Identifying biomarkers associated with tumor growth rate: a longitudinal preoperative magnetic resonance imaging follow-up of low-grade gliomas
Introduction
Low-grade gliomas (LGGs) are brain tumors that generally grow linearly and infiltrate diffusely (1,2). Although most LGGs are indolent, their clinical behavior is highly variable and cannot be adequately predicted based on their histologic class (3). Molecular examination has become an important part of glioma diagnosis, and many diffuse astrocytomas with wild-type isocitrate dehydrogenase (IDH)—previously considered low-grade tumors—which may be more likely to progress aggressively, are now classified as World Health Organization (WHO) grade 4 tumors (4). Although complete neurosurgical resection of LGGs is sometimes impossible, maximum safe resection remains the goal of management of LGG, which also involves clinical monitoring, chemotherapy, and radiotherapy, and depends on the histological type and molecular subtype of the tumor and the clinical status of the patient (5).
The tumor growth rate directly reflects the biological characteristics of gliomas during their natural growth process. Fast-growing gliomas should be treated immediately, and timely examinations should be performed after surgery. Radius or diameter measurements of LGGs reveal linear growth, and the velocity of diameter expansion (VDE) is generally used as a quantitative indicator of the tumor growth rate (1,2,6). The VDE is an independent prognostic factor in patients with gliomas (7-9) and has been used to assess the effectiveness of treatments such as chemotherapy (10-12) and radiotherapy (11,13). The VDE is also considered a quantitative indicator of tumor malignancy (7,8). Compared to patient survival time, VDE better reflects the characteristics and invasiveness of the tumor itself, as it prevents clinical treatment factors, such as the extent of resection or any adjuvant therapy, from influencing the results obtained (14,15).
Molecular biomarkers play an important role in determining the biological behavior of gliomas and the survival time of patients (5,16). Mutant p53 protein overexpression has been shown to accelerate LGG growth rates (8), while 1p/19q codeletion has been shown to significantly decelerate LGG growth rates (9,10,14). In contrast, IDH1 variations are considered to have no significant influence on tumor growth (8,9,17). Although the influence of molecular biomarkers on the biological behavior of tumor cells has been investigated (18-21), their quantitative influence on the velocity of tumor growth remains unclear.
Only a few longitudinal studies have obtained multiple magnetic resonance (MR) scans of tumors prior to surgery (1,2,8-10,12-14,22-24). Hence, the factors that influence the natural development of LGGs are still unclear. Additionally, inconsistent results regarding the growth rate of LGGs have been reported. The present study systematically investigated the effects of molecular biomarker status on the natural growth rate of gliomas. We analyzed the following molecular biomarkers that determine the classification of adult diffuse glioma in the 2021 WHO classification of tumors of the central nervous system (4) and those recommended by the Chinese Glioma Cooperative Group (CGCG) guidelines on tumor growth, since they help define the biological and clinical behavior of gliomas (5): alpha-thalassemia/mental retardation, X-linked (ATRX), telomerase reverse transcriptase (TERT), epidermal growth factor receptor (EGFR), mutant p53, Ki-67, O6-methylguanine-DNA methyltransferase (MGMT), and 1p/19q. We present the following article in accordance with the REMARK reporting checklist (available at https://atm.amegroups.com/article/view/10.21037/atm-21-3998/rc).
Methods
Patient selection
Clinical information and imaging data from patients who underwent primary surgical treatment between January 2008 and October 2021 and were pathologically diagnosed with LGGs were retrospectively reviewed (Figure 1). The patient inclusion criteria were as follows: (I) diagnosis at age ≥18 years; (II) 2 or more magnetic resonance imaging (MRI) examinations before surgery; (III) no chemotherapy or radiotherapy received prior to surgery; and (IV) histologically confirmed WHO grade II glioma. Due to the value of the patient’s IDH status in diagnosing LGGs, patients with an IDH wild-type tumor or with no recorded IDH status were excluded. To avoid bias, patients for whom sequential MRIs were performed at intervals of less than 90 days were excluded from this study. A total of 56 patients with LGGs were finally included. The study was conducted in accordance with the Declaration of Helsinki (as revised in 2013). The study was approved by the ethics committee of Beijing Tiantan Hospital (No. KYSB2016-026) and as a retrospective study, all clinical information was collected from the institutional medical database, therefore individual consent for this retrospective analysis was waived.
