Screening of prognostic immune genes and establishment of prognostic model of cervical adenocarcinoma based on bioinformatics analysis
Introduction
Adenocarcinoma is a common pathological type of cervical cancer. Its incidence rate is lower than that of squamous cell carcinoma, accounting for 10–15% (1) of cervical cancer cases. The biological behavior of adenocarcinoma differs from that of squamous cell carcinoma. Adenocarcinoma is not always caused by high-risk human papillomavirus (HPV) infection, and therefore, the HPV vaccine may not prevent adenocarcinoma (2). The prognosis of patients with adenocarcinoma is controversial (3). A study pointed out that its prognosis is equivalent to that of squamous cell carcinoma (3). Meanwhile, Zhu et al. has illustrated that the mortality of adenocarcinoma is higher than that of squamous cell carcinoma over the same period, its sensitivity to chemotherapy and radiotherapy is low, and its prognosis is worse than that of squamous cell carcinoma over the same period (4). Therefore, cervical cancer has different etiologies, morphologies, and prognoses (5,6). Due to the existence of tumor heterogeneity, the prognosis of patients with cervical adenocarcinoma varies greatly. Accurately predicting the prognosis of patients with cervical adenocarcinoma has important clinical significance. At present, most studies are limited to assessing the prognosis of patients with cervical adenocarcinoma through clinical variables, including pathological stage, tumor-node-metastasis (TNM) classification stage, and patient age. Clinical variables cannot accurately predict the prognosis of patients with cervical cancer, and there is controversy among the results of various studies. A study pointed out that the depth of invasion and histological grade are related to prognosis (7). Research also emphasized that the clinical factors of cervical adenocarcinoma lack specificity, and only lymph node metastasis is an independent risk factor for the prognosis of cervical adenocarcinoma (8). There is currently a lack of clinical models to accurately predict the prognosis of cervical adenocarcinoma.
Immune genes have their advantages in judging tumor prognosis. The expression of immune genes has a significant impact on the infiltration of immune cells in tumors, and is a key factor in regulating the tumor microenvironment. This also determines the correlation between immune gene expression and prognosis of tumor patients. Not only that, tumor immunotherapy and the effects of immune-related genes on tumor formation and progression have become a research hotspot in recent years. Immune checkpoint inhibitors, represented by programmed cell death 1 (PD-1) and programmed death ligand 1 (PD-L1), have been widely used in clinical practice, and immunotherapy has been shown to be a good prospect for cervical adenocarcinoma. This study identified differentially expressed immune genes in cervical adenocarcinoma using the Gene Expression Omnibus (GEO) database and the ImmPort database. Combined with the prognostic information of patients, we screened out the immune genes related to prognosis, and established a prognosis prediction model for cervical adenocarcinoma patients. Our study can provide a basis for clinical assessment of patient prognosis and decision-making. We present the following article in accordance with the TRIPOD reporting checklist (available at https://atm.amegroups.com/article/view/10.21037/atm-22-1851/rc).
Methods
Data download
We downloaded and sorted the whole transcriptome sequencing data sets of cervical adenocarcinoma (GSE178629, GSE173112, GSE149450, and GSE192897) from the GEO database (http://www.ncbi.nlm.nih.gov/geo), which included 195 cases of cervical adenocarcinoma tissues and 43 cases of normal cervical tissues. All data sets were sorted into a matrix, and batch correction and normalization were carried out. The corresponding clinical information of the cervical adenocarcinoma samples was downloaded, including survival time, survival status, patient age, case stage, and TNM stage. In addition, immune gene-related information was obtained from the ImmPort database. The study was conducted in accordance with the Declaration of Helsinki (as revised in 2013).
Screening of differentially-expressed genes
The edgeR package (http://bioconductor.org/biocLite.R) was used to analyze the differences in gene expression between cervical adenocarcinoma and normal cervical tissues [fold change (FC) = expression in cervical adenocarcinoma/normal cervical tissue]. In this study, the differentially-expressed genes were screened according to a |log2FC| ≥1 and a false discovery rate (FDR) <0.05. The differentially-expressed genes of cervical adenocarcinoma were intersected with 2,499 immune genes obtained from the ImmPort database to obtain differentially-expressed immune genes.
