Screening of periodontitis-related genes and immune cells based on an integrated bioinformatics analysis
Introduction
Periodontitis is a common oral disease characterized by gingival inflammation, periodontal pocket formation, alveolar bone resorption and tooth loosening. It is a major oral disease that aggravates or induces systemic diseases, and the continued progression of chronic periodontitis leads to the loosening and loss of teeth and even the complete loss of oral occlusal function, seriously endangering the oral and systemic health of patients (1-4). A health and nutrition survey showed that the prevalence of periodontitis in adults (≥30 years) is 46%, and the likelihood of the disease steadily increases with age (5). A recent study has found that periodontitis susceptibility varies with increasing age, while the degree of periodontal tissue destruction is also correlated with age (6). Additionally, the theory of “age-related susceptibility” suggests that the dysregulation of the immune system or immune aging causes an increased susceptibility to periodontitis (7). Thus, the search for key genes and related immune cells in the pathogenesis of periodontitis is a hot topic of current research.
With the development of high-throughput sequencing technology, the study of differences in gene expression profiles during disease development by methods such as sequencing or microarray technology has become a powerful tool for screening disease-causing genes. Changes in gene expression often indicate alterations in physiological processes or the onset of pathological processes, while the molecular functions (MFs), biological processes (BPs), and signaling pathways involved in the products encoded by genes may be related to the development of diseases.
As the development of periodontitis is a joint effect of multiple genes and their products, changes in the expression of individual genes or proteins are often insufficient to fully reflect the disease development process. Additionally, due to differences in the handling of samples and detection platforms, the results obtained by different investigators are not entirely consistent. Thus, in this study, the Gene Expression Omnibus (GEO) database was screened to obtain a more ideal data set, and the ribonucleic acid-sequencing (RNA-seq) data of the same patients were analyzed before and after treatment, mainly to examine the functions of immune-related differentially expressed genes (iRDEGs), the related pathways, and core genes to inform future research directions. While previous studies have focused on individual cytokines or genes, in this study we integrated immune-related genes to provide a more complete and richer overall package. We present the following article in accordance with the STREGA reporting checklist (available at https://atm.amegroups.com/article/view/10.21037/atm-22-1592/rc).
Methods
Data acquisition
The GEO (https://www.ncbi.nlm.nih.gov/geo/) database was searched for gene expression data sets using the keyword “periodontitis.” The GSE6751 data set was obtained. A total of 59 samples were obtained, 29 of which were from 1 week before periodontitis treatment and 30 of which were from 6–10 weeks after treatment. The acquired RNA-seq data were normalized using R software. The study was conducted in accordance with the Declaration of Helsinki (as revised in 2013).
Screening for differentially expressed genes (DEGs)
A differential analysis was performed on the GSE6751 data set to identify DEGs before and after periodontitis treatment. The DEGs were intersected with the immune genes to identify the iRDEGs. Volcano and heat maps were plotted using the ggplot2 package in R language.
Enrichment function analysis and protein-protein interaction (PPI) network construction
The potential biological functions and pathways of the iRDEGs were explored using clusterProfiler, enrichplot and ggplot2 packages using the R language. The functional enrichment analysis included a gene ontology (GO) analysis of the genes and a pathway analysis. The GO analysis examined the 3 subordinate classes; that is, the MF, BP, and cellular component (CC). The biological pathway information in the pathway analysis was obtained from the Kyoto Encyclopedia of Genes and Genomes (KEGG) database. A P and q value <0.05 indicated significant enrichment functions. The PPI networks for different genes were constructed through STRING sites, and the top 10 genes were calculated using Cytoscape software through the Cytohubba plugin.
Correlation analysis of core genes with immune cells
The core genes among them were selected based on the iRDEGs and subsequently correlated with immune cell correlations for the correlation analysis. The bubble plots (Spearman) showing the correlations between the core genes and the immune cells were generated by the ggplot2, ggtext, and scales packages. A P value <0.05 was considered statistically significant.