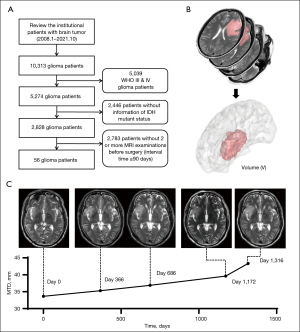
MRI data acquisition
A Magnetom Trio 3T scanner (Siemens AG, Munich, Germany) was used to acquire all MRI scans. The T2-weighted imaging parameters were as follows: repetition time =5,800 ms; echo time =110 ms; flip angle =150 degrees; 24 slices; field of view =240×188 mm2; and voxel size =0.6×0.6×5 mm3. The brain lesions of each patient were manually segmented by 2 board-certified neurosurgeons using the free access software MRIcro (http://www.mccauslandcenter.sc.edu/mricro/) and re-evaluated by a neuroradiologist with over 20 years of experience in tumor diagnosis. When there was a discrepancy exceeding 5% between the neurosurgeons, a senior neuroradiologist determined the lesion border (25).
Assessment of molecular biomarkers
The expression levels of ATRX, EGFR, mutant p53, and Ki-67 were examined in tumor tissue by immunohistochemistry (IHC), following the procedure introduced in our previous studies (26-28). Staining was scored on a 5-point scale from 0 to 4, where 0 = no or rare occurrence of staining; 1 = 10% of cells stained positively; 2 = 10–30% of cells stained positively; 3 = 30–60% of cells stained positively; and 4 = 60% or more of cells stained positively. Following our previous study (29), IDH variations were identified using DNA pyrosequencing, TERT promoter variational status was determined using Sanger sequencing, and 1p/19q codeletion status was detected by fluorescence in situ hybridization (FISH). The MGMT promoter methylation status was determined by methylation-specific polymerase chain reaction (PCR) after sodium bisulfite DNA modification, as described previously (30). A dichotomous classification method was used to obtain the subgroups for each biomarker according to the different expression levels or variation statuses. The final cutoff of expression levels of each biomarker examined by IHC is shown in Table S1.
Tumor growth model
Most studies quantitatively measuring tumor growth rates have used a linear growth model (1,8-10,12-14,23,24). Since it is not affected by the initial volume, a linear model can intuitively observe the differences in growth rates between different subgroups. Therefore, we chose the linear growth model to analyze the influence of different factors on tumor growth rates. Changes in tumor size are represented by the change in mean tumor diameter [MTD; ] (15) over time, and the tumor volume (V) was calculated using MATLAB (version 2014a, The MathWorks, Natick, MA, USA) based on the voxels of the segmented tumor region on T2-weighted images (Figure 1). The VDE was estimated using linear regression for diameter expansion for each case. To examine the overall tumor growth trend of the dataset, we used an improved linear mixed-effects model (LMEM) with a fixed slope unlike that used in previous studies (1,31) and calculated the equivalent VDE (eVDE) using the following formula:
where denotes the MTD for patient i at the time of observation j; represents the eVDE and is the slope of the regression line of the LMEM; is the intercept for patient i; represents the time of observation j for patient i; is the initial MTD of patient i; is the fixed effect of ; and is the residual term.
To describe the differences in tumor growth rates based on the status of each factor, such as the high or low expression level of a biomarker, a multivariate LMEM model (mLMEM) with an interaction term was used as follows:
where , , , , , , and are as defined above; , and are fixed effect coefficients; represents patient i in the kth status of factor I; is the interaction term of time and factor; and its coefficient represents the effects of factor I on tumor growth rate. Thus, the regression lines of the LMEM for gliomas that differ in factor I status can be compared quantitatively, and the interaction effects on tumor growth rate can be identified.
