA. Hallmarks of cancer: the next generation. Cell 2011;144:646-74. [Crossref] [PubMed]
- Sung JY, Cheong JH. Pan-Cancer Analysis Reveals Distinct Metabolic Reprogramming in Different Epithelial-Mesenchymal Transition Activity States. Cancers (Basel) 2021;13:1778. [Crossref] [PubMed]
- Fan C, Zhang S, Gong Z, et al. Emerging role of metabolic reprogramming in tumor immune evasion and immunotherapy. Sci China Life Sci 2021;64:534-47. [Crossref] [PubMed]
- Lawrence MS, Stojanov P, Mermel CH, et al. Discovery and saturation analysis of cancer genes across 21 tumour types. Nature 2014;505:495-501. [Crossref] [PubMed]
- Hoxhaj G, Manning BD. The PI3K-AKT network at the interface of oncogenic signalling and cancer metabolism. Nat Rev Cancer 2020;20:74-88. [Crossref] [PubMed]
- Kang H, Kim H, Lee S, et al. Role of Metabolic Reprogramming in Epithelial-Mesenchymal Transition (EMT). Int J Mol Sci 2019;20:2042. [Crossref]
- Li Y, Lu S, Wang S, et al. Identification of immune subtypes of cervical squamous cell carcinoma predicting prognosis and immunotherapy responses. J Transl Med 2021;19:222. [Crossref] [PubMed]