Identification of autophagy-related genes as potential biomarkers for type 1 diabetes mellitus
Introduction
Type 1 diabetes mellitus (T1DM) is an abnormal nutrient metabolism disease caused by the autoimmune destruction of pancreatic islet β-cells, which often results in absolute insulin deficiency (1,2). The global incidence of T1DM increases by approximately 3% per year, but the incidence varies widely between regions, such that there is an annual incidence of 57.6 per 100,000 in Finland but only 0.1 per 100,000 in China (3-5). Additionally, T1DM tends to occur in younger individuals, with children and adolescents accounting for approximately 90% of all T1DM patients in Europe and the United States, and T1DM is the most common form of childhood diabetes in most parts of the world (4,6). As T1DM is incurable and requires lifelong insulin therapy to maintain blood glucose levels, and the metabolic disorders caused by T1DM have serious adverse effects on children, adolescents, and pregnant women of childbearing age, T1DM is an important disease that seriously threatens the health of young adults and children and adolescents (7,8).
Despite extensive research, the pathogenesis of T1DM has not been fully elucidated. T1DM is an autoimmune disease caused by T cells; programmed cell death protein 1 (PD-1) is expressed in activated T cells, and programmed death-ligand 1 (PD-L1) deficiency acts directly on pathogenic T cells and increases individuals’ susceptibility to T1DM, while regulatory T cells and B cells prevent T1DM independently of the PD-1/PD-L1 pathway (9). Additionally, natural killer (NK) cells, Th1 cells, and regulatory T cells have been found to play important roles in the development of T1DM (10,11). In recent years, the positive effects of autophagy on organisms’ health have been widely recognized, and various studies have established that there is a close relationship between chronic metabolic diseases and autophagy, and more specifically, that the dysregulation of autophagy may affect the normal function of the body, and thus lead to the development of chronic metabolic diseases (12-14). Autophagy in the pathogenesis of T1DM is mainly reflected in the induction of antioxidant responses in cells, the reduction of endoplasmic reticulum stress, and the prevention of islet β-cell apoptosis, but excessive autophagy can lead to islet β-cell damage and death (15-17). As an intracellular protein degradation pathway and an organismal defense mechanism, diabetes affects cellular autophagy through oxidative stress response, endoplasmic reticulum stress, and mammalian target of rapamycin (mTOR)-dependent signaling pathways (18-20). In addition, autophagy significantly controls the immune response by regulating the function of immune cells and cytokine production (21).
Currently, the diagnosis of T1DM remains clinical, and there is a lack of sensitive and effective biomarkers that can be used as early predictors of T1DM. The roles of genes related to T1DM pathogenesis, immune cells, and autophagy, and their relationships to T1DM have not yet been clarified. Thus, this study sought to uncover potential biomarkers related to autophagy in T1DM using a bioinformatics approach and to provide a theoretical basis for predicting T1DM and developing targeted therapeutic agents. We present the following article in accordance with the STREGA reporting checklist (available at https://atm.amegroups.com/article/view/10.21037/atm-22-1812/rc).
Methods
Raw data
We acquired the transcriptome data of GSE30211 (including 302 T1DM samples and 422 healthy samples) from the Gene Expression Omnibus database. The sequence data from the different platforms were merged and normalized for further analysis. The study was conducted in accordance with the Declaration of Helsinki (as revised in 2013).
Differentially expressed genes (DEGs) between the T1DM and healthy samples
Using Limma package in R software, a differential analysis of the T1DM and healthy samples was conducted to identify the DEGs. The heatmap and volcano map of the DEGs were generated using the ggplot2 package.
Functional enrichment analysis of the DEGs
We performed a Gene Ontology (GO) and Kyoto Encyclopedia of Genes and Genomes (KEGG) functional enrichment analysis using the clusterProfiler package in R, and the results were displayed using the ggplot2 package.
Construction of the protein-protein interaction (PPI) network
The PPI network of the DEGs was constructed using Search Tool for the Retrieval of Interacting Genes/Proteins (STRING; https://string-db.org/) and displayed using Cytoscape software. The cytoHubba plugin was used to calculate the top 10 proteins for the number of link nodes.
