User acceptance of wearable intelligent medical devices through a modified unified theory of acceptance and use of technology
Introduction
With the spread of the concept of precision medicine and the urgent need for people to know their physical conditions in real-time, interest in intelligent medical products based on artificial intelligence is increasing (1,2). Recent technological advancements have facilitated individuals to monitor their fitness and health anytime, anywhere, on specific devices such as wearable intelligent medical devices (WIMDs) (3). These intelligent devices can provide a constant stream of healthcare data to help people pursue a healthier lifestyle by actively recording physiological parameters, tracking metabolic status, and sometimes even assisting in disease diagnosis and treatment (4). However, most commercially available WIMDs that have been qualified for use are usually simple and can only monitor vital signs (5). In addition, due to some other problems, such as difficulties in achieving user-friendly solutions and privacy disclosure, WIMDs now have significant limitations in medical practice. Therefore, studying the understanding and current use of these devices is essential to improve these devices and realize more medical services among people.
Most research regarding WIMDs has focused on establishing accuracy and reliability (6-8). Only a few studies have focused on WIMDs’ acceptance and the factors influencing behavioral intention to use them, which may improve the overall quality of the WIMDs industry and further drive the future mobile health market (8,9). As a result, this research aimed to increase the understanding of WIMDs by investigating the factors influencing the behavioral intention of WIMDs and how those factors affect their use.
The unified theory of acceptance and use of technology (UTAUT) and the technology acceptance model (TAM) have been widely used to explain the intention to use in various fields, including intelligent healthcare systems (10-12). The theory of perceived risk (TPR) pertains primarily to searching for and choosing information about products or services and depends on the subjective uncertainty of outcomes, which can also be utilized to analyze intelligent healthcare systems (13). At present, there is no research on the UTAUT model or TAM model integrating TPR to investigate the factors influencing the acceptance of WIMDs. Thus, we present a research framework by establishing a modified model based on UTAUT, which integrates TPR and TAM, to increase the current understanding of WIMDs. We present the following article in accordance with the STROBE reporting checklist (available at https://atm.amegroups.com/article/view/10.21037/atm-21-5510/rc).
Methods
Literature review
This research aimed to investigate people’s understanding of intelligent medical products, especially WIMDs, and investigate the antecedents of behavioral intention and use behavior. Therefore, a literature review was conducted to introduce WIMDs’ development status and existing problems, as well as the basis for establishing a modified model based on the UTAUT.
WIMDs
Intelligent medical products are based on artificial intelligence and machine learning, which can be applied in clinical practice to improve the existing medical model (14). WIMDs are intelligent medical products that should be worn directly on the body to monitor health status and diagnose and treat diseases (4). There are five main features of WIMDs (15): (I) wireless mobility; (II) interactivity and intelligence; (III) sustainability and durability; (IV) simple operation and miniaturization; and (V) wearability and portability. WIMDs can promote the development of precision medicine and facilitate the emergence of connected healthcare (16,17).
In clinical practice, intelligent medical product applications involve at least three subjects: social groups, doctors, and manufacturers. To realize the purpose of this research, our research subject was social groups. With the gradual aging of society and the extension of average life expectancy, the proportion of the population experiencing chronic diseases is expected to increase, which will require younger generations and society to expend more energy and financial resources to care for the elderly. Furthermore, a fast-paced lifestyle and work responsibilities negatively impact work-life balance for an increasing number of young and middle-aged people, resulting in some physical and psychological problems. WIMDs can help alleviate these problems by helping the elderly achieve a high-quality, independent life and helping young people maintain as regular a lifestyle as possible despite increased responsibilities. In this context, the market demand for WIMDs is increasing.
A series of studies have confirmed the feasibility and accuracy of wearable devices (18-22). However, little research has focused on acceptance of WIMDs and the factors influencing behavioral intention and use behavior. For example, Tsai and Lin (23) found that perceived usefulness indirectly affected behavioral intention through attitude in older adults using an extended TAM; however, their research did not recruit younger adults. In addition, although some studies have used UTAUT to build comprehensive models (including health awareness and TPR) to investigate the factors affecting the intention to use WIMDs, the sample sizes of these studies were small (<500) (24,25). Therefore, in light of these studies, we expanded the sample size and integrated TPR and TAM to construct a comprehensive modified UTAUT model to research the acceptance of WIMDs and the factors influencing behavioral intention.
Theories of TAM
The theory of technology acceptance is an analysis model of the research population’s acceptance of new things and their influencing factors, given the development of science and technology and the continuous emergence of innovative products. The classic theories include TAM, the theory of planned behavior, and UTAUT (26). These theories have been widely used to explain intention to use in intelligent healthcare systems, including the acceptance of wearable devices and intelligent health monitoring systems (10,27-29). However, UTAUT was developed by redefining representative technology acceptance theories without considering risk factors.
TPR
Perceived risk is the construction of a perceived situation that can be defined in various ways (26). TPR is mainly applied to the process of consumers’ purchase and use of products, including financial, performance, physical, psychological, social, and convenience risks (13). However, its application in intelligent medical devices has rarely been studied. As is well known, all medical practices, including WIMDs, have risks, including health, financial, and privacy risks (27-29). Therefore, TPR can compensate for the deficit of UTAUT. Combined models of UTAUT integrating TPR and TAM provide a better explanation for the acceptance of the WIMDs than that of the TAM or UTAUT model alone.