Statistical analyses
We included gender, age, and histological classification as clinical factors. The interaction effects of the clinical factors and time were analyzed using mLMEM. Age was used as a continuous variable, while gender, histological classification, and molecular expression status were included as categorical variables in the analysis model. We also calculated the effects of different factor statuses on the tumor growth rate (using mLMEM). The Akaike information criterion, Bayesian information criterion (BIC), R2 coefficient, R2-adjusted, and mean square error were used to evaluate the model. In addition, the tumor growth rate in all patients, or a single patient, and its 95% confidence interval (CI) were calculated using LMEM. A P value ≤0.05 was considered statistically significant. To avoid bias caused by missing data, we first evaluated the interaction effects of missing data to determine if the presence or absence of data for a biomarker created distribution inconsistencies between the subgroup with missing data and the subgroup with complete data. Only biomarkers with consistent distribution (for which the interaction effect was not significant) were incorporated in multivariable analysis.
Results
Patient demographics
The data from a total of 56 patients (39 males and 17 females) were used in this study. The median age at the time of tumor detection on the first MRI examination was 36 years (range: 21 to 62 years). The median interval between the first MRI and preoperative MRI examination was 472 days (range: 91 to 4,799 days). The median tumor volume at the first detection was 9.4 cm3 (range: 0.2 to 368.0 cm3), and that at the last examination before surgery was 19.8 cm3 (range: 1.9 to 404.1 cm3). The growth curve of each patient and the regression lines for all patients are shown in Figure 2.
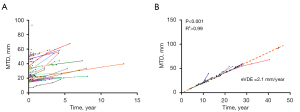
The VDE and clinical factors
For the 56 patients, the mean VDE was 3.3 mm/year, and the median VDE was 2.6 mm/year; the eVDE was 2.1 mm/year (95% CI: 1.8 to 2.4 mm/year, P<0.001, R2>0.99) (Figure 2). The influence of clinical characteristics on the tumor growth rate was assessed using mLMEMs (Table 1). Female patients were found to have a significantly higher eVDE than male patients (2.6 vs. 1.9 mm/year, P=0.02). Age was found to have a significant influence on eVDE (P<0.01). When the age at the first radiological diagnosis of LGGs increased by 1 year, the eVDE slowed by 0.05 mm/year. Among patients who could be clearly classified under the 2021 WHO classification, those with diffuse astrocytoma, IDH mutant-type exhibited significantly faster tumor growth than those with oligodendroglioma, IDH mutant-type (3.4 vs. 1.4 mm/year, P<0.01).
Table 1
Characteristic | N/value | eVDE (mm/year) | Estimated effects (mm/year ± SE) | P value |
---|---|---|---|---|
Gender | ||||
Male | 39 | 1.9 | 0.8±0.3 | 0.02* |
Female | 17 | 2.6 | ||
Age in years, median [range] | 36 [21–62] | −0.05±0.01 | <0.01* | |
Histological classification | ||||
Diffuse astrocytoma, IDH mutant-type | 21 | 3.4 | −2.1±0.3 | <0.01* |
Oligodendroglioma, IDH mutant-type | 19 | 1.4 | ||
Diffuse astrocytoma, NOS | 4 | 3.3 | – | – |
Oligodendroglioma, NOS | 4 | 1.7 | – | – |
Oligoastrocytoma, NOS | 8 | 2.8 | – | – |
Initial mean tumor diameter in cm, median (range) | 2.7 (0.8–9.0) | |||
Initial tumor volume in cm3, median (range) | 9.4 (0.2–368.0) | – | – | – |
Preoperative tumor volume in cm3, median (range) | 19.8 (1.9–404.1) | – | – | – |
Interval time between MRIs in days, median (range) | 472 (91–4,799) | – | – | – |
Number of available MRIs, average [range] | 2.6 [2–8] | – | – | – |
*, a P value ≤0.05 was considered statistically significant. eVDE, equivalent velocity of diameter expansion; IDH, isocitrate dehydrogenase; MRI, magnetic resonance imaging; SE, standard error.
Effects of biomarker status on eVDE
The influence of the molecular biomarkers on the eVDE was assessed using mLMEMs (Table 2). High expression level of p53 were found to be significantly associated with a high eVDE (high expression, 4.5 mm/year vs. low expression, 1.5 mm/year; P<0.01), as were high expression level of EGFR (high expression, 4.0 mm/year vs. low expression, 2.2 mm/year; P<0.01).
