A ceRNA regulatory network in systemic lupus erythematosus and its molecular interplay with cancer
Introduction
Systemic lupus erythematosus (SLE) is an autoimmune disease that is characterized by the production of autoantibodies, and involves multiple organs and systems (1,2). SLE usually occurs in women of childbearing age (3). In recent years, there has been an increase in early, mild, and atypical SLE cases (4). The molecular mechanisms defining the onset and development of SLE are complex, and various pathogeneses have different clinical manifestations and molecular bases. Previous reports have shown that the development of SLE is correlated with genetic, immune, environmental, and sex hormones (5-7). SLE mainly manifests by the production of autoantibodies and immune complexes, and participates in inflammatory processes as well as organ damage (8,9). However, the pathogenesis of SLE remains unclear. Hence, it is important to explore the underlying mechanisms involved in the onset and development of SLE, and identify new molecular markers for the diagnosis and treatment of SLE.
Although genetic factors have been shown to mediate the development of SLE, they cannot fully explain the SLE phenotype (10). Therefore, there is need to focus on the relationship between epigenetics and SLE. The role of non-coding Ribonucleic Acids (ncRNAs), as an important regulator of SLE pathogenesis, has received increased attention. A previous study has shown that most of the human genome does not encode protein-coding genes, which only account for less than 2% (11). Circular RNAs (circRNAs) is a common type of non-coding RNA that originates from precursor messenger RNA (mRNA). CircRNAs consist of a continuous covalently closed loop and do not have the 5’-cap structure and 3’-poly A tail. Owing to its specific structure, circRNA resists degradation by exonuclease Ribonucleases (12). Since circRNAs are mainly enriched in microRNA (miRNA) binding sites, they can act as competitive endogenous RNAs. Through binding with the miRNA, circRNAs can regulate the functions of miRNA target genes, such as ceRNAs (13,14). In addition, previous studies have shown that circRNAs are widely involved in the pathogeneses of lung, colon, gastric, and bladder cancers, as well as autoimmune diseases, such as SLE and rheumatoid arthritis (RA), and are abnormally expressed in serum, peripheral blood mononuclear cells (PBMCs), or kidneys of SLE patients (15-17). A recent study has shown that circRNAs have the potential to be new diagnostic markers in SLE and indicators of disease development (18).
In this study, we developed a circRNA-miRNA-mRNA interaction network consisting of 16 circRNAs, 10 miRNAs, and 40 mRNAs based on gene expression profiles from the Gene Expression Omnibus (GEO) database, our high-throughput sequencing data, and some publicly available bioinformatics platforms. We identified miRNAs and mRNAs that were implicated in the interaction network, as generated by weighted correlation network analysis (WGCNA), protein-protein interaction (PPI) network, as well as Gene Ontology (GO) and Kyoto Encyclopedia of Genes and Genomes (KEGG) pathway analyses to characterize the mechanism of circRNAs in the occurrence and development of SLE. Finally, we conducted a pan-cancer analysis of X-linked apoptosis inhibitory protein-associated factor (XAF1), a hub gene, and explored the role of hub genes, if any, in multiple tumor types. Overall, our study systematically combines internal samples and external GEO datasets to conduct a ceRNA regulatory network and also explore the molecular interactions of the hub gene XAF1 in cancer. This data could provide novel insights into the relationship between the tumors and SLE, and offer effective targeted therapeutic approaches for SLE. We present the following article in accordance with the STREGA reporting checklist (available at https://atm.amegroups.com/article/view/10.21037/atm-22-1533/rc).
Methods
High-throughput sequencing of PBMC samples to obtain circRNAs and miRNAs
We collected peripheral blood samples from inpatients at the Department of Dermatology and Venereology, the First Affiliated Hospital of Guangxi Medical University from June 2019 to July 2019. A human peripheral blood mononuclear cell separation solution (Beijing Solebao Technology Co., Ltd., China) was used to isolate the PBMCs. We used chloroform, isopropanol, 75% ethanol, and other reagents to extract the total RNA from the PBMCs according to the manufacturer’s instructions. We then synthesized complementary DNA (cDNA) and stored it at −20 ℃ for construction of an RNA-Sequencing (RNA-Seq) library. We performed sequencing using the HiSeq4000 sequencing platform (Illumina, USA). The study was conducted in accordance with the Declaration of Helsinki (as revised in 2013). The study was approved by the Ethics Committee of the First Affiliated Hospital of Guangxi Medical University [No. Court Review (2017 Mutual KY-Guoji-081)], and written informed consent was obtained from all patients.
Downloading and sorting of datasets
The mRNA expression dataset used in our study was downloaded from the GEO database, while the mRNA expression profile matrix was obtained from the Gene Expression Omnibus Series (GSE)121239 dataset (292 SLE and 20 normal PBMC samples), and included clinical information such as disease status, SLE disease activity index (SLEDAI), or percentage of neutrophils in peripheral blood.
Differentially-expressed circRNAs, miRNAs and mRNAs
Gene expression profiles were acquired from the GEO database and gene names were identified according to the GEO database platform. The Limma package in R software (Developed by Robert gentleman and Ross ihaka, New Zealand) was used to profile the expression of circRNAs and miRNAs. An adjusted P value <0.05 and |log2fc| >1 was used to screen differentially-expressed circRNAs (DEcircRNAs) and differentially-expressed miRNAs (DEmiRNAs). In addition, GEO2R was used to evaluate the expression of mRNA, and the criteria applied for screening the differentially-expressed mRNAs (DEmRNAs) was as follows: P<0.05 and |log2fc| >1.
