Choroid automatic segmentation and thickness quantification on swept-source optical coherence tomography images of highly myopic patients
Introduction
The prevalence of high myopia (HM) continues to increase (1-3). It is estimated that there will be 938 million people living with HM by 2050, accounting for 9.8% of the world’s population (1). Pathological myopia, which is a major cause of visual impairment and blindness worldwide, often occurs in eyes with HM (4-6).
The choroid is a vascular structure located between the retina and sclera, which supplies nutrients to the outer retina and serves critical physiological functions such as regulation of intraocular pressure and light absorption (7,8). Several studies on non-HM patients have shown that age can lead to thinning of the choroid (9-12); however, choroidal thinning is also related to many diseases, including myopia and age-related macular degeneration (AMD) (13-16).
The choroidal thickness (ChT) decreases with increasing levels of myopia (8,17). The ChT in HM patients can be about half to a quarter thinner when compared with that of patients with normal vision and correlates closely with the refractive error, axial length (AL), and posterior staphyloma height (8,18-20). The accurate quantification of the ChT is essential in the study of ocular diseases associated with the choroid. However, the manual measurement of ChT is time-consuming, limiting its use as a potential indicator for monitoring disease progression in HM patients.
The use of artificial intelligence (AI), especially deep learning algorithms, in ophthalmology has increased considerably in the past few years for the diagnosis, classification, prediction, and prognosis of ocular diseases (21-23). Optical coherence tomography (OCT) is often used to acquire high-resolution ocular images as part of a clinical examination and has an important role in the development of AI algorithms. As evidenced by previous studies, the use of AI models based on OCT images results in highly accurate detection of various pathological conditions, including retinal lesions, AMD, macular edema, retinoschisis, retinal detachment, and macular hole (24-26).
Although previous studies have applied traditional algorithms to segment the choroid, they have had limitations such as being applicable only to segment the normal choroid or requiring high-quality images (27-29). Regarding the thin choroid in HM, deformation of the retina caused by elongation of the AL, posterior staphyloma, or the possible existence of pathological atrophy regions makes the detection of the retinal and choroidal structure inaccurate and difficult.
Therefore, in this study, we aimed to develop a novel deep learning algorithm based on a group-wise context selection network (GCS-Net) to automatically segment the choroid region and quantify the ChT on swept-source optical coherence tomography (SS-OCT) images of HM patients. The accuracy of the algorithm was validated against a manually segmented choroid as ground truth. We present the following article in accordance with the TRIPOD reporting checklist (available at https://atm.amegroups.com/article/view/10.21037/atm-21-6736/rc).
Methods
Participants
We conducted a training, validation, and external testing study on an AI model using SS-OCT images. The data source of this study was a section of the Shanghai Eye Study for Older People, which was a population-based, cross-sectional study including individuals aged 50 years and older in Shanghai, China, between 2016 and 2018. Patients were excluded from the study if they had a history of eye surgery (except cataract surgery), corneal opacity, severe cataract, glaucoma, systemic diseases with ocular involvement, and fundus lesions unrelated to myopia (e.g., AMD, diabetic retinopathy, and optic neuropathy).
The study was conducted in accordance with the Declaration of Helsinki (as revised in 2013). The study was approved by the Ethics Committee of the Shanghai General Hospital, Shanghai Jiao Tong University School of Medicine (No. 2015KY153), and informed consent was provided by all individual participants.
Ophthalmic examinations
All patients enrolled in this study underwent comprehensive clinical interviews and ophthalmic examinations, including assessments of the refractive error using an autorefractor instrument (model KR-8900; Topcon, Tokyo, Japan), measurement of intraocular pressure (Full Auto Tonometer TX-F; Topcon), slit-lamp biomicroscopy, color fundus examination, and AL measurement using IOL Master (Carl Zeiss Meditec, Jena, Germany). Subjective refraction was performed by an experienced optometrist for all participants. The spherical equivalent (SE) was obtained as follows: SE = sphere power + (cylinder power/2). Eyes with an ocular AL greater or equal to 26 mm were defined as HM (13). The Topcon Atlantis DRI-1 SS-OCT scanner was used to acquire 12-line radial B-scans centered on the fovea. The SS-OCT parameters were as follows: wavelength of the light source =1,050 nm, scan rate =100,000 A-scans per second, depth resolution =8 µm, and lateral resolution =10 µm. The image size of each B-scan was 1,024 (width of B-scans) × 992 (depth of B-scans) pixels, which corresponds to a total area of 9×2.6 mm2.