Table 2
Molecular biomarker | Subgroup | N | eVDE (mm/year) | Estimated effects (mm/year ± SE) | P value |
---|---|---|---|---|---|
Ki-67 expression | Low | 18 | 2.6 | −0.7±0.4 | 0.05* |
High | 18 | 1.9 | |||
N/A | 20 | 1.9 | |||
Mutant p53 expression | Low | 22 | 1.5 | 3.0±0.4 | <0.01* |
High | 8 | 4.5 | |||
N/A | 26 | 2.0 | |||
TERT promoter type | Wild-type | 25 | 3.4 | −1.8±0.3 | <0.01* |
Mutant | 20 | 1.6 | |||
N/A | 11 | 1.5 | |||
1p/19q codeletion status | Non-codeletion | 21 | 3.4 | −1.9±0.3 | <0.01* |
Codeletion | 19 | 1.4 | |||
N/A | 16 | 2.2 | |||
EGFR expression | Low | 7 | 2.2 | 1.8±0.6 | <0.01* |
High | 9 | 4.0 | |||
N/A | 40 | 1.8 | |||
ATRX expression | Low | 9 | 3.6 | −2.5±0.4 | <0.01* |
High | 16 | 1.1 | |||
N/A | 31 | 1.9 | |||
MGMT promoter methylation status | Non-methylation | 8 | 5.0 | −3.0±0.6 | <0.01* |
Methylation | 31 | 2.0 | |||
N/A | 17 | 1.7 |
*, a P value ≤0.05 was considered statistically significant. N/A, not available/data missing; eVDE, equivalent velocity of diameter expansion; SE, standard error.
A low eVDE was found to be significantly associated with TERT promoter type (mutant, 1.6 mm/year vs. wild-type, 3.4 mm/year; P<0.01), the 1p/19q codeletion status (codeletion, 1.4 mm/year vs. non-codeletion 3.4 mm/year; P<0.01), the MGMT promoter methylation status (methylation, 2.0 mm/year vs. non-methylation, 5.0 mm/year; P<0.01), high expression level of ATRX (high expression, 1.1 mm/year vs. low expression, 3.6 mm/year; P<0.01), and high expression level of Ki-67 (high expression, 1.9 mm/year vs. low expression, 2.6 mm/year; P=0.05).
Multiple-factor analysis using mLMEM
The interaction effects of missing data for different biomarkers are shown in Table S2. The presence or absence of EGFR and TERT promoter data caused significant distribution inconsistencies, and both the group with missing data and the group with complete data were excluded from the model. Histological classifications determined by biomarkers were also excluded. We used Ki-67, mutant p53, 1p/19q, ATRX, MGMT promoter, age, and gender as interaction terms in the mLMEM to assess whether they were independent influencing factors. In the multiple-factor analysis, age (P<0.01), expression levels of mutant p53 (P<0.01) and ATRX (P<0.01), and MGMT promoter methylation (P<0.01) had a significant impact on the eVDE (Table 3, Figure 3).
Table 3
Factor | Estimated effects (mm/year ± SE) | P value |
---|---|---|
Age | −0.03±0.01 | <0.01* |
Gender (female vs. male) | 0.7±0.3 | 0.51 |
MGMT promoter (methylation vs. non-methylation) | −3.1±0.4 | <0.01* |
Mutant p53 (high vs. low expression) | 1.9±0.3 | <0.01* |
1p/19q (codeletion vs. non-codeletion) | −0.5±0.2 | 0.10 |
Ki-67 (high vs. low expression) | 0.4±0.2 | 0.07 |
ATRX (high vs. low expression) | −1.3±0.4 | <0.01* |
*, a P value of ≤0.05 was considered statistically significant. mLMEM, multivariate linear mixed effects model; SE, standard error.
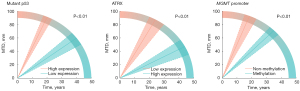
The estimated effect of factors on the diffuse astrocytoma, IDH mutant-type and oligodendroglioma, IDH mutant-type subgroups were also analyzed by multiple-factor analysis (Table 4). Age, expression levels of mutant p53, and MGMT promoter methylation were independent factors that influenced tumor growth rates in the diffuse astrocytoma, IDH mutant-type subgroup, while age and gender were independent factors that influenced tumor growth rates in the oligodendroglioma, IDH mutant-type subgroup.