Statistical analysis
The Wilcoxon rank-sum test was used to compare differences in gene expression between pre- and post-treatment periodontitis. Differential genes with a logarithmic fold change |logFC| >1 and a false discovery rate (FDR) <0.05 were considered significant; the cluterProfiler package and enrichplot package used hypergeometric distributions to calculate the P and q value for each of the GO or KEGG analyses. A P and q value <0.05 indicated significant enrichment functions; PPI networks for the differential genes were constructed, and those with confidence levels >0.7 and those ranked among the top 10 interacting proteins were selected. The Wilcoxon test and Spearman correlation test were used for the different analysis and the correlation analysis, respectively. All the statistics were completed using the R language package (https://www.r-project.org/), and a P value <0.05 was considered statistically significant.
Results
Screening of DEGs and immune-related DEGs
Based on the screening criteria of an adjusted P value <0.05 and a fold change absolute value >2, 327 DEGs were screened in the GSE6751 data set, of which 149 were upregulated and 178 were down-regulated (Figure 1A,1B). The DEGs were intersected with the immune-related genes, and a total of 27 iRDEGs were screened (Figure 2).
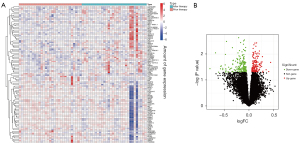
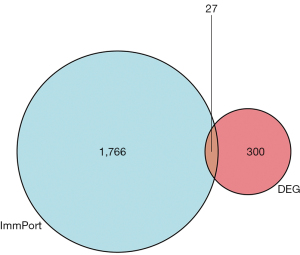
Functional enrichment analysis of immune-associated DEGs
The results of the GO analysis showed that the BPs were mainly involved in granulocyte chemotaxis, granulocyte migration, myeloid leukocyte migration, leukocyte chemotaxis and cell chemotaxis, the CCs were mainly involved in the secretory granule membrane, plasma membrane signaling receptor complex, membrane raft, platelet alpha granule lumen, and platelet alpha granule, while the MFs were mainly involved in the receptor ligand activity, signaling receptor activator activity, cytokine activity, cytokine receptor binding, Toll-like receptor (TLR) binding, and like receptor binding (Figures 1,3A,3B). The results of the pathway analysis showed that the main association with the cytokine-cytokine receptor interaction, phosphatidylinositol-3-kinase and protein kinase B signaling pathway, rheumatoid arthritis, viral protein interaction with cytokine and cytokine receptor, osteoclast differentiation, natural killer cell-mediated cytotoxicity, Ras-proximate-1 signaling pathway, Ras signaling pathway, mitogen-activated protein kinase (MAPK) signaling pathway, and Fluid shear stress and atherosclerosis-related (Figure 4A,4B).
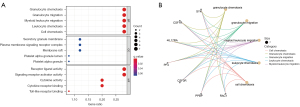
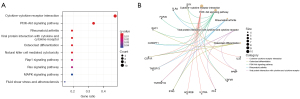
PPI network establishment and core gene screening
We screened the relationships between the iRDEGs and constructed PPI networks. The PF4, ANGPT1, TNFRSF1B, PPBP, CSF3R, IL17RA, SYK, CSF1R, IFNG, and TLR2 were the top 10 genes with the most relationships among nodes. Among them, IFNG and TLR2 had the highest number of adjacent nodes, and we believe that IFNG and TLR2 may be the core genes (Figure 5A,5B). When the expression analysis was performed before and after periodontitis treatment, IFNG (P=0.041) was significantly elevated after treatment and TLR2 (P=0.029) was significantly decreased after treatment (Figure 5C,5D). These results suggest that the screened iRDEGs have a role in the immune system.
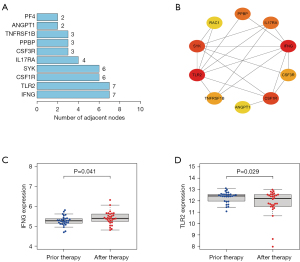
Correlation analysis of core genes and immune cells
We analyzed the correlation between IFNG and TLR2 core genes and immune cells. IFNG was positively correlated with activated CD4 T cells, activated CD8 T cells, CD56dim natural killer cells, natural killer T cells, and negatively correlated with central memory CD8 T cells, regulatory T cells (Tregs), natural killer cells, plasmacytoid dendritic cells, immature dendritic cells, monocytes, and neutrophils (Figure 6). TLR2 was negatively correlated with central memory CD8 T cells, Gamma delta T cells, Type 1 T helper cells, Tregs, immature B cells, memory B cells, myeloid derived suppressor cells, activated dendritic cells, plasmacytoid dendritic cells, immature dendritic cells, macrophages, mast cells, monocytes, and neutrophils, and negatively correlated with Type 17 T helper cells, central memory CD4 T cells, and CD56dim natural killer cells (Figure 7).