The ChT, defined as the vertical distance between Bruch’s membrane and the choroidal-scleral interface, was measured using a specialized grid focused on the macula as described in the Early Treatment of Diabetic Retinopathy Study (ETDRS). The diameters for the foveal circle, parafoveal circle, and perifoveal circle of the ETDRS grid were set at 1, 3, and 6 mm, respectively. The parafoveal and perifoveal regions were further divided into temporal, superior, nasal, and inferior quadrants. The average ChT was measured at 9 different regions, including the inner and outer parts of each of the 4 quadrants and the center of the choroid.
Datasets
The development dataset included a total of 720 SS-OCT B-scans obtained from 60 eyes, comprising 40 HM eyes and 20 non-HM eyes. Each B-scan was considered an independent image and exported as a jpeg to use in algorithm development. All images were labeled by 1 well-trained grader (ML) and supervised by a retina specialist (YF), who checked the manual segmentation at least once. Subsequently, these images were subdivided into a training dataset (80% of the images) for model development and a validation dataset (20% of the images) for validating the model. To facilitate calculation of the AI model, we adjusted the OCT image size to 512×256 pixels. We then applied online augmentation of left-right flip, which simulated the symmetry of right and left eyes, to increase the size of the training set and improve the generalization ability and robustness of the model. The AI system was verified using an independent real test clinical dataset consisting of 3,192 images obtained from 266 HM eyes according to the same criteria listed above. None of the latter images had been used previously in the training and validation datasets.
GCS-Net
A GCS-Net can effectively select multiscale context information to achieve accurate segmentation of choroid regions with different thicknesses (30). Extraction of multiscale features corresponding to different perception fields also gives the model strong ability in distinguishing choroid and other retinal structures. The novelty in designing GCS-Net lies in 2 modules, i.e., the group-wise channel dilation (GCD) module, the group-wise spatial dilation (GSD) module, and a boundary optimization sub-network (BON). Both GCD and GSD adopt the self-attention mechanism, where feature maps are recalibrated with adaptive weights. In this way, features with higher discriminating ability are emphasized. The GCD module can select multiscale information under the guidance of channel information, while the GSD module can use spatial information to guide the fusion of multiscale context information. The BON uses deep supervision to solve the problem of choroidal boundary blur.
In the GCD and GSD modules, the input feature maps are divided into groups and each group goes through dilated convolutions with different rates, thus obtaining multiscale features. The GCD module downsamples the feature matrix to obtain the channel weights and multiplies each convoluted group with the corresponding weight. Finally, the weighted multiscale features are added to the input features as the output. The difference between the GSD module and the GCD module is that the weights obtained by the GSD module are spatial weights.
The GCS-Net is a U-shaped network with 4-layer encoder and decoder. The GCD module is embedded between the encoder and decoder, where the output of each layer of the encoder is connected to the decoder through the GCD module. The GSD module is added after each deconvolution layer of the decoder. Except for the bottom layer, the sum of the outputs of the GCD and the GSD module forms the input of the next deconvolution layer. The boundary optimization network adopts a deep supervision strategy. That is, the output of each GSD module is up-sampled and convoluted, and based on the result, an edge loss is calculated compared with the ground truth edge map. The 4 edge losses obtained from each layer are added to the region loss to obtain the total loss of the GCS-Net. The overview of GCS-Net and the illustration of GCD and/or GSD module are shown in Figure 1.