Table 4
Molecular biomarker | Estimated effects (mm/year ± SE) | P value |
---|---|---|
Diffuse astrocytoma, IDH mutant-type | ||
Age | −0.09±0.03 | 0.01* |
Mutant p53 (high vs. low expression) | 3.6±1.7 | 0.04* |
MGMT promoter (methylation vs. non-methylation) | −2.6±0.5 | <0.01* |
Oligodendroglioma, IDH mutant-type | ||
Age | −0.02±0.006 | <0.01* |
Gender (female vs. male) | 0.7±0.2 | <0.01* |
*, a P value ≤0.05 was considered statistically significant. mLMEM, multivariate linear mixed-effects model; SE, standard error.
Discussion
Tumor growth rate is considered to directly reflect the characteristic natural malignancy of a tumor and its invasiveness in the absence of the influence of clinical treatment. The genetic background of gliomas has been hypothesized to influence the tumor growth rate (10,14). The current study quantitatively investigated whether the status of tumor-related biomarkers is associated with the tumor growth rate and found that the natural growth of LGGs could be influenced by the expression levels, codeletion, methylation and mutation status of specific tumor biomarkers.
It remains unclear whether a linear growth model or a non-linear growth model is better for evaluating tumor growth. The non-linear growth model is usually based on tumor volumes, whereas the linear growth model is usually based on equivalent tumor diameters (1,2,7,10,23,24,32,33). Most studies quantitatively measuring tumor growth rates have used a linear growth model based on the MTD (1,8-10,12-14,23,24). Although the eVDE in the first study (1) was 4.1 mm/year, calculated using a LMEM, in subsequent studies, the tumor growth rates, which were largely based on the mean or median VDE, were 3.5 to 5.9 mm/year (8,9,12-14,23,24). The growth rate in the pre-surgery linear growth model is only influenced by biological tumor properties. The linear growth model helps clarify whether there are any differences in tumor growth trends among groups with different molecular biomarker statuses, so this model was preferred for assessing tumor growth patterns in the present study.
The regression line of the LMEM indicates the overall trend of the growth of LGGs, and its slope represents the eVDE. In several previous studies (9,10,12-14,24,34), linear regression was used to calculate the growth rate of a tumor in a single patient, while the tumor growth rates in a group of patients were described using the mean or median growth rate. In addition, although the t-test and Wilcoxon test have been used to compare growth rates between groups of gliomas, these methods cannot fully describe the trend of the overall growth for a group of patients, with results easily affected by outliers. An LMEM can be used to avoid the disadvantages mentioned above and exclude the influence of the initial tumor volume; the regression line is a better representation of the overall trend in tumor growth for all patients.
The association between various clinical characteristics and tumor growth rates has been investigated in several previous studies (7-10,24). None of these analyses found an association between the tumor growth rate and age, contrary to our results which showed that age had a significant impact on the tumor growth rate. Our results indicated that for LGGs, the later the tumor presents, the more slowly it grows, a phenomenon that has been reported for other tumors (35) but needs to be tested in larger data studies. In previous studies, the histological classification of LGGs was not associated with VDE (8,9), which was contrary to our findings, perhaps because the previous studies were conducted before 2021, and their classification standards did not follow the 2021 version of the WHO classification system (4). The growth rate results were consistent with LGG survival for different histological classifications (36). Diffuse astrocytoma, IDH mutant-type, grew fastest and was reported to have a shorter survival time, while oligodendroglioma, IDH mutant-type, grew the most slowly and was reported to have a longer survival time.
