Bioinformatic analyses of the role of m6A RNA methylation regulators in abdominal aortic aneurysm
Introduction
Abdominal aortic aneurysm (AAA) is a permanent focal dilation of the abdominal aorta, and aorta aneurysm rupture has an incidence of approximately 80% (1-3). Ruptured abdominal aortic aneurysm (rAAA) is responsible for approximately 200,000 deaths annually (1,4). As an inflammatory and chronic vascular degenerative disease, AAA is characterized by remodeling and progressive dilation of the vessel wall (2,5-7). Lymphocytes and macrophages infiltrate the vascular wall, proteases destroy elastin and collagen in the middle and outer membrane, and there is smooth muscle cell apoptosis (8,9). Inflammatory cells activate and secrete various cytokines and chemokines to induce activation of matrix metalloproteinase, resulting in extracellular matrix degradation and smooth muscle cell apoptosis, leading to the formation and rupture of AAA (7,10-12). AAA usually develops asymptomatically and unpredictably in normal conditions until its rupture. Moreover, elective stable abdominal aortic aneurysms (eAAAs) can be fatal. In spite of extensive literature on the pathogenesis, evolution, and rupture of AAA (13-15), prevention or suppression of AAA in a clinical setting still remains an unanswered question.
It is well known that epigenetic regulation of gene expression directs and maintains cellular phenotypes (16,17). N6-methyladenosine (m6A) is the most abundant and reversible internal epigenetic modification in eukaryotic organisms (18,19). In the past few years, m6A modification of messenger RNA (mRNA) or non-coding RNA has been reported to play a vital role in almost all major normal biological processes, including the self-renewal and differentiation of embryonic stem cells, tissue development, response to heat shock or DNA damage, biological clock regulation, spermatogenesis, and transition between maternal and zygote (20,21). Using the “limma” R package (22), Li et al. noted low expression of METTL14 and HNRNPC, and reported that RBM15B was the upregulated methylation transferase used in human AAA. They also found that METTL14, HNRNPC, and RBM15B levels were correlated with immune infiltration (23). We hypothesized that m6A modification may play an important role in AAA. Nonetheless, the roles of m6A regulators in AAA unknown. Therefore, there is a pressing need to identify m6A RNA methylation regulators in the diagnosis of AAA, determination of individualized risk, discovery of therapeutic targets, and improve understanding of pathogenesis.
In this study, we firstly detected the m6A levels of total RNAs in AAA tissues and normal abdominal aortas using a m6A quantitative kit, and found high m6A levels in AAA. Furthermore, compared with eAAA, the m6A level in rAAA was upregulated. We compared the predictive performance of the support vector machine (SVM) and the random forest (RF) methods, which illustrated that RF has a substantially high accuracy in predicting the probability of AAA rupture. Moreover, we assessed the significance of differentially-expressed m6A methylation regulators in immune cell infiltration. We present the following article in accordance with the TRIPOD reporting checklist (available at https://atm.amegroups.com/article/view/10.21037/atm-22-1891/rc).
Materials
Data access
The GSE98278 dataset containing 31 eAAAs and 17 rAAAs patients were obtained from the Gene Expression Omnibus (GEO) database (https://www.ncbi.nlm.nih.gov/geo/). Twenty-one m6A methylation regulators were extracted from multiple literatures to identify different m6A modification patterns mediated by 21 m6A regulators. These regulators consisted of two erasers (ALKBH5 and FTO), 11 readers (YTHDC1, FMR1, ELAVL1, YTHDF1, IGF2BP1, YTHDC2, YTHDF2, HNRNPA2B1, YTHDF3, LRPPRC, and HNRNPC), and eight writers (RBM15, RBM15B, CBLL1, WTAP, METTL3, ZC3H13, METTL14, and KIAA1429).