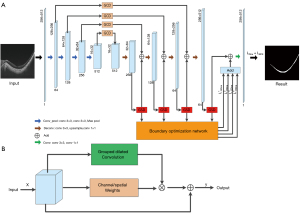
Evaluation metrics
In the validation dataset, the performance of the AI model in segmenting the choroid was quantitatively assessed using 4 evaluation metrics: the intersection-over-union (IoU), the Dice similarity coefficient (DSC), sensitivity, and specificity. The formulas used to calculate IoU and DSC are as follows:
The TP, FP, and FN represent the number of true positive, false positive, and false negative predictions. For image segmentation, TP represents the number of pixels predicted as foreground by automatic segmentation and labeled as foreground in the ground truth, FP represents the number of pixels predicted as foreground but labeled as background in the ground truth, and FN represents the number of pixels predicted as background but labeled as foreground in the ground truth (Figure 2). The IoU and DSC are used to measure the ratio of overlap, which reflects the similarity of the 2 samples. Sensitivity reflects the proportion of correctly segmented foreground parts in the ground truth. Specificity reflects the proportion of correctly segmented background parts in the ground truth. We used Python (v3.7; Python Software Foundation, Wilmington, DE, USA) and the Pytorch (v1.7) deep learning framework of Pycharm (2019, JetBrains, Prague, Czech Republic) software to perform model experiments and to calculate these evaluation metrics.
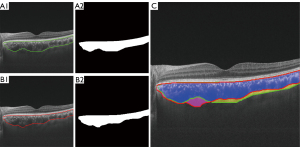
Comparison with clinical ophthalmologist and statistical analysis
After choroid automatic segmentation, the ChT was subsequently calculated by converting the pixel counts into µm, and the results of automatic segmentation were compared with manual segmentation calculated by the built-in Topcon software with its caliper in the ETDRS grid. The lateral magnification was adjusted using AL by Littmann’s formula (31). In the test dataset, the performance of the algorithm to measure the ChT was assessed by calculating the difference between the automated and manual measurements for all 9 measured regions. The intraclass correlation coefficient (ICC) was used to analyze the similarity between the 2 methods. The patients’ characteristics were shown as means ± standard deviation for continuous data and as counts or proportions for categorical data. Data distribution was examined using the Kolmogorov-Smirnov test. The Mann-Whitney U and chi-square tests were used to assess whether there were statistically significant differences between the training and validation datasets. A generalized estimation equation was used to account for internal correlation for binocular data. All statistical analyses were performed using the software SPSS 26.0 (IBM Corp., Armonk, NY, USA), and a 2-tailed P value below 0.05 was deemed statistically significant.
Results
The generalized estimation equation models showed no significant differences in ocular parameters between the 2 eyes; thus, there was no need to adjust for associations between them. A total of 326 eyes of 215 participants were included in this study after comprehensive ophthalmic examinations, comprising 60 eyes in the development dataset and 266 eyes in the test dataset. The development image dataset was subsequently divided into a training set (576 scans) and a validation set (144 scans). Table 1 shows the demographic and clinical characteristics of the training and validation sets, and no significant difference was found in these parameters (P>0.05).
Table 1
Variables | Training dataset | Validation dataset | P value | |||
---|---|---|---|---|---|---|
High myopia | Non-high myopia | High myopia | Non-high myopia | |||
No. of eyes | 32 | 16 | 8 | 4 | ||
No. of images | 384 | 192 | 96 | 48 | ||
Age, y | 70.03±5.96 | 65.00±6.79 | 67.75±6.41 | 68.50±3.79 | 0.956 | |
Gender, male/female | 17/15 | 7/9 | 4/4 | 1/3 | 0.605 | |
SE, diopter | −11.00±3.93 | −0.57±2.01 | −10.94±3.43 | −1.69±3.26 | 0.919 | |
IOP, mmHg | 13.66±2.87 | 14.33±2.33 | 14.19±2.72 | 13.63±3.35 | 0.892 | |
AL, mm | 28.13±0.86 | 23.38±0.48 | 27.50±1.25 | 23.12±1.57 | 0.405 |
SE, spherical equivalent; IOP, intraocular pressure; AL, axial length.
The performance of GCS-Net was evaluated in both HM and non-HM eyes (Table 2). In HM eyes, the IoU, DSC, sensitivity, and specificity were 87.89%, 93.40%, 92.42%, and 99.82%, respectively, thus supporting the ability of GCS-Net in choroidal segmentation. The visual assessment also showed a good agreement between the automated segmented choroid and the manual ground truth (Figure 3).