Acquisition of hub autophagy genes of T1DM
Autophagy-related genes were obtained from the Human Autophagy Database. The T1DM autophagy-related hub genes were obtained from the intersection of the DEGs. The top 10 PPI genes and the autophagy-related genes were displayed using the VennDiagram package.
Immune-infiltration analysis of T1DM
We conducted a single-sample gene set enrichment analysis (ssGSEA) to analyze the immune landscapes of the T1DM and healthy samples. We compared the infiltration of 28 immune cells in T1DM patients and healthy samples. Further, we also analyzed the relationship between the abundance of the 28 immune cells and the hub autophagy-related genes in T1DM.
Statistical analysis
All the statistical analyses were performed in R software. The difference analysis and correlation analysis were performed using the Wilcoxon and Spearman tests, respectively. A P value <0.05 was considered statistically significant.
Results
Principal component analysis (PCA) of raw transcriptome data before and after data normalization
The PCA indicated that the principal components of the gene expression levels of the 3 platforms were at the same level after standardization (Figure 1A); however, before normalization, the principal components of the gene expression levels differed significantly (Figure 1B).
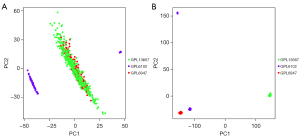
DEGs in T1DM
The T1DM-related DEGs showed that compared to the healthy samples, 301 genes were upregulated and 267 genes were downregulated in the T1DM samples (Figure 2).
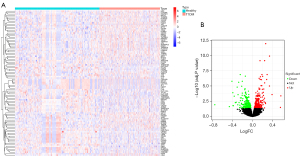
Functional enrichment analysis of the T1DM-related DEGs
The results of the GO functional enrichment analysis of the T1DM-related DEGs indicated that the top 5 biological processes in T1DM were blood coagulation, hemostasis, coagulation, the regulation of small GTPase-mediated signal transduction, and platelet activation, while the top 5 cell components were the cell leading edge, lamellipodium, cortical cytoskeleton, nuclear inner membrane, and tetraspanin-enriched microdomain, and the top 5 molecular functions were the transferase activity transferring alkyl or aryl (other than methyl) groups, glutathione transferase activity, glutathione binding, oligopeptide binding, and GDP-dissociation inhibitor activity (Figure 3A,3B).
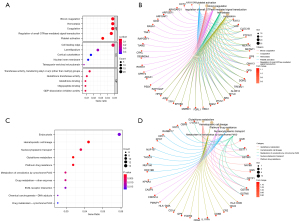
The results of the KEGG analysis indicated that the top 10 enrichment pathways were endocytosis, hematopoietic cell lineage, nucleocytoplasmic transport, glutathione metabolism, platinum drug resistance, the metabolism of xenobiotics by cytochrome P450, the drug metabolism of other enzymes, the extracellular matrix-receptor interaction, the chemical carcinogenesis of deoxyribonucleic acid adducts, and the drug metabolism of cytochrome P450 (Figure 3C,3D).
Top 10 ranked proteins of the PPI network
The PPI network of the DEGs indicated that the top 10 ranked proteins of the DEGs in the PPI network were RAB11A (a member of the RAS oncogene family), protein tyrosine phosphatase non-receptor type 11 (PTPN11), lamin A/C (LMNA), heat shock protein family A (HSP70), heat shock protein family A member 4 (HSPA4), cluster of differentiation 8A (CD8A), caspase 3 (CASP3), exportin 1 (XPO1), SRC proto-oncogene, non-receptor tyrosine kinase (SRC), SMAD family member 4 (SMAD4), and sirtuin 1 (SIRT1) (Figure 4).
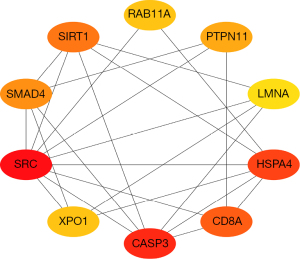
Acquisition and expression levels of the T1DM autophagy-related genes
The intersection of the DEGs, PPI network, and autophagy-related genes showed that T1DM had the following 3 autophagy-related genes: CASP3, RAB11A, and SIRT1 (Figure 5A). CASP3, RAB11A, and SIRT1 were more lowly expressed in the T1DM samples than the healthy samples (Figure 5B-5D).