The qualitative influence of molecular biomarkers on tumor growth has previously been investigated (18-21). It is well known that p53 plays an important role in mediating cell proliferation (18,37). A previous study reported that overexpression of mutant p53 led to a significantly faster growth rate than did expression of wild-type p53 (7.7 vs. 4.5 mm/year, respectively; P=0.004) (8), consistent with our results. In the present study, expression of ATRX was reported to have a significant impact on tumor growth rate in patients with LGGs, and a low expression of ATRX was significantly associated with a higher eVDE. Although mutation or loss of ATRX are associated with a better prognosis in patients with diffuse astrocytoma, ATRX mutation or loss are always accompanied by the alternative lengthening of telomeres (ALT) phenotype, which immortalizes the cell (38) and might influence tumor growth. Although MGMT promoter methylation status is a well-known indicator associated with drug resistance to alkylating agents (39), the current study indicated that it also had a significant impact on tumor growth, with MGMT promoter methylation significantly decreasing the eVDE. Further study is needed to reveal any possible role of MGMT promoter methylation in the natural growth of tumors. Previous studies have shown that 1p/19q codeletion significantly decelerates tumor growth rate in LGGs (9,10,14), consistent with the results obtained in our univariate analysis. However, in our multiple-factor analysis, the effect of 1p/19q codeletion was not significant. Therefore, investigations with larger sample sizes are needed in the future to evaluate the individual effects of the 1p/19q codeletion.
Although most important molecular biomarkers for LGGs were analyzed in this study, our limited time span meant that only a few patients with complete data were available for analysis. Furthermore, limited by the retrospective nature of this study, we did not have records of the patients’ CDKN2A/B status, which is an important indicator for diffuse astrocytoma, IDH mutant-type.
According to a previous study (15), fluid-attenuated inversion recovery (FLAIR) imaging shows clearer tumor boundaries, helping to mark tumor regions more accurately. Due to the nature of retrospective studies, a limitation of the current study was that not all patients underwent FLAIR imaging, although they did undergo T2-weighted imaging. To avoid bias from sequence differences, we did not include FLAIR sequence images in the current analysis. 3D FLAIR imaging can increase the resolution of MRI and tumor lesions. We will consider using 3D FLAIR imaging in future studies to increase the robustness of our results. We hope that in future investigations, we can integrate genomics, radiomics, and clinical information to establish the natural growth model of gliomas and provide a clearer understanding of the influences of genetic characteristics on tumor growth.
In conclusion, mutant p53 and ATRX expression levels and MGMT promoter methylation were found to be independent factors associated with tumor growth rate in LGGs. High expression level of mutant p53 were found to be significantly associated with a higher tumor growth rate. High expression level of ATRX and MGMT promoter methylation were found to be significantly associated with a lower tumor growth rate. Our study used a statistical model to quantitatively identify the effect of molecular biomarkers on the tumor growth rate in LGGs.
Acknowledgments
Funding: This study was supported by National Natural Science Foundation (82072786), Beijing Natural Science Foundation (7174295) and Beijing Hospitals Authority Youth Programme (QML 20170501).
Footnote
Reporting Checklist: The authors have completed the REMARK reporting checklist. Available at https://atm.amegroups.com/article/view/10.21037/atm-21-3998/rc
Data Sharing Statement: Available at https://atm.amegroups.com/article/view/10.21037/atm-21-3998/dss
Peer Review File: Available at https://atm.amegroups.com/article/view/10.21037/atm-21-3998/prf
Conflicts of Interest: All authors have completed the ICMJE uniform disclosure form (available at https://atm.amegroups.com/article/view/10.21037/atm-21-3998/coif). The authors have no conflicts of interest to declare.
Ethical Statement: The authors are accountable for all aspects of the work in ensuring that questions related to the accuracy or integrity of any part of the work are appropriately investigated and resolved. The study was conducted in accordance with the Declaration of Helsinki (as revised in 2013). The study was approved by the Ethics Committee of Beijing Tiantan Hospital (No. KYSB2016-026) and individual consent for this retrospective analysis was waived.
Open Access Statement: This is an Open Access article distributed in accordance with the Creative Commons Attribution-NonCommercial-NoDerivs 4.0 International License (CC BY-NC-ND 4.0), which permits the non-commercial replication and distribution of the article with the strict proviso that no changes or edits are made and the original work is properly cited (including links to both the formal publication through the relevant DOI and the license). See: https://creativecommons.org/licenses/by-nc-nd/4.0/.