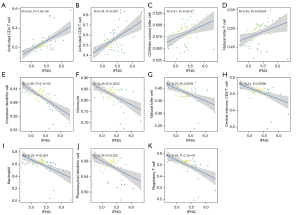
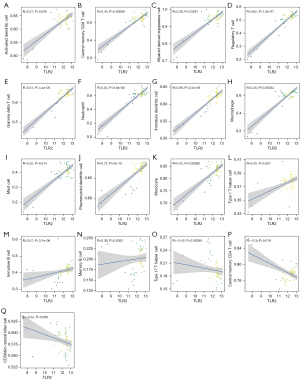
Discussion
Periodontitis is not only a common cause of tooth loss in adults, but also a potential risk factor for many diseases, such as diabetes, chronic obstructive pulmonary disease, and atherosclerosis (3,8). In recent years, the correlation between periodontitis, a chronic inflammatory disease with a high prevalence, and cancer has also received increasing attention. Studies have shown that patients with periodontitis have an elevated risk of developing cancer (9,10). Further, periodontitis has been shown to be correlated with cancers of the head and neck, lung, and gastrointestinal tract (11-13). Several previous studies have shown that biomarkers associated with periodontitis can be sought and established from saliva (14-16), but no biomarkers have been reported for predictable genes. The identification of relevant molecules and signaling pathways associated with the development of periodontitis will extend understanding of the pathogenesis of periodontitis and provide a theoretical basis for the early diagnosis and treatment of periodontitis and the prevention of concomitant diseases.
Based on the transcriptomic data of periodontitis in the GEO database, our findings provide a basis and research direction for the study of the periodontitis mechanism via systematic bioinformatics analysis. As the heterogeneity of samples in time and space reduces the credibility of the experimental results to a certain extent, this study screened the sequencing data before and after treatment for analysis. A total of 327 genes were significantly altered in periodontitis after treatment, 27 of which were immune-related genes. The results of the enrichment analysis showed that these genes were mainly involved in granulocyte chemotaxis, granulocyte migration, leukocyte chemotaxis, cytokine receptor binding, TLR binding, viral protein interactions with cytokines and cytokine receptors, and natural killer cell-mediated cytotoxicity, which suggests that immune response-related pathways play an important role in the development of periodontitis. Among the constructed PPI networks, IFNG and TLR2 are the key core genes and thus are most likely to be the key therapeutic targets for periodontitis.
IFNG is a member of the encoding type-II interferon family. The encoded protein is a soluble cytokine with antiviral, immunomodulatory and antitumor properties. IFNG is the most typical cytokine of Thl cells and also a key cytokine regulating the immune response, which is secreted mainly by CD4+ Th1 cells, CD8 cytotoxic cells, activated natural killer cells and macrophages, activating macrophages and increasing phagocytosis of macrophages (17). Studies have reported that IFNG is elevated in diseased periodontal tissues and is correlated with the severity of periodontitis (18-20). Thus, IFNG may be involved in the pathogenesis of periodontitis by modulating immunity.
TLR2 is a member of the TLR family that plays an important role in pathogen recognition and innate immune activation. TLRs are type-I transmembrane receptors that are significantly expressed in a variety of cell types associated with infections of endodontic origin. TLRs play an important role in the recognition of specific pathogen-associated components and in the transmission of appropriate signals to immune cells (21-24). Research has shown that the most important biological effect of TLRs is to promote the production and release of chemokines and cytokines that trigger the inflammatory response of the body, producing tumor necrosis factor α, interleukin (IL)-1, IL-6, and other cytokines, which are subsequently released into the extracellular space to trigger the inflammatory response (25). A study has shown that TLR2 expression levels are positively correlated with the severity of chronic periodontitis; thus, the detection of TLR2 levels may help to predict disease progression (26). Consistent with these previous findings, our findings suggest a possible potential therapeutic target for periodontitis. TLR2 expression can be used clinically to assess the extent of a patient’s disease and develop and adjust treatment plans with positive implications for improving clinical outcomes.