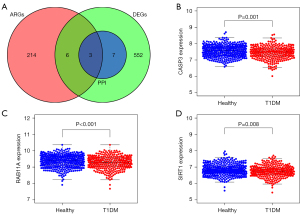
ssGSEA analysis of immune infiltration in the T1DM and healthy samples
Compared to the healthy samples, activated CD8 T cells, NK cells, activated dendritic cells, and monocytes were highly infiltrated in T1DM, while activated B cells, mature B cells, and Mast cells were lowly infiltrated in T1DM (Figure 6A). The correlation analysis showed that CASP3 and RAB11A were positively correlated with the abundance of most immune cell infiltrates, while SIRT1 was negatively correlated with the abundance of most immune cell infiltrates (Figure 6B).
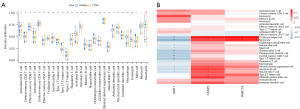
Discussion
Patients with diabetes mellitus often have immune abnormalities, such as reduced immune cells and reduced NK cell activity, and are at high risk of developing various infections (22). Diabetes is one of the most common comorbidities in patients and is a potential risk factor for the poor prognosis of patients with Middle-East respiratory syndrome, severe acute respiratory syndrome, coronavirus disease 2019, and cancer (23-26). Identifying and analyzing the signaling pathways associated with the development of T1DM will extend understandings of the pathogenesis of T1DM and provide a basis for the early diagnosis and treatment of T1DM and the prevention of concomitant diseases.
In this study, based on the GSE30211 data set, 422 healthy samples and 320 T1DM cases were analyzed, and 568 DEGs were obtained in both groups, of which 301 genes were upregulated and 267 genes were downregulated. The results of the functional and pathway enrichment analysis showed that the DEGs were closely associated with autophagy and immunity, which led us to speculate that these molecules are active in T1DM pathogenesis. RAB11A, PTPN11, LMNA, HSP70, HSPA4, CD8A, CASP3, XPO1, SRC, SMAD4, and SIRT1 were located at the center of the PPI network as core genes, and thus have the potential to be therapeutic targets for T1DM.
To screen for autophagy-related biomarkers in T1DM, we performed a cross-tab analysis and identified a total of 3 candidate autophagy-related biomarkers (i.e., RAB11A, CASP3, and SIRT1). RAB11A encodes a protein belonging to the Rab family of the small GTPase superfamily, which is associated with constitutive and regulatory secretory pathways and may be involved in protein transport (27). RAB11A plays an important role in the membrane receptor-mediated cytokinesis of macromolecules, and the high expression of RAB11A inhibits cellular autophagy and enhances the proliferation of pancreatic cancer cells (28,29). Recent research suggests that RAB11A may be an intra-nuclear module affecting T2DM (30). Multiple cell death modalities, such as apoptosis, autophagy, and necrosis, have been found to be associated with the pathogenesis of T1DM. The protein encoded by the CASP3 gene is a member of the cysteine-aspartate protease family, and the sequential activation of cysteine plays a central role in the execution phase of apoptosis (31). Tumor necrosis factor activates CASP3, which causes apoptosis in T1DM islet β-cells (32). Recent research has shown that CASP3 can predict the onset of diabetic events (33). However, the exact mechanism of CASP3 in TIDM is not yet clear. SIRT1, a NAD-dependent protein deacetylase involved in the amelioration of several metabolic diseases, increases insulin sensitivity in the liver, and is strongly associated with insulin resistance in different pathological states (34). SIRT1 regulates the activity of forkhead box O1 (FOXO1) through the regulation of the occurrence of autophagy (35). Additionally, SIRT1 is a potential target for protecting pancreatic β-cells from hypoxic damage during islet transplantation (36). The above report is consistent with our findings that RAB11A, CASP3, and SIRT1 are all lowly expressed in T1DM. Thus, these 3 genes represent promising biomarkers and therapeutic targets.