The feature genes were screened based on the RF analysis
A flowchart for the data processing as in Figure S1. Differentially-expressed m6A methylation regulators between eAAA and rAAA were screened using the “limma” R package, and the criterion for screening differential genes was P<0.05. Pearson correlation coefficients were used to analyze differential m6A methylation regulators (r>0.3, P<0.05). The SVM and RF methods were adopted to construct a training model to predict the occurrence of rAAA. We used “Boxplots of residual”, “Reverse cumulative distribution of residual”, and a receiver operating characteristic (ROC) curve to evaluate the accuracy of the model. The RF method was used to differentially express m6A methylation regulators to predict the occurrence of rAAA, and the calculations were performed using the R library “randomForest”. In this study, mtry and ntree were set to 3 and 500, respectively. The optimal ntree was selected according to minimum cross-validation error in 10-fold cross-validation. We assessed the significance of differentially-expressed m6A methylation regulators with the optimal ntree. Nomogram construction was performed using the “rms” package. The consistency between the actual observed values and the predicted values was evaluated using calibration curves. Finally, we conducted a clinical impact curve and decision curve analysis to evaluate the clinical benefit of our model.
Kyoto Encyclopedia of Genes and Genomes (KEGG) and Gene Ontology (GO) pathway enrichment analyses
Differentially-expressed genes between eAAA and rAAA were screened using the “limma” package in R, and the criterion for screening differential genes was P<0.05. Meanwhile, KEGG pathway and GO enrichment analyses were performed using the R package, including “clusterProfiler”, “org.Hs.eg.db”, “enrichplot”, “ggplot2”, “RColorBrewer”, “dplyr”, and “ComplexHeatmap”.
m6A cluster analysis and estimation of immune cell infiltration
Using the “ConsensusClusterPlus” R package, consensus clustering was used to identify m6A clusters based on m6A methylation regulators. The single sample gene set enrichment analysis (ssGSEA) method was then used to evaluate the abundance of 23 immune cells in AAA to further investigate the correlation, and a bar plot was constructed to visualize the features.
Cell culture and transfection
Human aortic smooth muscle cells (HASMCs) from The First Hospital of China Medical University were isolated by an enzyme digestion method from the human aorta and cultured. The study was conducted in accordance with the Declaration of Helsinki (as revised in 2013). The present study was approved by the Ethics Committee of The First Hospital of China Medical University (No. AF-SOP-07-1.1-01), and informed consent was taken from all the patients. HASMCs were cultured in a smooth muscle cell growth medium (SMCM), which included smooth muscle basal medium, penicillin and streptomycin (1%), Fetal bovine serum (FBS) (5%), and smooth muscle cell growth supplement (1%), at 37 ℃ in 5% carbon dioxide (CO2). HASMCs were seeded into six-well plates (1×105 cells) and then transfected with small interfering Ribonucleic Acid (siRNA). The siRNA sequence was as follows: 5'-GGUGAUAGUUGGGCAUAUA-3'.
m6A quantification
For m6A quantitation, the change in global m6A mRNA levels was measured using a m6A RNA Methylation Quantification Kit (Colorimetric, Epigentek, USA). Briefly, total RNA was extracted from AAA samples with an RNA extraction kit (TIANGEN, Beijing, China). Next, the RNA was incubated with m6A antibody in wells, and m6A levels were measured at 450 nm using a microplate reader (BioTek microplate reader, Colmar, France).
Protein extraction and Western blot analysis
A total protein extraction kit (KeyGen Biotech, Nanjing, China) was used for total protein extraction, and a bicinchoninic acid (BCA) Protein Assay Kit (KeyGen Biotech, Nanjing, China) was used for total protein quantification. Total protein was subjected to SDS-polyacrylamide gel electrophoresis (SDS-PAGE) and Western blot analysis. Primary antibodies were incubated overnight at 4 ℃. The following antibodies were used: RBM15, Caspase-3, and Actin (Cell Signaling Technology, MA, USA). Membranes were washed three times in Tris-buffered saline containing 0.05% Tween-20 (TBST) buffer and incubated with the appropriate secondary antibodies (Cell Signaling Technology, MA, USA) for 2h. The chemiluminescence method was used to detect protein bands.
Quantitative real-time polymerase chain reaction (RT-qPCR)
Total RNA was extracted using the Total RNA Extraction Kit (Solarbio, Beijing, China) according to the manufacturer’s instructions. The RNA was reverse-transcribed using the TaKaRa RNA PCR kit (TaKaRa, Dalian, China). SYBR Premix Ex Taq (TaKaRa, Dalian, China) was used for the RT-qPCR experiments. RT-qPCR was performed using an ABS 7500 Realtime PCR System (Thermo Fisher, Waltham, MA, USA) according to the manufacturer’s protocol. The qPCR primer sequences are listed in Table S1.
