New classification for advanced breast cancer patients experiencing disease progression during salvage treatment: a single-center retrospective cohort study
Introduction
Breast cancer (BC) is the most common cancer in women worldwide (1). In 2021, more than 280,000 new cases and 43,000 deaths of BC were recorded in the United States (2). Despite dramatic progress of BC adjuvant treatment, recurrence and distant metastasis still occurs in about 30% of patients (3,4).
Tumor heterogeneity is an important feature of malignant tumor, manifesting as differences of genotype and phenotype between patients or malignant lesions of different organs in the same patient (inter-tumor heterogeneity) (5). Due to the different subtypes of tumor cells, which show various phenotypic characteristics, growth rates and invasion ability, the therapeutic sensitivity to anti-tumor treatment is diverse among various tumor lesions (6,7).
Assessment of therapeutic effect is based on the determination of response or progression. The most frequently used response evaluation criterion in clinical trial is the Response Evaluation Criteria in Solid Tumors (RECIST), firstly published in 2000 (8). The updated RECIST 1.1 further updated the assessment of tumor burden and lymph nodes.
Based on RECIST 1.1 criteria, the determination of overall response evaluation is dependent on the sum of the longest diameters for all target lesions and the evaluation of non-target lesions. The response assessment is classified as complete response (CR), partial response (PR), stable disease (SD) and progressive disease (PD). The PD status includes 3 conditions: target lesions progression, non-target lesions progression and new lesions. For patients with new lesions and no progression of target/non-target lesions, only the new lesions are deemed insensitive to systemic treatment, while the original target/non-target lesions remain under acceptably control. However, no prognostic predictive model of ABC patients considered the therapeutic responses of different lesions.
In the present study, we enrolled advanced breast cancer (ABC) patients with PD after first-line treatment and proposed 3 classification models based on the evaluation of the response status of all malignant lesions. The 3 classification models were proposed and verified to facilitate more precise identification of patients with different prognoses. We aimed to evaluate and verify the most accurate model that could further subclassify the PD status and identify patients with different prognoses. We present the following article in accordance with the STROBE reporting checklist (available at https://atm.amegroups.com/article/view/10.21037/atm-22-1618/rc).
Methods
Study population
The present study was retrospective cohort study, and electronic medical records of ABC patients from January 2018 to December 2021 at Guangdong Provincial Hospital of Chinese Medicine were retrospectively reviewed. The inclusion criteria were as follows: (I) pathologically and/or radiographically confirmed advanced metastasis; (II) age: 18–75 years old; (III) female; (IV) Eastern Cooperative Oncology Group (ECOG) score: 0–2 grade. The exclusion criteria were as follows: (I) previous immunotherapy for metastatic BC; (II) another non-measurable disease present at the time of ABC diagnosis (osteoblastic bone metastasis, meningeal lesions, ascites, pleural effusion, pericardial effusion, pulmonary cancerous lymphangitis); (III) brain metastasis; (IV) had previously received local treatment (surgery or radiotherapy) for breast tumor lesion or metastatic lesions after ABC diagnosis; (V) did not undergo regular follow-up and therapeutic evaluation; (VI) insufficient clinicopathological data.
The study was conducted in accordance with the Declaration of Helsinki (as revised in 2013). The study was approved by institutional ethics board of Guangdong Provincial Hospital of Chinese Medicine (No. ZE2020-349-01) and informed consent was taken from all the patients before inclusion.
Data collection
Age, malignancy family history data and diagnosis timing were collected for each participant. Data of pathologic characteristics were recorded, including pathologic stage, histologic type, grade, hormonal receptor status, human epidermal growth factor receptor-2 (HER2) expression and Ki-67. For treatment of ABC, the first- and second-line therapeutic information were recorded (drug regimens, duration). Furthermore, the changes of radial line of target and non-target lesions were recorded to perform therapeutic evaluation. The overall therapeutic evaluation was assessed according to the RECIST 1.1 criteria respectively.