Development of circRNA-miRNA-mRNA regulatory network and PPI interaction map
Based on the results from the differential expression analysis, we obtained the DEcircRNAs from the high-throughput sequencing dataset. The circRNA-targeted miRNA dataset was downloaded from the circBank database (http://www.circbank.cn/), and Perl language (Developed by Larry Wall, USA) was used to predict DEcircRNA target miRNAs. We then intersected these targets with the DEmiRNAs, and the results were referred to as IDEmiRNAs. We also used the TargetScan database (http://www.targetscan.org/) to download the miRNA-targeted mRNA dataset, and the Perl language to predict the IDEmiRNA target mRNA, which were then intersected with DEmRNAs and denoted as IDEmRNAs. The interaction between DEcircRNAs, IDEmiRNAs, and IDEmRNAs was used to construct a ceRNA network. The PPI of the IDEmRNAs was analyzed using the Search Tool for Recurring Instances of Neighbouring Genes (STRING) database and then visualized using Cytoscape software.
Quantitative real-time polymerase chain reaction (qRT-PCR)
After the total RNA was extracted, the mRNA level was assessed using TB Green® Premix Ex TaqTMIIKit (Takara, Dalian, China) according to the manufacturer’s instructions, using primers shown in Table 1. The relative expression of the mRNA was calculated by the 2−ΔΔCt method and normalized to β-actin.
Table 1
Gene | Sequences |
---|---|
XAF1 | Forward: 5'-GTGTCCTGCTTGGTGCCTGAATC-3' |
Reverse: 5'-GTCCTTCCGTCCCTTTCTACAGTTC-3' | |
RSAD2 | Forward: 5'-GTGTCCTGCTTGGTGCCTGAATC-3' |
Reverse: 5'-GTCCTTCCGTCCCTTTCTACAGTTC-3' | |
β-actin | Forward: 5'-CAGGCACCAGGGCGTGAT-3' |
Reverse: 5'-TAGCAACGTACATGGCTGGG-3' |
Correlation analysis by WGCNA
WGCNA is a system biology approach that is used to define the interplay between genes and proteins. Furthermore, the genes are integrated into some modules and used to characterize the relationship between each module and the clinical features to identify some clinical parameter-related genes (19). First, we calculated the appropriate soft threshold power (β) and then obtained scale-free topology using a criterion set as R2>0.85. Thereafter, we used the average linkage hierarchical clustering approach to separate genes into distinct modules. There were at least 20 genes in every module, and the module merging threshold was set to 0.25. Pearson correlation analysis was used to assess the relationship between each module and SLE. Modules with a P value <0.05 and high correlation coefficient were selected for further analysis.
Functional enrichment analysis
The database with annotation, visualization, and integrated discovery functions (DAVID) was used for GO and KEGG enrichment analyses (19). We enriched and analyzed the genes involved in key modules identified by WGCNA. The analysis was visualized to explore the potential pathways affected by these genes. GO terms with a P value <0.01 were considered as a significant enrichment.
Pan-cancer analysis of the hub gene
We selected the hub genes by integrating results from the WGCNA key module analysis, IDEmRNA genes, clinical phenotypes, and RT-PCR analysis. To identify the common molecular characteristics in SLE and cancers, the hub gene with the highest Maximal Clique Centrality (MCC) score in the PPI network was submitted for pan-cancer analysis. Pan-cancer analyses were mainly focused on gene expression, survival status, immune-related characteristics, and gene enrichment analysis.
Gene expression analysis
In this study, we used the Kruskal-Wallis test to assess the differential expression of the hub gene in cancer and paracancerous tumors from The Cancer Genome Atlas (TCGA) and Genotype-Tissue Expression Project (GTEx) databases, in order to profile the expression of the hub gene in 34 types of tumor tissues. P<0.05 was considered statistically significant.
Patient survival and prognosis
We first used univariate survival analysis to calculate the relationship between overall survival (OS) and the expression of the hub gene in 44 different tumor types. Kaplan-Meier (KM) plots were then used to obtain the prognostic KM curve of the hub gene in significant tumors. Univariate Cox regression analysis and the log-rank test were used to determine the hazard ratio (HR) and P value of the 95% confidence interval (CI), respectively.
Relationship between gene expression and immunity in different tumors
The tumor immune assessment resource (TIMER) database was systematically used to analyze the immune infiltration of different tumor types using a variety of immune estimation approaches. In addition, the Spearman correlation approach was employed to assess the relationship between the expression of XAF1 and the infiltration levels of immune cells (B cells, CD4+ T cells, CD8+ T cells, dendritic cells, macrophages, and neutrophils). The Spearman approach was also used to analyze the correlation between common immune checkpoint genes and the expression of the hub gene.
Statistical analysis
Statistical analyses involved in this study were all done using R software (version 3.6.3). The comparison between the two groups was done using the independent sample t-test. P<0.05 was considered statistically significant.
Results
Differentially-expressed genes
Integrated analysis of our high-throughput sequencing dataset identified 70 DEcircRNAs, which included seven upregulated DEcircRNAs and 63 downregulated DEcircRNAs (Figure 1A,1B). We also obtained 38 DEmiRNAs, of which 34 were upregulated and four were downregulated (Figure 1C,1D). Finally, we analyzed the expression of genes from the GSE121239 00 dataset using the GEO2R online analysis tool; 289 DEmRNAs were identified, including 98 upregulated and 191 downregulated DEmRNAs (Figure 1E,1F).
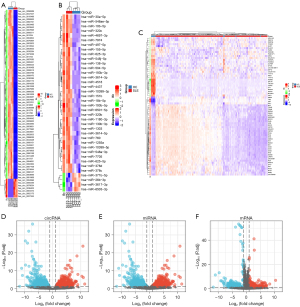
Construction of the ceRNA network and PPI
Using the circRNA-targeted miRNA dataset downloaded from the circBank database, we predicted 571 upregulated miRNAs and 3,227 downregulated miRNAs. Further analysis of the correlation between circRNA and miRNA showed that there was one downregulated IDEmiRNA from the intersection of upregulated miRNAs and downregulated DEmiRNAs (Figure 2A), and 21 upregulated IDEmiRNAs from the intersection of downregulated miRNAs and upregulated DEmiRNAs (Figure 2B). Furthermore, based on the miRNA-targeted mRNA dataset downloaded from the TargetScan database, we predicted 4,291 downregulated IDEmiRNA-targeted mRNAs and 8,812 upregulated IDEmiRNA-targeted mRNAs using Perl language. We intersected the downregulated mRNAs and upregulated DEmRNAs, and obtained 27 upregulated IDEmRNAs (Figure 2C). On the other hand, we also intersected the upregulated IDEmiRNA-targeted mRNAs and downregulated DEmRNAs, and obtained 135 IDEmRNAs (Figure 2D).

