Gut microbiome dysbiosis in patients with hepatitis B virus-related hepatocellular carcinoma after extended hepatectomy liver failure
Introduction
Hepatocellular carcinoma (HCC) is one of the leading causes of cancer-related deaths worldwide, with a relatively higher incidence rate in developing countries, including China (1-3). The main causes of HCC in the Chinese population are viral hepatitis or cirrhosis, especially the hepatitis B virus (4,5). Currently, numerous therapeutic approaches have been applied for treating HCC, such as surgical resection, liver transplantation, ablation treatments (3,6), transcatheter arterial chemoembolization (TACE) (7), radiotherapy (8), and targeted therapy (9). In advanced cases, tyrosine-protein kinase inhibitors (i.e., sorafenib) are considered conventional first-line therapy (10), whereas immune-checkpoint inhibitors are regarded as new treatment options with a limited therapeutic response (11). Surgical treatment is still the most commonly used and effective treatment method for HCC, despite the advances in the treatment mode development and application (12).
Nevertheless, post-hepatectomy liver failure (PHLF) is the most serious complication after liver resection for HCC (13). Extended liver resection is performed during surgery to achieve radical resection margins, which increases the incidence of PHLF (14). As an intrinsic risk of mortality and a life-threatening complication, PHLF is a particular focus for hepatic surgeons in clinical practice. At present, different scoring methods of multiple clinical factors can predict the incidence and outcomes of PHLF (15); however, the basic treatment options are limited to standard intensive care measures, such as mechanical ventilation, vasopressor therapy, dialysis, and administration of coagulation factors and albumin, which provide unsatisfactory results (16). Thus, the development of a comprehensive evaluation system and effective treatment options are urgently required for PHLF.
In recent years, the gut microbiome has received significant attention; it plays a critical role in human health and has been widely regarded as a “hidden organ” (17). In-depth studies have emphasized that the gut microbiome is a key regulator of host metabolism, and there is a complex interaction between the host and intestinal microbiota. It is well known that the liver is an important metabolic organ of the human body. A growing body of evidence suggests that considerable attention is being paid to the interaction between the gut microbiome and liver diseases, since alterations in the microbial composition may induce or reverse liver damage (18,19). For instance, the increased abundance of Prevotella, Bacteroides, and ethanol-producing gut bacteria, and their detrimental metabolites in non-alcoholic fatty liver disease (NAFLD) contributes to liver inflammation by providing toll-like receptor ligands and altering lipid metabolic pathways (20,21). In addition, the increased abundance of Streptococcaceae and endotoxin-producing Enterobacteriaceae coupled with the reduction in short-chain fatty acid (SCFA)-producing taxa are associated with the severity of alcoholic liver disease (ALD) (22).
Chronic hepatitis B leads to liver fibrosis, cirrhosis, and liver cancer, dysregulating gut microbiota and reducing their diversity (23). Bacteroides, Sclerotinia, and Proteus are the characteristic gut microbiota at the onset of hepatitis B, but as the disease progresses (i.e., hepatitis B progression to liver fibrosis/cirrhosis), the abundance of butyrate-producing species (Lachnospiraceae and Ruminococcaceae) decreases, which enhances intestinal barrier permeability and the gut-liver axis, converting some bacteria from beneficial to pathogenic (5,24). In patients with hepatitis B virus-related hepatocellular carcinoma (B-HCC), the gut microbiome is dysbiotic; the high abundance in Ruminococcus, Faecalibacterium, and Ruminiclostridium alters multiple metabolic pathways (such as increased levels of anti-inflammatory SCFAs), thereby accelerating disease progression (25).
In addition, the study has shown that surgical treatment for some liver diseases can also cause damage to the host liver (26), which leads to a series of complications. According to a large number of reported data, the occurrence of complications after liver surgery depends on a variety of factors, such as ischemia reperfusion injury (27), application of immunosuppressive drugs after liver transplantation, or matching based on the donor-organ recipient (28). The study of gut microbiota in liver transplantation and mouse models of hepatectomy has demonstrated that the microbiota plays a vital role in postoperative outcomes (29). For example, the gut microbiota exhibited a decreased abundance of Firmicutes, Clostridiales, Ruminococcaceae, and Lachnospiraceae in a rat experimental model of hepatectomy. In addition, hepatic metabolism has been shown to be associated with the abundance of Lachnospiraceae and Ruminococcaceae, and the gut microbiota impacts hepatocyte regeneration by neutralizing the toxicity of bile salts and altering the bile acid (BA) pool secreted by the liver (30). The imbalanced BA metabolism increases intestinal permeability or decreases the production of SCFAs via the bridging function of the gut-liver axis, altering the gut microbiome composition and stimulating fibrogenesis, together with immune response activation in the graft. Thus, the gut microbiome can be considered a new therapeutic target for improving the long-term survival rates after liver transplantation (31).
Previous study has shown that many metabolites are of microbial origin. For instance, patients with acute-on-chronic liver failure (ACLF) have a relatively higher abundance of Enterococcaceae and phylum Proteobacteria, but a lower abundance of Lachnospiraceae; these changes in the gut microbiota are an important factor in altering crucial metabolic pathways (32). Thus, intestinal microbiota disorders are closely related to metabolic disorders and have profound effects on the prognosis of patients with liver diseases.
The core treatment method for liver cancer is still hepatectomy, despite the dangers of PHLF. Although the relationship between the gut microbiota and liver diseases is widely recognized, there remains a lack of clinical studies exploring the relationship between PHLF after extended hepatectomy (e-PHLF) and the intestinal microbiome. In the present study, we recruited 30 patients with B-HCC who met the inclusion criteria and performed 16S ribosomal RNA (16S rRNA) gene sequencing to identify significant differences in gut microbial abundance and composition related to e-PHLF and non-e-PHLF patients as well as multiple environmental factors. Our data might help reduce the incidence rate of e-PHLF and improve therapeutic outcomes. We present the following article in accordance with the MDAR reporting checklist (available at https://atm.amegroups.com/article/view/10.21037/atm-22-1958/rc).
Methods
Ethics statement
The study was conducted in accordance with the Declaration of Helsinki (as revised in 2013). The study was approved by the Research Ethics Committee of Guangxi Medical University Cancer Hospital (No. KY2019009) and informed consent was taken from all the patients.
Study cohort and sample collection
All patients who were initially diagnosed with B-HCC (Child-Pugh Class A) and only treated with extended hepatectomy at the Department of Hepatobiliary Surgery, Guangxi Medical University Cancer Hospital (Nanning, China), from September 2020 to May 2021 were recruited in this study. Patients with a history of alcohol addiction, drug allergy, chronic infectious diseases, metabolic diseases, inflammatory bowel diseases, non-alcoholic fatty liver, malignant tumors in sites other than the liver, gastrointestinal disease, or diabetes were excluded from the study. None of the participants received any pro-gastrointestinal prokinetic agents, acid suppressants, probiotics, or antibiotics within 4 weeks before surgery.
Our analysis cohort included 30 patients with (Group A, n=15) or without (Group B, n=15) e-PHLF according to the International Study Group of Liver Surgery (ISGLS) (33). Groups A and B were further divided into pre-operation (Ebo.PHLF and Enbo.PHLF, respectively) and post-operation (Eao.PHLF and Enao.PHLF, respectively) groups. Fecal samples were collected at 1 d pre-hepatectomy and 5 d post-hepatectomy, and were immediately stored at −80 ℃.
