Identification of metabolism-related long non-coding RNA (lncRNA) signature predicts prognosis and immune infiltrates in hepatocellular carcinoma
Introduction
Primary liver cancer is one of the 7 most common malignancies worldwide and is also a leading cause of cancer-related death (1). Hepatocellular carcinoma (HCC) is the most common type of primary liver cancer, accounting for approximately 80% of all cases (2). To date, multiple risk factors have been associated with the development and progression of HCC, including chronic infection with viruses, alcohol abuse, chronic obesity, or exposure to aflatoxins (2,3). Despite significant advances in clinical cancer treatment, the overall prognosis of patients with HCC remains poor due to high rates of postoperative tumor recurrence and metastasis (4). Previous study has shown that the progression of HCC is a complex process involving multiple factors and genes (5). The underlying molecular mechanisms of HCC development are unknown, and therefore, a better understanding of these is important for the prognosis and targeted therapy of HCC.
Cancer cannot develop without the metabolic reprogramming of cells, which is a direct and indirect consequence of oncogenic mutations (6,7). Cancer metabolic reprogramming can take many forms, such as the classic Warburg effect (8). Regardless of the mode of metabolic reprogramming, a common feature of cancer cells is the ability to obtain nutrients necessary for growth from a nutrient-deficient acidic environment and use these nutrients to maintain their growth transfer viability and build new material (9). The altered intra- and extra-cellular metabolites accompanying cancer-associated metabolic reprogramming are accompanied by corresponding changes in gene expression, which further affects cell differentiation and can even influence the tumor immune microenvironment (TIME) (10,11). The liver is the central metabolic organ that maintains blood glucose levels, ammonia metabolism, biotransformation of xenobiotics and endogenous metabolic by-products of metabolism, and bile synthesis under the action of multiple enzymes and pathways (12). Targeting cancer metabolic reprogramming therapy is expected to improve the risk of recurrence and metastasis in patients with HCC.
RNA-sequencing have revealed distinct long non-coding RNA (lncRNA) expression profiles in HCC tissues and several previously published papers have reported that lncRNAs affect metabolic reprogramming in HCC (13,14). Perturbation of the CCT3-LINC00326 regulatory network led to decreased lipid accumulation and inhibited HCC growth (15). LINC01234 was highly expressed in HCC and associated with poor prognosis. Mechanistically, LINC01234 downregulated the expression of Argininosuccinate synthase 1 (ASS1), upregulated aspartate level and activation of the mammalian target of rapamycin pathway (16). Nevertheless, a single metabolism-related lncRNA cannot reflect the metabolic panorama of tumor tissue. Therefore, we aimed to construct a metabolism-related lncRNA prognosis signature for HCC and clarify the molecular and immune characteristics in defined subgroups. We present the following article in accordance with the TRIPOD reporting checklist (available at https://atm.amegroups.com/article/view/10.21037/atm-22-2194/rc).
Methods
Data acquisition
After approval from the database of Genotypes and Phenotypes (dbGaP) data access committee, the raw RNA sequencing (RNA-Seq) datasets and clinical information for LIHC patients were downloaded and extracted from TCGA (https://portal.gdc.cancer.gov/) database. All available raw RNA-seq data in the database were downloaded and normalized by using R language (version 4.1.2; https://www.r-project.org/). Patients with a precise pathological diagnosis, gene expression, and clinical data were included in our study. In the end, we obtained 343 samples after excluding patients with no mutation information. The clinical data collected from 343 LIHC patients included age, gender, grade, pathologic stage, tumor-node-metastasis (TNM), TP53 mutational status, survival status, and survival time. Baseline characteristics of all included LIHC cases are summarized in Table 1. Then, TCGA-LIHC patients with complete clinical information were split into a training cohort (n=207) and a testing cohort (internal validation set; n=136) randomly. The study was conducted in accordance with the Declaration of Helsinki (as revised in 2013).