To achieve better visualization of the ceRNA network, we obtained 13 IDEmRNA with more than 14 interactions with miRNAs. The analysis showed that circRNAs and miRNAs that were not in the interaction were deleted. After integration, we obtained 17 DEcircRNA, including two upregulated IDEcircRNA and 15 downregulated DEcircRNAs. We also obtained 10 IDEmiRNA, including nine upregulated IDEmiRNA and one downregulated IDEmiRNA. We also obtained 40 IDEmRNA, including 27 upregulated IDEmRNAs and 13 downregulated IDEmRNAs.
Finally, we used R language software package to construct the Sanji diagram of the visual circRNA-miRNA-mRNA interaction network (Figure 3). To construct the PPI network, we fed 40 IDEmRNAs into the STRING database for analysis, and then visualized the results with Cytoscape and its plugin, cytoHubba. We identified a key module by cytoHubba, which included 11 nodes and 110 edges, of which XAF1 and RSAD2 were seed genes (Figure 4).
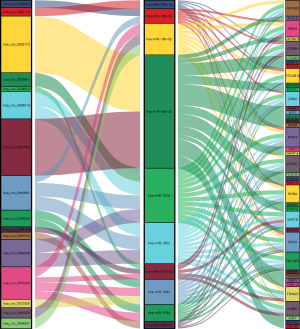
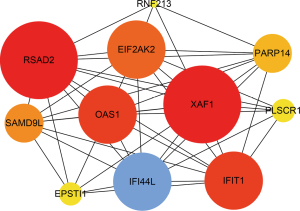
Validation of differentially expressed hub genes in SLE patients of a new group
To further estimate the effect of the GSE121239 dataset, the top two hub genes with the highest MCC scores in the PPI network were applied to assess the expression using in-house samples. Similar to the RNA sequencing results, the expression of XAF1 and RSAD2 were significantly upregulated in the SLE PBMC samples compared to the control samples (Figure 5A,5B).
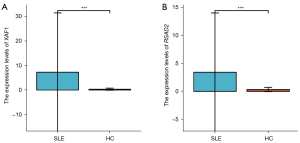
WGCNA identified clinically valuable modules
We used 9,998 genes from the GSE121239 dataset to construct a weighted gene co-expression network. We first constructed a sample clustering tree and showed no obvious outlier samples (Figure 6A). Secondly, when R2>0.85, the soft threshold reached 6, and the average connectivity was relatively high (Figure 6B). Finally, a total of 20 modules were obtained (Figure 6C). Among these modules, the tan module had a correlation coefficient of 0.35 with phenotype 1 (SLEDAI), which was the highest correlation coefficient. Its correlation coefficient with percentage of neutrophils (phenotype 2) was 0.21. Therefore, we selected the tan module, which included 183 genes, as the key module (Table 2).
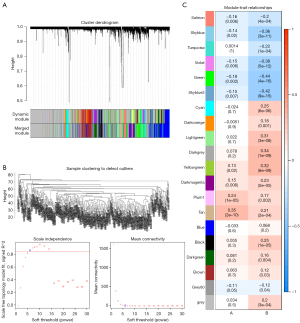
Table 2
Gene | Module | cor_R | A | B |
---|---|---|---|---|
OASL | tan | 0.941313 | 0.368074 | 0.22114 |
XAF1 | tan | 0.931237 | 0.315032 | 0.109385 |
OAS2 | tan | 0.924781 | 0.343379 | 0.115091 |
IFIT3 | tan | 0.920817 | 0.312403 | 0.197628 |
OAS1 | tan | 0.917161 | 0.361821 | 0.209484 |
IFIT1 | tan | 0.915736 | 0.311374 | 0.208413 |
IFI44L | tan | 0.911379 | 0.320178 | 0.17644 |
ISG15 | tan | 0.908596 | 0.323301 | 0.169446 |
LY6E | tan | 0.907333 | 0.342591 | 0.148327 |
LAMP3 | tan | 0.904366 | 0.332263 | 0.161075 |
UBE2L6 | tan | 0.903763 | 0.319622 | 0.216449 |
MX1 | tan | 0.901689 | 0.315547 | 0.206889 |
SERPING1 | tan | 0.900717 | 0.328277 | 0.186711 |
IFIT5 | tan | 0.900539 | 0.353306 | 0.219406 |
PLSCR1 | tan | 0.881469 | 0.318648 | 0.226721 |
EIF2AK2 | tan | 0.878226 | 0.321303 | 0.233111 |
TIMM10 | tan | 0.859709 | 0.331572 | 0.114634 |
IFIH1 | tan | 0.855937 | 0.313833 | 0.190282 |
IFI27 | tan | 0.848601 | 0.309747 | 0.156279 |
MX2 | tan | 0.846245 | 0.356944 | 0.329675 |
Enrichment analysis
The DAVID online analysis tool was used to perform the GO function and KEGG enrichment analyses of proteins encoded by the 183 genes in the tan module, and their biological effects were studied. P<0.01 was used for the GO terms. Significant enrichment was indicated for the following biological processes: defense response to viruses, the type I interferon signaling pathway, innate immune response, negative regulation of viral genome replication, the interferon-gamma-mediated signaling pathway, apoptosis, inflammatory response, immune response, the cell surface receptor signaling pathway, response to interferon-gamma, positive regulation of nuclear factor kappa-B (NF-κB) transcription factor activity, tumor abnormal protein (TAP) dependent mRNA stability regulation, positive regulation of sequence-specific DNA binding transcription factor activity, the tumor necrosis factor (TNF) mediated signaling pathway, and the typical Wingless/Integrated (Wnt) signaling pathway (Figure 7A).