Model construction and follow-up
In the RECIST 1.1 criteria, the following conditions are defined as PD: (I) progression of target lesions; (II) progression of non-target lesions; (III) occurrence of new lesions. Based on the permutation and combination of various conditions with potential clinical implication, several testing models were constructed for subclassification of ABC patients (Figure 1). After the therapeutic evaluation as disease progression and the initiation of second-line treatment, enrolled participants received regular symptom assessment and computerized tomography (every 2–4 cycles for chemotherapy patients and every 2–6 months for endocrine therapy patients) based on the National Comprehensive Cancer Network (NCCN) guideline. The primary end point was disease progression after the second-line treatment according to RECIST 1.1 criteria.
Statistical analyses
Mann-Whitney U test or Student’s were utilized for comparison of continuous variables, and the comparison of categorical variables was via χ2 or the Fisher’s exact test. Any locoregional recurrence, distant recurrence or contralateral primary BC was considered as disease-free survival (DFS) event among non-de novo BC patients after adjuvant treatment. The first-line progression free survival (PFS) was defined as the interval from the first diagnosis of metastasis to disease progression, and the second-line PFS was defined as the interval between the initiation of second-line treatment and the disease progression/subsequent disease progression). Patients without event at the date of last follow-up or lost to follow-up were censored. The primary outcome was second-line PFS. Kaplan-Meier survival analyses were performed to preliminarily evaluate the classification models and subgroups were compared by the log-rank test, and the most suitable model was chosen for further analyses. Multivariate analyses were performed using the Cox proportional hazards model to identify potential factors associated with second-line PFS, estimating hazard ratios (HRs) and 95% confidence intervals (95% CIs). Stratified analyses were performed to assess the difference between 2 groups of ABC patients. The software SPSS 19.0 and GraphPad Prism 9.3 were used for all analyses, and 2-tailed P≤0.05 was considered statistically significant.
Results
Clinicopathologic characteristics of participants
A total of 70 ABC patients were identified and finally enrolled in the study analyses (Figure 2). Table 1 showed the baseline clinicopathologic characteristics. There were 25 de novo metastatic BC patients, with 45 participants experiencing disease recurrence during the postoperative follow-up. There were 62.9% and 38.6% of overall participants were estrogen receptor-positive/progesterone receptor-positive and HER2-positive respectively. Seventy percent of patients received chemotherapy as first-line salvage treatment, and all HER2-positive ABC participants received anti-HER2 therapies (trastuzumab, pertuzumab or lapatinib).
Table 1
Characteristics | Overall (N=70) | Heterogeneous PD (N=38) | Homogeneous PD (N=32) | P value |
---|---|---|---|---|
Age, years | 49.71±10.28 | 49.87±10.06 | 49.53±10.68 | 0.981 |
Initial stage, n (%) | 0.775 | |||
Stage I–III | 45 (64.3) | 25 (65.8) | 20 (62.5) | |
Stage IV | 25 (35.7) | 13 (34.2) | 12 (37.5) | |
HR status, n (%) | 0.580 | |||
Negative | 26 (37.1) | 13 (34.2) | 13 (40.6) | |
Positive | 44 (62.9) | 25 (65.8) | 19 (59.4) | |
HER2 status, n (%) | 0.866 | |||
Negative | 43 (61.4) | 23 (60.5) | 20 (62.5) | |
Positive | 27 (38.6) | 15 (39.5) | 12 (37.5) | |
Metastatic sites, n (%) | 0.834 | |||
Visceral | 49 (70.0) | 27 (71.1) | 22 (68.8) | |
Bone & soft tissue | 21 (30.0) | 11 (28.9) | 10 (31.2) | |
First-line chemotherapy#, n (%) | 0.567 | |||
Taxane-containing regimens | 27 (54.0) | 17 (58.6) | 10 (47.6) | |
Non taxane-containing regimens | 23 (46.0) | 12 (41.4) | 11 (52.4) | |
First-line endocrine therapy#, n (%) | 0.218 | |||
AI | 17 (85.0) | 9 (100) | 8 (72.7) | |
FUL | 3 (15.0) | 0 (0) | 3 (27.3) | |
Partial drug retainment, n (%) | 0.147 | |||
Yes | 19 (27.1) | 13 (34.2) | 6 (18.7) | |
No | 51 (72.9) | 25 (65.8) | 26 (81.3) | |
DFS, months* | 48.73±36.96 | 49.96±37.89 | 47.18±36.68 | 0.247 |
First-line PFS, months | 7.96±1.23 | 7.96±1.81 | 7.50±1.18 | 0.658 |
*, for the de novo advanced breast cancer patients, the DFS information were not collected; #, 50 patients received chemotherapy as first-line treatment and the remaining 20 patients received endocrine therapy. HR, hormone receptor; HER2, human epidermal growth factor receptor-2; AI, aromatase inhibitor; Ful, fulvestrant; DFS, disease-free survival; PFS, progression-free survival.