Table 1
Characteristics | Training (n=207) | Testing (n=136) | Total (n=343) | P value | FDR |
---|---|---|---|---|---|
Age (years) | |||||
Mean ± SD | 60.58±13.31 | 57.59±12.97 | 59.40±13.24 | ||
Median (min–max) | 64.00 (16.00–90.00) | 59.00 (18.00–85.00) | 61.00 (16.00–90.00) | ||
Gender, n (%) | 0.98 | 1 | |||
Male | 140 (40.82) | 93 (27.11) | 233 (67.93) | ||
Female | 67 (19.53) | 43 (12.54) | 110 (32.07) | ||
Grade, n (%) | 0.79 | 1 | |||
Unknown | 2 (0.58) | 3 (0.87) | 5 (1.46) | ||
G4 | 7 (2.04) | 5 (1.46) | 12 (3.50) | ||
G3 | 68 (19.83) | 44 (12.83) | 112 (32.65) | ||
G2 | 95 (27.70) | 66 (19.24) | 161 (46.94) | ||
G1 | 35 (10.20) | 18 (5.25) | 53 (15.45) | ||
Stage, n (%) | 0.39 | 1 | |||
Unknown | 13 (3.79) | 9 (2.62) | 22 (6.41) | ||
Stage IV | 3 (0.87) | 0 (0.0e+0) | 3 (0.87) | ||
Stage III | 44 (12.83) | 36 (10.50) | 80 (23.32) | ||
Stage II | 44 (12.83) | 33 (9.62) | 77 (22.45) | ||
Stage I | 103 (30.03) | 58 (16.91) | 161 (46.94) | ||
T, n (%) | 0.45 | 1 | |||
Unknown | 3 (0.87) | 0 (0.0e+0) | 3 (0.87) | ||
T4 | 8 (2.33) | 5 (1.46) | 13 (3.79) | ||
T3 | 44 (12.83) | 31 (9.04) | 75 (21.87) | ||
T2 | 46 (13.41) | 38 (11.08) | 84 (24.49) | ||
T1 | 106 (30.90) | 62 (18.08) | 168 (48.98) | ||
M, n (%) | 0.23 | 1 | |||
Unknown | 61 (17.78) | 34 (9.91) | 95 (27.70) | ||
M1 | 3 (0.87) | 0 (0.0e+0) | 3 (0.87) | ||
M0 | 143 (41.69) | 102 (29.74) | 245 (71.43) | ||
N, n (%) | 0.57 | 1 | |||
Unknown | 63 (18.37) | 38 (11.08) | 101 (29.45) | ||
N1 | 1 (0.29) | 2 (0.58) | 3 (0.87) | ||
N0 | 143 (41.69) | 96 (27.99) | 239 (69.68) |
lncRNA, long non-coding RNA; TCGA, The Cancer Genome Atlas; LIHC, liver hepatocellular carcinoma; FDR, false discovery rate.
Identification of hub metabolism-related lncRNAs
Metabolism-related lncRNAs were searched for through Pearson correlation analysis between messenger RNA (mRNA) and lncRNA expression matrices extracted from the enrolled TCGA-LIHC cohort and metabolism-related genes obtained from gene set enrichment analysis (GSEA). The screening criteria were correlation coefficient |R2|>0.4 and P value <0.001. Tumor and paired normal tissue samples were screened from the samples to which metabolism-related lncRNAs belonged. The “limma” R package was used to select differentially expressed metabolism-related lncRNAs. The screening threshold was false discovery rate (FDR) <0.05 and |log2FC| ≥1. Univariate Cox regression analysis was performed to identify the metabolism-related lncRNAs significantly correlated with the overall survival (OS) in TCGA-LIHC cohort (P<0.05) by using the “survival” R package. To obtain the hub genes, we set the threshold as hazard ratio (HR) >1.35 or HR <0.65 and P<0.01 in Cox regression analysis and finally obtained a total of 34 hub metabolism-related lncRNAs. These identified hub prognosis-related characteristic lncRNAs were used to construct a multigene signature.
Construction and validation of the metabolism-related lncRNAs prognostic signature
The least absolute shrinkage and selection operator (LASSO) Cox regression analysis and construction of metabolism-related lncRNAs prognostic signature were performed as previously described (17). All HCC cases in the training set were separated into two groups by the cutoff point. Survival curves of two groups were constructed by the Kaplan-Meier method. To evaluate the predictive accuracy of the prognostic metabolism-related lncRNA signature, time-dependent ROC curves were derived with the “survival ROC” R package and the corresponding area under curve (AUC) values calculated. Furthermore, the training set applied univariate and multivariate Cox regression analyses to determine the effect of metabolism-related lncRNAs prognostic signature and clinical parameters (including age, gender, grade, stage, and TP53 mutational status) on OS. Finally, we used the testing set as internal validation set to examine the reliability of the prognostic metabolism-related lncRNA signature.
Identification of the metabolism-related lncRNAs prognostic signature associated biological characteristics
To explore the associated biological characteristics and potential signaling pathways, all the hub prognosis-related characteristic lncRNAs were subjected to Gene Ontology (GO) and Kyoto Encyclopedia of Genes and Genomes (KEGG) analysis. The Cell-type Identification by Estimating Relative Subsets of RNA Transcripts (CIBERSORT) algorithm and single sample gene set enrichment analysis (ssGSEA) were performed as previously described (18). The “GSVA” R package was used to calculate the enrichment score of each sample. The “pRRophetic” R package was used to predict differences in drug sensitivity between high- and low-risk groups. Somatic mutation analysis between the 2 groups was performed using the “maftools” R package.