Research model and hypotheses
Based on WIMDs’ features, we propose a research model that identifies several factors influencing their acceptance, integrating the theoretical background of the UTAUT model, TPR, and TAM (Figure 1).
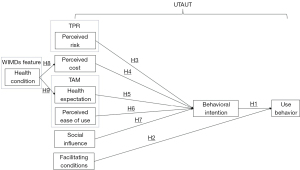
The basic assumption is that WIMDs’ acceptance is reflected in the behavior of use, which is jointly determined by behavioral intention and facilitating conditions. Behavioral intention is influenced by five motivational factors: perceived risk, perceived cost, health expectation, perceived ease of use, and social influence. On the one hand, different people have different health conditions, which is a feature of WIMDs. On the other hand, people with various health conditions have different health expectations and perceived costs related to their health conditions.
Modified UTAUT model
To test the dependent variable of use behavior, the modified UTAUT model was applied to test the relationships between behavioral intention and facilitating conditions. Furthermore, we separately tested the dependent variable of behavioral intention by removing the facilitating conditions.
Behavioral intention
According to Venkatesh and Davis, behavioral intention refers to “the degree to which a person has formulated conscious plans to perform or not perform some specified future behavior” (30). Further research has indicated that intention to use is the main indicator of an information system (31). The intention to use WIMDs is also a form of information system adoption. Thus, we propose the following research hypothesis:
- H1: behavioral intention toward wearable fitness technology will be positively related to WIMD use.
Facilitating conditions
Facilitating conditions refer to the degree to which an individual believes that a technical infrastructure exists to support technology use (32). In promoting and using WIMDs, real-time data transmission requires network devices’ support, and a timely feedback platform is needed when the devices fail; these are necessary technical infrastructure (7). With the technological advancement of WIMDs and the expansion of demand for their functions, more advanced information transmission and medical chip technology have recently been introduced in the WIMD adoption literature, representing a significant development direction of future technology infrastructure (4). Thus, we propose the following research hypothesis:
- H2: facilitating conditions have a positive effect on WIMD use.
Perceived risk
Online medical advice based on WIMDs is riskier and more uncertain than traditional medicine. Two important reasons why patients do not accept online medical advice are the security of online visits and personal information privacy concerns (8,33). Online patients cannot decipher if the doctor is real and can provide practical advice. Patients also feel insecure when registering personal information and paying fees, such as transmitting identification and credit card numbers via the internet (33). All of the above findings indicate that perceived risk negatively influences behavioral intention. Thus, perceived risk is a measure that determines intention prior to use. Accordingly, we propose the following research hypothesis:
- H3: perceived risk has a negative effect on the intention to use WIMDs.
Perceived cost
Perceived cost in this research refers to the additional payout that is believed necessary prior to using WIMDS, including the perceived time and perceived fee (7,26). First, because WIMDs transmit information in real-time, people pay more attention to what is happening to their bodies and spend more time communicating with healthcare professionals (34,35). In addition, it takes time to learn how to use WIMDS. Second, WIMDs require both a wearable product and a subscription to a related service, which results in ongoing costs. Although studies have shown that health plans for health promotion, early detection, and prevention reduce costs in the long term, costs can negatively impact behavioral intention before use. Thus, we propose the following research hypothesis:
- H4: perceived cost has a negative effect on the intention to use WIMDs.
Health expectation
Health expectation is similar to health consciousness but is more subjective. It includes the degree to which health concerns are integrated into people’s daily activities and the extent to which they control their health (24,25). People with a higher health expectation care more about their health and are thus more motivated to improve or maintain their health. Thus, we propose the following research hypothesis:
- H5: health expectation has a positive effect on the intention to use WIMDs.
Perceived ease of use
Perceived ease of use is defined as how a person believes that using technology will take minimal effort (36). Improvements in perceived ease of use may enhance productivity, performance, and effectiveness (i.e., usefulness) (13). Prior research has provided evidence of the significant effect of perceived ease of use on behavioral intention, either directly or indirectly, on perceived usefulness (13). WIMDs require users to learn how to use the internet and WMIDs’ operating system, which is generally very difficult for the elderly and requires assistance from family and friends (7). Therefore, in this research, we speculate that wearable devices’ ease of use severely impacts intention to use them, especially in the elderly population. Thus, we propose the following research hypothesis:
- H6: perceived ease of use has a positive effect on the intention to use WIMDs.
Social influence
Social influence refers to how others’ perceptions influence a user’s decision-making (24,25). “Others” include family members, close friends, influential people such as employers or peers, professionals such as physicians, and technical specialists (37). Taylor and Todd (38) indicated that peer influence from friends and classmates and superior influence from professors indirectly influenced behavioral intention through the mediator of subjective norms. Given that WIMD use is likely affected by the influence of superiors or other influential people, we propose the following research hypothesis:
- H7: social influence has a positive effect on the intention to use WIMDs.