Table 2
Variables | IoU (%) | DSC (%) | Sensitivity (%) | Specificity (%) |
---|---|---|---|---|
Total | 87.89±6.93 | 93.40±4.10 | 92.81±6.34 | 99.66±0.52 |
High myopia | 87.89±7.10 | 93.40±4.22 | 92.42±6.56 | 99.82±0.11 |
Non-high myopia | 87.88±6.57 | 93.42±3.84 | 93.59±5.79 | 99.33±0.80 |
GCS-Net, group-wise context selection network; IoU, intersection-over-union; DSC, Dice similarity coefficient.
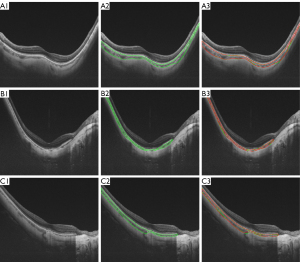
In the test dataset, the algorithm was used for automatic ChT calculation and compared with manual segmentation to further verify the accuracy of the calculation. In this HM test dataset, the average SE was −10.52±3.50 D and the average AL was 27.76±0.99 mm. The average center foveal ChT was 69.6±39.1 µm, and a decreasing trend was found horizontally from the temporal to nasal region (Figure 4). The average difference between the 2 methods was 5.54±4.57 µm with a maximum error of 24.07 µm (Table 3). The ICC was above 0.90 (P<0.001) for all choroid regions. The lowest ICC (0.944, P<0.001) was found in the outer nasal region of the ETDRS grid. With the exception of this region, the ICCs in all other sectors were above 0.97 (P<0.001).
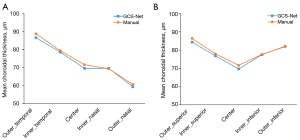
Table 3
Regions (ETDRS) | GCS-Net thickness (μm) | Manual thickness (μm) | Minimum error (μm) | Maximum error (μm) | Error (μm) | ICC (P value) |
---|---|---|---|---|---|---|
Center | 69.6±39.1 | 71.6±35.5 | 0.02 | 28.86 | 5.27±5.13 | 0.981 (P<0.001) |
Inner_temporal | 78.6±40.5 | 79.4±38.1 | 0.02 | 35.58 | 4.79±4.81 | 0.985 (P<0.001) |
Inner_superior | 76.8±41.2 | 77.9±38.2 | 0.08 | 47.81 | 5.42±5.90 | 0.980 (P<0.001) |
Inner_nasal | 69.6±35.8 | 69.4±33.0 | 0.05 | 24.76 | 5.03±4.07 | 0.982 (P<0.001) |
Inner_inferior | 77.4±37.1 | 77.6±35.0 | 0.01 | 46.42 | 5.14±5.22 | 0.979 (P<0.001) |
Outer_temporal | 86.5±39.9 | 88.7±38.7 | 0.03 | 31.70 | 5.92±5.26 | 0.980 (P<0.001) |
Outer_superior | 84.3±38.3 | 86.3±36.4 | 0.02 | 26.53 | 5.19±5.20 | 0.981 (P<0.001) |
Outer_nasal | 59.4±26.6 | 60.7±23.7 | 0.02 | 39.14 | 6.59±5.32 | 0.944 (P<0.001) |
Outer_inferior | 82.1±37.6 | 81.8±36.2 | 0.02 | 23.75 | 4.44±3.71 | 0.988 (P<0.001) |
Average | 75.9±34.4 | 78.5±30.6 | <0.01 | 24.07 | 5.54±4.57 | 0.976 (P<0.001) |
GCS-Net, group-wise context selection network; ETDRS, Early Treatment of Diabetic Retinopathy Study; ICC, intraclass correlation coefficient.
Furthermore, the GCS-Net algorithm was shown to be time-saving. It took about 10 minutes to segment and calculate ChT for 3,192 B-scans in the test dataset, while the same task took approximately 72 hours to complete manually by 1 clinician alone.
Discussion
To our knowledge, this was the first study to use HM eyes as test objects for evaluating automated choroid segmentation and thickness calculation. The GCS-Net shows great agreements with manual segmentation and provides a fast and reliable tool for calculating the thin choroid in HM eyes.