Evaluation of the clinical validity of the prognostic signature
We used age, gender, grade, stage, and risk score to construct a nomogram for the training set via the “rms” R package. Calibration curves were plotted to evaluate actual and predicted survival consistency. The “ggDCA” R package was used to plot decision curve analysis (DCA) curves to verify the accuracy of prognostic signature.
Statistical analysis
Wilcoxon and Kruskal-Wallis tests were used to compare continuous variables. Categorical variables were analyzed using the chi-square test. Mann-Whitney test was used to compare the ssGSEA scores of immune characteristics between the high- and low-risk groups. We adjusted P values with the Benjamini & Hochberg method. The OS between different groups was compared by Kaplan-Meier analysis with the log-rank test. Significant variables on univariate Cox regression analysis (P<0.05) were subjected to multivariate Cox regression analysis to identify independent predictors of OS. All P values were based on a 2-sided statistical test, and P<0.05 was considered statistically significant. All statistical analyses were conducted using the R software.
Results
Identification of differential metabolism-related lncRNAs and visualization of their biological functions
The acquisition of metabolism-related lncRNAs in the current study is displayed in the flowchart in Figure 1A. All differentially expressed genes and metabolism-related lncRNAs are presented in the form of volcano maps (Figure 1B,1C). In GO and KEGG analysis, characteristic lncRNAs were significantly associated with metabolic pathways, such as glycolytic pathways, certain amino acid metabolism, and so on (Figure 1D,1E).
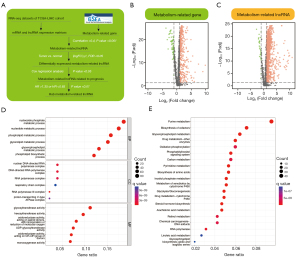
Construction of the metabolism-related lncRNAs prognostic signature model
Univariate Cox regression analysis was performed on enrolled HCC patients to explore the prognostic value of metabolism-related lncRNAs. A total of 34 lncRNAs were screened for prognosis-related lncRNAs based on the criterion of P<0.05. The forest map showing the risk ratios and corresponding confidence intervals for each prognosis-related lncRNAs showed that most of these genes were risk genes (Figure 2A). Next, these prognostic lncRNAs were included in further LASSO Cox regression analysis. The prognostic lncRNAs with the highest contribution to the OS of HCC were generated by performing LASSO Cox regression analysis in the training cohort. The corresponding regression coefficients were calculated. Figure 2B illustrates that the model performed best with 9 lncRNAs involved. Figure 2C indicates the spectrum of LASSO regression coefficients for the 9 lncRNAs.
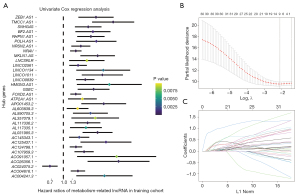
Evaluation of the prognostic model
Based on the HCC prognostic signature, we divided the cases into high- and low-risk groups. Kaplan-Meier survival curves suggested that HCC patients with higher risk scores had less favorable OS in the training, test, and entire cohorts, respectively (Figure 3A-3C, upper). The time-dependent ROC curve indicated that the prognostic signature model could accurately predict prognosis in 3 separate cohorts (training cohort 1-year AUC =0.811, 3-year AUC =0.773, 5-year AUC =0.753; test cohort 1-year AUC =0.757, 3-year AUC =0.72, 5-year AUC =0.671; entire cohort 1-year AUC =0.789, 3-year AUC =0.752, 5-year AUC =0.729; Figure 3A-3C, middle). AUC values higher than 0.5 indicate a better prognosis predictive ability. Survival status distributions and risk scores were plotted. In addition, the characteristic lncRNAs associated with the prognostic model in each cohort are presented in the form of heat maps (Figure 3A-3C, bottom).
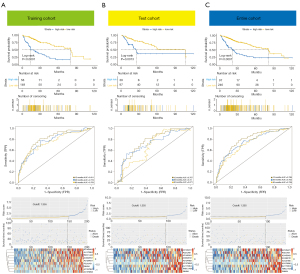
Description and evaluation of the metabolism-related lncRNAs as a crucial prognostic factor
Univariable and multivariable Cox Regression analysis revealed that metabolism-related lncRNAs signatures and stage are independent predictors of HCC survival (P<0.001; Figure 4A). Next, a nomogram containing the prognostic signature and traditional clinicopathological features was used to assess further the accuracy and clinical applicability of the prognostic (Figure 4B). The calibration plots indicated that the observed versus predicted rates of 1-, 2-, and 3-year OS had an excellent concordance in the HCC patients (Figure 4C). Similarly, the DCA curves showed the accuracy of the prognostic model (Figure 4D,4E). The above results indicated that the metabolism-related lncRNAs signature could be identified as a crucial prognostic factor for HCC patients.