Terminal deoxynucleotidyl transferase mediated dUTP nick-end labeling (TUNEL) staining assay
The apoptotic cells were detected according to the TUNEL apoptosis assay kit instructions (in situ cell death detection kit-POD system, Roche, USA). HASMCs were seeded into six-well plates (1×105 cells) with 100 µm hydrogen peroxide (H2O2) treatment, and then fixed, permeabilized, and incubated with the TUNEL reagent.
RNA immunoprecipitation (RIP) assay
A RIP assay was carried out using the Magna RIP RNA-binding protein immunoprecipitation kit (Millipore, USA) according to the manufacturer’s protocol. Briefly, total RNA was extracted using the total RNA extraction kit (Solarbio, Beijing, China) according to the manufacturer’s instructions. Fragmented RNA was incubated with an antibody. The beads were washed with RIP buffer, followed by RNA purification and qRT-PCR analysis.
Methylated RNA immunoprecipitation-quantitative polymerase chain reaction (MeRIP-qPCR)
The m6A modifications of individual genes were detected using a MeRIP-qPCR assay according to the manufacturer’s instructions (Millipore, Germany). We used purified RNA incubated with m6A antibody in immunoprecipitation buffer containing RNasin Plus RNase inhibitor (Promega, Madison WI, USA). Precipitated RNA was reverse-transcribed and quantified by qPCR for m6A enrichment.
Statistical analysis
Linear regression analysis and the Pearson correlation coefficient (r) were used to determine the correlation between the gene expression levels. Non-parametric one-way analysis of variance (ANOVA) was utilized to compare variables between different groups. Two-tailed t-tests were used for all analyses comparing two groups. All statistical analysis was performed in R (Version 4.1.0). Significant significance was found when the p-values were less than 0.05.
Results
Relationship between m6A RNA methylation and rAAA
To elucidate the m6A methylation changes associated with rAAA, a differential m6A methylation regulator expression analysis was performed. The GSE98278 dataset was downloaded from the GEO database. We analyzed differentially-expressed m6A methylation regulators in the eAAA (n=31) and rAAA (n=17), and a heat map was constructed to display the expression of differential genes (Figure 1A). Specifically, RBM15, WTAP, ALKBH5, and IGFBP3 were highly expressed in eAAA. In contrast, RBM15B showed opposite results (Figure 1B). Correlation analysis also revealed a positive correlation between MTTL3 and FTO expression levels based on the GSE98278 dataset (Figure 1C). The m6A levels of total RNAs in AAA tissues and normal abdominal aortas were detected using a m6A quantitative kit showing the high m6A levels in AAA. Moreover, the m6A level in rAAA was upregulated compared with that in eAAA (Figure 1D). The changes in m6A methylation regulator expression in rAAA were validated by qRT-PCR (Figure 1E). Taken together, a high m6A modification methylation level was related to rAAA.
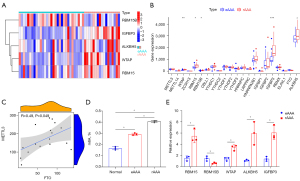
The SVM and RF methods were used to construct the rAAA predictive models
We compared the prediction performance of the SVM and RF methods. “Boxplots of residual” (Figure 2A), “reverse cumulative distribution of residual” (Figure 2B), and a ROC curve (Figure 2C) showed that RF has substantially high prediction accuracy. All of the samples in the model had minimum residuals, indicating that the model is optimal. In this study, mtry and ntree were set to 3 and 500, respectively. The optimal ntree was selected according to the minimum cross-validation error in 10-fold cross-validation (Figure 2D). After ranking the genes according to their importance, we identified the five m6A methylation regulators (Figure 2E).