Manual image analysis is time-consuming and subjective. Moreover, automatic segmentation using an internal algorithm in SS-OCT is sometimes inaccurate, which may be due to errors caused by over-smoothing or artifact interference. Therefore, it is necessary to develop credible, objective automated methods to segment the choroid and measure its thickness. There are some studies that included the quantification of retinal features and ChT in AMD eyes (32-34), investigations on optic disc changes and their association with ChT in young myopic patients (35), and the use of algorithms to determine changes in ChT and volume of choroidal vessels (34,36,37). However, previous studies of automated ChT calculations were designed based on mostly non-HM eyes (38-40). Automated identification and calculation of ChT in HM eyes still warrant further study, and our team has research experience in this field (30,41).
Our proposed GCS-Net algorithm to automatically segment the choroid showed good agreement with manual segmentation. The thickness of the choroid was subsequently calculated and its results were compared with manual segmentation calculated by the built-in Topcon software. The average ChT in the test dataset composed of images with HM was 75.9±34.4 µm. As expected, the ChT was thinner than that reported in previous studies, as they had tended to focus on non-HM participants (38,39). By analyzing the difference of ICCs in the 9 regions, the ChT calculated in the outer nasal region was less consistent with the manual. The possible reason is that this region is close to the optic disc, which increases the segmentation difficulty. However, ICC was above 0.90 for all regions of the choroid, and we believe this slight inaccuracy in the outer nasal region is clinically acceptable.
In this study, we measured the average ChT at 9 different regions, and a decreasing trend of ChT was found from the temporal to the nasal region of the macula, which corresponded with some previous research (42,43). In contrast, in the vertical direction, the ChT was quite symmetrical (Figure 4). The superior and inferior regions were thicker, and the fovea was thinnest. Most manual ChT measuring methods in previous studies were at the subfoveal location and at certain distances of the temporal, superior, nasal, and inferior quadrants from the fovea (42,44-46). However, the selected points may not necessarily represent all choroidal pathologies, and variations in the selection of the point used by the observers may lead to interobserver variation. Our study has provided an effective method to manage these problems, and the data could be used to facilitate the differential diagnosis of ocular pathologies that may cause variations in thickness at specific zones within the choroid.
The deep learning algorithm-based GCS-Net is much more efficient and robust than traditional methods, and our previous study compared it to other algorithms (30). Using traditional methods, preprocessing is often needed, such as denoising, enhancement, and retina layer segmentation (27-29), while the GCS-Net is an end-to-end method that produces results from the original B-scans. While many traditional methods such as graph-based ones require the choroid region to be continuous, the GCS-Net can accurately detect the discontinuity caused by the optic disc, pathological atrophy, or retinal folding artifacts. In addition, GCS-Net is a lightweight network with a relatively small number of parameters. This allows successful learning even with a medium-sized training dataset.
Our study had several limitations that have to be acknowledged. First, the algorithm was learnt and tested in the same environment (i.e., the same clinical dataset), thus limiting its application. Second, the algorithm was developed and tested mainly on SS-OCT images centered on the fovea, which highlights the need to validate the algorithm’s performance for the choroid located near the optic disc. Finally, the ChT maps were not evaluated in this study as the automatic topographic map reconstruction from 12-radial scans would lead to large errors. The assessment of the thickness of the choroid on volumetric scans was beyond the scope of this study. To achieve choroid segmentation on images acquired with other scanning protocols, the model has to be retrained with the specific images, or transfer learning techniques need to be applied. The current model segments each B-scan independently. Further extensions that use multiple adjacent B-scans or the whole volume as input should further explore context information to improve accuracy.
In conclusion, the GCS-Net algorithm proposed in our study provides a reliable and fast method to automatically segment and calculate the ChT in HM eyes. Therefore, this tool could be used as a monitoring tool to assess the ChT in HM patients. Moreover, it could also provide a powerful tool for further research on ocular diseases related to thinning of the choroid that could lead to visual impairment.