References
- Mandonnet E, Delattre JY, Tanguy ML, et al. Continuous growth of mean tumor diameter in a subset of grade II gliomas. Ann Neurol 2003;53:524-8. [Crossref] [PubMed]
- Mandonnet E, Pallud J, Clatz O, et al. Computational modeling of the WHO grade II glioma dynamics: principles and applications to management paradigm. Neurosurg Rev 2008;31:263-9. [Crossref] [PubMed]
- Cancer Genome Atlas Research Network. Comprehensive, Integrative Genomic Analysis of Diffuse Lower-Grade Gliomas. N Engl J Med 2015;372:2481-98. [Crossref] [PubMed]
- Louis DN, Perry A, Wesseling P, et al. The 2021 WHO Classification of Tumors of the Central Nervous System: a summary. Neuro Oncol 2021;23:1231-51. [Crossref] [PubMed]
- Jiang T, Mao Y, Ma W, et al. CGCG clinical practice guidelines for the management of adult diffuse gliomas. Cancer Lett 2016;375:263-73. [Crossref] [PubMed]
- Harpold HL, Alvord EC Jr, Swanson KR. The evolution of mathematical modeling of glioma proliferation and invasion. J Neuropathol Exp Neurol 2007;66:1-9. [Crossref] [PubMed]
- Pallud J, Mandonnet E, Duffau H, et al. Prognostic value of initial magnetic resonance imaging growth rates for World Health Organization grade II gliomas. Ann Neurol 2006;60:380-3. [Crossref] [PubMed]
- Pallud J, Blonski M, Mandonnet E, et al. Velocity of tumor spontaneous expansion predicts long-term outcomes for diffuse low-grade gliomas. Neuro Oncol 2013;15:595-606. [Crossref] [PubMed]
- Gozé C, Blonski M, Le Maistre G, et al. Imaging growth and isocitrate dehydrogenase 1 mutation are independent predictors for diffuse low-grade gliomas. Neuro Oncol 2014;16:1100-9. [Crossref] [PubMed]
- Ricard D, Kaloshi G, Amiel-Benouaich A, et al. Dynamic history of low-grade gliomas before and after temozolomide treatment. Ann Neurol 2007;61:484-90. [Crossref] [PubMed]
- Ribba B, Kaloshi G, Peyre M, et al. A tumor growth inhibition model for low-grade glioma treated with chemotherapy or radiotherapy. Clin Cancer Res 2012;18:5071-80. [Crossref] [PubMed]
- Blonski M, Pallud J, Gozé C, et al. Neoadjuvant chemotherapy may optimize the extent of resection of World Health Organization grade II gliomas: a case series of 17 patients. J Neurooncol 2013;113:267-75. [Crossref] [PubMed]
- Pallud J, Llitjos JF, Dhermain F, et al. Dynamic imaging response following radiation therapy predicts long-term outcomes for diffuse low-grade gliomas. Neuro Oncol 2012;14:496-505. [Crossref] [PubMed]
- Gozé C, Bezzina C, Gozé E, et al. 1P19Q loss but not IDH1 mutations influences WHO grade II gliomas spontaneous growth. J Neurooncol 2012;108:69-75. [Crossref] [PubMed]
- Pallud J, Taillandier L, Capelle L, et al. Quantitative morphological magnetic resonance imaging follow-up of low-grade glioma: a plea for systematic measurement of growth rates. Neurosurgery 2012;71:729-39; discussion 739-40. [Crossref] [PubMed]
- Louis DN, Perry A, Reifenberger G, et al. The 2016 World Health Organization Classification of Tumors of the Central Nervous System: a summary. Acta Neuropathol 2016;131:803-20. [Crossref] [PubMed]
- Lu KV, Chang JP, Parachoniak CA, et al. VEGF inhibits tumor cell invasion and mesenchymal transition through a MET/VEGFR2 complex. Cancer Cell 2012;22:21-35. [Crossref] [PubMed]
- Michalovitz D, Halevy O, Oren M. Conditional inhibition of transformation and of cell proliferation by a temperature-sensitive mutant of p53. Cell 1990;62:671-80. [Crossref] [PubMed]
- Deryugina EI, Quigley JP. Matrix metalloproteinases and tumor metastasis. Cancer Metastasis Rev 2006;25:9-34. [Crossref] [PubMed]
- Jutten B, Rouschop KM. EGFR signaling and autophagy dependence for growth, survival, and therapy resistance. Cell Cycle 2014;13:42-51. [Crossref] [PubMed]