Enrichment analysis of differentially-expressed immune genes
The differentially-expressed immune genes were enriched and analyzed using the Kyoto Encyclopedia of Genes and Genomes (KEGG) database. Gene Ontology (GO) functional enrichment analysis of differentially-expressed immune genes was carried out using the database for annotation, visualization, and integrated discovery (DAVID). Taking FDR <0.05 as the screening standard, KEGG pathways and GO functional items with significant enrichment were screened.
Screening of prognosis-related immune genes
According to the expression of immune genes and survival information, univariate and multivariate Cox analyses were used to screen the immune genes related to the prognosis of cervical adenocarcinoma, with P<0.05 as the screening standard.
Establishment and evaluation of a risk assessment model
The risk value of each patient was calculated by multiplying the expression of independent prognostic-related genes by the multivariate Cox regression coefficient. The patients were divided into high- and low-risk groups according to the optimal cut-off value. Kaplan-Meier analysis was performed on the two groups of patients using the R-language survival package based on the log-rank test, and the survival curve was drawn. The receiver operating characteristic (ROC) curve was drawn using the R language survivalROC package, and the area under the ROC curve (AUC) was calculated. Area under the curve >0.6 indicates better prediction performance.
Risk score and prognosis of clinical factors were correlated by univariate and multivariate Cox analyses
According to the clinical information and risk score, univariate and multivariate Cox analyses were used to screen the independent prognostic risk factors of cervical adenocarcinoma patients, with P<0.05 as the screening standard.
Statistical analysis
All data in this study were statistically analyzed using R software (V3.5.1) and related R packages. Two-sided P<0.05 or FDR <0.05 was considered to indicate a statistically significant difference.
Results
Differential gene screening
This study analyzed the sequencing data set of 195 cases of cervical adenocarcinoma and 43 cases of normal cervical tissues. Taking |log2FC| ≥1 and FDR <0.05 as the screening criteria, a total of 1,871 differentially-expressed genes were screened, including 1,103 upregulated genes and 768 downregulated genes in cervical adenocarcinoma, as shown in Figure 1A. The immune gene information was obtained from the ImmPort database and intersected with differential genes, and a total of 246 differentially-expressed immune genes were obtained, including 173 upregulated immune genes and 73 downregulated immune genes that were expressed in cervical cancer tissues, as shown in Figure 1B.
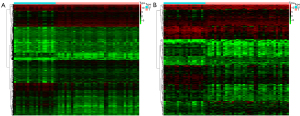
Enrichment analysis of differentially-expressed immune genes
The differentially-expressed immune genes were analyzed using the GO and KEGG pathways. Taking FDR <0.001 as the screening standard, the enrichment results are shown in Table 1.
Table 1
Items | ID | Description | Counts | FDR |
---|---|---|---|---|
MF | GO:0008083 | Growth factor activity | 16 | <0.001 |
GO:0005128 | Cytokine activity | 18 | <0.001 | |
GO:0005179 | Response to oxygen levels | 17 | <0.001 | |
GO:0008009 | Adrenergic receptor activity | 23 | <0.001 | |
CC | GO:0005576 | Extracellular region | 54 | <0.001 |
GO:0005615 | Extracellular space | 51 | <0.001 | |
BP | GO:0007267 | Intercellular signal | 19 | <0.001 |
GO:0006954 | Inflammatory response | 25 | <0.001 | |
GO:0008284 | Cell proliferation was positively regulated | 24 | <0.001 | |
GO:0006955 | Immune response | 31 | <0.001 | |
GO:0070098 | Response to oxygen levels | 16 | <0.001 | |
KEGG | hsa04060 | Cytokine-cytokine receptor interaction | 43 | <0.001 |
hsa04630 | IL-17 signaling pathway | 18 | <0.001 | |
hsa04668 | TNF signaling pathway | 17 | <0.001 | |
hsa04062 | Chemokine signaling pathway | 21 | <0.001 |
MF, molecular function; CC, cellular component; BP, biological process; KEGG, Kyoto Encyclopedia of Genes and Genomes; GO, Gene Ontology; IL-17, interleukin-17; TNF, tumor necrosis factor; FDR, false discovery rate.