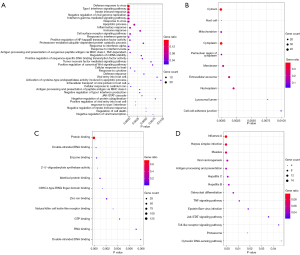
For the cell composition analysis, gene expression was significantly enriched in the cytoplasm, host cell, mitochondria, cytoplasm, perinuclear region, membrane, extracellular body, nuclear plasma, lysosomal lumen, and cell-cell adhesion junction (Figure 7B). In addition, molecular functions were significantly enriched in protein binding, double-stranded RNA binding, enzyme binding, 2-amino-5-oligoadenylate synthase activity, identical protein knot, C3HC4 ring finger domain binding, zinc ion binding, natural killer cell lectin-like receptor binding, guanosine triphosphate (GTP) binding, RNA binding, and double-stranded DNA binding (Figure 7C). A KEGG with a P value <0.01 was considered to indicate significant enrichment of pathways affected by influenza A, herpes simplex virus infection, measles virus carcinogenesis, antigen processing and presentation, hepatitis C, hepatitis B, osteoclast differentiation, the TNF signaling pathway, Epstein-Barr (EB) virus infection, the Janus Kinase-Signal Transducer and Activator of Transcription (JAK-STAT) signaling pathway, the Toll-like receptor signaling pathway, and the proteasome cytoplasmic DNA sensing pathway (Figure 7D).
Pan-cancer analysis of XAF1
Although the GO and KEGG enrichment analyses suggested a potential common biological effect of XAF1 in SLE and tumors, similarities between SLE and tumors in the transcriptome are currently still unclear. XAF1 is one of the two top hub genes in the IDEmRNAs and PPI interaction network that constructed the ceRNA network, and is the key tan module in WGCNA, which is positively correlated with phenotypes 1 and 2. Thus, we performed pan-cancer analysis of XAF1 to understand the biological role of XAF1 in human pan-cancer and explore the similarities between XAF1 in SLE and cancers.
Expression of XAF1 in pan-cancer
We used both the TCGA and the GTEx databases to analyze expression of XAF1 in 34 different types of cancerous and normal tissues. The data showed that XAF1 was significantly downregulated in GBMLGG, LGG, UCEC, BRCA, LUAD, KIRP, COAD, COADREAD, PRAD, LUSC, LIHC, WT, SKCM, THCA, OV, TGCT, UCS, ACC, and KICH. Thus, XAF1 might play a carcinogenic role in multiple cancer types (Figure 8).
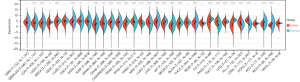
Survival analysis
Survival difference between high and low XAF1 expressions was analyzed using KM survival analysis. Our analyses showed that increased XAF1 expression levels were closely associated with inferior OS in glioblastoma multiforme/lower grade glioma (GBMLGG) (P=1.7e-25), LGG (P=5.7e-16), Pan-kidney cohort (KICH + KIRC + KIRP) (KIPAN) (P=1.7e-6), Acute Myeloid Leukemia (LAML) (P=6.7e-5), and kidney renal clear cell carcinoma (KIRC) (P=7.3e-3), but with superior OS in SKCM (P=8.9e-6) and SKCM-M (P=7.2e-6) (Figure 9). Our findings showed that the expression of XAF1 was closely related to numerous cancer types.
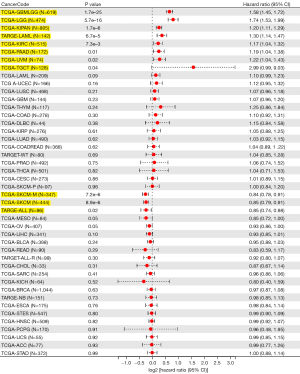
Immune infiltration
To validate the role of XAF1 in the tumor immune microenvironment, the TIMER database was utilized to verify the relationship between XAF1 expression and tumor-infiltrating immune cells (Figure 10A). Correlation analyses showed that XAF1 expression was associated with B cells in 27 cancer types, CD4+ T cells in 35 cancer types, CD8+ T cells in 30 cancer types, neutrophils in 34 cancer types, macrophages in 26 cancer types, and DC cells in 36 cancer types. Also, the expression of XAF1 was markedly positively correlated with infiltrating immune cells in most cancer types.
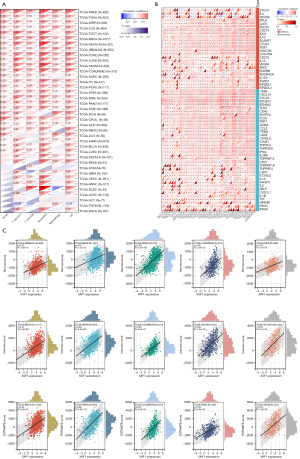
We performed further analysis utilizing the ESTIMATE algorithm to estimate the stromal score, immune score, and estimate score of the infiltration of stromal cells and immunocytes in 44 tumors (Figure 10B). In most tumor types, the expression of XAF1 was positively correlated with infiltrating immune cells. The following tumor types were significantly correlated with the XAF1 expression level: GBMLGG, BRCA, KIPAN, COADREAD, and PRAD (immune score); BRCA, KIPAN, COADREAD, SKCM, and SKCM-M (estimate score); and BRCA, KIPAN, COADREAD, PRAD, and SKCM (stromal score). Immune checkpoints were also shown to be very important in the immunotherapy response. Moreover, to explore the role of XAF1 as a potential therapeutic target, we collected data on 60 common immune checkpoint genes, and analyzed the correlation between the XAF1 expression level and common immune checkpoint genes (Figure 10C).