Models analyses and patient subgroup definition
The mean follow-up time was 10.36 months and 94.3% of participants experienced PD during second-line therapy at the end of the follow-up. The second-line treatment was based on the respective clinician decision, and the median second-line PFS for the overall cohort was 7.10 (95% CI: 4.21–10.01) months. Ultimately, 3 testing models were proposed (details in Table 2). The survival curves of the 3 testing models were shown in the Figure 3, and the results indicated that Model C could further distinctly classify ABC patients with different PFS during second-line treatment. Therefore, based on Model C, we defined group 2 as the homogeneous progression-disease (Hom-PD) group (all the target and non-target lesions were evaluated as PD based on RECIST 1.1, with or without new lesions), and group 1 as heterogeneous progression-disease (Heter-PD) group.
Table 2
Models | Group 1 | Group 2 |
---|---|---|
Model A | Only new lesion occurrence or non-target lesions progression | The other patients assessed as disease progression |
Model B | Only new lesion occurrence, target and non-target lesions remain stable or response | The other patients assessed as disease progression |
Model C | The other patients assessed as disease progression | All the target and non-target lesions assessed as disease progression, with or without new lesions |
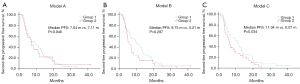
Among all participants, 54.3% (n=38) of ABC patients experienced Heter-PD after first-line treatment, while Hom-PD was observed among 32 participants (45.7%). Clinicopathologic characteristics between the Heter-PD and Hom-PD groups were compared and no significant difference was proven (Table 1). The median first-line PFS was similar between these 2 groups (7.96 vs. 7.50 months, P=0.658). The second-line PFS was significantly different between Heter-PD and Hom-PD groups (11.04 vs. 6.07 months, HR =0.604, 95% CI: 0.366–0.998, P=0.49, Figure 3C).
Identification of independent prognostic parameters
In the univariate and multivariate survival analyses evaluating the prognostic factors of second-line PFS (Table 3), the results indicated that the mode of disease progression was significantly associated with second-line PFS (HR =0.570, 95% CI: 0.338–0.960, P=0.034). Whereas, age, initial stage, hormone receptor status, and HER2 status did not significantly influence survival of ABC patients during the second-line treatment (Table 3, all P>0.05).
Table 3
Variables | Univariate analysis | Multivariate analysis | |||
---|---|---|---|---|---|
HR (95% CI) | P value | HR (95% CI) | P value | ||
Age at BC diagnosis (<45 vs. ≥45 years) | 1.022 (0.611–1.708) | 0.935 | 0.812 (0.446–1.479) | 0.497 | |
Initial stage (stage I–III vs. stage IV) | 1.133 (0.954–1.346) | 0.154 | 1.146 (0.956–1.373) | 0.141 | |
Hormone receptor status | 1.362 (0.819–2.267) | 0.234 | 1.239 (0.726–2.113) | 0.432 | |
HER2 status | 0.847 (0.516–1.390) | 0.511 | 0.732 (0.436–1.230) | 0.239 | |
First-line PFS | 0.980 (0.960–1.002) | 0.037 | 0.972 (0.949–0.996) | 0.020 | |
Heter-PD vs. Hom-PD | 0.604 (0.366–0.998) | 0.049 | 0.570 (0.338–0.960) | 0.034 |
HR, hazard ratio; CI, confidence interval; BC, breast cancer; HER2, human epidermal growth factor receptor-2; PFS, progression-free survival; Heter-PD, heterogeneous progression disease; Hom-PD, homogeneous progression disease.