Feature of WIMDs
Because health conditions are a prominent feature of people who use WIMDs, this research is interested in WIMDs’ effects on the two primary constructs: perceived cost and health expectation.
People with various health conditions pay more attention to healthy living differently than healthy people do. Research shows that those with a more substantial subjective health complaint are more concerned about modern health (39). People with high-risk diseases, such as high blood pressure and diabetes, focus more on their health conditions (40). Another study reported that patients who had undergone coronary artery bypass surgery had higher depression and anxiety levels and may pay more attention to their health conditions (41). Concerns about health conditions will likely urge people to increase their investment in their health, and the need to maintain it through external measures will also be stronger. Thus, we propose the following hypotheses:
- H8: health conditions have a negative effect on the perceived cost of WIMDs;
- H9: health conditions have a negative effect on the health expectation of WIMDs.
Questionnaire development
As shown in Appendix 1, we used a questionnaire survey with four sections to test our theoretical model. The first section included demographic questions about the respondents. The second section assessed the state of understanding and adoption of intelligent medical products. The third section contained questions for various constructs presented in the research model, measured using multiple entries, as shown in Table S1. A 5-point Likert scale was used, with answer options ranging from strongly disagree (1) to strongly agree (5). The fourth section requested respondents’ subjective feelings and suggestions for the functionality of intelligent medical products. To validate the questionnaire’s effectiveness, a pilot study was conducted at Capital Medical University, for which five professors and five postgraduate students were recruited. The respondents were chosen because of their technical competencies and thorough understanding of medicine. Questionnaires collected from the pilot research helped improve its effectiveness with some specific adjustments.
Data collection
The target respondents of this study were the entire population. We sent online surveys to WeChat Moments via https://www.wjx.cn/. Data were collected from September to December 2020; overall, 2,192 valid surveys were returned.
Statistical analysis
H1–H7 were tested collectively using structural equation modeling implemented via partial least squares. These techniques allow the assessment of relationships among theoretical constructs in a structural model. The structural model estimates the path coefficients, which indicate the relationships between the dependent and independent variables. Before testing the research model, SPSS 25.0 (IBM Corporation) for Windows was used to determine descriptive statistics on demographic variables, including characteristics such as gender, age, and educational background. In the last step, t-tests were conducted to compare mean differences in the constructs, including perceived cost and health expectation between people with different health conditions, to test H8 and H9, and differences were considered statistically significant at two-sided P values <0.05.
Ethics
The study was conducted in accordance with the Declaration of Helsinki (as revised in 2013). It was approved by the Ethics Committee of Beijing TongRen Hospital, Capital Medical University (No. TRECKY2019-049), and all respondents provided informed consent online. Personal data were anonymized prior to analysis.
Results
Sample description
A total of 2,192 distributed questionnaires were selected for data analysis. As shown in Table 1, 62.0% of the respondents were men. Respondents’ median age was 26 (interquartile range, 22–31) years. Most respondents had less than a bachelor’s degree (93.1%). Regarding regional background, 78.1% lived in Eastern China, and 76.7% lived in urban areas. Most respondents’ monthly income was less than 20,000 yuan (67.0%). In terms of health conditions, 84.7% had no underlying disease, and 82.9% had never undergone surgery.
Table 1
Variable | Category | Frequency | Percent (%) |
---|---|---|---|
Gender | Male | 1,360 | 62.0 |
Female | 832 | 38.0 | |
Age | <20 years | 299 | 13.6 |
20–30 years | 1,222 | 55.7 | |
30–40 years | 405 | 18.5 | |
40–50 years | 118 | 5.4 | |
50–60 years | 71 | 3.2 | |
≥60 years | 77 | 3.5 | |
Education | Less than diploma’s | 833 | 38.0 |
Diploma’s | 566 | 25.8 | |
Bachelor’s | 641 | 28.3 | |
Master’s or above | 152 | 6.9 | |
Occupation | Professional | 467 | 21.3 |
Service personnel | 327 | 14.9 | |
Freelancer | 195 | 8.9 | |
Worker | 79 | 3.6 | |
Company or government staff | 262 | 12.0 | |
Student | 235 | 10.7 | |
Others | 627 | 28.6 | |
Location | Eastern China | 1,711 | 78.1 |
Mid-China | 331 | 15.1 | |
Western China | 150 | 6.8 | |
Region | Urban | 1,682 | 76.7 |
Suburban | 234 | 10.7 | |
Rural | 276 | 12.6 | |
Monthly income (yuan) | <10,000 | 943 | 43.0 |
10,000–20,000 | 525 | 24.0 | |
20,000–30,000 | 185 | 8.4 | |
30,000–40,000 | 97 | 4.4 | |
≥40,000 | 442 | 20.2 | |
Underlying diseases | Hypertension | 139 | 6.3 |
Diabetes | 55 | 2.5 | |
Coronary heart disease (CHD) | 43 | 2.0 | |
Stroke | 24 | 1.1 | |
Others | 17 | 0.8 | |
No underlying diseases | 1,856 | 84.7 | |
Surgery | Yes | 374 | 17.1 |
No | 1,818 | 82.9 |
As shown in Table 2, according to the analysis of respondents’ current use status of intelligent medical products, only 188 (8.6%) understood and used intelligent medical products, among which WIMDs were the most widely used (49.5%). Of the respondents who used intelligent medical products, 47.3% primarily acquired them on their initiative, and 27.7% acquired them based on friends’ recommendations. More than half of the users (51.6%) believed that the current intelligent medical products are relatively easy to use and that their functions can meet their current needs.