Deoxyribonucleic acid (DNA) extraction and 16S rRNA gene amplicon sequencing
Bacterial DNA was extracted from each fecal sample using the cetyltrimethylammonium bromide (CTAB) method and used for library construction. The V3–V4 region of the bacterial small-subunit 16S rRNA gene was amplified using the primer pair, 341F (5'-CCTAYGGGRBGCASCAG-3')/806R (5'-GACTACHVGGGTATCTAATCC-3'), as well as and universal sequencing primers. Polymerase chain reaction (PCR) products were purified using the Genejet Gel Extraction kit (Thermo-Fisher Scientific, Waltham, MA, USA). The amplicon libraries were constructed using the TruSeq DNA PCR-Free Sample Preparation kit (Illumina, San Diego, CA, USA). The quality of the library was assessed using the Qubit 2.0 Fluorometer (Thermo-Fisher Scientific) and the Agilent Bioanalyzer 2100 system (Agilent, Santa Clara, CA, USA). The libraries were sequenced on the Illumina Novaseq6000 platform.
16S rRNA data analysis
Quality filtering and analysis of the original FASTQ file were performed using FLASH 1.2.7 (http://ccb.jhu.edu/software/FLASH/) (34) and Qiime 1.9.1 (http://qiime.org/scripts/split_libraries_fastq.html) (35). Operational taxonomic units (OTUs) were obtained using Uparse 7.0.1001 (http://www.drive5.com/uparse/), according to a sequence identity of >97%, and were used to classify bacteria at the genus level (36). Subsequent analyses of α- and β-diversity were performed using OTU normalized data at the phylum, class, order, family, genus, and species levels. Statistical differences in bacterial composition between the groups were assessed using an analysis of similarities (ANOSIM) with the weighted UniFrac. The linear discriminant analysis (LDA) effect size (LEfSe) method was performed to identify differentially-represented biomarker(s) between Groups A and B. Redundancy analysis (RDA) was performed to identify any associations between the microbial composition and environmental factors. PICRUST2 (37) was applied to functional information from the Integrated Microbial Genomes and Microbiomes system to predict metabolic pathways based on the Kyoto Encyclopedia of Genes and Genomes (KEGG) database. The read abundance data for all predicted pathways were converted into relative abundance (%). The co-occurrence network pattern displayed any relationships in the gut microbiome, and also between gut microbiota and different functions. The relationship between gut microbiome and function was investigated using Spearman’s rank-order correlation.
Statistical analysis
All statistical analyses were performed using SPSS 25.0 (IBM, Armonk, NY, USA). Differences between groups were identified using Fisher’s exact test and t-test. Analysis of α- and β-diversity was performed using QIIME 1.9.1 and R (version 2.15.3). LEfSe combines the standard tests for statistical significance (Kruskal-Wallis test and pairwise Wilcoxon test) with LDA. The threshold for the logarithmic LDA score of the discriminative features was set at 3.0. The Mann-Whitney rank-sum test was used for comparisons between groups using R 2.15.3. A permutation test at P<0.05 was used to select a set of environmental factors that significantly affected the microbial distribution. Statistical significance was set at P<0.05. The co-occurrence network pattern, RDA, and Spearman correlations were depicted using R 3.6.2, and any corrections were performed using the Benjamini-Hochberg procedure.
Results
Basic clinical characteristics
The demographic and clinical characteristics of Groups A and B pre-hepatectomy, along with the operation time and intraoperative bleeding volume, are summarized in Table 1. Except for the liver failure severity grading (P<0.001), no other indices were significantly different between the two groups.
Table 1
Characteristic | Overall (n=30) | Group A (n=15) | Group B (n=15) | P value |
---|---|---|---|---|
Age (years) | 47.50±10.81 | 45.33±8.39 | 49.67±12.72 | 0.280 |
Age (years), n (%) | >0.999 | |||
<45 | 13 (43.3) | 7 (46.7) | 6 (40.0) | |
≥45 | 17 (56.7) | 8 (53.3) | 9 (60.0) | |
Sex, n (%) | >0.999 | |||
Female | 5 (16.7) | 2 (13.3) | 3 (20.0) | |
Male | 25 (83.3) | 13 (86.7) | 12 (80.0) | |
BMI (kg/m2), n (%) | 0.715 | |||
≤24 | 16 (53.3) | 7 (46.7) | 9 (60.0) | |
>24 | 14 (46.7) | 8 (53.3) | 6 (40.0) | |
PLT (109/L), n (%) | >0.999 | |||
100–300 | 15 (50.0) | 3 (50.0) | 12 (50.0) | |
<100 or >300 | 15 (50.0) | 3 (50.0) | 12 (50.0) | |
RBP (μg/mL) | 27.23±10.86 | 26.65±11.67 | 27.81±10.36 | 0.775 |
TBIL (μmol/L), n (%) | >0.999 | |||
≤17.1 | 19 (63.3) | 9 (60.0) | 10 (66.7) | |
>17.1 | 11 (36.7) | 6 (40.0) | 5 (33.3) | |
ALB (g/L), n (%) | >0.999 | |||
<35 | 19 (63.3) | 9 (60.0) | 10 (66.7) | |
≥35 | 11 (36.7) | 6 (40.0) | 5 (33.3) | |
PA (mg/L) | 197.68±54.81 | 194.05±56.56 | 201.32±54.73 | 0.723 |
ALT (U/L), n (%) | >0.999 | |||
≤40 | 16 (53.3) | 8 (53.3) | 8 (53.3) | |
>40 | 14 (46.7) | 7 (46.7) | 7 (46.7) | |
AST (U/L), n (%) | >0.999 | |||
≤40 | 17 (56.7) | 8 (53.3) | 9 (60.0) | |
>40 | 13 (43.3) | 7 (46.7) | 6 (40.0) | |
HBV-DNA, n (%) | >0.999 | |||
≤103 | 3 (10.0) | 1 (6.7) | 2 (13.3) | |
>103 | 27 (90.0) | 14 (93.3) | 13 (86.7) | |
PT (s), n (%) | 0.450 | |||
≤13 | 19 (63.3) | 8 (53.3) | 11 (73.3) | |
>13 | 11 (36.7) | 7 (46.7) | 4 (26.7) | |
INR, n (%) | 0.450 | |||
≤1.5 | 19 (63.3) | 8 (53.3) | 11 (73.3) | |
>1.5 | 11 (36.7) | 7 (46.7) | 4 (26.7) | |
AFP (ng/mL), n (%) | >0.999 | |||
≤400 | 14 (46.7) | 7 (46.7) | 7 (46.7) | |
>400 | 16 (53.3) | 8 (53.3) | 8 (53.3) | |
Ascites, n (%) | >0.999 | |||
No | 28 (93.3) | 14 (93.3) | 14 (93.3) | |
Yes | 2 (6.7) | 1 (6.7) | 1 (6.7) | |
Smoking, n (%) | >0.999 | |||
No | 20 (66.7) | 10 (66.7) | 10 (66.7) | |
Yes | 10 (33.3) | 5 (33.3) | 5 (33.3) | |