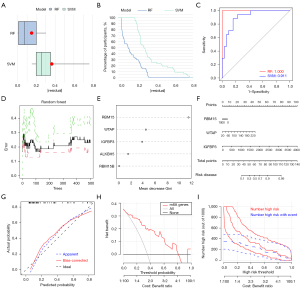
The nomogram evaluation model was constructed based on three m6A methylation regulators to predict the probability of AAA rupture (Figure 2F). The calibration curves showed that the nomogram model may be an ideal predictive model for rAAA (Figure 2G). Decision curve analysis (DCA) and clinical impact plots were used to determine the clinical utility of the risk prediction nomograms (Figure 2H,2I). The nomogram showed remarkable predictive power for rAAA.
GO and KEGG pathway enrichment analyses
To further understand the differences between eAAA and rAAA, we screened the differential genes from the GSE98278 dataset. A total of 2,899 differential genes were screened using the “limma” package in R and displayed via a heat map (Figure 3A).
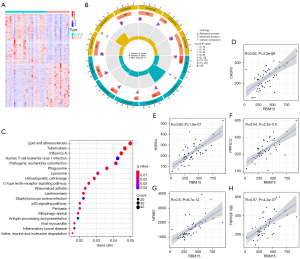
Meanwhile, the GO and KEGG pathway enrichment analyses of differential genes were also performed. The top 12 GO results based on the P value are shown in Figure 3B. KEGG pathway enrichment analysis showed that the most significantly enriched signaling pathway was the lipid and atherosclerosis signaling pathway (Figure 3C). The previous result showed that RBM15 is the most crucial m6A methylation regulator in rAAA (Figure 2E). Correlation analysis was carried out to explore further the relationship between RBM15 and the lipid and atherosclerosis signaling pathway-related genes. A total of 22 genes were found to be positively correlated with RBM15 expression, and the most significant were CASP3, HSPA4, NFKB1, PPP3CC, and TNFRSF10B (Figure 3D-3H).
RBM15 regulates the expression of CASP3 in an m6A-dependent manner
To further explore the role of RBM15 in AAA, the expression of RBM15 was measured in eAAA and rAAA by Western blotting. RBM15 was found to be more highly expressed in rAAA compared with eAAA (Figure 4A). Next, we examined the effects of RBM15 knockdown on CASP3, HSPA4, NFKB1, PPP3CC, and TNFRSF10B expression in HASMCs. The knockdown efficiency of RBM15 was assessed by Western blotting (Figure 4B). RT-qPCR and Western blotting showed that the mRNA level of CASP3 was decreased upon RBM15 knockdown of HASMCs (Figure 4C,4D).
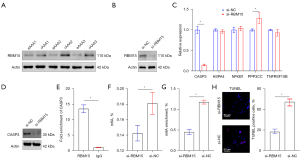
Furthermore, we investigated the regulatory mechanism of CASP3 expression by RBM15. The RIP assay revealed an interaction between RBM15 and mRNA-CASP3 (Figure 4E). In addition, we wondered whether RBM15 could affect m6A levels, and detected m6A levels after RBM15 knockdown. RBM15 knockdown significantly decreased the m6A level (Figure 4F). We also measured the m6A levels of CASP3 by MeRIP-qPCR in HASMCs, which showed that m6A modification of CASP3 mRNA decreased relative to the normal control (NC) group (Figure 4G).
We then examined the effects of RBM15 knockdown on apoptosis by TUNEL staining. The result showed that RBM15 knockdown inhibited apoptosis (Figure 4H). As mentioned above, RBM15 knockdown decreased the expression of CASP3 in an m6A-dependent manner and inhibited HASMC apoptosis.
Three distinct m6A clusters were identified and immune cell infiltration
Consensus clustering was performed using the “ConsensusClusterPlus” R package, which identified three m6A clusters (m6Acluster.A, m6Acluster.B, and m6Acluster.C) based on the five m6A methylation regulators (Figure 5A). The heat map showing the differential gene expression in three m6A clusters is displayed in Figure 5B. Principal component analysis (PCA) was used to verify the three distinct m6A clusters divided by consensus clustering of the five m6A RNA methylation regulators (Figure 5C). Moreover, a significant difference in ALKBH5 expression was identified between the three m6A clusters (Figure 5D).