Table 2
Items | Type | Frequency | Percent (%) |
---|---|---|---|
Level of usage (N=2,192) | No understand, no use | 1,497 | 68.3 |
Understand, no use | 507 | 23.1 | |
Understand and use | 188 | 8.6 | |
Classification of intelligent medical products usage (n=188) | Intelligent monitoring equipment | 62 | 33.0 |
Wearable intelligent medical devices | 93 | 49.5 | |
Application of health management | 72 | 38.3 | |
The online medical service platform | 78 | 41.5 | |
Others | 25 | 13.3 | |
Ways to access intelligent medical devices (n=188) | Actively acquired | 89 | 47.3 |
Doctor recommend | 49 | 26.1 | |
Family recommend | 49 | 26.1 | |
Friend recommend | 52 | 27.7 | |
Enterprise promotion | 25 | 13.3 | |
Others | 33 | 17.6 | |
Degree of intelligent medical device usage (n=188) | Very difficult | 24 | 12.8 |
Difficult | 16 | 8.5 | |
General | 51 | 27.1 | |
Easy | 41 | 21.8 | |
Very easy | 56 | 29.8 | |
Effect of intelligent medical device (n=188) | Very satisfied | 53 | 28.2 |
Satisfied | 41 | 21.8 | |
General | 68 | 36.2 | |
Dissatisfied | 13 | 6.9 | |
Very dissatisfied | 13 | 6.9 |
Structural model for hypotheses testing
Statistical analysis indicated that the data followed normality characteristics. The skewness value for all variables was in the range of +1 to −1, indicating that the data distribution was normal. Moreover, all of the determinants’ kurtosis results were in the range of +2.58 to −2.58, indicating that the data distribution was normal.
Once data normality was confirmed, the model’s internal reliability, convergent validity, and discriminant validity were determined. To measure the data’s internal reliability, Cronbach’s α was evaluated against the standard threshold of 0.7, the criterion for acceptable internal consistency of data (42,43).
Convergent validity was calculated using average variance extracted (AVE), composite reliability (CR), and item loadings, such that a minimum of 0.50 of AVE exists for construct validity. As clearly depicted in Table 3, the calculated values of Cronbach’s α ranged from 0.942 to 0.973, and CR varied in the range of 0.943–0.967. As the calculated indices were all above the recommended thresholds, the strong internal reliability of the data was confirmed (44). The individual item loadings of the constructs ranged between 0.760 and 0.940, whereas AVE values ranged from 0.846 to 0.923, both exceeding the recommended levels. Hence, the required criterion for convergent validity was fulfilled.
Table 3
Constructs | Construct code | Items loading | Average variance extracted (AVE) | Composite reliability (CR) | Cronbach’s α |
---|---|---|---|---|---|
Use behavior (UB) | UB1 | 0.94 | 0.923 | 0.960 | 0.973 |
UB2 | 0.91 | ||||
Behavioral intention (BI) | BI1 | 0.82 | 0.839 | 0.940 | 0.965 |
BI2 | 0.85 | ||||
BI3 | 0.85 | ||||
Perceived risk (PR) | PR1 | 0.76 | 0.861 | 0.961 | 0.960 |
PR2 | 0.91 | ||||
PR3 | 0.93 | ||||
PR4 | 0.85 | ||||
Perceived cost (PC) | PC1 | 0.87 | 0.878 | 0.956 | 0.956 |
PC2 | 0.91 | ||||
PC3 | 0.86 | ||||
Health expectancy (HE) | HE1 | 0.88 | 0.908 | 0.967 | 0.967 |
HE2 | 0.93 | ||||
HE3 | 0.92 | ||||
Perceived ease of use (PEOU) | PEOU1 | 0.92 | 0.930 | 0.964 | 0.964 |
PEOU2 | 0.94 | ||||
Social influence (SI) | SI1 | 0.85 | 0.846 | 0.943 | 0.942 |
SI2 | 0.89 | ||||
SI3 | 0.80 | ||||
Facilitation conditions (FC) | FC1 | 0.89 | 0.881 | 0.957 | 0.957 |
FC2 | 0.88 | ||||
FC3 | 0.87 |
A cross-loading matrix along with the square root of AVE was determined to measure the discriminant validity of the data. For discriminant validity, the square root of AVE of a determinant must surpass the correlation it exhibits with other constructs (45). The results presented in Table 4 confirmed the data’s discriminant validity.