Univariate Cox analysis of immune gene prognosis
Univariate Cox analysis was performed by combining the prognostic information and the differential immune gene expression. CXCL9, FGF10, ESM1, FGF9, and BMP5 were found to be protective factors for the prognosis of patients with cervical adenocarcinoma [hazard ratio (HR) <1; P<0.05]. Also, S100A7A, CGB5, CXCL12, PTX3, DEFB126, CXCL10, and CST4 were identified as risk factors for prognosis in patients with cervical adenocarcinoma (HR >1; P<0.05), as shown in Table 2.
Table 2
ID | HR | 95% CI | P value | |
---|---|---|---|---|
Lower | Upper | |||
CXCL9 | 0.58 | 0.14 | 0.84 | 0.022 |
FGF10 | 0.60 | 0.18 | 0.94 | 0.013 |
ESM1 | 0.71 | 0.26 | 0.88 | 0.031 |
FGF9 | 0.77 | 0.20 | 1.09 | 0.027 |
BMP5 | 0.78 | 0.10 | 1.02 | 0.046 |
S100A7A | 1.15 | 0.88 | 1.88 | 0.048 |
CGB5 | 1.32 | 0.85 | 2.77 | 0.026 |
CXCL12 | 1.50 | 0.74 | 2.37 | 0.011 |
PTX3 | 1.71 | 0.62 | 2.29 | 0.041 |
DEFB126 | 1.74 | 0.79 | 1.76 | 0.039 |
CXCL10 | 2.62 | 0.72 | 2.94 | 0.028 |
CST4 | 2.65 | 0.74 | 2.23 | 0.007 |
HR, hazard ratio; CI, confidence interval.
Multivariate Cox analysis of immune gene prognosis
Multivariate Cox analysis of immune gene prognosis was carried out according to the screening results of the univariate Cox analysis. CXCL9 was found to be an independent protective factor for the prognosis of patients with cervical adenocarcinoma (HR <1; P<0.05). Moreover, CGB5, CXCL12, PTX3, and CXCL10 were identified as risk factors for prognosis in patients with cervical adenocarcinoma (HR >1; P<0.05), as shown in Table 3.
Table 3
ID | HR | 95% CI | P value | |
---|---|---|---|---|
Lower | Upper | |||
CXCL9 | 0.63 | 0.35 | 0.96 | 0.025 |
FGF10 | 0.74 | 0.63 | 0.99 | 0.062 |
FGF9 | 0.82 | 0.53 | 1.24 | 0.060 |
BMP5 | 0.82 | 0.53 | 1.10 | 0.084 |
DEFB126 | 0.84 | 0.53 | 1.03 | 0.077 |
S100A7A | 1.04 | 0.88 | 1.23 | 0.323 |
CGB5 | 1.22 | 0.76 | 2.14 | 0.034 |
CXCL12 | 1.33 | 0.93 | 1.86 | 0.023 |
PTX3 | 1.53 | 0.96 | 2.01 | 0.024 |
ESM1 | 1.62 | 0.62 | 1.83 | 0.263 |
CST4 | 1.82 | 1.24 | 2.42 | 0.017 |
CXCL10 | 2.31 | 1.47 | 2.86 | 0.031 |
HR, hazard ratio; CI, confidence interval.
Establishment and evaluation of a survival risk scoring model
A prognostic risk score model was constructed by multivariate Cox regression analysis. The risk score was calculated as follows: risk score = (0.005 × CXCL10) + (0.076 × CGB5) + (0.061 × CXCL12) + (0.034 × PTX3) + (−0.004 × CXCL9). The patients were divided into high- and low-risk scores according to the optimal cut-off risk score values. The prognoses of patients in the high- and low-risk groups were compared by survival analysis. Kaplan-Meier survival analysis of the high- and low-risk groups showed that the prognosis of patients in the low-risk score group was better than that in the high-risk score group (P<0.05), as shown in Figure 2A. The ROC curve was used to evaluate the survival risk score and predict the prognostic efficacy of patients. As shown in Figure 2B, the AUC was 0.713.
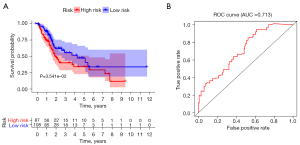
Univariate Cox analysis of risk score and prognosis of clinical variables
As shown in Table 4, univariate Cox analysis showed that the pathological stage, T stage, N stage, and risk score were risk factors for the prognosis of cervical adenocarcinoma (HR >1; P<0.05).