Drinking, n (%) | >0.999 | |||
No | 28 (93.3) | 14 (93.3) | 14 (93.3) | |
Yes | 2 (6.7) | 1 (6.7) | 1 (6.7) | |
Portal hypertension, n (%) | >0.999 | |||
No | 21 (70.0) | 10 (66.7) | 11 (73.3) | |
Yes | 9 (30.0) | 5 (33.3) | 4 (26.7) | |
Tumor size (cm) | 9.18±4.50 | 9.24±5.09 | 9.12±4.00 | 0.977 |
With or without envelope, n (%) | >0.999 | |||
No | 13 (43.3) | 7 (46.7) | 6 (40.0) | |
Yes | 17 (56.7) | 8 (53.3) | 9 (60.0) | |
Is the envelope intact, n (%) | 0.715 | |||
No | 14 (46.7) | 6 (40.0) | 8 (53.3) | |
Yes | 16 (53.3) | 9 (60.0) | 7 (46.7) | |
Microvascular tumor thrombus, n (%) | 0.715 | |||
No | 16 (53.3) | 9 (60.0) | 7 (46.7) | |
Yes | 14 (46.7) | 6 (40.0) | 8 (53.3) | |
Liver extended resection, n (%) | >0.999 | |||
Left | 10 (33.3) | 5 (33.3) | 5 (33.3) | |
Right | 20 (66.7) | 10 (66.7) | 10 (66.7) | |
γ-GGT (U/L), n (%) | 0.264 | |||
≤50 | 12 (40.0) | 4 (26.7) | 8 (53.3) | |
>50 | 18 (60.0) | 11 (73.3) | 7 (46.7) | |
ICG excretion test | 7.34±3.87 | 8.07±4.46 | 6.61±3.16 | 0.310 |
Intraoperative bleeding (mL), n (%) | >0.999 | |||
≤500 | 21 (70.0) | 11 (73.3) | 10 (66.7) | |
>500 | 9 (30.0) | 4 (26.7) | 5 (33.3) | |
Operation time (minutes) | 255.63±67.50 | 247.07±53.98 | 264.20±79.80 | 0.497 |
Hepatic hilar occlusion time (minutes) | 37.77±21.44 | 33.87±18.53 | 41.67±24.00 | 0.381 |
BCLC stage, n (%) | 0.462 | |||
A | 17 (56.7) | 10 (66.7) | 7 (46.7) | |
B | 13 (43.3) | 5 (33.3) | 8 (53.3) | |
Liver failure grade, n (%) | <0.001 | |||
No | 15 (50.0) | 0 (0.0) | 15 (100.0) | |
1 | 5 (16.7) | 5 (33.3) | 0 (0.0) | |
2 | 9 (30.0) | 9 (60.0) | 0 (0.0) | |
3 | 1 (3.3) | 1 (6.7) | 0 (0.0) |
The P value was based on Fisher Exact test and t-test. Group A and Group B: Group A (n=15) and Group B (n=15) were divided based on the presence or absence of PHLF, which was defined according to the consensus definition and severity grading in the ISGLS report. BMI, body mass index; PLT, platelet; RBP, retinol blinding protein; TBIL, total bilirubin; ALB, albumin; PA, prealbumin; ALT, alanine aminotransferase; AST, aspartate aminotransferase; HBV-DNA, deoxyribonucleic acid of hepatitis B virus; PT, prothrombin time; INR, international normalized ratio; AFP, alpha fetoprotein; γ-GGT, γ-gamma-glutamyltransferase; ICG, constitutional indocyanine green; BCLC stage, Barcelona Clinic Liver Cancer stage.
OTUs and α- and β-diversity
Different microbial taxa were reflected by OTUs. In total, 890 OTUs were observed in Ebo.PHLF vs. Enbo.PHLF; 1,186 in Eao.PHLF vs. Enao.PHLF; 1,522 in Eao.PHLF vs. Ebo.PHLF; and 1,357 in Enao.PHLF vs. Enbo.PHLF (Figure 1A). In order to understand the differences in microbial community structure between different groups, alpha diversity was measured using the Shannon and Simpson indices. The gut microbiota of Eao.PHLF indices were all higher than those of Enao.PHLF (P=0.034 and P=0.031, respectively), but there were no significant differences between Ebo.PHLF and Enbo.PHLF (Figure 1B). As shown in Figure 2A, β-diversity was based on the weighted UniFrac and was represented by principal coordinates analysis (PCoA), which showed that Ebo.PHLF vs. Enbo.PHLF, P=0.081 and R=0.051; Eao.PHLF vs. Enao.PHLF, P=0.004 and R=0.100; Eao.PHLF vs. Ebo.PHLF, P=0.002, R=0.180; and Enao.PHLF vs. Enbo.PHLF, P=0.001, R=0.220. Overall, these results revealed differences in the gut microbial structure and abundance between Eao.PHLF and Enao.PHLF.
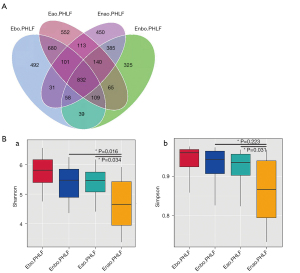
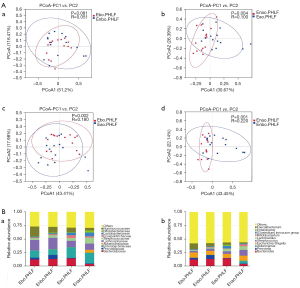
Microbial taxa signatures
The top 10 bacterial abundances at the phylum, class, order, family, and genus levels in Ebo.PHLF, Enbo.PHLF, Eao.PHLF, and Enao.PHLF are shown in Figure S1 & Figure 2B. At the phylum level, the top 10 bacterial members in Ebo.PHLF, Enbo.PHLF, Eao.PHLF, and Enao.PHLF were identical, with some proportional differences: Firmicutes (56.32–47.54%), Bacteroidetes (7.37–24.27%), Proteobacteria (11.31–23.12%), Verrucomicrobiota (0.14–5.30%), Actinobacteriota (2.40–7.97%), Fusobacteriota (0.26–3.15%), unidentified bacteria (0.35–1.60%), Euryarchaeota (0.06–0.91%), Acidobacteriota (0.03–0.28%), and Chloroflexi (0.03–0.25%). The dominant bacteria in Eao.PHLF (21.15%) and Enao.PHLF (7.37%) were Bacteroidota.
At the family and genus levels, the dominant bacteria in the Ebo.PHLF, Enbo.PHLF, Eao.PHLF, and Enao.PHLF were identical, with some proportional differences. At the family level, the dominant bacteria in Ebo.PHLF and Enbo.PHLF were Ruminococcaceae (18.03% and 10.58%, respectively), whereas in Eao.PHLF and Enao.PHLF groups were Bacteroidaceae (14.42% and 4.24%, respectively) and Ruminococcaceae (8.62% and 4.81%, respectively). At the genus level, the dominant bacteria in Ebo.PHLF and Enbo.PHLF were Faecalibacterium (10.75% and 5.88%, respectively), whereas in Eao.PHLF and Enao.PHLF were Bacteroides (14.42% and 4.2%, respectively) and Faecalibacterium (4.15% and 1.63%, respectively).
LEfSe analysis results suggested a significant difference in gut microbiota between Eao.PHLF vs. Enao.PHLF and Ebo.PHLF vs. Enbo.PHLF. At the genus level, the relative abundances of UBA1819, Subdoligranulum, Faecalibacterium, Dongia, and Nordella were higher in Enbo.PHLF than in Ebo.PHLF, whereas the relative abundances of genera UCG-009 (the abundance <0.0001) were higher in Ebo.PHLF than in Enbo.PHLF (Figure 3A). In addition, five genera (Turicibacter, Papillibacter, Scardovia, Catabacter, and Senegalimassilia) were significantly enriched in Enao.PHLF, whereas 10 genera (Bacteroides, Faecalibacterium, Pantoea, Allisonella, Helicobacter, Inquilinus, Mycobacterium, Methylobacterium-Methylorubrum, GCA_900066575, and IS_44) were significantly enriched in Eao.PHLF (Figure 3B).