Discussion
With the continuous improvement in the diagnosis and treatment of SLE, there has been decline in SLE-related mortality over the past 50 years. However, its pathogenesis is still unclear, and high-dose glucocorticoid therapy causes considerable pain to patients, resulting in a poor quality of life (20). Since the expression of circRNAs is very stable and has space-time specificity, circRNAs could be reliable biomarkers with high clinical application prospects (21). In addition, compared with lncRNAs, circRNAs expression in mammalian cells had wider range, higher specificity, and higher stability (22,23). Therefore, there is need to assess the regulatory role, if any, of circRNAs in the pathogenesis of SLE. In this study, we demonstrated novel circRNAs that regulate downstream target genes by acting as sponges that adsorb miRNAs, and estimated the molecular mechanism of the circRNAs. We also intersected datasets downloaded from the GEO database and our high-throughput sequencing dataset according to the negative relationship between circRNA-miRNA and miRNA-mRNA. Finally, we constructed a tertiary circRNA-miRNA-mRNA interaction network based on DEcircRNA, IDEmiRNA, and IDEmRNA.
In our study, we combined the GSE121239 dataset and internal qRT-PCR data to construct a ceRNA network of SLE through various methods. Specifically,we obtained a total of 16 circRNAs (hsa_circ_0027353, hsa_circ_0002173, hsa_circ_0002110, hsa_circ_0045861, hsa_circ_0002003, hsa_circ_0084691, hsa_circ_0081828, hsa_circ_0073236, hsa_circ_0066636, hsa_circ_0003661, hsa_circ_0006515, hsa_circ_0004604, hsa_circ_0060158, hsa_circ_0056589, hsa_circ_0073505, hsa_circ_0061938) in this ceRNA network. Of these, only one study reported up-regulation of hsa_circ_0002003 in Crohn’s disease (CD). The etiology of CD was unclear, but immune factors are thought to be involved (24). As an autoimmune disease, autoimmunity was an important culprit in the pathogenesis of SLE (25). The above evidence indicated that the up-regulated hsa_circ_0002003 expression in SLE may be involved the regulation of immune responses, thereby playing a vital in the occurrence and development of SLE. Data on the other 15 circRNAs has not been previously reported in the literature. Additional experiments are required in the future to define the expression profile of these circRNAs as well as their impact on the pathogenesis of SLE.
In this era of precision medicine, there is more interest in targeted therapy for specific molecular biomarkers as opposed to diseases. A previous study has shown that SLE is associated with an overall increased risk of cancer compared with the general population, but this risk is a function of the type of cancer (26). Therefore, it is imperative to explore common molecular mechanisms between SLE and cancers. Potential risk factors include inherent autoimmune features, such as chronic inflammation, use of immunosuppressive drugs (ISDs), and susceptibility to viral infections (26). SLE patients have an increased incidence of hematological malignancies (non-Hodgkin’s lymphoma, leukemia) and certain solid cancers (vulva and cervix, thyroid, lung, liver) (27). In contrast, SLE appears to play a protective role in hormone-sensitive cancers, such as breast and prostate cancers (28,29). It is possible that SLE-related chronic immune disorders, such as T and B lymphocyte dysfunction, could lead to the uncontrolled activation and proliferation of lymphocytes, thereby increasing the possibility of the malignant transformation of lymphocytes, such as Hodgkin’s lymphoma, non-Hodgkin’s lymphoma, multiple myeloma, and lymphocytic leukemia (30). Notably, immune phenotypes have been shown to be important mediators of carcinogenesis and cancer development.
Multiple carcinoma pathways, such as the Wnt/β-catenin, JAK-STAT, and NF-κB signaling pathways, also actively participate in the development of SLE. Numerous studies have shown that the Wnt/β-catenin signaling pathway is involved in the occurrence and development of a variety of human cancers, such as colorectal, breast, prostate, and liver cancers (31-34). Abnormalities in dendritic cells, B cells, CD4+ T cells, and other immune cells in the pathogenesis of SLE are also related to abnormal Wnt/β-catenin signaling (35-37). Furthermore, the JAK-STAT signaling pathway has been shown to play an important role in the occurrence and development of many types of hematological and solid tumors (38). Similarly, this pathway is also closely related to the occurrence, development and prognosis of SLE (39). NF-κB is a key transcription factor family, which is not only involved in innate immunity, but is also involved in the onset and development of tumors (40). Interestingly, the NF-κB pathway is considered to be a classic pro-inflammatory signaling pathway that can regulate the transcriptional activation of genes related to the pathogenesis of SLE, and its key proteins are significantly upregulated in patients with SLE (41,42). Our GO and KEGG enrichment analyses of the key module genes of the WGCNA showed significant enrichment of the positively regulated status of the typical Wnt signaling pathway, NF-κB transcription factor activity, as well as the JAK-STAT signaling pathway. These findings highlighted multiple similarities between SLE and cancers, which should be explored in the future.
Apoptosis is a programmed cell death process that is activated by several caspases. Previous data has demonstrated that apoptosis can destabilize or degrade structural elements of cells or activate the executors of subsequent apoptotic processes (43,44). X-linked inhibitor of apoptosis protein (XIAP) is a strong apoptosis inhibitory protein that blocks the terminal apoptotic response by binding to Caspases 3, 7, and 9 (45). In contrast, XAF1, an endogenous inhibitor of XIAP, promotes apoptosis by antagonizing XIAP and releasing Caspases via XIAP-mediated inhibition. One of the key features of cancer is the ability to circumvent apoptosis (46). Indeed, there is low expression or deletion of XAF1 in some malignancies, such as prostate cancer, ovarian carcinoma, and cutaneous melanoma (47-50), which is mainly caused by promoter hypermethylation (51). Restoration of the XAF1 expression status inhibits tumor growth, promotes apoptogenesis, and increases tumor sensitivity to apoptosis-inducing factors (52). In addition, XAF1 inhibits the cycle progression of tumor cells (53) and promotes mitotic catastrophe (54). In some malignant tissue samples, XAF1 expression and methylation status have been shown to be correlated with tumor drug resistance and survival, with lower XAF1 expression indicating a worse tumor prognosis (51,55). Therefore, XAF1 plays significant roles in tumorigenesis and development.