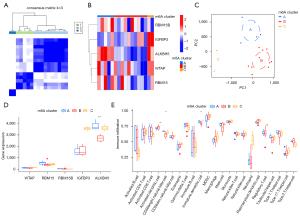
Given that AAA has been shown to be infiltrated with a large number of immune cells, we further analyzed the infiltrating immune cells between the three m6A clusters. A significant difference was found in cluster difference 56 (CD56) bright natural killer cells and immature dendritic cells between the different m6A clusters (Figure 5E). Moreover, we analyzed the relationship between the expression of the five m6A methylation regulators and immune cell infiltration using the ssGSEA method (Figure 6A). The results were as follows: (I) ALKBH5 was positively correlated with CD56 bright natural killer cells and T follicular helper cells (Figure 6B); (II) RBM15 was negatively correlated with macrophages (Figure 6C); (III) RBM15B was positively correlated with monocytes and type 1 T helper cells (Figure 6D); (IV) WTAP was positively correlated with type 17 T helper cells (Figure 6E); and (V) IGFBP3 levels were not statistically significantly correlated with immune cell infiltration (Figure 6F).
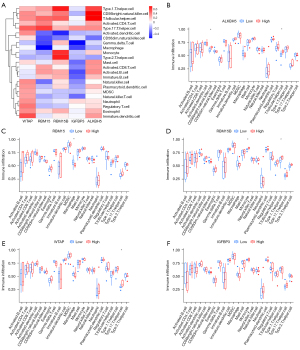
Discussion
AAA is a degenerative disease that may lead to aortic rupture (2,5). m6A modification is the most ubiquitous mRNA modification in eukaryotes, and the role of m6A modification has been well defined in mRNA (18,21,23). Reversible m6A modification regulates a series of biological progressions, such as RNA stability, alternative splicing, and mRNA translation (19). Abnormal expression of m6A modified protein or m6A binding protein or abnormal m6A modified level can lead to abnormal metabolism of m6A-modified related RNA and affect gene expression, which is closely related to the occurrence and development of many diseases (18,19,23,24). He et al. reported a significantly high m6A level in AAA tissues compared to normal aortic tissues, and high m6A levels are correlated with a greater risk of AAA rupture (25).
Similarly, we confirmed the high m6A level in eAAA, and the m6A level in rAAA was further upregulated compared with eAAA. In contrast to He et al., who used a multivariable model to screen risk genes, the SVM and RF Methods were applied in this study (25). RF exhibited substantially high prediction accuracy compared to “Boxplots of residual”, Reverse cumulative distribution of residual”, and the ROC curve. We compared the predictive performance of the SVM and RF. As a result, we identified the five m6A methylation regulators after ranking these genes according to their importance by RF. RBM15 is the most crucial gene.
The five m6A methylation regulators expression in rAAA were validated by RT-PCR, and RBM15 was found to be highly expressed in rAAA compared with eAAA by Western blotting. These results indicated a high m6A modification methylation level was related to rAAA. Due to the small sample size, further research is needed. Thus, we finally selected RBM15 for further analysis in this study. RBM15 is a member of the split-end (SPEN) family of proteins, which can bind to RNA by interacting with spliceosome components (26,27). RBM15/RBM15B recruit the WTAP-METTL3-METTLE14 RNA methyltransferase complex to install m6A (28). To further explore the role of RBM15 in AAA, RBM15 was depleted by siRNA-mediated knockdown. We found that RBM15 knockdown inhibited apoptosis in HASMCs.
Meanwhile, GO and KEGG pathway enrichment analyses of differential genes was performed based on the GSE98278 dataset, and the lipid and atherosclerosis signaling pathway was the most significant. Therefore, the relationship between RBM15 and the lipid and atherosclerosis signaling pathway was further investigated. A total of 22 genes were positively correlated with RBM15 expression, and the most significant were CASP3, HSPA4, NFKB1, PPP3CC, and TNFRSF10B.