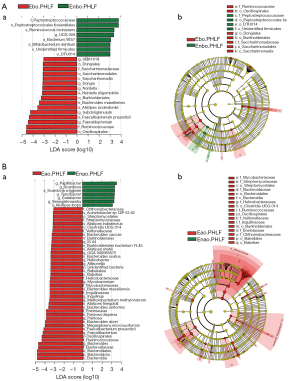
The high abundances of UBA1819 (P=0.015), Subdoligranulum (P=0.002), and Faecalibacterium (P=0.029) were significantly altered between Ebo.PHLF and Enbo.PHLF. Moreover, the abundances of Bacteroides (P=0.002), Faecalibacterium (P=0.026), and Pantoea (P=0.045) were significantly higher in Eao.PHLF than in Enao.PHLF, whereas the abundance of Turicibacter (P=0.041) was lower in Eao.PHLF than in Enao.PHLF. Postoperatively, no significant differences were observed in the taxonomic alterations between Subdoligranulum and UBA1819 microbiota (Figure 4A). Taxonomic alterations of Subdoligranulum and UBA1819 were significant between Ebo.PHLF and Eao.PHLF (P=0.001 and P=0.026, respectively), but not between Enbo.PHLF and Enao.PHLF. The abundance of Subdoligranulum was reduced postoperatively, and that of UBA1819 increased (Figure S2A). The relative abundance of Faecalibacterium decreased in postoperatively compared to that preoperatively, and the differences were statistically significant in both PHLF (P=0.001) and non-PHLF (P<0.001) patients (Figure 4B). However, the different relative abundance of Faecalibacterium in Groups A and B pre- and post-operatively were not significant (Figure 4B).
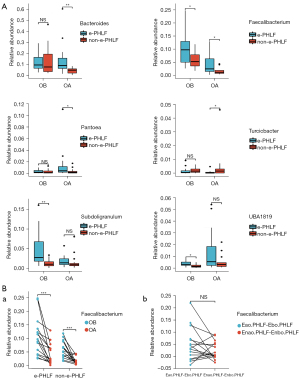
In addition, the postoperative values in high-abundance species were not significantly different from the preoperative values, except for those of Faecalibacterium. Thus, the postoperative alterations in bacterial taxa might be reliable microbial markers for detecting the occurrence of liver failure. We also found an interaction network between differential bacteria in Eao.PHLF vs. Enao.PHLF and Ebo.PHLF vs. Enbo.PHLF, as well as in the top 30 bacteria (Figure S2B).
Gut microbiota functional signatures in Eao.PHLF vs. Enao.PHLF
Analysis of the functional capacity of the gut microbiota in Eao.PHLF vs. Enao.PHLF predicted 57 pathways related to the differential gut microbiome at the genus level. As shown in Figure 5 and Table 2, the microbial metabolic pathways mainly included multiple amino acid metabolic pathways, organic acid metabolism pathways, pyrimidine metabolism pathways, palmitate biosynthesis, and stearate biosynthesis.
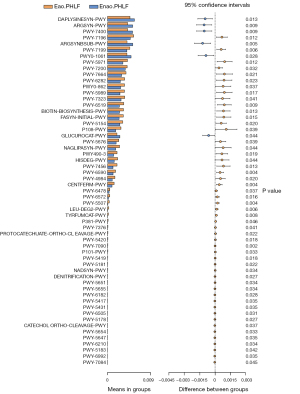
Table 2
Pathway ID | Altered pathway | P value |
---|---|---|
DAPLYSINESYN-PWY | L-lysine biosynthesis I | 0.013 |
ARGSYN-PWY | L-arginine biosynthesis I (via L-ornithine) | 0.009 |
PWY-7400 | L-arginine biosynthesis IV (archaebacteria) | 0.009 |
PWY-7196 | Superpathway of pyrimidine ribonucleosides salvage | 0.012 |
ARGSYNBSUB-PWY | L-arginine biosynthesis II (acetyl cycle) | 0.005 |
PWY-7199 | Pyrimidine deoxyribonucleosides salvage | 0.006 |
PWY0-1061 | Superpathway of L-alanine biosynthesis | 0.028 |
PWY-5971 | Palmitate biosynthesis II (bacteria and plants) | 0.012 |
PWY-7200 | Superpathway of pyrimidine deoxyribonucleoside salvage | 0.032 |
PWY-7664 | Oleate biosynthesis IV (anaerobic) | 0.021 |
PWY-6282 | Palmitoleate biosynthesis I (from (5Z)-dodec-5-enoate) | 0.023 |
PWY0-862 | (5Z)-dodec-5-enoate biosynthesis | 0.037 |
PWY-5989 | Stearate biosynthesis II (bacteria and plants) | 0.017 |
PWY-7323 | Superpathway of GDP-mannose-derived O-antigen building blocks | 0.041 |
PWY-6519 | 8-amino-7-oxononanoate biosynthesis I | 0.009 |
BIOTIN-BIOSYNTHESIS-PWY | Biotin biosynthesis I | 0.013 |
FASYN-INITIAL-PWY | Superpathway of fatty acid | 0.015 |
PWY-5154 | L-arginine biosynthesis III (via N-acetyl-L-citrulline) | 0.020 |
P108-PWY | Pyruvate fermentation to propanoate I | 0.039 |
GLUCUROCAT-PWY | Superpathway of β-D-glucuronide and D-glucuronate degradation | 0.044 |
PWY-5676 | Acetyl-CoA fermentation to butanoate II | 0.039 |
NAGLIPASYN-PWY | Lipid IVA biosynthesis | 0.044 |
PWY490-3 | L-tryptophan degradation | 0.019 |
HISDEG-PWY | L-histidine degradation I | 0.044 |
PWY-7456 | Mannan degradation | 0.013 |
PWY-6590 | Superpathway of Clostridium acetobutylicum acidogenic | 0.004 |
PWY-4984 | Urea cycle | 0.020 |
CENTFERM-PWY | Pyruvate fermentation to butanoate | 0.004 |
PWY-6478 | GDP-D-glycero-α-D-manno-heptose biosynthesis | 0.037 |
PWY-6572 | Chondroitin sulfate degradation I (bacterial) | 0.016 |
PWY-5507 | Adenosylcobalamin biosynthesis I (early cobalt insertion) | 0.004 |
LEU-DEG2-PWY | L-tyrosine degradation I | 0.006 |
TYRFUMCAT-PWY | L-tyrosine degradation I | 0.008 |
P381-PWY | Adenosylcobalamin biosynthesis II (late cobalt incorporation) | 0.046 |
PWY-7376 | Cob(II)yrinate a,c-diamide biosynthesis II (late cobalt incorporation) | 0.041 |
PROTOCATECHUATE-ORTHO-CLEAVAGE-PWY | Protocatechuate degradation II (ortho-cleavage pathway) | 0.022 |
PWY-5420 | Catechol degradation II (meta-cleavage pathway) | 0.018 |
PWY-7090 | UDP-2,3-diacetamido-2,3-dideoxy-alpha-D-mannuronate biosynthesis | 0.002 |
P101-PWY | Ectoine biosynthesis | 0.033 |
PWY-5419 | Catechol degradation to 2-oxopent-4-enoate II | 0.018 |
PWY-5181 | Toluene degradation III (aerobic) (via p-cresol) | 0.022 |
NADSYN-PWY | NAD biosynthesis II (from tryptophan) | 0.034 |
DENITRIFICATION-PWY | Nitrate reduction I | 0.027 |
PWY-5651 | L-tryptophan degradation to 2-amino-3-carboxymuconate semialdehyde | 0.034 |
PWY-5655 | L-tryptophan degradation IX | 0.034 |
PWY-6182 | Superpathway of salicylate degradation | 0.028 |
PWY-5417 | Catechol degradation III (ortho-cleavage pathway) | 0.035 |
PWY-5431 | Aromatic compounds degradation via &beta | 0.035 |
PWY-6505 | L-tryptophan degradation XII (Geobacillus) | 0.031 |
PWY-5178 | Toluene degradation IV (aerobic) (via catechol) | 0.027 |
CATECHOL-ORTHO-CLEAVAGE-PWY | Catechol degradation to β-ketoadipate | 0.037 |
PWY-5654 | 2-amino-3-carboxymuconate semialdehyde degradation to 2-oxopentenoate | 0.033 |
PWY-5647 | 2-nitrobenzoate degradation I | 0.035 |
PWY-6210 | 2-aminophenol degradation | 0.034 |
PWY-5183 | Superpathway of aerobic toluene degradation | 0.042 |
PWY-6992 | 1,5-anhydrofructose degradation | 0.035 |
PWY-7084 | Nitrifier denitrification | 0.045 |
Correlation between microbial composition and environmental factors
The relationship between the environmental factors and different microbial compositions was analyzed in Eao.PHLF vs. Enao.PHLF. For bacteria and Eao.PHLF vs. Enao.PHLF, the RDA axes 1 and 2 accounted for 42.1% and 26.85% of the total variation, respectively. The permutation test at P<0.05 showed that total bilirubin (TBIL), international normalized ratio (INR), prealbumin (PA), and albumin (ALB) were associated with differential bacterial compositions in Eao.PHLF vs. Enao.PHLF. Bacteroides, Faecalibacterium, and Pantoea were positively correlated with TBIL, INR, and ALB, but negatively correlated with PA. Turicibacter was negatively correlated with TBIL, INR, and ALB, but positively correlated with PA (Figure 6A).