Table 4
Constructs | UB | BI | PR | PC | HE | PEOU | SI | FC |
---|---|---|---|---|---|---|---|---|
UB | 1 | |||||||
BI | 0.869 | 1 | ||||||
PR | 0.829 | 0.788 | 1 | |||||
PC | 0.894 | 0.880 | 0.839 | 1 | ||||
HE | 0.893 | 0.924 | 0.829 | 0.924 | 1 | |||
PEOU | 0.898 | 0.880 | 0.910 | 0.925 | 0.917 | 1 | ||
SI | 0.904 | 0.834 | 0.851 | 0.896 | 0.853 | 0.888 | 1 | |
FC | 0.940 | 0.875 | 0.849 | 0.903 | 0.907 | 0.915 | 0.919 | 1 |
UB, use behavior; BI, behavioral intention; PR, perceived risk; PC, perceived cost; HE, health expectancy; PEOU, perceived ease of use; SI, social influence; FC, facilitation conditions.
Figure 2 illustrates the resulting path coefficients of the proposed research model. A summary of the hypotheses testing results of the standardized path coefficients and path significance was provided in Table 5. As shown in Table 5, the positive influence of behavioral intention, facilitating conditions, health expectation, perceived ease of use, and social influence was supported by hypothesis testing. The negative influence of perceived risk was also validated by hypothesis testing. However, the proposed negative effect of perceived cost was rejected by hypothesis testing.
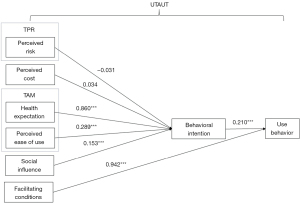
Table 5
The hypothesis | Path coefficient | P value | Support |
---|---|---|---|
H1: behavioral intention toward wearable fitness technology will be positively related to WIMDs use | 0.210 | <0.001 | Yes |
H2: facilitating conditions has a positive effect on WIMDs use | 0.942 | <0.001 | Yes |
H3: perceived risk has a negative effect on the intention to use WIMDs | −0.031 | >0.05 | Yes |
H4: perceived cost has a negative effect on the intention to use WIMDs | 0.034 | >0.05 | No |
H5: health expectation has a positive effect on the intention to use WIMDs | 0.860 | <0.001 | Yes |
H6: perceived ease of use has a positive effect on the intention to use WIMDs | 0.289 | <0.001 | Yes |
H7: social influence has a positive effect on the intention to use WIMDs | 0.153 | <0.001 | Yes |
WIMDs, wearable intelligent medical devices.
After removing the facilitating conditions, which were the most critical to use behavior, the above results were also confirmed in Figure 3.
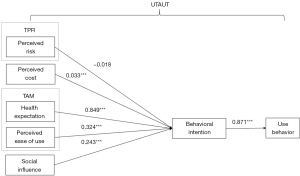
Differences between people with different health conditions
A t-test was conducted to compare the mean differences in the constructs, including perceived cost and health expectation, between people with different health conditions. As indicated in Table 6, people with underlying diseases had a lower health expectation and perceived cost than those without underlying diseases did (P<0.05). The health expectation and perceived cost of patients who had undergone surgery were higher than those who had not received surgery, but there was no statistically significant difference in perceived cost (P>0.05).
Table 6
Variable | Underlying disease, mean (SD) | Surgery, mean (SD) | |||||
---|---|---|---|---|---|---|---|
Yes | No | P value | Yes | No | P value | ||
Health expectation | 9.813 (3.505) | 10.307 (2.850) | <0.01 | 10.505 (3.012) | 10.125 (2.951) | <0.05 | |
Perceived cost | 9.634 (3.339) | 10.003 (2.810) | <0.05 | 10.187 (2.944) | 9.897 (2.889) | >0.05 |
Discussion
As shown in previous studies and our questionnaire (Appendix 1), intelligent medical devices include WIMDs, intelligent monitoring equipment, application of health management, etc.; WIMDs are the most widely used. Therefore, this research aimed to advance our understanding of WIMDs and investigate the factors influencing their behavioral intentions. Compared with prior work, this study’s major contribution is to empirically investigate the application of a modified UTAUT model in WIMDs, integrating TPR and TAM (7,46). Based on a literature review of the UTAUT model, TPR, TAM, and WIMDs, and a pilot study, we developed a comprehensive research framework and used structural equation modeling to assess relationships among theoretical constructs in the modified model. The results showed that there were significant relationships between the research variables. Facilitating conditions were the most influential factors on people’s use behavior, which has not been found in previous studies. Perceived risk was negatively related to behavioral intention, but perceived cost was positively related. Health expectation, perceived ease of use, and social influence positively affected behavioral intention, which in turn affected actual use behavior regarding WIMDs. Because the functions of WIMDs include monitoring fitness and health conditions, this research investigated people with different health conditions and found that people without underlying diseases had a higher health expectation and expectation for using WIMDs, which was not consistent with the previous literature (40).