Additionally, we analyzed the differences in the levels of immune cells in T1DM and healthy subjects and found that activated CD8 T cells, NK cells, activated dendritic cells, and monocytes were higher in T1DM patients than normal subjects, and activated B cells, immature B cells, and mast cells were lower in T1DM patients than normal subjects, indicating that these immune cells play an important role in the development of T1DM. The maintenance of immune homeostasis is the result of an interaction between immune cells and regulatory immune cells. A study has reported that the proportion of both B cells and follicular helper T (Tfh) cells is significantly higher in T1DM patients than healthy patients, and the suppression of B cell function delays the onset of diabetes to some extent (37). Tfh cells play a key role in the process of helper humoral immunity by secreting interlukin-21, inducing the massive proliferation of B cells, and differentiating to form plasma cells (38). In relation to T cells, current studies have shown an increase in Th1 and Th17 cells and a decrease in regulatory T cell subpopulations during T1DM disease episodes (39,40). In the peripheral blood of children with T1DM, the percentage of intermediate T cells and memory T cells is increased, and both are positively correlated with and specific to T1DM (41). Our results showed that T cells were increased and B cells were decreased in patients with T1DM, which differed from the current report, suggesting that cellular immunity is activated, and humoral immunity is suppressed in T1DM patients, which in turn suggests that T1DM may be in a stable phase and may be an important cause of islet cell damage. Subsequently, we examined the correlations between RAB11A, CASP3, and SIRT1 and immune cells, and found that RAB11A and CASP3 were positively correlated with most of the immune cells, and SIRT1 was negatively correlated with most of the immune cells. Thus, SIRT1 may be associated with T1DM stabilization, while RAB11A and CASP3 may be associated with TIDM progression.
We systematically analyzed DEGs, autophagy-related genes, and immune cells and their relationships with T1DM. However, this study had a number of limitations, including that only a single data set was analyzed and that experimental validation is required. Further expansion of the data set and population and basic experiments need to be conducted to reveal the detailed mechanisms of autophagy-related genes in T1DM.
Conclusions
In this study, RAB11A, CASP3, and SIRT1 were identified as potential autophagy-related biomarkers and clinical therapeutic targets in T1DM. This study revealed for the first time the association between autophagy-associated genes and immune cells. However, further experiments still need to be conducted to explore the functions and mechanisms of autophagy-related genes in T1DM.
Acknowledgments
Funding: None.
Footnote
Reporting Checklist: The authors have completed the STREGA reporting checklist. Available at https://atm.amegroups.com/article/view/10.21037/atm-22-1812/rc
Conflicts of Interest: All authors have completed the ICMJE uniform disclosure form (available at https://atm.amegroups.com/article/view/10.21037/atm-22-1812/coif). The authors have no conflicts of interest to declare.
Ethical Statement: The authors are accountable for all aspects of the work in ensuring that questions related to the accuracy or integrity of any part of the work are appropriately investigated and resolved. The study was conducted in accordance with the Declaration of Helsinki (as revised in 2013).
Open Access Statement: This is an Open Access article distributed in accordance with the Creative Commons Attribution-NonCommercial-NoDerivs 4.0 International License (CC BY-NC-ND 4.0), which permits the non-commercial replication and distribution of the article with the strict proviso that no changes or edits are made and the original work is properly cited (including links to both the formal publication through the relevant DOI and the license). See: https://creativecommons.org/licenses/by-nc-nd/4.0/.