Acknowledgments
Funding: This study was supported by the National Natural Science Foundation of China (Nos. 81970846 and 81703287), the National Key R&D Program of China (Nos. 2016YFC0904800, 2018YFA0701700, and 2019YFC0840607), the Shanghai Municipal Commission of Health (Public Health System Three-Year Plan - Key Subjects; No. GWV10.1-XK7), the National Science and Technology Major Project of China (No. 2017ZX09304010), the Research Project of Shanghai Health Committee (No. 2019240241), and the Clinical Science and Technology Innovation Project of Shanghai Shenkang Hospital (Nos. SHDC12019X18 and SHDC12020127).
Footnote
Reporting Checklist: The authors have completed the TRIPOD reporting checklist. Available at https://atm.amegroups.com/article/view/10.21037/atm-21-6736/rc
Data Sharing Statement: Available at https://atm.amegroups.com/article/view/10.21037/atm-21-6736/dss
Peer Review File: Available at https://atm.amegroups.com/article/view/10.21037/atm-21-6736/prf
Conflicts of Interest: All authors have completed the ICMJE uniform disclosure form (available at https://atm.amegroups.com/article/view/10.21037/atm-21-6736/coif). The authors have no conflicts of interest to declare.
Ethical Statement: The authors are accountable for all aspects of the work in ensuring that questions related to the accuracy or integrity of any part of the work are appropriately investigated and resolved. The study was conducted in accordance with the Declaration of Helsinki (as revised in 2013). The study was approved by the Ethics Committee of the Shanghai General Hospital, Shanghai Jiao Tong University School of Medicine (No. 2015KY153), and informed consent was provided by all individual participants.
Open Access Statement: This is an Open Access article distributed in accordance with the Creative Commons Attribution-NonCommercial-NoDerivs 4.0 International License (CC BY-NC-ND 4.0), which permits the non-commercial replication and distribution of the article with the strict proviso that no changes or edits are made and the original work is properly cited (including links to both the formal publication through the relevant DOI and the license). See: https://creativecommons.org/licenses/by-nc-nd/4.0/.
References
- Holden BA, Fricke TR, Wilson DA, et al. Global Prevalence of Myopia and High Myopia and Temporal Trends from 2000 through 2050. Ophthalmology 2016;123:1036-42. [Crossref] [PubMed]
- Morgan IG, French AN, Ashby RS, et al. The epidemics of myopia: Aetiology and prevention. Prog Retin Eye Res 2018;62:134-49. [Crossref] [PubMed]
- Williams KM, Bertelsen G, Cumberland P, et al. Increasing Prevalence of Myopia in Europe and the Impact of Education. Ophthalmology 2015;122:1489-97. [Crossref] [PubMed]
- Ohno-Matsui K, Wu PC, Yamashiro K, et al. IMI Pathologic Myopia. Invest Ophthalmol Vis Sci 2021;62:5. [Crossref] [PubMed]
- Wong TY, Ferreira A, Hughes R, et al. Epidemiology and disease burden of pathologic myopia and myopic choroidal neovascularization: an evidence-based systematic review. Am J Ophthalmol 2014;157:9-25.e12. [Crossref] [PubMed]
- Ohno-Matsui K, Lai TY, Lai CC, et al. Updates of pathologic myopia. Prog Retin Eye Res 2016;52:156-87. [Crossref] [PubMed]
- Nickla DL, Wallman J. The multifunctional choroid. Prog Retin Eye Res 2010;29:144-68. [Crossref] [PubMed]
- Read SA, Fuss JA, Vincent SJ, et al. Choroidal changes in human myopia: insights from optical coherence tomography imaging. Clin Exp Optom 2019;102:270-85. [Crossref] [PubMed]