WIMDs have been one of the fastest-growing products in intelligent medical devices over the last decade, and the global market for WIMDs is growing at a remarkable rate (47). Some studies have focused on people’s intention to use WIMDs and potential influencing factors (8,16,22,24,25). However, several issues still hinder the adoption and development of WIMDs. First, as noted in previous research, most of the 2,192 respondents were aged 20–30 years, as in previous studies, with a small percentage of respondents over 50 years of age (25). Among the people we followed up with, 68.3% did not know any information about WIMDs, which indicates that the social publicity of WIMDs is insufficient and that further promotion by manufacturers, medical institutions, and medical personnel is needed. Second, approximately 40% of current WIMDs users reported that it was not easy to use WIMDs. Combined with the results elicited from the fourth section of the questionnaire on subjective feelings and suggestions for WIMDs, we found that the main complaints with ease of use included the following: (I) the data transmission of WIMDs was not accurate enough; (II) the operation interface needed to be further humanized, especially for the elderly; and (III) the function was not intelligent enough, meaning that WIMDs alone cannot facilitate closed-loop management of medical behavior. With the rapid growth of the aging population in China and worldwide, using intelligent medical products, including WIMDs, as part of the existing workflow, such as medical behavior and community management, and to form an interactive mode of sharing information may be a valuable approach for people to adopt intelligent medical products (4,7).
Facilitating conditions are the most critical drivers for WIMDs in a developing country. Communities where users of WIMDs live need to strengthen their network support, and manufacturers need to follow up their after-sales service to match users’ preferences (7). In addition, this study verifies that health expectation is the most significant positive predictor of users’ intention to adopt WIMDs, which supports the research by Gao and Yang Meier (7,46). Developing, analyzing, and employing medical and health big data to expand WIMDs in other health fields, such as telemedicine, preventive medicine, and epidemiology, are the goals of WIMDs’ development (17). Further, perceived ease of use was an essential driver for acceptance of WIMDs, which is consistent with previous studies (48,49). Therefore, this factor is also significant in China’s other healthcare contexts. The more people view intelligent medical products such as WIMDs as easy-to-use technology, the more motivated they will be to utilize them. Consequently, WIMDs must be designed according to users’ acceptance level of technology. In this way, people will not have to spend a tremendous amount of time and effort learning to utilize these products.
The research results for social influence indicated its significance for large-scale implementation of WIMDs, confirming previous studies’ findings (24,25,36). People will be more likely to use WIMDs if their family members, acquaintances, and private doctors view these products as effective for improving health. For this purpose, prominent community members and health workers must be engaged in research discourse regarding WIMDs, leading to more positive results.
Although previous studies have suggested that perceived risk is a significant barrier to acceptance of WIMDs (46,50), it was not validated as a significant negative predictor of behavioral intention. This may be related to the younger age of the respondents surveyed in this study. Young people may not have experienced severe health problems and may not have privacy concerns about disclosing their health information that deter them from using wearable devices, consistent with Yang Meier’s findings (24). In contrast to our predictions regarding the negative effects of perceived cost on the intention to use WIMDs, H4 is not supported by the survey data. One explanation for this unexpected finding is that WIMDs users are generally young people who do not think it takes extra time and experience to learn and use WIMDs. Since there are significant differences in perceived costs among people of different ages, manufacturers should design products tailored to different ages.
Our study is the first to show that people with underlying diseases had a lower health expectation and lower perceived cost, with further negative effects on use intention and behavior. However, this is likely because people with underlying diseases tend to have negative feelings about medical behavior because of chronic illness. Perhaps in a different research context, the explanatory power of each factor of UTAUT would vary (25).
This study has several limitations. First, more than 90.0% of the respondents were younger than 50 years, and their behavior may differ somewhat from the rest of the population, which may have biased the results. Future research should examine more older people to validate a more generalized model. Second, although the gender, regional background, and educational background of the respondents were included in this study, they were not analyzed in detail. Further research is expected to help generalize our findings and discussions to include different genders, regions, and educational backgrounds in which WIMDs are utilized.
Conclusions
Through the largest questionnaire so far, this study investigated the use of WIMDs in the Chinese population, and its findings contribute to the existing body of knowledge of WIMDs. The findings also highlight how the adoption process of WIMDs in developing countries can be improved. Based on the identified factors—including facilitating conditions, health expectation, perceived ease of use, social influence, and health conditions-countries, communities, medical institutions, and manufacturers can design better strategies and development plans to promote intelligent medical products.
Acknowledgments
We thank all volunteers for participation in the study. We thank the School of Public Health, Capital Medical University, China.
Funding: This study was supported by a project grant from National Key Research and Development Program of China: Artificial Intelligence Management and Service Model for Public Health and Medicine (2018YFC0116800).
Footnote
Reporting Checklist: The authors have completed the STROBE reporting checklist. Available at https://atm.amegroups.com/article/view/10.21037/atm-21-5510/rc
Data Sharing Statement: Available at https://atm.amegroups.com/article/view/10.21037/atm-21-5510/dss
Conflicts of Interest: All authors have completed the ICMJE uniform disclosure form (available at https://atm.amegroups.com/article/view/10.21037/atm-21-5510/coif). The authors have no conflicts of interest to declare.
Ethical Statement: The authors are accountable for all aspects of the work in ensuring that questions related to the accuracy or integrity of any part of the work are appropriately investigated and resolved. The study was conducted in accordance with the Declaration of Helsinki (as revised in 2013). The study was approved by the Ethics Committee of Beijing TongRen Hospital, Capital Medical University (No. TRECKY2019-049) and informed consent was taken from all individual participants.