Table 4
Clinical factor | HR | 95% CI | P value | |
---|---|---|---|---|
Lower | Upper | |||
Age | 1.03 | 1.00 | 1.06 | 0.052 |
Gender | 0.59 | 0.34 | 1.03 | 0.062 |
Stage | 1.76 | 1.24 | 2.51 | 0.002 |
T | 1.70 | 1.15 | 2.49 | 0.007 |
M | 2.09 | 0.75 | 5.80 | 0.158 |
N | 1.53 | 1.16 | 2.01 | 0.002 |
Risk score | 1.34 | 1.18 | 1.53 | <0.001 |
T, tumor; M, metastasis; N, node; HR, hazard ratio; CI, confidence interval.
Risk score and clinical variables prognosis related multivariate Cox
As shown in Table 5, multivariate Cox analysis showed that the N stage and risk score were independent risk factors for the prognosis of cervical adenocarcinoma (HR >1; P<0.001).
Table 5
Clinical factor | HR | 95% CI | P value | |
---|---|---|---|---|
Lower | Upper | |||
Age | 1.03 | 1.00 | 1.06 | 0.062 |
Gender | 0.62 | 0.35 | 1.10 | 0.104 |
Stage | 1.01 | 0.49 | 2.08 | 0.968 |
T | 1.58 | 0.95 | 2.64 | 0.079 |
M | 1.08 | 0.34 | 3.41 | 0.902 |
N | 1.34 | 0.79 | 2.28 | 0.035 |
Risk score | 1.37 | 1.19 | 1.59 | <0.001 |
T, tumor; M, metastasis; N, node; HR, hazard ratio; CI, confidence interval.
Discussion
Cervical adenocarcinoma is one of the most common malignant reproductive system tumors in women. The prognosis of patients with cervical adenocarcinoma has been controversial. A study emphasized that the pathological type of cervical adenocarcinoma differs from that of squamous cell carcinoma, but its biological behavior does not affect the prognosis of patients with cervical cancer (3). Meanwhile, another study proposed different views, arguing that the prognosis of cervical adenocarcinoma is worse than that of cervical squamous cell carcinoma (4). In the past, the prognostic evaluation of patients with cervical adenocarcinoma was mainly based on the clinicopathological stage and imaging parameters, which was also the basis for tumor treatment decision-making (8,9). Screening the genes related to the prognosis of cervical adenocarcinoma and constructing an accurate prognosis prediction model can provide a basis for clinical treatment decision-making and identify new treatment targets.
This study screened the immune genes related to prognosis through univariate and multivariate Cox analyses, and constructed a risk score model to predict the prognosis of patients with cervical adenocarcinoma. These immune genes included CXCL10, CGB5, CXCL12, PTX3, and CXCL9. A previous study shown that the prognosis of ovarian cancer patients with high CXCL9 expression is better than that of patients with low CXCL9 expression (10). The present study also showed that CXCL9 was a protective factor for the prognosis of cervical adenocarcinoma (10). Researchers have also shown that CXCL12 promotes the progression of cervical adenocarcinoma, indicating that higher pathological stages are correlated with higher CXCL12 expression. The overexpression of CXCL12 and its receptor CXCR4 may promote lymph node metastasis in cervical adenocarcinoma (11). Also, CXCL10 has been shown to be upregulated in cervical cancer, which is a poor prognostic factor and may be involved in the chemotaxis of neutrophils in tumor tissues (12,13). However, there is no research on the relationship between CXCL10 and the biological behavior of cervical adenocarcinoma.
PTX3 is an inflammatory factor in body fluid and is characterized by a cyclic polymer structure. It can participate in wound healing, tissue remodeling, natural immunity, inflammation, and other processes (14). Recent studies have found that PTX3 is involved in the progression of various tumors and is an independent prognostic factor for lung cancer, cervical cancer, colorectal cancer, primary bone marrow fibrosis, and other tumors (15,16). In cervical cancer, PTX3 may promote tumor cell migration, invasion, and metastasis by regulating epithelial-mesenchymal transdifferentiation-related proteins (17). Another study has shown that PTX3 can promote the dryness of tumor cells, thereby encouraging tumor progression (18).