- Margolis R, Spaide RF. A pilot study of enhanced depth imaging optical coherence tomography of the choroid in normal eyes. Am J Ophthalmol 2009;147:811-5. [Crossref] [PubMed]
- Ikuno Y, Kawaguchi K, Nouchi T, et al. Choroidal thickness in healthy Japanese subjects. Invest Ophthalmol Vis Sci 2010;51:2173-6. [Crossref] [PubMed]
- Ding X, Li J, Zeng J, et al. Choroidal thickness in healthy Chinese subjects. Invest Ophthalmol Vis Sci 2011;52:9555-60. [Crossref] [PubMed]
- Ruiz-Medrano J, Flores-Moreno I, Peña-García P, et al. Analysis of age-related choroidal layers thinning in healthy eyes using swept-source optical coherence tomography. Retina 2017;37:1305-13. [Crossref] [PubMed]
- Ruiz-Medrano J, Montero JA, Flores-Moreno I, et al. Myopic maculopathy: Current status and proposal for a new classification and grading system (ATN). Prog Retin Eye Res 2019;69:80-115. [Crossref] [PubMed]
- Yiu G, Chiu SJ, Petrou PA, et al. Relationship of central choroidal thickness with age-related macular degeneration status. Am J Ophthalmol 2015;159:617-26. [Crossref] [PubMed]
- Chung SE, Kang SW, Lee JH, et al. Choroidal thickness in polypoidal choroidal vasculopathy and exudative age-related macular degeneration. Ophthalmology 2011;118:840-5. [Crossref] [PubMed]
- Kardes E, Sezgin Akçay BI, Unlu C, et al. Choroidal Thickness in Eyes with Fuchs Uveitis Syndrome. Ocul Immunol Inflamm 2017;25:259-66. [Crossref] [PubMed]
- Deng J, Li X, Jin J, et al. Distribution Pattern of Choroidal Thickness at the Posterior Pole in Chinese Children With Myopia. Invest Ophthalmol Vis Sci 2018;59:1577-86. [Crossref] [PubMed]
- Ikuno Y, Tano Y. Retinal and choroidal biometry in highly myopic eyes with spectral-domain optical coherence tomography. Invest Ophthalmol Vis Sci 2009;50:3876-80. [Crossref] [PubMed]
- Ikuno Y. OVERVIEW OF THE COMPLICATIONS OF HIGH MYOPIA. Retina 2017;37:2347-51. [Crossref] [PubMed]
- Jin P, Zou H, Zhu J, et al. Choroidal and Retinal Thickness in Children With Different Refractive Status Measured by Swept-Source Optical Coherence Tomography. Am J Ophthalmol 2016;168:164-76. [Crossref] [PubMed]
- De Fauw J, Ledsam JR, Romera-Paredes B, et al. Clinically applicable deep learning for diagnosis and referral in retinal disease. Nat Med 2018;24:1342-50. [Crossref] [PubMed]
- Ting DSW, Peng L, Varadarajan AV, et al. Deep learning in ophthalmology: The technical and clinical considerations. Prog Retin Eye Res 2019;72:100759. [Crossref] [PubMed]
- Sengupta S, Singh A, Leopold HA, et al. Ophthalmic diagnosis using deep learning with fundus images - A critical review. Artif Intell Med 2020;102:101758. [Crossref] [PubMed]
- Lee CS, Baughman DM, Lee AY. Deep learning is effective for the classification of OCT images of normal versus Age-related Macular Degeneration. Ophthalmol Retina 2017;1:322-7. [Crossref] [PubMed]
- Lu W, Tong Y, Yu Y, et al. Deep Learning-Based Automated Classification of Multi-Categorical Abnormalities From Optical Coherence Tomography Images. Transl Vis Sci Technol 2018;7:41. [Crossref] [PubMed]
- Li Y, Feng W, Zhao X, et al. Development and validation of a deep learning system to screen vision-threatening conditions in high myopia using optical coherence tomography images. Br J Ophthalmol 2022;106:633-9. [Crossref] [PubMed]
- Shi F, Tian B, Zhu W, et al. Automated choroid segmentation in three-dimensional 1-μm wide-view OCT images with gradient and regional costs. J Biomed Opt 2016;21:126017. [Crossref] [PubMed]
- Zhang L, Lee K, Niemeijer M, et al. Automated segmentation of the choroid from clinical SD-OCT. Invest Ophthalmol Vis Sci 2012;53:7510-9. [Crossref] [PubMed]