Open Access Statement: This is an Open Access article distributed in accordance with the Creative Commons Attribution-NonCommercial-NoDerivs 4.0 International License (CC BY-NC-ND 4.0), which permits the non-commercial replication and distribution of the article with the strict proviso that no changes or edits are made and the original work is properly cited (including links to both the formal publication through the relevant DOI and the license). See: https://creativecommons.org/licenses/by-nc-nd/4.0/.
References
- O'Neill T, Miksad R, Miller D, et al. ISPOR, the FDA, and the Evolving Regulatory Science of Medical Device Products. Value Health 2019;22:754-61. [Crossref] [PubMed]
- Sohn K, Kwon O. Technology acceptance theories and factors influencing artificial Intelligence-based intelligent products. Telemat Inform 2020;47:101324. [Crossref]
- Lunney A, Cunningham NR, Eastin MS. Wearable fitness technology: A structural investigation into acceptance and perceived fitness outcomes. Comput Hum Behav 2016;65:114-20. [Crossref]
- Lu L, Zhang J, Xie Y, et al. Wearable Health Devices in Health Care: Narrative Systematic Review. JMIR Mhealth Uhealth 2020;8:e18907. [Crossref] [PubMed]
- Haghi M, Thurow K, Stoll R. Wearable Devices in Medical Internet of Things: Scientific Research and Commercially Available Devices. Healthc Inform Res 2017;23:4-15. [Crossref] [PubMed]
- Salim A, Lim S. Recent advances in noninvasive flexible and wearable wireless biosensors. Biosens Bioelectron 2019;141:111422. [Crossref] [PubMed]
- Lewy H. Wearable technologies - future challenges for implementation in healthcare services. Healthc Technol Lett 2015;2:2-5. [Crossref] [PubMed]
- Wen D, Zhang X, Lei J. Consumers' perceived attitudes to wearable devices in health monitoring in China: A survey study. Comput Methods Programs Biomed 2017;140:131-7. [Crossref] [PubMed]
- Mackintosh KA, Chappel SE, Salmon J, et al. Parental Perspectives of a Wearable Activity Tracker for Children Younger Than 13 Years: Acceptability and Usability Study. JMIR Mhealth Uhealth 2019;7:e13858. [Crossref] [PubMed]
- Fan W, Liu J, Zhu S, et al. Investigating the impacting factors for the healthcare professionals to adopt artificial intelligence-based medical diagnosis support system (AIMDSS). Ann Oper Res 2020;294:567-92. [Crossref]
- Chen Y, Le D, Yumak Z, et al. EHR: A sensing technology readiness model for lifestyle changes. Mobile Netw 2017;22:478-92. [Crossref]
- Hsieh PJ. Healthcare professionals’ use of health clouds: Integrating technology acceptance and status quo bias perspectives. Int J Med Inform 2015;84:512-23. [Crossref] [PubMed]
- Li YH, Huang JW. Applying Theory of Perceived Risk and Technology Acceptance Model in the Online Shopping Channel. World Acad Sci Eng Technol 2019;53:919-25.
- Muehlematter UJ, Daniore P, Vokinger KN. Approval of artificial intelligence and machine learning-based medical devices in the USA and Europe (2015-20): a comparative analysis. Lancet Digit Health 2021;3:e195-203. [Crossref] [PubMed]
- Qian RC, Long YT. Wearable Chemosensors: A Review of Recent Progress. ChemistryOpen 2018;7:118-30. [Crossref] [PubMed]
- Jeong IC, Bychkov D, Searson PC. Wearable Devices for Precision Medicine and Health State Monitoring. IEEE Trans Biomed Eng 2019;66:1242-58. [Crossref] [PubMed]
- Loncar-Turukalo T, Zdravevski E, Machado da Silva J, et al. Literature on Wearable Technology for Connected Health: Scoping Review of Research Trends, Advances, and Barriers. J Med Internet Res 2019;21:e14017. [Crossref] [PubMed]
- Baig MM, Afifi S. A Systematic Review of Wearable Sensors and IoT-Based Monitoring Applications for Older Adults - a Focus on Ageing Population and Independent Living. J Med Syst 2019;43:233. [Crossref] [PubMed]
- Sequeira L, Perrotta S, LaGrassa J, et al. Mobile and wearable technology for monitoring depressive symptoms in children and adolescents: A scoping review. J Affect Disord 2020;265:314-24. [Crossref] [PubMed]
- van der Heide I, Poureslami I, Mitic W, et al. Health literacy in chronic disease management: a matter of interaction. J Clin Epidemiol 2018;102:134-8. [Crossref] [PubMed]
- Godfrey A. Wearables for independent living in older adults: Gait and falls. Maturitas 2017;100:16-26. [Crossref] [PubMed]
- Wearable medical device improves survival for glioblastoma patients. Cancer 2017;123:3433. [Crossref] [PubMed]
- Tsai TH, Lin WY, Chang YS, et al. Technology anxiety and resistance to change behavioral study of a wearable cardiac warming system using an extended TAM for older adults. PLoS One 2020;15:e0227270. [Crossref] [PubMed]