References
- Kurrer MO, Pakala SV, Hanson HL, et al. Beta cell apoptosis in T cell-mediated autoimmune diabetes. Proc Natl Acad Sci U S A 1997;94:213-8. [Crossref] [PubMed]
- Syed FZ. Type 1 Diabetes Mellitus. Ann Intern Med 2022;175:ITC33-48. [Crossref] [PubMed]
- Craig ME, Hattersley A, Donaghue KC. Definition, epidemiology and classification of diabetes in children and adolescents. Pediatr Diabetes 2009;10:3-12. [Crossref] [PubMed]
- Harjutsalo V, Sjöberg L, Tuomilehto J. Time trends in the incidence of type 1 diabetes in Finnish children: a cohort study. Lancet 2008;371:1777-82. [Crossref] [PubMed]
- Chan JC, Cho NH, Tajima N, et al. Diabetes in the Western Pacific Region--past, present and future. Diabetes Res Clin Pract 2014;103:244-55. [Crossref] [PubMed]
- Watanabe S, Kido J, Ogata M, et al. Hyperglycemic hyperosmolar state in an adolescent with type 1 diabetes mellitus. Endocrinol Diabetes Metab Case Rep 2019; Epub ahead of print. [Crossref] [PubMed]
- Rydén A, Sörstadius E, Bergenheim K, et al. The Humanistic Burden of Type 1 Diabetes Mellitus in Europe: Examining Health Outcomes and the Role of Complications. PLoS One 2016;11:e0164977. [Crossref] [PubMed]
- McGuire HC, Ji L, Kissimova-Skarbek K, et al. Type 1 diabetes mellitus care and education in China: The 3C study of coverage, cost, and care in Beijing and Shantou. Diabetes Res Clin Pract 2017;129:32-42. [Crossref] [PubMed]
- Dai S, Jia R, Zhang X, et al. The PD-1/PD-Ls pathway and autoimmune diseases. Cell Immunol 2014;290:72-9. [Crossref] [PubMed]
- Rodacki M, Svoren B, Butty V, et al. Altered natural killer cells in type 1 diabetic patients. Diabetes 2007;56:177-85. [Crossref] [PubMed]
- Mandrup-Poulsen T, Pickersgill L, Donath MY. Blockade of interleukin 1 in type 1 diabetes mellitus. Nat Rev Endocrinol 2010;6:158-66. [Crossref] [PubMed]
- Quan W, Hur KY, Lim Y, et al. Autophagy deficiency in beta cells leads to compromised unfolded protein response and progression from obesity to diabetes in mice. Diabetologia 2012;55:392-403. [Crossref] [PubMed]
- Mir SU, George NM, Zahoor L, et al. Inhibition of autophagic turnover in β-cells by fatty acids and glucose leads to apoptotic cell death. J Biol Chem 2015;290:6071-85. [Crossref] [PubMed]
- Ma S, Feng J, Zhang R, et al. SIRT1 Activation by Resveratrol Alleviates Cardiac Dysfunction via Mitochondrial Regulation in Diabetic Cardiomyopathy Mice. Oxid Med Cell Longev 2017;2017:4602715. [Crossref] [PubMed]
- Hayes HL, Peterson BS, Haldeman JM, et al. Delayed apoptosis allows islet β-cells to implement an autophagic mechanism to promote cell survival. PLoS One 2017;12:e0172567. [Crossref] [PubMed]
- Lambelet M, Terra LF, Fukaya M, et al. Dysfunctional autophagy following exposure to pro-inflammatory cytokines contributes to pancreatic β-cell apoptosis. Cell Death Dis 2018;9:96. [Crossref] [PubMed]
- Marasco MR, Linnemann AK. β-Cell Autophagy in Diabetes Pathogenesis. Endocrinology 2018;159:2127-41. [Crossref] [PubMed]
- Dehdashtian E, Mehrzadi S, Yousefi B, et al. Diabetic retinopathy pathogenesis and the ameliorating effects of melatonin; involvement of autophagy, inflammation and oxidative stress. Life Sci 2018;193:20-33. [Crossref] [PubMed]
- Song S, Tan J, Miao Y, et al. Crosstalk of autophagy and apoptosis: Involvement of the dual role of autophagy under ER stress. J Cell Physiol 2017;232:2977-84. [Crossref] [PubMed]
- Zhao Y, Qu T, Wang P, et al. Unravelling the relationship between macroautophagy and mitochondrial ROS in cancer therapy. Apoptosis 2016;21:517-31. [Crossref] [PubMed]
- Jiang GM, Tan Y, Wang H, et al. The relationship between autophagy and the immune system and its applications for tumor immunotherapy. Mol Cancer 2019;18:17. [Crossref] [PubMed]