CGB5 is highly expressed in ovarian cancer and lowly expressed in adjacent tissues, and can further facilitate the invasion of ovarian cancer cells by promoting angiogenesis (19). Its cancer-promoting effect is consistent with the trend of our analysis. We found that the AUC of the risk score model constructed based on the above genes was 0.713, indicating that the model has a high predictive value for the prognosis of cervical adenocarcinoma. Unfortunately, we did not have access to external data to validate our model.
This study also explored the correlation between risk score and clinical factors and the prognosis of patients with cervical cancer. Univariate Cox analysis showed that pathological stage, T stage, N stage, and risk score were risk factors for the prognosis of cervical adenocarcinoma. Furthermore, multivariate analysis showed that N stage and risk score were independent risk factors for the prognosis of cervical adenocarcinoma. These results are consistent with some previously reported research findings. A study has shown that the factors affecting the prognosis of cervical adenocarcinoma include histological grade, lymph node metastasis, and tumor size (7). Another study has pointed out that the prognosis of cervical adenocarcinoma patients with late International Federation of Gynecology and Obstetrics (FIGO) stage, local tumor diameter >4 cm, and poor tumor cell differentiation is poor (20). Previous research has also emphasized that the clinical factors of cervical adenocarcinoma lack specificity, and only lymph node metastasis is an independent risk factor for the prognosis of cervical adenocarcinoma (18). These results are also consistent with our research findings. Nakanishi et al. (21) confirmed that the 5-year survival rate of patients with cervical adenocarcinoma without lymph node metastasis was 94%, while that of patients with cervical adenocarcinoma with lymph node metastasis was only 63%. A study shown that lymph node metastasis is an independent risk factor for cervical adenocarcinoma recurrence (22). As an independent risk factor for the prognosis of cervical adenocarcinoma, a risk score can provide an essential basis for clinical evaluation.
In conclusion, this study established an immune gene prognosis prediction model based on the GEO and ImmPort databases. The model showed good prediction efficiency and can provide a decision-making basis for clinical treatment.
Acknowledgments
Funding: The study was supported by the Shanghai Health System Talent Training Program (No. 2018YQ60 to JN).
Footnote
Reporting Checklist: The authors have completed the TRIPOD reporting checklist. Available at https://atm.amegroups.com/article/view/10.21037/atm-22-1851/rc
Conflicts of Interest: All authors have completed the ICMJE uniform disclosure form (available at https://atm.amegroups.com/article/view/10.21037/atm-22-1851/coif). The authors have no conflicts of interest to declare.
Ethical Statement: The authors are accountable for all aspects of the work in ensuring that questions related to the accuracy or integrity of any part of the work are appropriately investigated and resolved. The study was conducted in accordance with the Declaration of Helsinki (as revised in 2013).
Open Access Statement: This is an Open Access article distributed in accordance with the Creative Commons Attribution-NonCommercial-NoDerivs 4.0 International License (CC BY-NC-ND 4.0), which permits the non-commercial replication and distribution of the article with the strict proviso that no changes or edits are made and the original work is properly cited (including links to both the formal publication through the relevant DOI and the license). See: https://creativecommons.org/licenses/by-nc-nd/4.0/.