- Yang Meier D, Barthelmess P, Sun W, et al. Wearable technology acceptance in health care based on national culture differences: cross-country analysis between chinese and swiss consumers. J Med Internet Res 2020;22:e18801. [Crossref] [PubMed]
- Chang CC. Exploring the Usage Intentions of Wearable Medical Devices: A Demonstration Study. Interact J Med Res 2020;9:e19776. [Crossref] [PubMed]
- Ye T, Xue J, He M, et al. Psychosocial Factors Affecting Artificial Intelligence Adoption in Health Care in China: Cross-Sectional Study. J Med Internet Res 2019;21:e14316. [Crossref] [PubMed]
- Chuah SHW, Rauschnabel PA, Krey N, et al. Wearable technologies: The role of usefulness and visibility in smartwatch adoption. Comput Hum Behav 2016;65:276-84. [Crossref]
- Basoglu N, Ok AE, Daim TU. What will It take to adopt smart glasses: A consumer choice based review? Technol Soc 2017;50:50-6. [Crossref]
- Tseng KC, Hsu CL, Chuang YH. Designing an intelligent health monitoring system and exploring user acceptance for the elderly. J Med Syst 2013;37:9967. [Crossref] [PubMed]
- Venkatesh V, Davis FD. A Theoretical Extension of the Technology Acceptance Model: Four Longitudinal Field Studies. Manage Science 2000;46:186-204. [Crossref]
- Venkatesh V, Morris MG, Davis GB, et al. User Acceptance of Information Technology: Toward a Unified View. MIS Quarterly 2003;27:425-78. [Crossref]
- Ajzen I. The theory of planned behavior. Organ Behav Hum 1991;50:179-211. [Crossref]
- Cilliers L. Wearable devices in healthcare: Privacy and information security issues. Health Inf Manag 2020;49:150-6. [PubMed]
- Liang J, Xian D, Liu X, et al. Usability Study of Mainstream Wearable Fitness Devices: Feature Analysis and System Usability Scale Evaluation. JMIR Mhealth Uhealth 2018;6:e11066. [Crossref] [PubMed]
- Iftikhar P, Kuijpers MV, Khayyat A, et al. Artificial Intelligence: A New Paradigm in Obstetrics and Gynecology Research and Clinical Practice. Cureus 2020;12:e7124. [Crossref] [PubMed]
- Kamal SA, Shafiq M, Kakria P. Investigating acceptance of telemedicine services through an extended technology acceptance model (TAM). Technol Soc 2019;60:101212. [Crossref]
- Vest BM, Kahn LS, Danzo A, et al. Diabetes self-management in a low-income population: impacts of social support and relationships with the health care system. Chronic Illn 2013;9:145-55. [Crossref] [PubMed]
- Taylor S, Todd PA. Understanding Information Technology Usage: A Test of Competing Models. Information Systems Research 1995;6:144-76. [Crossref]
- Indregard AM, Ihlebæk CM, Eriksen HR. Modern health worries, subjective health complaints, health care utilization, and sick leave in the Norwegian working population. Int J Behav Med 2013;20:371-7. [Crossref] [PubMed]
- Liu F, Li Y, Ju X. Exploring Patients’ Consultation Behaviors in the Online Health Community: The Role of Disease Risk. Telemed J E Health 2019;25:213-20. [Crossref] [PubMed]
- Açıkel MET. Evaluation of Depression and Anxiety in Coronary Artery Bypass Surgery Patients: A Prospective Clinical Study. Braz J Cardiovasc Surg 2019;34:389-95. [Crossref] [PubMed]
- Hair JF. Multivariate data analysis (7th Edition). Upper Saddle River: Prentice Hall, 2009.
- Astrachan CB, Patel VK, Wanzenried G. A comparative study of CB-SEM and PLS-SEM for theory development in family firm research. J Fam Bus Strategy 2014;5:116-28. [Crossref]
- Henseler J, Ringle CM, Sarstedt M. A new criterion for assessing discriminant validity in variance-based structural equation modeling. J Acad Mark Sci 2015;43:115-35. [Crossref]
- Venkatesh V, Thong JYL, Xu X. Consumer Acceptance and Use of Information Technology: Extending the Unified Theory of Acceptance and Use of Technology. MIS Quarterly 2012;36:157-78. [Crossref]
- Gao Y, Li H, Luo Y. An empirical study of wearable technology acceptance in healthcare. Ind Manag Data Syst 2015;115:1704-23. [Crossref]
- Framingham M. IDC Reports Strong Growth in the Worldwide Wearables Market, Led by Holiday Shipments of Smartwatches, Wrist Bands, and Ear-Worn Devices. IDC, March 05, 2019.
- Rho MJ, Choi IY, Lee J. Predictive factors of telemedicine service acceptance and behavioral intention of physicians. Int J Med Inform 2014;83:559-71. [Crossref] [PubMed]
- Adenuga KI, Iahad NA, Miskon S. Towards reinforcing telemedicine adoption amongst clinicians in Nigeria. Int J Med Inform 2017;104:84-96. [Crossref] [PubMed]
- Lee MC. Factors influencing the adoption of internet banking: an integration of TAM and TPB with perceived risk and perceived benefit. Electron Commer Res Appl 2009;8:130-41. [Crossref]