- Weathers SP, de Groot J. VEGF Manipulation in Glioblastoma. Oncology (Williston Park) 2015;29:720-7. [PubMed]
- Pallud J, Fontaine D, Duffau H, et al. Natural history of incidental World Health Organization grade II gliomas. Ann Neurol 2010;68:727-33. [Crossref] [PubMed]
- Peyre M, Cartalat-Carel S, Meyronet D, et al. Prolonged response without prolonged chemotherapy: a lesson from PCV chemotherapy in low-grade gliomas. Neuro Oncol 2010;12:1078-82. [Crossref] [PubMed]
- Mandonnet E, Pallud J, Fontaine D, et al. Inter- and intrapatients comparison of WHO grade II glioma kinetics before and after surgical resection. Neurosurg Rev 2010;33:91-6. [Crossref] [PubMed]
- Li Y, Liu X, Qian Z, et al. Genotype prediction of ATRX mutation in lower-grade gliomas using an MRI radiomics signature. Eur Radiol 2018;28:2960-8. [Crossref] [PubMed]
- Wang Y, Fan X, Zhang C, et al. Identifying radiographic specificity for phosphatase and tensin homolog and epidermal growth factor receptor changes: a quantitative analysis of glioblastomas. Neuroradiology 2014;56:1113-20. [Crossref] [PubMed]
- Zhang W, Lin Y, Chen B, et al. Recurrent glioblastoma of childhood treated with bevacizumab: case report and molecular features. Childs Nerv Syst 2010;26:137-43. [Crossref] [PubMed]
- Li S, Jiang T, Li G, et al. Impact of p53 status to response of temozolomide in low MGMT expression glioblastomas: preliminary results. Neurol Res 2008;30:567-70. [Crossref] [PubMed]
- Yang P, Cai J, Yan W, et al. Classification based on mutations of TERT promoter and IDH characterizes subtypes in grade II/III gliomas. Neuro Oncol 2016;18:1099-108. [Crossref] [PubMed]
- Zhang CB, Bao ZS, Wang HJ, et al. Correlation of IDH1/2 mutation with clinicopathologic factors and prognosis in anaplastic gliomas: a report of 203 patients from China. J Cancer Res Clin Oncol 2014;140:45-51. [Crossref] [PubMed]
- Bernal-Rusiel JL, Greve DN, Reuter M, et al. Statistical analysis of longitudinal neuroimage data with Linear Mixed Effects models. Neuroimage 2013;66:249-60. [Crossref] [PubMed]
- Hlaihel C, Guilloton L, Guyotat J, et al. Predictive value of multimodality MRI using conventional, perfusion, and spectroscopy MR in anaplastic transformation of low-grade oligodendrogliomas. J Neurooncol 2010;97:73-80. [Crossref] [PubMed]
- Pallud J, Varlet P, Devaux B, et al. Diffuse low-grade oligodendrogliomas extend beyond MRI-defined abnormalities. Neurology 2010;74:1724-31. [Crossref] [PubMed]
- Pallud J, Mandonnet E, Deroulers C, et al. Pregnancy increases the growth rates of World Health Organization grade II gliomas. Ann Neurol 2010;67:398-404. [PubMed]
- Huang MS, Kato H, Konaka C, et al. Quantitative cytochemical differences between young and old patients with lung cancer. Chest 1985;88:864-9. [Crossref] [PubMed]
- Jiang T, Nam DH, Ram Z, et al. Clinical practice guidelines for the management of adult diffuse gliomas. Cancer Lett 2021;499:60-72. [Crossref] [PubMed]
- Serrano M, Lin AW, McCurrach ME, et al. Oncogenic ras provokes premature cell senescence associated with accumulation of p53 and p16INK4a. Cell 1997;88:593-602. [Crossref] [PubMed]
- Li F, Deng Z, Zhang L, et al. ATRX loss induces telomere dysfunction and necessitates induction of alternative lengthening of telomeres during human cell immortalization. EMBO J 2019;38:e96659. [Crossref] [PubMed]
- van Thuijl HF, Mazor T, Johnson BE, et al. Evolution of DNA repair defects during malignant progression of low-grade gliomas after temozolomide treatment. Acta Neuropathol 2015;129:597-607. [Crossref] [PubMed]