Among visceral metastases subgroup, ABC patients with Heter-PD experienced longer second-line PFS compared with Hom-PD ABC patients (9.50 vs. 4.28 months, P=0.045, Figure 4), and a similar tendency was observed in the bone/soft tissue metastases subgroup but no statistical significance was revealed (HR =0.508, 95% CI: 0.171–1.509, P=0.223) in the subgroup survival analyses. For ABC patients with negative hormone receptor, heterogeneous mode of disease progression was also associated with better second-line PFS (13.85 vs. 6.07 months, P=0.001, Figure 4). Furthermore, the length of first-line PFS could influence the survival outcome of the 2 groups. Among ABC patients who experienced shorter first-line PFS (<6 months), the Heter-PD group had superior second-line PFS compared with the Hom-PD group (11.14 vs. 3.78 months, P=0.001). In subgroup analyses based on the treatment regimens, the result showed that the second-line PFS of Heter-PD group was significantly longer than that of Hom-PD group in chemotherapy subgroup (11.04 vs. 5.79 months, P=0.043). No statistical difference of second-line PFS between groups was found in the endocrine treatment groups (9.75 vs. 8.54 months, P=0.764).
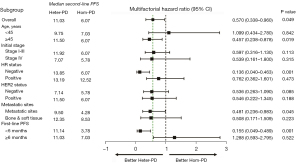
Among the 70 enrolled ABC patients, 19 retained partial medication (all were trastuzumab among HER2-positive patients). Based on the therapeutic response during the first-line treatment, 13 participants were classified as the Heter-PD group and the other 6 participants were Hom-PD group. The survival analysis indicated that the Heter-PD group experienced better second-line PFS than the Hom-PD group (13.18 vs. 3.61 months). Nevertheless, due to the limited number of participants, no statistical significance was verified (P=0.430).
Discussion
The RECIST 1.1 criteria is the common tool to monitor the treatment response in solid tumor. Oncologists maintain, adjust or discontinue current treatment depending on the therapeutic assessment by RECIST criteria. At baseline, the total tumor burden is categorized as measurable or non-measurable lesions, and the former is further categorized as target lesions and non-target lesions. All target lesions are measured according to the long-axis diameter (LAD) of solid lesions and short-axis diameter (SAD) of lymph nodes. The status of PD is defined as unequivocal progression of target lesions and/or existing non-target lesions and/or occurrence of new lesions. However, distinct treatment response might occur in various metastatic lesions due to the tumor heterogeneity.
Genetic instability is displayed by BC cells (deletion, amplification, point-mutations, and so on), leading to the high variability of cancer genomes and promoting genetic heterogeneity and different treatment response. Tumor heterogeneity is perhaps the primary cause of drug resistance and plays a significant role in treatment failure (9), which can be further classified as inter- and intra-tumor heterogeneity. The former represents the interindividual heterogeneity of tumors or differences between distinct lesions within the same patient, while the latter means cellular differences among individual lesions (10). Previous studies have indicated that tumor heterogeneity is produced by the interplay between the genome and epigenome/transcriptome (11,12).
The stochastic model proposed by Peter Nowell proposes that tumors arise from a single mutated cell and acquire mutations eternally (13). Genomic alterations are obtained via multiple mutational processes, producing spatial and temporal genetic diversity (14). Each mutation might produce specific subpopulation of tumor cells, possessing different capacities for proliferation, invasion, and migration. According to basic principles of Darwinian evolution, cellular clones with advantages would survive and expand, while those with less favorable adaptations become extinct (10). Under ecosystem-selective pressure, cellular mutation could lead to convergent evolution or spatial segregation of clones in primary and metastatic sites (15). Based on this speculation, the therapeutic responses of different malignant lesions are probably diverse due to the tumor heterogeneity.