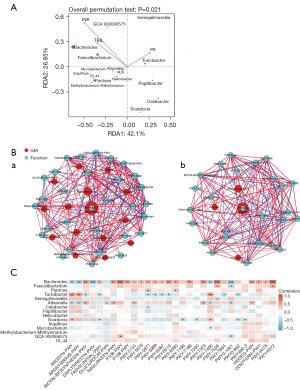
Complex postoperative network features
In Eao.PHLF vs. Enao.PHLF, 15 different gut microbiota and the top 30 functional groups were well suited to display the interaction between the microbiome and various functions (Figure 6B). In particular, high-abundance species, including Bacteroides, Faecalibacterium, Pantoea, and Turicibacter, were found to play a significant role in the association between major metabolic pathways, including multiple amino acid metabolic pathways, organic acid metabolism pathways, pyrimidine metabolism pathways, palmitate biosynthesis, and stearate biosynthesis, suggesting their central role in the Eao.PHLF vs. Enao.PHLF microbiome (Figure 6C).
Discussion
The gut microbiome is considered a “hidden organ” that regulates human health, including liver function. It seems to play a complex role in the progression of liver diseases, and thus, identifying any alterations in intestinal microbial composition might help to establish novel therapeutic targets (38). Extended hepatectomy is a well-established risk factor for PHLF. Previous study has explored the correlation between liver diseases and gut microbiota; however, information on any changes in the intestinal flora of B-HCC patients with or without PHLF is still limited (39). In our study, we used 16S rRNA gene sequencing to identify differential gut microbiota in Eao.PHLF vs. Enao.PHLF that could be used as non-invasive biomarkers for diagnosis and treatment in B-HCC patients with e-PHLF.
Based on α- and β-diversity analysis, we found that the microbial composition and relative abundance at the genus level changed markedly in Eao.PHLF vs. Enao.PHLF, but not in Ebo.PHLF vs. Enbo.PHLF. Previous study has shown that the intestinal microbiome causes health disorders under various conditions, such as abdominal surgery and antibacterial therapy (40). Consistent with these reports, our results showed that extended hepatectomy causes dysbiosis of the intestinal microbiome. In Eao.PHLF vs. Enao.PHLF, 15 genera had a LDA >3. The abundances of Bacteroides, Faecalibacterium, and Pantoea were higher in Eao.PHLF, whereas that of Turicibacter was higher in Enao.PHLF. The dominant bacteria in Ebo.PHLF were Faecalibacterium, Subdoligranulum, and UBA1819. The bacteria with significant postoperative differences showed no significant differences preoperatively, except for Faecalibacterium, which could be a potential marker of disease diagnosis.
Faecalibacterium were reported to be potential markers of gut health (41); inflammatory processes were promoted when Faecalibacterium was decreased, leading to the occurrence of disease (42). Many beneficial bacteria that promote gut health can be consumed to resist disease (43). We found that Faecalibacterium both decreased after extended hepatectomy, especially in the presence of PHLF. Additionally, these different gut microbiota and the top 30 bacteria were well suited to promote and repress each other in a cooperative manner both pre- and post-operatively. We assumed that the steep reduction in the abundance of Faecalibacterium derived from its resistance to the occurrence of liver failure as well as possible constraints from other bacteria. In summary, we believe that Bacteroides, Faecalibacterium, Pantoea, and Turicibacter could be used as non-invasive characteristic biomarkers for e-PHLF.
PICRUST2 analysis revealed significant differences in the microbiome functional abundance between Eao.PHLF and Enao.PHLF. In Eao.PHLF, L-lysine biosynthesis, L-arginine biosynthesis, and L-alanine biosynthesis decreased, whereas L-tyrosine degradation, L-histidine degradation, L-leucine degradation, and L-tryptophan degradation increased. The functional abundance was very low during L-leucine degradation. In addition, organic acid metabolism pathways (acetyl-CoA fermentation to butanoate II and pyruvate fermentation to propanoate I), pyrimidine metabolism pathways, palmitate biosynthesis, and stearate biosynthesis were all increased. Amino acid metabolism only functions in the liver, and any disease or injury, such as hepatitis B, cirrhosis, and hepatic encephalopathy, is inevitably accompanied by amino acid balance disorders (44-46). For instance, arginine reduction is a specific biomarker for acute liver injury (47); lysine exhibits strong free radical scavenging activities,inhibits free radical-mediated damage, and protects against liver injury (48,49); and decreased alanine inhibits gluconeogenesis and leaves the glucose-dependent liver at risk of energy deprivation, ultimately leading to damage (50,51). Our study found that arginine, lysine, and alanine biosynthesis decreased significantly in e-PHLF. Moreover, tyrosine degradation produces metabolites, such as succinylacetone, that are toxic to the liver (52). In contrast, histidine supplementation has been shown to suppress inflammatory processes and decrease liver injury (53). We observed a significant increase in the histidine degradation pathway in Group A. Previous studies suggested that hepatic injury might be related to metabolic disorders in tryptophan metabolism and that pyrimidine metabolism disorders are associated with cellular liver damage (54,55). All of these previous reports are consistent with the findings of our research.