Gene microarray technology has been developed to help identify oral microorganisms and analyze the diversity and abundance of microorganisms in samples, which can help study the etiology, diagnosis, treatment, and assessment of prognosis of periodontal disease. Based on previous studies, combined with the present study, we can attempt to establish gene chip technology to suggest gene chips of candidate targets for the prevention and treatment of periodontitis, providing a theoretical basis for translating basic research into clinical applications.
Periodontal tissues are exposed to microorganisms for a long time, and multiple immune cells constantly recognize, monitor, and coexist with invaders to maintain periodontal stability and establish an immune system (27). When pathogenic bacteria and their metabolites invade or evade the immune system in large numbers, they cause direct and secondary damage to periodontal tissues (28). Additionally, as the disease progresses and the organism ages, the host’s innate and adaptive immunity against infection is impaired, the degree and duration of the inflammatory response increases, and immune function and antibody-mediated immune response decreases (29,30). It is evident that immune changes in innate and adaptive immunity may affect the development and progression of periodontitis. The interaction between microbial dysbiosis and destructive inflammation is a key pathogenesis of periodontal disease, and up- or down-regulation of genes may affect the levels of immune cells and factors, altering the immune microenvironment and thus influencing the progression of periodontitis.
The presence of a large infiltration of T lymphocytes in the early stages of periodontitis lesions suggests an important role of T cell-mediated cellular immunity in the pathogenesis of periodontal disease. T lymphocytes are derived from bone marrow progenitor cells and are exported to the periphery after developmental maturation in the thymus (31). Upon maturation, T cells are divided into CD4+ T cells and CD8+ T cells based on CD molecules. CD4+ T cells, under the guidance of antigen-presenting cells, differentiate into cell subsets, such as Th1, Th2, Th17, and Tregs, which act on target cells by forming a complex cytokine network and subsequently mediate periodontal immunity (32). CD8+ T cells downregulate inflammation under homeostatic conditions and initiate repair mechanisms to maintain gingival tissue integrity in the presence of tissue damage, inhibit bone destruction cytokines, and repair alveolar bone (33). A study found that maternal CD8+ T cells were more increased in mothers with healthy periodontium than those with periodontitis and suggested that the CD4+/CD8+ ratio is an important indicator of altered host defense systems (34). These studies suggest that immune cells and immune factors play an important role in the development of periodontitis.
Our study showed that IFNG was negatively correlated with most of the immune cells and decreased immune cell levels after treatment, while TLR2 exhibited the opposite behavior to IFNG, which suggests that these 2 core genes play opposite roles in periodontitis. The results of this study are limited and need to be further validated by subsequent molecular biology experiments and animal experiments.
Conclusions
In summary, this study was based on a sample transcriptome sequencing data set, and the genes and related signaling pathways associated with the development of periodontitis were screened and identified by a bioinformatics analysis. A total of 327 periodontitis-related differential genes were identified, including 27 immune-related genes. IFNG and TLR2 may be a key core gene. Viral protein interaction with cytokine and cytokine receptor and Natural killer cell mediated Cytotoxicity and other pathways are newly found signal pathways related to the pathogenesis of periodontitis in this study. Further, in-depth studies on these molecules and pathways will help to elucidate the pathogenesis of periodontitis and identify therapeutic targets.
Acknowledgments
Funding: None.
Footnote
Reporting Checklist: The authors have completed the STREGA reporting checklist. Available at https://atm.amegroups.com/article/view/10.21037/atm-22-1592/rc
Conflicts of Interest: All authors have completed the ICMJE uniform disclosure form (available at https://atm.amegroups.com/article/view/10.21037/atm-22-1592/coif). The authors have no conflicts of interest to declare.