It is considered that selective therapeutic pressure could facilitate the emergence of new resistance mutations and change of tumor phenotype finally (16). In the present study, we retrospectively analyzed the treatment response of ABC patients after first-line treatment. Based on the clinical implication and the diameter alternation of each malignant lesion, we proposed 3 classification models to further classify ABC patients with different prognoses during second-line treatment. The results indicated that ABC patients experiencing PD of target and non-target lesions regardless of new lesions occurrence (Hom-PD group) had shorter second-line PFS than the Heter-PD group (11.04 vs. 6.07 months).
The heterogeneous nature of BC increases drug resistance of the tumor (17). Therapeutic regimens can target cancer cells, and the genetic alterations of cancer cells influence the therapeutic response of antineoplastic drugs (18). Furthermore, the tumor microenvironment is different among various metastatic lesions, and the interaction between different subclones of cancer cells and stromal cells complicate the diversity of drug resistance (19). Inter-tumor heterogeneity means the differences between various tumor lesions of the same origin. Due to the existence of spatial heterogeneity, it is essential to monitor the evolution of malignant burden and modify antineoplastic treatment. These heterogeneous tumors manifest unique biological behaviors and molecular markers, causing variable drug resistance and clinical outcomes (20). Previous studies have indicated that genetic mutations, epigenetic modifications, and/or tumor microenvironment are the primary causes of intratumor heterogeneity (21-23).
For patients with heterogeneous PD, even the overall evaluation of the therapeutic response is disease progression, some tumor lesions are still under acceptable control with existing treatment, while other lesions manifest drug resistance. Conversely, homogeneous PD indicates the total resistance of medication among all lesions. The possible mechanism may be due to the potential tumor mutation burden or key genetic locus mutation, causing the improvement of tumor cell proliferation and drug resistant capacity. These 2 distinct disease progression modes may indicate a variety of mutation burden and genetic modification ability of cancer cells, being equal to the difficulty degree of anti-tumor treatment. However, further analyses, such next generation sequencing, are required to verify these hypotheses.
Clinically, visceral metastases are always correlated with higher tumor burden, reduced therapeutic response, and shorter survival (24). Tumor burden is a vital factor associated with the curability (25). In tumors that are highly sensitive to treatment, combining multiple drugs could permit sequential decreases in tumor burden until complete eradication (26). The Goldie–Coldman hypothesis incorporates tumor size and emergence of resistance (27). In our study, even the occurrence of heterogeneous disease progression was not associated with metastatic sites; Heter-PD patients experienced better second-line PFS than the Hom-PD patients (9.50 vs. 4.28 months, P=0.045) among visceral metastatic BC patients in the subgroup survival analysis. However, in the bone and soft tissue metastases subgroup, no difference in PFS was observed between Heter-PD group and Hom-PD group (P=0.223).
Despite the breakthrough of antineoplastic medication, no effective and specific treatment for heterogeneous tumors has yet been applied (7). The rapid development of inter-tumor heterogeneity causes difficulty in clinical tumor treatment (28,29). It is vital to measure the tumor heterogeneity in clinical practice. The comparison of multiple, spatially separated breast tumor lesions, integrating genetic, epigenetic, transcriptomic, and proteomic analyses, could provide more information of tumor heterogeneity and refine patient treatment (30,31). Currently, next-generation sequencing (NGS) of tumor samples at diagnosis or at recurrence is frequently-used tool for evaluation of tumor heterogeneity. However, it cannot accurately evaluate tumor heterogeneity. Several strategies may be feasible to solve the problem of tumor heterogeneity. The first strategy is to target common pathway among different cancer cell clones (28). The second strategy is to inhibit the transition of cellular heterogeneity by interfering the cell shifting, such as inhibiting c-Met, the TGF-beta gene (32,33).
Our study has some limitations. Firstly, due to the single-institution retrospective characteristic, the overall sample size was relatively small, which may have influenced the accuracy and reliability of the statistical analyses. Secondly, inherent selection bias existed due to the retrospective design of the present study. Thirdly, salvage treatment regimens were not unified, which may influence the accuracy of relevant statistical analyses.
Conclusions
For ABC patients, the mode of disease progression after first-line treatment is an independent factor influencing the therapeutic response of sequential treatment. Patients with Heter-PD experienced better PFS than the Hom-PD subgroup, which might help clinicians to further classify specific patients and make more informed treatment decisions.