In e-PHLF, butyrate and propionate are the products of organic acid metabolism, which induce gut hormones and reduce liver inflammation (56). We believe that the gut microbiota performs multiple functions and plays a vital role in numerous metabolic systems, especially in the interaction between the metabolic pathway (57,58). Among them, amino acid metabolism is affected and/or regulated by diverse factors, such as synthesis and catabolism, and interacts with other metabolic pathways (59). Although organic acid metabolism plays a role in protecting the liver, it cannot resist other functions that lead to liver injury. In a mouse model, palmitoleate supplementation was shown to reduce the number of macrophages/Kupffer cells in the liver and promote the expression of proinflammatory cytokines (60). In the present study, palmitoleate biosynthesis and stearate biosynthesis were significantly increased in Group A. Overexposure to palmitoleate aggravates cell regeneration difficulty (61). Also, palmitoleate can further elongate into stearic acid (59), which promotes the expression of inflammatory factors (62). Overall, we concluded that the gut microbiome is involved in the pathogenesis of liver injury.
The levels of TBIL, INR, ALB, and PAare biomarkers of hepatic damage (63-65). We found that increased TBIL and INR levels and decreased ALB levels, which reflect severe liver damage, were negatively correlated with the RDA1 axis. In contrast, PA levels were positively correlated with the RDA1 axis. There was also a significant correlation between TBIL, INR, ALB, and PA and the abundance and composition of intestinal bacteria, which indicated that the gut microbiome is highly susceptible to liver disease. Thus, exploring the changes and functions of intestinal microbiota post-extended hepatectomy might reveal novel targets for preventing and treating e-PHLF.
Through network analysis, we found that the differential bacteria in Groups A and B after surgery were closely related to the function of the top 30. The performance of species was significantly related to their abundance. It is known that an increase in Bacteroides promotes liver injury by reducing competitive inhibition between gut bacteria, increasing the release of inflammatory factors, and upregulating bacterial toxins (66). Our research found that an increase in Bacteroides was negatively correlated with amino acid metabolism, but positively correlated with pyrimidine metabolism, organic acid metabolism, palmitoleate biosynthesis, and stearate biosynthesis, thereby promoting liver injury. It has been reported that the enteric microbiome and the host complement each other, whereas any disorder in the gut microecology is exacerbated during the disease state, with beneficial functions being markedly impaired (67). Faecalibacterium has been reported to be a potential marker of gut health (68). We found that it was negatively correlated with amino acid metabolism in e-PHLF, and thus, its beneficial function was not sufficient to protect the liver or may also inhibit the protective effect of amino acid metabolism on liver. Pantoea is a genus of gram-negative bacteria belonging to the Enterobacteriaceae family,which has been isolated in patients with liver failure accompanied by bloodstream infections (69,70). Congruent with this, we found that an increase in Pantoea significantly elevated palmitoleate biosynthesis and organic acid metabolism. Furthermore, Turicibacter modulates inflammatory responses and exerts anti-inflammatory effects (67). In Enbo.PHLF, an increase in Turicibacter enhanced the synthesis and metabolism of arginine, but decreased palmitoleate biosynthesis and stearate biosynthesis, probably protecting the liver. Therefore, dysbiotic changes in microbial functions and interaction patterns might affect the progression of e-PHLF.
Our study had some limitations that should be noted. Firstly, the number of patients involved was small, and thus, our findings need to be confirmed in a more extensive cohort. Secondly, we only used 16S rRNA gene sequencing data, and hence, metagenomics needs to be involved for further investigation and functional analysis. Lastly, we only identified associations between e-PHLF and the gut microbiome, without providing direct causal evidence. Therefore, research in isolation and cultivation strains as well as animal models will be beneficial to elucidate the mechanism of intestinal bacteria in e-PHLF.
Conclusions
In this study, we analyzed the changes in the composition and diversity of gut bacteria in B-HCC patients with extended hepatectomy, and identified specific microbiotas that could be used as diagnostic biomarkers for e-PHLF. Overall, our data might assist in the development of novel strategies for minimizing the occurrence of e-PHLF.
Acknowledgments
Funding: The study was supported by National Nature Science Foundation of China (Grant/Award Nos. 81960534 and 81972306) and Key Laboratory of High-Incidence-Tumor Prevention & Treatment (Guangxi Medical University), Ministry of Education (No. GKE-ZZ202008).
Footnote
Reporting Checklist: The authors have completed the MDAR reporting checklist. https://atm.amegroups.com/article/view/10.21037/atm-22-1958/rc
Data Sharing Statement: Available at https://atm.amegroups.com/article/view/10.21037/atm-22-1958/dss
Conflicts of Interest: All authors have completed the ICMJE uniform disclosure form (available at https://atm.amegroups.com/article/view/10.21037/atm-22-1958/coif). The authors have no conflicts of interest to declare.
Ethical Statement: The authors are accountable for all aspects of the work in ensuring that questions related to the accuracy or integrity of any part of the work are appropriately investigated and resolved. The study was conducted in accordance with the Declaration of Helsinki (as revised in 2013). The study was approved by the Research Ethics Committee of Guangxi Medical University Cancer Hospital (No. KY2019009). All patients signed informed consent forms and agreed to their anthropometric data being used in the analysis.
Open Access Statement: This is an Open Access article distributed in accordance with the Creative Commons Attribution-NonCommercial-NoDerivs 4.0 International License (CC BY-NC-ND 4.0), which permits the non-commercial replication and distribution of the article with the strict proviso that no changes or edits are made and the original work is properly cited (including links to both the formal publication through the relevant DOI and the license). See: https://creativecommons.org/licenses/by-nc-nd/4.0/.