References
- Sánchez-Cuerda C, Cuadra M, Gámir S, et al. Cervical adenocarcinoma in situ during pregnancy and subsequent fertility-sparing therapy challenge. Int J Gynaecol Obstet 2021; Epub ahead of print. [Crossref] [PubMed]
- Park KJ. Cervical adenocarcinoma: integration of HPV status, pattern of invasion, morphology and molecular markers into classification. Histopathology 2020;76:112-27. [Crossref] [PubMed]
- Rivera-Colon G, Chen H, Niu S, et al. Cervical Adenocarcinoma: Histopathologic Features From Biopsies to Predict Tumor Behavior. Am J Surg Pathol 2020;44:247-54. [Crossref] [PubMed]
- Zhu HT, Xia R, Zhang X. Clinical characteristics and prognostic factors of cervical adenocarcinoma. Asian J Surg 2021;44:1283-5. [Crossref] [PubMed]
- Wu SY, Huang EY, Lin H. Optimal treatments for cervical adenocarcinoma. Am J Cancer Res 2019;9:1224-34. [PubMed]
- Ma Z, Chen J, Luan T, et al. Proteomic analysis of human cervical adenocarcinoma mucus to identify potential protein biomarkers. PeerJ 2020;8:e9527. [Crossref] [PubMed]
- Zhou J, Chen Y, Xu X, et al. Postoperative clinicopathological factors affecting cervical adenocarcinoma: Stages I-IIB. Medicine (Baltimore) 2018;97:e9323. [Crossref] [PubMed]
- Mabuchi Y, Yahata T, Kobayashi A, et al. Clinicopathologic Factors of Cervical Adenocarcinoma Stages IB to IIB. Int J Gynecol Cancer 2015;25:1677-82. [Crossref] [PubMed]
- Saida T, Sakata A, Tanaka YO, et al. Clinical and MRI Characteristics of Uterine Cervical Adenocarcinoma: Its Variants and Mimics. Korean J Radiol 2019;20:364-77. [Crossref] [PubMed]
- Bronger H, Singer J, Windmüller C, et al. CXCL9 and CXCL10 predict survival and are regulated by cyclooxygenase inhibition in advanced serous ovarian cancer. Br J Cancer 2016;115:553-63. [Crossref] [PubMed]
- Wei M, Liang LZ, Zhang CQ, et al. Correlation of CXCR4/CXCL12 overexpression to lymph node metastasis and chronic inflammation in cervical adenocarcinoma. Ai Zheng 2007;26:298-302. [PubMed]
- Chen X, He H, Xiao Y, et al. CXCL10 Produced by HPV-Positive Cervical Cancer Cells Stimulates Exosomal PDL1 Expression by Fibroblasts via CXCR3 and JAK-STAT Pathways. Front Oncol 2021;11:629350. [Crossref] [PubMed]
- Zhao M, Ma Q, Xu J, et al. Combining CXCL10 gene therapy and radiotherapy improved therapeutic efficacy in cervical cancer HeLa cell xenograft tumor models. Oncol Lett 2015;10:768-72. [Crossref] [PubMed]
- Doni A, Mantovani A, Bottazzi B, et al. PTX3 Regulation of Inflammation, Hemostatic Response, Tissue Repair, and Resolution of Fibrosis Favors a Role in Limiting Idiopathic Pulmonary Fibrosis. Front Immunol 2021;12:676702. [Crossref] [PubMed]
- Garlanda C, Bottazzi B, Magrini E, et al. PTX3, a Humoral Pattern Recognition Molecule, in Innate Immunity, Tissue Repair, and Cancer. Physiol Rev 2018;98:623-39. [Crossref] [PubMed]
- Rubino M, Kunderfranco P, Basso G, et al. Epigenetic regulation of the extrinsic oncosuppressor PTX3 gene in inflammation and cancer. Oncoimmunology 2017;6:e1333215. [Crossref] [PubMed]
- Yu LM, Wang WW, Qi R, et al. MicroRNA-224 inhibition prevents progression of cervical carcinoma by targeting PTX3. J Cell Biochem 2018;119:10278-90. [Crossref] [PubMed]
- Bonavita E, Gentile S, Rubino M, et al. PTX3 is an extrinsic oncosuppressor regulating complement-dependent inflammation in cancer. Cell 2015;160:700-14. [Crossref] [PubMed]
- Andrusiewicz M, Skibińska I, Gąsiorowska E, et al. Fluorescence resonance energy transfer usage to track the sequence promoter changes in CGB5 gene in ovarian cancer patients. Biomed Pharmacother 2017;90:139-46. [Crossref] [PubMed]
- Wang M, Yuan B, Zhou ZH, et al. Clinicopathological characteristics and prognostic factors of cervical adenocarcinoma. Sci Rep 2021;11:7506. [Crossref] [PubMed]
- Nakanishi T, Ishikawa H, Suzuki Y, et al. A comparison of prognoses of pathologic stage Ib adenocarcinoma and squamous cell carcinoma of the uterine cervix. Gynecol Oncol 2000;79:289-93. [Crossref] [PubMed]
- Tabata T, Takeshima N, Tanaka N, et al. Clinical value of tumor markers for early detection of recurrence in patients with cervical adenocarcinoma and adenosquamous carcinoma. Tumour Biol 2000;21:375-80. [Crossref] [PubMed]
(English Language Editor: A. Kassem)