Acknowledgments
Funding: This study was supported by Beijing Medical Award Foundation (No. YXJL-2020-0941-0755).
Footnote
Reporting Checklist: The authors have completed the STROBE reporting checklist. Available at https://atm.amegroups.com/article/view/10.21037/atm-22-1618/rc
Data Sharing Statement: Available at https://atm.amegroups.com/article/view/10.21037/atm-22-1618/dss
Conflicts of Interest: All authors have completed the ICMJE uniform disclosure form (available at https://atm.amegroups.com/article/view/10.21037/atm-22-1618/coif). The authors have no conflicts of interest to declare.
Ethical Statement: The authors are accountable for all aspects of the work in ensuring that questions related to the accuracy or integrity of any part of the work are appropriately investigated and resolved. The study was conducted in accordance with the Declaration of Helsinki (as revised in 2013). The study was approved by institutional ethics board of Guangdong Provincial Hospital of Chinese Medicine (No. ZE2020-349-01) and informed consent was taken from all the patients before inclusion.
Open Access Statement: This is an Open Access article distributed in accordance with the Creative Commons Attribution-NonCommercial-NoDerivs 4.0 International License (CC BY-NC-ND 4.0), which permits the non-commercial replication and distribution of the article with the strict proviso that no changes or edits are made and the original work is properly cited (including links to both the formal publication through the relevant DOI and the license). See: https://creativecommons.org/licenses/by-nc-nd/4.0/.
References
- Sung H, Ferlay J, Siegel RL, et al. Global Cancer Statistics 2020: GLOBOCAN Estimates of Incidence and Mortality Worldwide for 36 Cancers in 185 Countries. CA Cancer J Clin 2021;71:209-49. [Crossref] [PubMed]
- Siegel RL, Miller KD, Fuchs HE, et al. Cancer Statistics, 2021. CA Cancer J Clin 2021;71:7-33. [Crossref] [PubMed]
- Waks AG, Winer EP. Breast Cancer Treatment: A Review. JAMA 2019;321:288-300. [Crossref] [PubMed]
- Loibl S, Poortmans P, Morrow M, et al. Breast cancer. Lancet 2021;397:1750-69. [Crossref] [PubMed]
- Jamal-Hanjani M, Quezada SA, Larkin J, et al. Translational implications of tumor heterogeneity. Clin Cancer Res 2015;21:1258-66. [Crossref] [PubMed]
- Vogelstein B, Papadopoulos N, Velculescu VE, et al. Cancer genome landscapes. Science 2013;339:1546-58. [Crossref] [PubMed]
- Pasha N, Turner NC. Understanding and overcoming tumor heterogeneity in metastatic breast cancer treatment. Nat Cancer 2021;2:680-92. [Crossref] [PubMed]
- Therasse P, Arbuck SG, Eisenhauer EA, et al. New guidelines to evaluate the response to treatment in solid tumors. European Organization for Research and Treatment of Cancer, National Cancer Institute of the United States, National Cancer Institute of Canada. J Natl Cancer Inst 2000;92:205-16. [Crossref] [PubMed]
- Pe'er D, Ogawa S, Elhanani O, et al. Tumor heterogeneity. Cancer Cell 2021;39:1015-7. [Crossref] [PubMed]
- Turnquist C, Watson RA, Protheroe A, et al. Tumor heterogeneity: does it matter? Expert Rev Anticancer Ther 2019;19:857-67. [Crossref] [PubMed]
- Hayford CE, Tyson DR, Robbins CJ 3rd, et al. An in vitro model of tumor heterogeneity resolves genetic, epigenetic, and stochastic sources of cell state variability. PLoS Biol 2021;19:e3000797. [Crossref] [PubMed]