Moreover, the mRNA level of CASP3 was decreased upon RBM15 knockdown of HASMCs. Mechanically, RBM15 knockdown decreased the expression of CASP3 in an m6A-dependent manner by RIP and MeRIP-qPCR. Furthermore, consensus clustering identified three m6A clusters based on the five m6A methylation regulators. Consider that AAA has been shown to be infiltrated with a large number of immune cells, we further analyzed the infiltrating immune cells between the three m6A clusters. A significant difference was observed in CD56 bright natural killer cells and immature dendritic cells between the different m6A clusters. Moreover, we determined the relationship between the expression of the five m6A methylation regulators and immune cell infiltration by the ssGSEA method.
Taken together, m6A plays a significant role in eAAA. RBM15 knockdown decreased the expression of CASP3 in an m6A-dependent manner and inhibited HASMC apoptosis. A strong correlation between the five m6A methylation regulators and immune cell infiltration was identified.
Conclusions
In this study, we observed a significantly higher m6A level in AAA tissues compared to normal aortic tissues, and high m6A levels increase the risk of AAA rupture. After ranking these genes according to their importance by RF, we identified five m6A methylation regulators, of which RBM15 was the most crucial factor. RBM15 knockdown decreased the expression of CASP3 in an m6A-dependent manner and inhibited HASMC apoptosis. Therefore, m6A may be a potential therapeutic target for inhibiting AAA rupture.
Acknowledgments
We are very grateful to all of the participants in the present research. We also thank the reviewers for their valuable advice.
Funding: None.
Footnote
Reporting Checklist: The authors have completed the TRIPOD reporting checklist. Available at https://atm.amegroups.com/article/view/10.21037/atm-22-1891/rc
Data Sharing Statement: Available at https://atm.amegroups.com/article/view/10.21037/atm-22-1891/dss
Conflicts of Interest: All authors have completed the ICMJE uniform disclosure form (available at https://atm.amegroups.com/article/view/10.21037/atm-22-1891/coif). The authors have no conflicts of interest to declare.
Ethical Statement: The authors are accountable for all aspects of the work in ensuring that questions related to the accuracy or integrity of any part of the work are appropriately investigated and resolved. The study was conducted in accordance with the Declaration of Helsinki (as revised in 2013). The present study was approved by the Ethics Committee of The First Hospital of China Medical University (No. AF-SOP-07-1.1-01), and informed consent was taken from all the patients.
Open Access Statement: This is an Open Access article distributed in accordance with the Creative Commons Attribution-NonCommercial-NoDerivs 4.0 International License (CC BY-NC-ND 4.0), which permits the non-commercial replication and distribution of the article with the strict proviso that no changes or edits are made and the original work is properly cited (including links to both the formal publication through the relevant DOI and the license). See: https://creativecommons.org/licenses/by-nc-nd/4.0/.
References
- Schanzer A, Oderich GS. Management of Abdominal Aortic Aneurysms. N Engl J Med 2021;385:1690-8. [Crossref] [PubMed]
- Golledge J. Abdominal aortic aneurysm: update on pathogenesis and medical treatments. Nat Rev Cardiol 2019;16:225-42. [Crossref] [PubMed]
- Ayoub C, Murad MH. Population-based screening for vascular disease. Lancet 2017;390:2218-20. [Crossref] [PubMed]
- O'Donnell TFX, Landon BE, Schermerhorn ML. AAA Screening Should Be Expanded. Circulation 2019;140:889-90. [Crossref] [PubMed]
- Sakalihasan N, Michel JB, Katsargyris A, et al. Abdominal aortic aneurysms. Nat Rev Dis Primers 2018;4:34. [Crossref] [PubMed]