- Carey IM, Critchley JA, DeWilde S, et al. Risk of Infection in Type 1 and Type 2 Diabetes Compared With the General Population: A Matched Cohort Study. Diabetes Care 2018;41:513-21. [Crossref] [PubMed]
- Garbati MA, Fagbo SF, Fang VJ, et al. A Comparative Study of Clinical Presentation and Risk Factors for Adverse Outcome in Patients Hospitalised with Acute Respiratory Disease Due to MERS Coronavirus or Other Causes. PLoS One 2016;11:e0165978. [Crossref] [PubMed]
- Yang JK, Feng Y, Yuan MY, et al. Plasma glucose levels and diabetes are independent predictors for mortality and morbidity in patients with SARS. Diabet Med 2006;23:623-8. [Crossref] [PubMed]
- Zhu L, She ZG, Cheng X, et al. Association of Blood Glucose Control and Outcomes in Patients with COVID-19 and Pre-existing Type 2 Diabetes. Cell Metab 2020;31:1068-1077.e3. [Crossref] [PubMed]
- Zhu B, Qu S. The Relationship Between Diabetes Mellitus and Cancers and Its Underlying Mechanisms. Front Endocrinol (Lausanne) 2022;13:800995. [Crossref] [PubMed]
- Bryant DM, Datta A, Rodríguez-Fraticelli AE, et al. A molecular network for de novo generation of the apical surface and lumen. Nat Cell Biol 2010;12:1035-45. [Crossref] [PubMed]
- Longatti A, Tooze SA. Recycling endosomes contribute to autophagosome formation. Autophagy 2012;8:1682-3. [Crossref] [PubMed]
- Yu L, Li X, Li H, et al. Rab11a sustains GSK3β/Wnt/β-catenin signaling to enhance cancer progression in pancreatic cancer. Tumour Biol 2016;37:13821-9. [Crossref] [PubMed]
- Boutchueng-Djidjou M, Belleau P, Bilodeau N, et al. A type 2 diabetes disease module with a high collective influence for Cdk2 and PTPLAD1 is localized in endosomes. PLoS One 2018;13:e0205180. [Crossref] [PubMed]
- Elmore S. Apoptosis: a review of programmed cell death. Toxicol Pathol 2007;35:495-516. [Crossref] [PubMed]
- Wong RS. Apoptosis in cancer: from pathogenesis to treatment. J Exp Clin Cancer Res 2011;30:87. [Crossref] [PubMed]
- Sun J, Singh P, Österlund J, et al. Hyperglycaemia-associated Caspase-3 predicts diabetes and coronary artery disease events. J Intern Med 2021;290:855-65. [Crossref] [PubMed]
- Sui M, Chen G, Mao X, et al. Gegen Qinlian Decoction Ameliorates Hepatic Insulin Resistance by Silent Information Regulator1 (SIRT1)-Dependent Deacetylation of Forkhead Box O1 (FOXO1). Med Sci Monit 2019;25:8544-53. [Crossref] [PubMed]
- Xu J, Huang XL. Research progress on SIRT1-FoxO-autophagy pathway. Chin Pharmacol Bull 2014;30:901-4.
- Lee YJ, Lee E, You YH, et al. Role of sirtuin-1 (SIRT1) in hypoxic injury in pancreatic β-cells. J Drug Target 2021;29:88-98. [Crossref] [PubMed]
- Botta D, Fuller MJ, Marquez-Lago TT, et al. Dynamic regulation of T follicular regulatory cell responses by interleukin 2 during influenza infection. Nat Immunol 2017;18:1249-60. [Crossref] [PubMed]
- Essig K, Hu D, Guimaraes JC, et al. Roquin Suppresses the PI3K-mTOR Signaling Pathway to Inhibit T Helper Cell Differentiation and Conversion of Treg to Tfr Cells. Immunity 2017;47:1067-1082.e12. [Crossref] [PubMed]
- Ferraro A, Socci C, Stabilini A, et al. Expansion of Th17 cells and functional defects in T regulatory cells are key features of the pancreatic lymph nodes in patients with type 1 diabetes. Diabetes 2011;60:2903-13. [Crossref] [PubMed]
- Honkanen J, Nieminen JK, Gao R, et al. IL-17 immunity in human type 1 diabetes. J Immunol 2010;185:1959-67. [Crossref] [PubMed]
- Teniente-Serra A, Pizarro E, Quirant-Sánchez B, et al. Identifying Changes in Peripheral Lymphocyte Subpopulations in Adult Onset Type 1 Diabetes. Front Immunol 2021;12:784110. [Crossref] [PubMed]
(English Language Editor: L. Huleatt)