- Brady L, Kriner M, Coleman I, et al. Inter- and intra-tumor heterogeneity of metastatic prostate cancer determined by digital spatial gene expression profiling. Nat Commun 2021;12:1426. [Crossref] [PubMed]
- Nowell PC. The clonal evolution of tumor cell populations. Science 1976;194:23-8. [Crossref] [PubMed]
- Alexandrov LB, Nik-Zainal S, Wedge DC, et al. Signatures of mutational processes in human cancer. Nature 2013;500:415-21. [Crossref] [PubMed]
- Greaves M. Evolutionary determinants of cancer. Cancer Discov 2015;5:806-20. [Crossref] [PubMed]
- Crucitta S, Cucchiara F, Mathijssen R, et al. Treatment-driven tumour heterogeneity and drug resistance: Lessons from solid tumours. Cancer Treat Rev 2022;104:102340. [Crossref] [PubMed]
- Koren S, Bentires-Alj M. Breast Tumor Heterogeneity: Source of Fitness, Hurdle for Therapy. Mol Cell 2015;60:537-46. [Crossref] [PubMed]
- Valastyan S, Weinberg RA. Tumor metastasis: molecular insights and evolving paradigms. Cell 2011;147:275-92. [Crossref] [PubMed]
- Neophytou CM, Panagi M, Stylianopoulos T, et al. The Role of Tumor Microenvironment in Cancer Metastasis: Molecular Mechanisms and Therapeutic Opportunities. Cancers (Basel) 2021;13:2053. [Crossref] [PubMed]
- Mahdipour-Shirayeh A, Kaveh K, Kohandel M, et al. Phenotypic heterogeneity in modeling cancer evolution. PLoS One 2017;12:e0187000. [Crossref] [PubMed]
- Tlsty TD, Coussens LM. Tumor stroma and regulation of cancer development. Annu Rev Pathol 2006;1:119-50. [Crossref] [PubMed]
- Marusyk A, Janiszewska M, Polyak K. Intratumor Heterogeneity: The Rosetta Stone of Therapy Resistance. Cancer Cell 2020;37:471-84. [Crossref] [PubMed]
- Gerashchenko TS, Denisov EV, Litviakov NV, et al. Intratumor heterogeneity: nature and biological significance. Biochemistry (Mosc) 2013;78:1201-15. [Crossref] [PubMed]
- Robertson JFR, Di Leo A, Johnston S, et al. Meta-analyses of visceral versus non-visceral metastatic hormone receptor-positive breast cancer treated by endocrine monotherapies. NPJ Breast Cancer 2021;7:11. [Crossref] [PubMed]
- Glickman MS, Sawyers CL. Converting cancer therapies into cures: lessons from infectious diseases. Cell 2012;148:1089-98. [Crossref] [PubMed]
- Baliu-Piqué M, Pandiella A, Ocana A. Breast Cancer Heterogeneity and Response to Novel Therapeutics. Cancers (Basel) 2020;12:3271. [Crossref] [PubMed]
- Goldie JH, Coldman AJ. A mathematic model for relating the drug sensitivity of tumors to their spontaneous mutation rate. Cancer Treat Rep 1979;63:1727-33. [PubMed]
- Uhlen M, Zhang C, Lee S, et al. A pathology atlas of the human cancer transcriptome. Science 2017;357:eaan2507. [Crossref] [PubMed]
- Zhu L, Jiang M, Wang H, et al. A narrative review of tumor heterogeneity and challenges to tumor drug therapy. Ann Transl Med 2021;9:1351. [Crossref] [PubMed]
- Bedard PL, Hansen AR, Ratain MJ, et al. Tumour heterogeneity in the clinic. Nature 2013;501:355-64. [Crossref] [PubMed]
- Alizadeh AA, Aranda V, Bardelli A, et al. Toward understanding and exploiting tumor heterogeneity. Nat Med 2015;21:846-53. [Crossref] [PubMed]
- Bhola NE, Balko JM, Dugger TC, et al. TGF-β inhibition enhances chemotherapy action against triple-negative breast cancer. J Clin Invest 2013;123:1348-58. [Crossref] [PubMed]
- Meric-Bernstam F, Brusco L, Shaw K, et al. Feasibility of Large-Scale Genomic Testing to Facilitate Enrollment Onto Genomically Matched Clinical Trials. J Clin Oncol 2015;33:2753-62. [Crossref] [PubMed]