Similarly, apoptosis also plays an important role in the pathogenesis of SLE (56). Patients with SLE exhibit increased levels of apoptotic total T-lymphocytes and CD4+ T cells (57). Moreover, increased apoptosis is correlated with SLE disease activity and might be responsible for reduced T cell frequency (58). The major apoptosis induction route in activated lymphocytes is through Fas (59); increased FasL/Fas and caspase-3 expression together with subsequent T-lymphocyte cell apoptosis, particularly in CD4+ T cells, has been detected in human SLE (60,61). However, the response mechanisms to the regulation of apoptosis in SLE remain unclear.
In this study, we identified a hub gene, XAF1, based on evidence from a ceRNA network, WGCNA key module genes, and PPI network analyses. Moreover, qRT-PCR analysis demonstrated that the expression of XAF1 was significantly upregulated in SLE, which was consistent with the bioinformatics analysis results. Therefore, XAF1 might play a potentially important role in SLE. However, there is still no report on the relationship between XAF1 and SLE. According to the pan-cancer analysis conducted in this study, it was demonstrated that increased XAF1 expression levels were positively correlated with OS in SKCM and SKCM-M. Thus, XAF1 seems to play a protective role in SKCM and SKCM-M. Song et al. showed that SLE could decrease the risks of cutaneous melanoma (62). Furthermore, TIMER analysis indicated that XAF1 was markedly positively correlated with tumor lymphocytes levels in most cancer types, including SKCM and SKCM-M. Based on the above evidence, we hypothesize that increased XAF1 expression may induce apoptosis of lymphocytes, especially CD4+ T cells, and also promote the apoptosis of melanoma cells.
Despite the important findings highlighted in this study, there was a lack of in vivo or in vitro evidence to validate the upstream and downstream molecular regulatory mechanisms of XAF1 in SLE. Notably, our study showed that the XAF1 gene plays a key role in the development of multiple tumors and in SLE. Therefore, the specific and common molecular roles of XAF1 in the pathogenesis of SLE and tumors, especially cutaneous melanoma, should be further explored, which might provide new insights into individualized medicine.
Conclusions
Our study comprehensively profiled the expression of circRNAs, miRNAs, and mRNAs in SLE. Further analyses showed that XAF1 is a key molecular biomarker in SLE. In addition, pan-cancer analysis defined the common genomic characteristics in SLE and cancers, especially for SKCM. However, further analysis is needed to validate our findings.
Acknowledgments
Funding: This study was financially supported by the National Natural Science Foundation of China (No. 81760561) in analysis and interpretation of data.
Footnote
Reporting Checklist: The authors have completed the STREGA reporting checklist. Available at https://atm.amegroups.com/article/view/10.21037/atm-22-1533/rc
Data Sharing Statement: Available at https://atm.amegroups.com/article/view/10.21037/atm-22-1533/dss
Conflicts of Interest: All authors have completed the ICMJE uniform disclosure form (available at https://atm.amegroups.com/article/view/10.21037/atm-22-1533/coif). All authors report that this study was financially supported by the National Natural Science Foundation of China (No. 81760561) in analysis and interpretation of data. The authors have no other conflicts of interest to declare.
Ethical Statement: The authors are accountable for all aspects of the work in ensuring that questions related to the accuracy or integrity of any part of the work are appropriately investigated and resolved. The study was conducted in accordance with the Declaration of Helsinki (as revised in 2013). The study was approved by the Ethics Committee of The First Affiliated Hospital of Guangxi Medical University [No. Court Review (2017 Mutual KY-Guoji-081)], and written informed consent was obtained from all patients.
Open Access Statement: This is an Open Access article distributed in accordance with the Creative Commons Attribution-NonCommercial-NoDerivs 4.0 International License (CC BY-NC-ND 4.0), which permits the non-commercial replication and distribution of the article with the strict proviso that no changes or edits are made and the original work is properly cited (including links to both the formal publication through the relevant DOI and the license). See: https://creativecommons.org/licenses/by-nc-nd/4.0/.
References
- Wu H, Zeng J, Yin J, et al. Organ-specific biomarkers in lupus. Autoimmun Rev 2017;16:391-7. [Crossref] [PubMed]
- Wu H, Zhao M, Tan L, et al. The key culprit in the pathogenesis of systemic lupus erythematosus: Aberrant DNA methylation. Autoimmun Rev 2016;15:684-9. [Crossref] [PubMed]
- Zucchi D, Elefante E, Calabresi E, et al. One year in review 2019: systemic lupus erythematosus. Clin Exp Rheumatol 2019;37:715-22. [PubMed]
- Dörner T, Furie R. Novel paradigms in systemic lupus erythematosus. Lancet 2019;393:2344-58. [Crossref] [PubMed]
- Constantin MM, Nita IE, Olteanu R, et al. Significance and impact of dietary factors on systemic lupus erythematosus pathogenesis. Exp Ther Med 2019;17:1085-90. [PubMed]