Ethical Statement: The authors are accountable for all aspects of the work in ensuring that questions related to the accuracy or integrity of any part of the work are appropriately investigated and resolved. The study was conducted in accordance with the Declaration of Helsinki (as revised in 2013).
Open Access Statement: This is an Open Access article distributed in accordance with the Creative Commons Attribution-NonCommercial-NoDerivs 4.0 International License (CC BY-NC-ND 4.0), which permits the non-commercial replication and distribution of the article with the strict proviso that no changes or edits are made and the original work is properly cited (including links to both the formal publication through the relevant DOI and the license). See: https://creativecommons.org/licenses/by-nc-nd/4.0/.
References
- Souto GR, Queiroz CM Jr, Costa FO, et al. Relationship between chemokines and dendritic cells in human chronic periodontitis. J Periodontol 2014;85:1416-23. [Crossref] [PubMed]
- Kassebaum NJ, Smith AGC, Bernabé E, et al. Global, Regional, and National Prevalence, Incidence, and Disability-Adjusted Life Years for Oral Conditions for 195 Countries, 1990-2015: A Systematic Analysis for the Global Burden of Diseases, Injuries, and Risk Factors. J Dent Res 2017;96:380-7. [Crossref] [PubMed]
- Slots J. Periodontitis: facts, fallacies and the future. Periodontol 2000 2017;75:7-23. [Crossref] [PubMed]
- Figueredo CM, Lira-Junior R, Love RM T. Int J Mol Sci 2019;20:3949. [Crossref]
- Eke PI, Wei L, Thornton-Evans GO, et al. Risk Indicators for Periodontitis in US Adults: NHANES 2009 to 2012. J Periodontol 2016;87:1174-85. [Crossref] [PubMed]
- López R, Smith PC, Göstemeyer G, et al. Ageing, dental caries and periodontal diseases. J Clin Periodontol 2017;44:S145-52. [Crossref] [PubMed]
- Hajishengallis G. Too old to fight? Aging and its toll on innate immunity. Mol Oral Microbiol 2010;25:25-37. [Crossref] [PubMed]
- Tsuchida S. Proteome Analysis of Molecular Events in Oral Pathogenesis and Virus: A Review with a Particular Focus on Periodontitis. Int J Mol Sci 2020;21:5184. [Crossref] [PubMed]
- Dizdar O, Hayran M, Guven DC, et al. Increased cancer risk in patients with periodontitis. Curr Med Res Opin 2017;33:2195-200. [Crossref] [PubMed]
- Michaud DS, Kelsey KT, Papathanasiou E, et al. Periodontal disease and risk of all cancers among male never smokers: an updated analysis of the Health Professionals Follow-up Study. Ann Oncol 2016;27:941-7. [Crossref] [PubMed]
- Abnet CC, Qiao YL, Dawsey SM, et al. Tooth loss is associated with increased risk of total death and death from upper gastrointestinal cancer, heart disease, and stroke in a Chinese population-based cohort. Int J Epidemiol 2005;34:467-74. [Crossref] [PubMed]
- Michaud DS, Fu Z, Shi J, et al. Periodontal Disease, Tooth Loss, and Cancer Risk. Epidemiol Rev 2017;39:49-58. [Crossref] [PubMed]
- Yoon HS, Wen W, Long J, et al. Association of oral health with lung cancer risk in a low-income population of African Americans and European Americans in the Southeastern United States. Lung Cancer 2019;127:90-5. [Crossref] [PubMed]
- Arias-Bujanda N, Regueira-Iglesias A, Balsa-Castro C, et al. Accuracy of single molecular biomarkers in saliva for the diagnosis of periodontitis: A systematic review and meta-analysis. J Clin Periodontol 2020;47:2-18. [Crossref] [PubMed]
- Kim S, Kim HJ, Song Y, et al. Metabolic phenotyping of saliva to identify possible biomarkers of periodontitis using proton nuclear magnetic resonance. J Clin Periodontol 2021;48:1240-9. [Crossref] [PubMed]
- Zhang Y, Kang N, Xue F, et al. Evaluation of salivary biomarkers for the diagnosis of periodontitis. BMC Oral Health 2021;21:266. [Crossref] [PubMed]
- Holla LI, Hrdlickova B, Linhartova P, et al. Interferon-γ +874A/T polymorphism in relation to generalized chronic periodontitis and the presence of periodontopathic bacteria. Arch Oral Biol 2011;56:153-8. [Crossref] [PubMed]
- Maulani C, Lelyati S, Masulili C, et al. Positive Correlation Between the Level of Interferon-Gamma and the Severity of Periodontitis. Pesquisa Brasileira em Odontopediatria e Clínica Integrada 2019;2019;19:e5106.