References
- Zhou K, Fountzilas C. Outcomes and Quality of Life of Systemic Therapy in Advanced Hepatocellular Carcinoma. Cancers (Basel) 2019;11:861. [Crossref] [PubMed]
- Liu J, Liu X, Ma J, et al. The clinical efficacy and safety of kanglaite adjuvant therapy in the treatment of advanced hepatocellular carcinoma: A PRISMA-compliant meta-analysis. Biosci Rep 2019;39:BSR20193319. [Crossref] [PubMed]
- Wu B, Zhou J, Ling G, et al. CalliSpheres drug-eluting beads versus lipiodol transarterial chemoembolization in the treatment of hepatocellular carcinoma: a short-term efficacy and safety study. World J Surg Oncol 2018;16:69. [Crossref] [PubMed]
- Zheng L, Liang P, Li J, et al. ShRNA-targeted COMMD7 suppresses hepatocellular carcinoma growth. PLoS One 2012;7:e45412. [Crossref] [PubMed]
- Yang XA, Lv F, Wang R, et al. Potential role of intestinal microflora in disease progression among patients with different stages of Hepatitis B. Gut Pathog 2020;12:50. [Crossref] [PubMed]
- He G, Chen Y, Zhu C, et al. Application of plasma circulating cell-free DNA detection to the molecular diagnosis of hepatocellular carcinoma. Am J Transl Res 2019;11:1428-45. [PubMed]
- Lu H, Zheng C, Liang B, et al. Mechanism and risk factors of nausea and vomiting after TACE: a retrospective analysis. BMC Cancer 2021;21:513. [Crossref] [PubMed]
- Cho Y, Kim JW, Kim JK, et al. Phase I Radiation Dose-Escalation Study to Investigate the Dose-Limiting Toxicity of Concurrent Intra-Arterial Chemotherapy for Unresectable Hepatocellular Carcinoma. Cancers (Basel) 2020;12:1612. [Crossref] [PubMed]
- Zhang X, Kang Z, Xie X, et al. Silencing of HIF-1α inhibited the expression of lncRNA NEAT1 to suppress development of hepatocellular carcinoma under hypoxia. Am J Transl Res 2020;12:3871-83. [PubMed]
- Peng Y, Leng W, Duan S, et al. Long noncoding RNA BLACAT1 is overexpressed in hepatocellular carcinoma and its downregulation suppressed cancer cell development through endogenously competing against hsa-miR-485-5p. Biomed Pharmacother 2019;116:109027. [Crossref] [PubMed]
- Yang PM, Lin LS, Liu TP. Sorafenib Inhibits Ribonucleotide Reductase Regulatory Subunit M2 (RRM2) in Hepatocellular Carcinoma Cells. Biomolecules 2020;10:117. [Crossref] [PubMed]
- Liu J, Wang Y, Tang Y, et al. Clinical effect and safety evaluation of hydromorphone combined with sufentanil in patient-controlled intravenous analgesia for patients with hepatocellular cancer and its effect on serum immune factors. Oncol Lett 2020;20:296. [Crossref] [PubMed]
- van Mierlo KM, Schaap FG, Dejong CH, et al. Liver resection for cancer: New developments in prediction, prevention and management of postresectional liver failure. J Hepatol 2016;65:1217-31. [Crossref] [PubMed]
- Zhang ZQ, Xiong L, Zhou JJ, et al. Ability of the ALBI grade to predict posthepatectomy liver failure and long-term survival after liver resection for different BCLC stages of HCC. World J Surg Oncol 2018;16:208. [Crossref] [PubMed]
- Ye JZ, Mai RY, Guo WX, et al. Nomogram for prediction of the international study Group of Liver Surgery (ISGLS) grade B/C Posthepatectomy liver failure in HBV-related hepatocellular carcinoma patients: an external validation and prospective application study. BMC Cancer 2020;20:1036. [Crossref] [PubMed]
- Wittauer EM, Oldhafer F, Augstein E, et al. Porcine model for the study of liver regeneration enhanced by non-invasive 13C-methacetin breath test (LiMAx test) and permanent portal venous access. PLoS One 2019;14:e0217488. [Crossref] [PubMed]
- Mitev K, Taleski V. Association between the Gut Microbiota and Obesity. Open Access Maced J Med Sci 2019;7:2050-6. [Crossref] [PubMed]
- Bian X, Yang L, Wu W, et al. Pediococcus pentosaceus LI05 alleviates DSS-induced colitis by modulating immunological profiles, the gut microbiota and short-chain fatty acid levels in a mouse model. Microb Biotechnol 2020;13:1228-44. [Crossref] [PubMed]
- Cani PD, Van Hul M, Lefort C, et al. Microbial regulation of organismal energy homeostasis. Nat Metab 2019;1:34-46. [Crossref] [PubMed]
- Hu H, Lin A, Kong M, et al. Intestinal microbiome and NAFLD: molecular insights and therapeutic perspectives. J Gastroenterol 2020;55:142-58. [Crossref] [PubMed]
- Ling JR, Zhang YJ, Zhang ZH, et al. Specific changes of intestinal microflora in children with nonalcoholic fatty liver disease. Zhonghua Er Ke Za Zhi 2018;56:850-5. [PubMed]
- Bajaj JS. Alcohol, liver disease and the gut microbiota. Nat Rev Gastroenterol Hepatol 2019;16:235-46. [Crossref] [PubMed]
- Yuan Y, Yuan H, Yang G, et al. IFN-α confers epigenetic regulation of HBV cccDNA minichromosome by modulating GCN5-mediated succinylation of histone H3K79 to clear HBV cccDNA. Clin Epigenetics 2020;12:135. [Crossref] [PubMed]
- Qin N, Yang F, Li A, et al. Alterations of the human gut microbiome in liver cirrhosis. Nature 2014;513:59-64. [Crossref] [PubMed]
- Yu LX, Schwabe RF. The gut microbiome and liver cancer: mechanisms and clinical translation. Nat Rev Gastroenterol Hepatol 2017;14:527-39. [Crossref] [PubMed]
- Micó-Carnero M, Rojano-Alfonso C, Álvarez-Mercado AI, et al. Effects of Gut Metabolites and Microbiota in Healthy and Marginal Livers Submitted to Surgery. Int J Mol Sci 2020;22:44. [Crossref] [PubMed]
- Cornide-Petronio ME, Álvarez-Mercado AI, Jiménez-Castro MB, et al. Current Knowledge about the Effect of Nutritional Status, Supplemented Nutrition Diet, and Gut Microbiota on Hepatic Ischemia-Reperfusion and Regeneration in Liver Surgery. Nutrients 2020;12:284. [Crossref] [PubMed]
- Annavajhala MK, Gomez-Simmonds A, Macesic N, et al. Colonizing multidrug-resistant bacteria and the longitudinal evolution of the intestinal microbiome after liver transplantation. Nat Commun 2019;10:4715. [Crossref] [PubMed]
- Liu HX, Keane R, Sheng L, et al. Implications of microbiota and bile acid in liver injury and regeneration. J Hepatol 2015;63:1502-10. [Crossref] [PubMed]
- Liu HX, Rocha CS, Dandekar S, et al. Functional analysis of the relationship between intestinal microbiota and the expression of hepatic genes and pathways during the course of liver regeneration. J Hepatol 2016;64:641-50. [Crossref] [PubMed]
- Qin T, Fu J, Verkade HJ. The role of the gut microbiome in graft fibrosis after pediatric liver transplantation. Hum Genet 2021;140:709-24. [Crossref] [PubMed]
- Acharya C, Bajaj JS. Chronic Liver Diseases and the Microbiome-Translating Our Knowledge of Gut Microbiota to Management of Chronic Liver Disease. Gastroenterology 2021;160:556-72. [Crossref] [PubMed]
- Rahbari NN, Garden OJ, Padbury R, et al. Posthepatectomy liver failure: a definition and grading by the International Study Group of Liver Surgery (ISGLS). Surgery 2011;149:713-24. [Crossref] [PubMed]
- Magoč T, Salzberg SL. FLASH: fast length adjustment of short reads to improve genome assemblies. Bioinformatics 2011;27:2957-63. [Crossref] [PubMed]
- Caporaso JG, Kuczynski J, Stombaugh J, et al. QIIME allows analysis of high-throughput community sequencing data. Nat Methods 2010;7:335-6. [Crossref] [PubMed]