- Pan L, Lu MP, Wang JH, et al. Immunological pathogenesis and treatment of systemic lupus erythematosus. World J Pediatr 2020;16:19-30. [Crossref] [PubMed]
- Goulielmos GN, Zervou MI, Vazgiourakis VM, et al. The genetics and molecular pathogenesis of systemic lupus erythematosus (SLE) in populations of different ancestry. Gene 2018;668:59-72. [Crossref] [PubMed]
- Durcan L, O'Dwyer T, Petri M. Management strategies and future directions for systemic lupus erythematosus in adults. Lancet 2019;393:2332-43. [Crossref] [PubMed]
- Atisha-Fregoso Y, Toz B, Diamond B. Meant to B: B cells as a therapeutic target in systemic lupus erythematosus. J Clin Invest 2021;131:149095. [Crossref] [PubMed]
- Tsokos GC, Lo MS, Costa Reis P, et al. New insights into the immunopathogenesis of systemic lupus erythematosus. Nat Rev Rheumatol 2016;12:716-30. [Crossref] [PubMed]
- Djebali S, Davis CA, Merkel A, et al. Landscape of transcription in human cells. Nature 2012;489:101-8. [Crossref] [PubMed]
- Kristensen LS, Andersen MS, Stagsted LVW, et al. The biogenesis, biology and characterization of circular RNAs. Nat Rev Genet 2019;20:675-91. [Crossref] [PubMed]
- Chen LL. The expanding regulatory mechanisms and cellular functions of circular RNAs. Nat Rev Mol Cell Biol 2020;21:475-90. [Crossref] [PubMed]
- Tay Y, Rinn J, Pandolfi PP. The multilayered complexity of ceRNA crosstalk and competition. Nature 2014;505:344-52. [Crossref] [PubMed]
- Chen L, Shan G. CircRNA in cancer: Fundamental mechanism and clinical potential. Cancer Lett 2021;505:49-57. [Crossref] [PubMed]
- Zhou Z, Sun B, Huang S, et al. Roles of circular RNAs in immune regulation and autoimmune diseases. Cell Death Dis 2019;10:503. [Crossref] [PubMed]
- Xie R, Zhang Y, Zhang J, et al. The Role of Circular RNAs in Immune-Related Diseases. Front Immunol 2020;11:545. [Crossref] [PubMed]
- Wang X, Ma R, Shi W, et al. Emerging roles of circular RNAs in systemic lupus erythematosus. Mol Ther Nucleic Acids 2021;24:212-22. [Crossref] [PubMed]
- Dennis G Jr, Sherman BT, Hosack DA, et al. DAVID: Database for Annotation, Visualization, and Integrated Discovery. Genome Biol 2003;4:3. [Crossref] [PubMed]
- Eric Y, Shaheen, Magda, Jennifer MP, Mercer, Neil, et al. 46-Year Trends in Systemic Lupus Erythematosus Mortality in the United States, 1968 to 2013 A Nationwide Population-Based Study.
- Yang Q, Li F, He AT, et al. Circular RNAs: Expression, localization, and therapeutic potentials. Mol Ther 2021;29:1683-702. [Crossref] [PubMed]
- Jiang Z, Zhong Z, Miao Q, et al. circPTPN22 as a novel biomarker and ceRNA in peripheral blood mononuclear cells of rheumatoid arthritis. Mol Med Rep 2021;24:617. [Crossref] [PubMed]
- Qu F, Wang L, Wang C, et al. Circular RNA circ_0006168 enhances Taxol resistance in esophageal squamous cell carcinoma by regulating miR-194-5p/JMJD1C axis. Cancer Cell Int 2021;21:273. [Crossref] [PubMed]
- Xu H, Chen W, Zheng F, et al. Reconstruction and analysis of the aberrant lncRNA-miRNA-mRNA network in systemic lupus erythematosus. Lupus 2020;29:398-406. [Crossref] [PubMed]
- Al-Motwee S, Jawdat D, Jehani GS, et al. Association of HLA-DRB1*15 and HLADQB1*06 with SLE in Saudis. Ann Saudi Med 2013;33:229-34. [Crossref] [PubMed]
- Hardenbergh D, Naik R, Manno R, et al. The Cancer Risk Profile of Systemic Lupus Erythematosus Patients. J Clin Rheumatol 2022;28:e257-62. [Crossref] [PubMed]
- Ladouceur A, Clarke AE, Ramsey-Goldman R, et al. Malignancies in systemic lupus erythematosus: an update. Curr Opin Rheumatol 2019;31:678-81. [Crossref] [PubMed]
- Bernatsky S, Ramsey-Goldman R, Labrecque J, et al. Cancer risk in systemic lupus: an updated international multi-centre cohort study. J Autoimmun 2013;42:130-5. [Crossref] [PubMed]
- Bernatsky S, Ramsey-Goldman R, Foulkes WD, et al. Breast, ovarian, and endometrial malignancies in systemic lupus erythematosus: a meta-analysis. Br J Cancer 2011;104:1478-81. [Crossref] [PubMed]
- Bernatsky S, Ramsey-Goldman R, Joseph L, et al. Lymphoma risk in systemic lupus: effects of disease activity versus treatment. Ann Rheum Dis 2014;73:138-42. [Crossref] [PubMed]
- Cheng X, Xu X, Chen D, et al. Therapeutic potential of targeting the Wnt/β-catenin signaling pathway in colorectal cancer. Biomed Pharmacother 2019;110:473-81. [Crossref] [PubMed]
- Wang Z, Li B, Zhou L, et al. Prodigiosin inhibits Wnt/β-catenin signaling and exerts anticancer activity in breast cancer cells. Proc Natl Acad Sci U S A 2016;113:13150-5. [Crossref] [PubMed]
- Schneider JA, Logan SK. Revisiting the role of Wnt/β-catenin signaling in prostate cancer. Mol Cell Endocrinol 2018;462:3-8. [Crossref] [PubMed]
- Vilchez V, Turcios L, Marti F, et al. Targeting Wnt/β-catenin pathway in hepatocellular carcinoma treatment. World J Gastroenterol 2016;22:823-32. [Crossref] [PubMed]
- Szodoray P, Nakken B, Barath S, et al. Altered Th17 cells and Th17/regulatory T-cell ratios indicate the subsequent conversion from undifferentiated connective tissue disease to definitive systemic autoimmune disorders. Hum Immunol 2013;74:1510-8. [Crossref] [PubMed]