- Verstraeten A, Luyckx I, Loeys B. Aetiology and management of hereditary aortopathy. Nat Rev Cardiol 2017;14:197-208. [Crossref] [PubMed]
- Martinez-Gonzalez J, Canes L, Alonso J, et al. NR4A3: A Key Nuclear Receptor in Vascular Biology, Cardiovascular Remodeling, and Beyond. Int J Mol Sci 2021;22:11371. [Crossref] [PubMed]
- Raffort J, Lareyre F, Clement M, et al. Monocytes and macrophages in abdominal aortic aneurysm. Nat Rev Cardiol 2017;14:457-71. [Crossref] [PubMed]
- Rombouts KB, van Merrienboer TAR, Ket JCF, et al. The role of vascular smooth muscle cells in the development of aortic aneurysms and dissections. Eur J Clin Invest 2022;52:e13697. [Crossref] [PubMed]
- Meng X, Yang J, Dong M, et al. Regulatory T cells in cardiovascular diseases. Nat Rev Cardiol 2016;13:167-79. [Crossref] [PubMed]
- Harrison SC, Smith AJ, Jones GT, et al. Interleukin-6 receptor pathways in abdominal aortic aneurysm. Eur Heart J 2013;34:3707-16. [Crossref] [PubMed]
- Yuan Z, Lu Y, Wei J, et al. Abdominal Aortic Aneurysm: Roles of Inflammatory Cells. Front Immunol 2021;11:609161. [Crossref] [PubMed]
- Kessler V, Klopf J, Eilenberg W, et al. AAA Revisited: A Comprehensive Review of Risk Factors, Management, and Hallmarks of Pathogenesis. Biomedicines 2022;10:94. [Crossref] [PubMed]
- Qian G, Adeyanju O, Olajuyin A, et al. Abdominal Aortic Aneurysm Formation with a Focus on Vascular Smooth Muscle Cells. Life (Basel) 2022;12:191. [Crossref] [PubMed]
- Wu JQ, Wang W, Zheng YH. Role of Vascular Aging in the Pathogenesis of Abdominal Aortic Aneurysm and Potential Therapeutic Targets. Zhongguo Yi Xue Ke Xue Yuan Xue Bao 2021;43:962-8. [PubMed]
- Farina FM, Serio S, Hall IF, et al. The epigenetic enzyme DOT1L orchestrates vascular smooth muscle cell-monocyte crosstalk and protects against atherosclerosis via the NF-kappaB pathway. Eur Heart J 2022; [Epub ahead of print]. [Crossref] [PubMed]
- Skourti E, Dhillon P. Cancer epigenetics: promises and pitfalls for cancer therapy. FEBS J 2022;289:1156-9. [Crossref] [PubMed]
- Peng L, Long T, Li F, et al. Emerging role of m(6) A modification in cardiovascular diseases. Cell Biol Int 2022;46:711-22. [Crossref] [PubMed]
- Yang Y, Hsu PJ, Chen YS, et al. Dynamic transcriptomic m(6)A decoration: writers, erasers, readers and functions in RNA metabolism. Cell Res 2018;28:616-24. [Crossref] [PubMed]
- Deng X, Su R, Weng H, et al. RNA N(6)-methyladenosine modification in cancers: current status and perspectives. Cell Res 2018;28:507-17. [Crossref] [PubMed]
- Lan Y, Liu B, Guo H. The role of M(6)A modification in the regulation of tumor-related lncRNAs. Mol Ther Nucleic Acids 2021;24:768-79. [Crossref] [PubMed]
- Ritchie ME, Phipson B, Wu D, et al. limma powers differential expression analyses for RNA-sequencing and microarray studies. Nucleic Acids Res 2015;43:e47. [Crossref] [PubMed]
- Li T, Wang T, Jing J, et al. Expression Pattern and Clinical Value of Key m6A RNA Modification Regulators in Abdominal Aortic Aneurysm. J Inflamm Res 2021;14:4245-58. [Crossref] [PubMed]
- Jiang X, Liu B, Nie Z, et al. The role of m6A modification in the biological functions and diseases. Signal Transduct Target Ther 2021;6:74. [Crossref] [PubMed]
- He Y, Xing J, Wang S, et al. Increased m6A methylation level is associated with the progression of human abdominal aortic aneurysm. Ann Transl Med 2019;7:797. [Crossref] [PubMed]
- Hu M, Yang Y, Ji Z, et al. RBM15 Functions in Blood Diseases. Curr Cancer Drug Targets 2016;16:579-85. [Crossref] [PubMed]
- Dai XY, Shi L, Li Z, et al. Main N6-Methyladenosine Readers: YTH Family Proteins in Cancers. Front Oncol 2021;11:635329. [Crossref] [PubMed]
- Brockdorff N, Bowness JS, Wei G. Progress toward understanding chromosome silencing by Xist RNA. Genes Dev 2020;34:733-44. [Crossref] [PubMed]
(English Language Editor: A. Kassem)