- Alonso-Caneiro D, Read SA, Collins MJ. Automatic segmentation of choroidal thickness in optical coherence tomography. Biomed Opt Express 2013;4:2795-812. [Crossref] [PubMed]
- Shi F, Cheng X, Feng S, et al. Group-wise context selection network for choroid segmentation in optical coherence tomography. Phys Med Biol 2021; [Crossref] [PubMed]
- Bennett AG, Rudnicka AR, Edgar DF. Improvements on Littmann's method of determining the size of retinal features by fundus photography. Graefes Arch Clin Exp Ophthalmol 1994;232:361-7. [Crossref] [PubMed]
- Lee H, Kang KE, Chung H, et al. Automated Segmentation of Lesions Including Subretinal Hyperreflective Material in Neovascular Age-related Macular Degeneration. Am J Ophthalmol 2018;191:64-75. [Crossref] [PubMed]
- Liefers B, Taylor P, Alsaedi A, et al. Quantification of Key Retinal Features in Early and Late Age-Related Macular Degeneration Using Deep Learning. Am J Ophthalmol 2021;226:1-12. [Crossref] [PubMed]
- Zheng F, Gregori G, Schaal KB, et al. Choroidal Thickness and Choroidal Vessel Density in Nonexudative Age-Related Macular Degeneration Using Swept-Source Optical Coherence Tomography Imaging. Invest Ophthalmol Vis Sci 2016;57:6256-64. [Crossref] [PubMed]
- Sun D, Du Y, Chen Q, et al. Imaging Features by Machine Learning for Quantification of Optic Disc Changes and Impact on Choroidal Thickness in Young Myopic Patients. Front Med (Lausanne) 2021;8:657566. [Crossref] [PubMed]
- Zhou H, Chu Z, Zhang Q, et al. Attenuation correction assisted automatic segmentation for assessing choroidal thickness and vasculature with swept-source OCT. Biomed Opt Express 2018;9:6067-80. [Crossref] [PubMed]
- Zhou H, Dai Y, Shi Y, et al. Age-Related Changes in Choroidal Thickness and the Volume of Vessels and Stroma Using Swept-Source OCT and Fully Automated Algorithms. Ophthalmol Retina 2020;4:204-15. [Crossref] [PubMed]
- Zhang L, Buitendijk GH, Lee K, et al. Validity of Automated Choroidal Segmentation in SS-OCT and SD-OCT. Invest Ophthalmol Vis Sci 2015;56:3202-11. [Crossref] [PubMed]
- Lee K, Warren AK, Abràmoff MD, et al. Automated segmentation of choroidal layers from 3-dimensional macular optical coherence tomography scans. J Neurosci Methods 2021;360:109267. [Crossref] [PubMed]
- Masood S, Fang R, Li P, et al. Automatic Choroid Layer Segmentation from Optical Coherence Tomography Images Using Deep Learning. Sci Rep 2019;9:3058. [Crossref] [PubMed]
- Cheng X, Chen X, Feng S, et al. editors. Group-wise attention fusion network for choroid segmentation in OCT images. Progress in Biomedical Optics and Imaging - Proceedings of SPIE; 2020.
- Duan F, Yuan Z, Deng J, et al. Choroidal Thickness and Associated Factors among Adult Myopia: A Baseline Report from a Medical University Student Cohort. Ophthalmic Epidemiol 2019;26:244-50. [Crossref] [PubMed]
- Zhang Q, Neitz M, Neitz J, et al. Geographic mapping of choroidal thickness in myopic eyes using 1050-nm spectral domain optical coherence tomography. J Innov Opt Health Sci 2015;8:1550012. [Crossref] [PubMed]
- Fang Y, Du R, Nagaoka N, et al. OCT-Based Diagnostic Criteria for Different Stages of Myopic Maculopathy. Ophthalmology 2019;126:1018-32. [Crossref] [PubMed]
- El-Shazly AA, Farweez YA, ElSebaay ME, et al. Correlation between choroidal thickness and degree of myopia assessed with enhanced depth imaging optical coherence tomography. Eur J Ophthalmol 2017;27:577-84. [Crossref] [PubMed]
- Lee K, Lee J, Lee CS, et al. Topographical variation of macular choroidal thickness with myopia. Acta Ophthalmol 2015;93:e469-74. [Crossref] [PubMed]