- Wang T, Sun X, Zhao J, et al. Regulatory T cells in rheumatoid arthritis showed increased plasticity toward Th17 but retained suppressive function in peripheral blood. Ann Rheum Dis 2015;74:1293-301. [Crossref] [PubMed]
- Orme JJ, Du Y, Vanarsa K, et al. Leukocyte Beta-Catenin Expression Is Disturbed in Systemic Lupus Erythematosus. PLoS One 2016;11:e0161682. [Crossref] [PubMed]
- Kong J, Li L, Zhimin L, et al. Potential protein biomarkers for systemic lupus erythematosus determined by bioinformatics analysis. Comput Biol Chem 2019;83:107135. [Crossref] [PubMed]
- Goropevšek A, Holcar M, Pahor A, et al. STAT signaling as a marker of SLE disease severity and implications for clinical therapy. Autoimmun Rev 2019;18:144-54. [Crossref] [PubMed]
- Pikarsky E, Porat RM, Stein I, et al. NF-kappaB functions as a tumour promoter in inflammation-associated cancer. Nature 2004;431:461-6. [Crossref] [PubMed]
- Hellweg CE. The Nuclear Factor κB pathway: A link to the immune system in the radiation response. Cancer Lett 2015;368:275-89. [Crossref] [PubMed]
- Liu J, Huang X, Hao S, et al. Peli1 negatively regulates noncanonical NF-κB signaling to restrain systemic lupus erythematosus. Nat Commun 2018;9:1136. [Crossref] [PubMed]
- Elmore S. Apoptosis: a review of programmed cell death. Toxicol Pathol 2007;35:495-516. [Crossref] [PubMed]
- Knorr DY, Georges NS, Pauls S, et al. Acetylcholinesterase promotes apoptosis in insect neurons. Apoptosis 2020;25:730-46. [Crossref] [PubMed]
- Liston P, Fong WG, Kelly NL, et al. Identification of XAF1 as an antagonist of XIAP anti-Caspase activity. Nat Cell Biol 2001;3:128-33. [Crossref] [PubMed]
- Fulda S, Vucic D. Targeting IAP proteins for therapeutic intervention in cancer. Nat Rev Drug Discov 2012;11:109-24. [Crossref] [PubMed]
- Lee MG, Huh JS, Chung SK, et al. Promoter CpG hypermethylation and downregulation of XAF1 expression in human urogenital malignancies: implication for attenuated p53 response to apoptotic stresses. Oncogene 2006;25:5807-22. [Crossref] [PubMed]
- Zhao WJ, Deng BY, Wang XM, et al. XIAP associated factor 1 (XAF1) represses expression of X-linked inhibitor of apoptosis protein (XIAP) and regulates invasion, cell cycle, apoptosis, and cisplatin sensitivity of ovarian carcinoma cells. Asian Pac J Cancer Prev 2015;16:2453-8. [Crossref] [PubMed]
- Ng KC, Campos EI, Martinka M, et al. XAF1 expression is significantly reduced in human melanoma. J Invest Dermatol 2004;123:1127-34. [Crossref] [PubMed]
- Kim MA, Lee HE, Lee HS, et al. Expression of apoptosis-related proteins and its clinical implication in surgically resected gastric carcinoma. Virchows Arch 2011;459:503-10. [Crossref] [PubMed]
- Reich TR, Switzeny OJ, Renovanz M, et al. Epigenetic silencing of XAF1 in high-grade gliomas is associated with IDH1 status and improved clinical outcome. Oncotarget 2017;8:15071-84. [Crossref] [PubMed]
- Zhu LM, Shi DM, Dai Q, et al. Tumor suppressor XAF1 induces apoptosis, inhibits angiogenesis and inhibits tumor growth in hepatocellular carcinoma. Oncotarget 2014;5:5403-15. [Crossref] [PubMed]
- Wang J, Gu Q, Li M, et al. Identification of XAF1 as a novel cell cycle regulator through modulating G(2)/M checkpoint and interaction with checkpoint kinase 1 in gastrointestinal cancer. Carcinogenesis 2009;30:1507-16. [Crossref] [PubMed]
- Kim KS, Heo JI, Choi KJ, et al. Enhancement of cellular radiation sensitivity through degradation of Chk1 by the XIAP-XAF1 complex. Cancer Biol Ther 2014;15:1622-34. [Crossref] [PubMed]
- Huang J, Yao WY, Zhu Q, et al. XAF1 as a prognostic biomarker and therapeutic target in pancreatic cancer. Cancer Sci 2010;101:559-67. [Crossref] [PubMed]
- Munoz LE, van Bavel C, Franz S, et al. Apoptosis in the pathogenesis of systemic lupus erythematosus. Lupus 2008;17:371-5. [Crossref] [PubMed]
- Shah D, Aggarwal A, Bhatnagar A, et al. Association between T lymphocyte sub-sets apoptosis and peripheral blood mononuclear cells oxidative stress in systemic lupus erythematosus. Free Radic Res 2011;45:559-67. [Crossref] [PubMed]
- Dhir V, Singh AP, Aggarwal A, et al. Increased T-lymphocyte apoptosis in lupus correlates with disease activity and may be responsible for reduced T-cell frequency: a cross-sectional and longitudinal study. Lupus 2009;18:785-91. [Crossref] [PubMed]
- Trauth BC, Klas C, Peters AM, et al. Monoclonal antibody-mediated tumor regression by induction of apoptosis. Science 1989;245:301-5. [Crossref] [PubMed]
- Amasaki Y, Kobayashi S, Takeda T, et al. Up-regulated expression of Fas antigen (CD95) by peripheral naive and memory T cell subsets in patients with systemic lupus erythematosus (SLE): a possible mechanism for lymphopenia. Clin Exp Immunol 1995;99:245-50. [Crossref] [PubMed]
- Xue C, Lan-Lan W, Bei C, et al. Abnormal Fas/FasL and caspase-3-mediated apoptotic signaling pathways of T lymphocyte subset in patients with systemic lupus erythematosus. Cell Immunol 2006;239:121-8. [Crossref] [PubMed]
- Song L, Wang Y, Zhang J, et al. The risks of cancer development in systemic lupus erythematosus (SLE) patients: a systematic review and meta-analysis. Arthritis Res Ther 2018;20:270. [Crossref] [PubMed]
(English Language Editor: A. Kassem)