- Ramadan DE, Hariyani N, Indrawati R, et al. Cytokines and Chemokines in Periodontitis. Eur J Dent 2020;14:483-95. [Crossref] [PubMed]
- Taheri M, Gholami L, Nicknafs F, et al. Transcript levels of cytokine coding genes in peripheral blood and tissues of patients with periodontitis. Hum Antibodies 2022;30:47-55. [Crossref] [PubMed]
- Hirao K, Yumoto H, Takahashi K, et al. Roles of TLR2, TLR4, NOD2, and NOD1 in pulp fibroblasts. J Dent Res 2009;88:762-7. [Crossref] [PubMed]
- Medzhitov R, Preston-Hurlburt P, Janeway CA Jr. A human homologue of the Drosophila Toll protein signals activation of adaptive immunity. Nature 1997;388:394-7. [Crossref] [PubMed]
- Nilsen N, Nonstad U, Khan N, et al. Lipopolysaccharide and double-stranded RNA up-regulate toll-like receptor 2 independently of myeloid differentiation factor 88. J Biol Chem 2004;279:39727-35. [Crossref] [PubMed]
- Freen-van Heeren JJ. Toll-like receptor-2/7-mediated T cell activation: An innate potential to augment CD8+ T cell cytokine production. Scand J Immunol 2021;93:e13019. [Crossref] [PubMed]
- Rider D, Furusho H, Xu S, et al. Elevated CD14 (Cluster of Differentiation 14) and Toll-Like Receptor (TLR) 4 Signaling Deteriorate Periapical Inflammation in TLR2 Deficient Mice. Anat Rec (Hoboken) 2016;299:1281-92. [Crossref] [PubMed]
- Sumedha S, Kotrashetti VS, Nayak RS, et al. Immunohistochemical localization of TLR2 and CD14 in gingival tissue of healthy individuals and patients with chronic periodontitis. Biotech Histochem 2017;92:487-97. [Crossref] [PubMed]
- Ebersole JL, Dawson D 3rd, Emecen-Huja P, et al. The periodontal war: microbes and immunity. Periodontol 2000 2017;75:52-115. [Crossref] [PubMed]
- Harvey JD. Periodontal Microbiology. Dent Clin North Am 2017;61:253-69. [Crossref] [PubMed]
- Hazeldine J, Lord JM. Innate immunesenescence: underlying mechanisms and clinical relevance. Biogerontology 2015;16:187-201. [Crossref] [PubMed]
- Pinti M, Appay V, Campisi J, et al. Aging of the immune system: Focus on inflammation and vaccination. Eur J Immunol 2016;46:2286-301. [Crossref] [PubMed]
- Linton PJ, Dorshkind K. Age-related changes in lymphocyte development and function. Nat Immunol 2004;5:133-9. [Crossref] [PubMed]
- Irie K, Tomofuji T, Ekuni D, et al. Age-related changes of CD4+ T cell migration and cytokine expression in germ-free and SPF mice periodontium. Arch Oral Biol 2018;87:72-8. [Crossref] [PubMed]
- Cardoso EM, Arosa FA. CD8+ T Cells in Chronic Periodontitis: Roles and Rules. Front Immunol 2017;8:145. [Crossref] [PubMed]
- Kayar NA, Çelik İ, Alptekin NÖ. Immune responses in women with periodontitis and preterm low birth weight: Levels of CD4+ and CD8+ T cells in gingival granulation tissue. Arch Oral Biol 2020;109:104551. [Crossref] [PubMed]