- Wang Q, Garrity GM, Tiedje JM, et al. Naive Bayesian classifier for rapid assignment of rRNA sequences into the new bacterial taxonomy. Appl Environ Microbiol 2007;73:5261-7. [Crossref] [PubMed]
- Douglas GM, Maffei VJ, Zaneveld JR, et al. PICRUSt2 for prediction of metagenome functions. Nat Biotechnol 2020;38:685-8. [Crossref] [PubMed]
- Qiu T, Fu R, Ling W, et al. Comparison between preoperative two-dimensional shear wave elastography and indocyanine green clearance test for prediction of post-hepatectomy liver failure. Quant Imaging Med Surg 2021;11:1692-700. [Crossref] [PubMed]
- Peng W, Li JW, Zhang XY, et al. A novel model for predicting posthepatectomy liver failure in patients with hepatocellular carcinoma. PLoS One 2019;14:e0219219. [Crossref] [PubMed]
- Liu L, Wen L, Gao C, et al. Effects of Non-directional Mechanical Trauma on Gastrointestinal Tract Injury in Rats. Front Physiol 2021;12:649554. [Crossref] [PubMed]
- Dong R, Bai M, Zhao J, et al. A Comparative Study of the Gut Microbiota Associated With Immunoglobulin a Nephropathy and Membranous Nephropathy. Front Cell Infect Microbiol 2020;10:557368. [Crossref] [PubMed]
- Cornejo-Pareja I, Ruiz-Limón P, Gómez-Pérez AM, et al. Differential Microbial Pattern Description in Subjects with Autoimmune-Based Thyroid Diseases: A Pilot Study. J Pers Med 2020;10:192. [Crossref] [PubMed]
- Ulbrich T, Plogsted S, Geraghty ME, et al. Probiotics and prebiotics: why are they "bugging" us in the pharmacy? J Pediatr Pharmacol Ther 2009;14:17-24. [Crossref] [PubMed]
- Ke XH, Wang CG, Luo WZ, et al. Metabolomic Study to Determine the Mechanism Underlying the Effects of Sagittaria sagittifolia Polysaccharide on Isoniazid- and Rifampicin-Induced Hepatotoxicity in Mice. Molecules 2018;23:3087. [Crossref] [PubMed]
- Li WW, Shan JJ, Lin LL, et al. Disturbance in Plasma Metabolic Profile in Different Types of Human Cytomegalovirus-Induced Liver Injury in Infants. Sci Rep 2017;7:15696. [Crossref] [PubMed]
- Yang XX, Wei JD, Mu JK, et al. Integrated metabolomic profiling for analysis of antilipidemic effects of Polygonatum kingianum extract on dyslipidemia in rats. World J Gastroenterol 2018;24:5505-24. [Crossref] [PubMed]
- Gao D, Pang JY, Zhang CE, et al. Poria Attenuates Idiosyncratic Liver Injury Induced by Polygoni Multiflori Radix Praeparata. Front Pharmacol 2016;7:386. [Crossref] [PubMed]
- Lee HG, Kim HS, Oh JY, et al. Potential Antioxidant Properties of Enzymatic Hydrolysates from Stichopus japonicus against Hydrogen Peroxide-Induced Oxidative Stress. Antioxidants (Basel) 2021;10:110. [Crossref] [PubMed]
- Sakran M, Selim Y, Zidan N. A new isoflavonoid from seeds of Lepidium sativum L. and its protective effect on hepatotoxicity induced by paracetamol in male rats. Molecules 2014;19:15440-51. [Crossref] [PubMed]
- Zhang Y, Zhang H, Chang D, et al. Metabolomics approach by 1H NMR spectroscopy of serum reveals progression axes for asymptomatic hyperuricemia and gout. Arthritis Res Ther 2018;20:111. [Crossref] [PubMed]
- Brun T, Jiménez-Sánchez C, Madsen JGS, et al. AMPK Profiling in Rodent and Human Pancreatic Beta-Cells under Nutrient-Rich Metabolic Stress. Int J Mol Sci 2020;21:3982. [Crossref] [PubMed]
- Yoo HJ, Jung KJ, Kim M, et al. Liver Cirrhosis Patients Who Had Normal Liver Function Before Liver Cirrhosis Development Have the Altered Metabolic Profiles Before the Disease Occurrence Compared to Healthy Controls. Front Physiol 2019;10:1421. [Crossref] [PubMed]
- Diao W, Labaki WW, Han MK, et al. Disruption of histidine and energy homeostasis in chronic obstructive pulmonary disease. Int J Chron Obstruct Pulmon Dis 2019;14:2015-25. [Crossref] [PubMed]
- Xu L, Wang Y, Ma Z, et al. Urine Metabolomics Study on Potential Hepatoxic Biomarkers Identification in Rats Induced by Aurantio-Obtusin. Front Pharmacol 2020;11:1237. [Crossref] [PubMed]
- Enea M, Araújo AM, Almeida MP, et al. A Metabolomic Approach for the In Vivo Study of Gold Nanospheres and Nanostars after a Single-Dose Intravenous Administration to Wistar Rats. Nanomaterials (Basel) 2019;9:1606. [Crossref] [PubMed]
- Lourenço C, Kelly D, Cantillon J, et al. Monitoring type 2 diabetes from volatile faecal metabolome in Cushing's syndrome and single Afmid mouse models via a longitudinal study. Sci Rep 2019;9:18779. [Crossref] [PubMed]
- Wang J, Gu X, Yang J, et al. Gut Microbiota Dysbiosis and Increased Plasma LPS and TMAO Levels in Patients With Preeclampsia. Front Cell Infect Microbiol 2019;9:409. [Crossref] [PubMed]
- Wang L, Fu J, Li M, et al. Metabolomic and Proteomic Profiles Reveal the Dynamics of Primary Metabolism during Seed Development of Lotus (Nelumbo nucifera). Front Plant Sci 2016;7:750. [Crossref] [PubMed]
- Guo X, Li H, Xu H, et al. Palmitoleate induces hepatic steatosis but suppresses liver inflammatory response in mice. PLoS One 2012;7:e39286. [Crossref] [PubMed]
- Symmank J, Chorus M, Appel S, et al. Distinguish fatty acids impact survival, differentiation and cellular function of periodontal ligament fibroblasts. Sci Rep 2020;10:15706. [Crossref] [PubMed]
- Wang Y, Bi C, Pang W, et al. Plasma Metabolic Profiling Analysis of Gout Party on Acute Gout Arthritis Rats Based on UHPLC-Q-TOF/MS Combined with Multivariate Statistical Analysis. Int J Mol Sci 2019;20:5753. [Crossref] [PubMed]
- Li Y, Qi B. Progress toward Understanding Protein S-acylation: Prospective in Plants. Front Plant Sci 2017;8:346. [Crossref] [PubMed]
- Song JH, Jeong BK, Choi HS, et al. Defining Radiation-Induced Hepatic Toxicity in Hepatocellular Carcinoma Patients Treated with Stereotactic Body Radiotherapy. J Cancer 2017;8:4155-61. [Crossref] [PubMed]
- Duque-Correa MA, Karp NA, McCarthy C, et al. Exclusive dependence of IL-10Rα signalling on intestinal microbiota homeostasis and control of whipworm infection. PLoS Pathog 2019;15:e1007265. [Crossref] [PubMed]
- Lu WW, Fu TX, Wang Q, et al. The effect of total glucoside of paeony on gut microbiota in NOD mice with Sjgren's syndrome based on high-throughput sequencing of 16SrRNA gene. Chinese Medicine 2020;15:61. [Crossref] [PubMed]
- Prossomariti A, Scaioli E, Piazzi G, et al. Short-term treatment with eicosapentaenoic acid improves inflammation and affects colonic differentiation markers and microbiota in patients with ulcerative colitis. Sci Rep 2017;7:7458. [Crossref] [PubMed]
- Asai N, Koizumi Y, Yamada A, et al. Pantoea dispersa bacteremia in an immunocompetent patient: a case report and review of the literature. J Med Case Rep 2019;13:33. [Crossref] [PubMed]
- Zhuang H, Cheng L, Wang Y, et al. Dysbiosis of the Gut Microbiome in Lung Cancer. Front Cell Infect Microbiol 2019;9:112. [Crossref] [PubMed]
- Soliman AR, Ahmed RM, Abdalla A, et al. Impact of Enterobacteriaceae bacteremia on survival in patients with hepatorenal failure. Saudi J Kidney Dis Transpl 2018;29:1311-9. [Crossref] [PubMed]
- Cai TT, Ye XL, Li RR, et al. Resveratrol Modulates the Gut Microbiota and Inflammation to Protect Against Diabetic Nephropathy in Mice. Front Pharmacol 2020;11:1249. [Crossref] [PubMed]
(English Language Editor: A. Kassem)