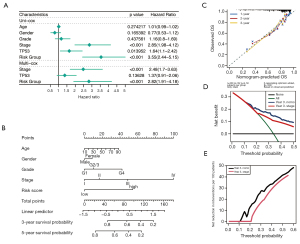
Stratified analysis of clinical parameters
Based on the optimal cut-off value of the training cohort calculated by “survminer” package, entire cohorts were classified into high- and low-risk groups. We aimed to ascertain whether the prognostic signature could be applied to different clinicopathologic subgroups (Figure 5). However, subgroup survival analyses found that whether age ≥60 or <60 years, gender male or female, tumour stage I/II or III/IV, and TP53 mutant or not, the patients in the high-risk group were significantly associated with a poor prognosis (all P<0.001).
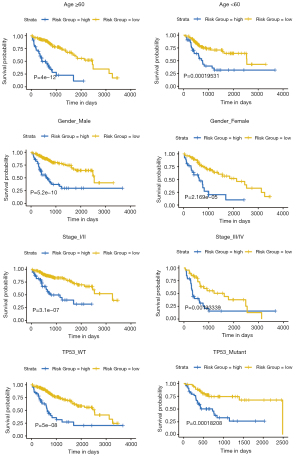
Correlation between metabolism-related lncRNAs and status of TIICs
The TIICs influence tumorigenesis by affecting the immune microenvironment. The TIICs influence tumorigenesis by affecting the immune microenvironment. We inferred the absolute level of immune infiltrate within each sample in the high- and low-risk groups using the CIBERSORT algorithm (Figure 6A). The ssGSEA showed the differences between the 28 TIICs in the high- and low-risk groups more clearly (Figure 6B). It could be seen that immune-activated cell infiltration was significantly reduced in the high-risk group, such as activated B cells, activated CD8+T cells, Th1 cells, and NK cells.
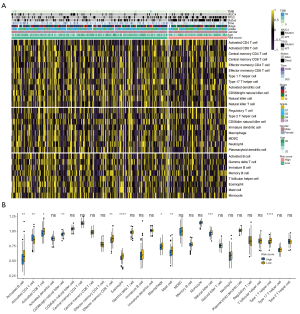
Molecular characteristics of patients in the high- and low-risk groups
We performed GSEA to determine the gene sets enriched between the high- and low-risk group. In the low-risk group, the enriched gene sets included BILE ACID METABOLISM and CHOLESTEROL HOMEOSTASIS (Figure 7A), where KRAS SIGNALING DN was enriched in the high-risk group (Figure 7B). We predict the drug sensitivity of cisplatin and sorafenib using the pRRophetic algorithm in the high- and low-risk groups, and the results suggested that the high-risk group was less sensitive to both drugs (Figure 7C).
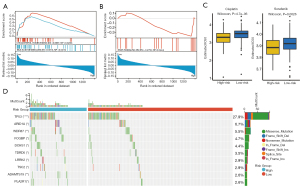
Next, gene variations were detected to more clearly elucidate these characteristic lncRNAs' biology. The top 10 mutated genes shared in the 2 groups were ranked by mutation rate (Figure 7D). We found that only TP53 mutation frequency exceeded 20% in both groups. The gene variations in both groups were predominantly deletion mutations.
Discussion
Abnormal cancer metabolism, such as increased glycolytic and glutamine metabolic pathways, has an important role in tumor development, metastasis, drug resistance, and cancer stem cells (19-21). Tumor neovascularization causes cancer cells to grow in an environment of hypoxia and nutrient deficiency (22). To adapt to these harsh environments, cancer cells constantly alter their metabolic pathways to obtain external metabolites and maximize the maintenance of high metabolic enzyme activity (6). Classical cancer-related signaling pathways, such as the PI3K/AKT and myc pathways, can mediate aberrant metabolic gene expression and increase key metabolic enzyme activities (23,24). Cancer-associated metabolism is based on a differential metabolic stress response between cancer and normal cells; targeting cancer-associated metabolism is expected to selectively inhibit cancer progression with less cytotoxicity to normal cells.
In this study, we explored the role of metabolism-related prognostic signature lncRNAs in HCC. A prognostic model containing 9 metabolism-related lncRNAs was first constructed and validated in the HCC training set. In addition, immunoassays using various bioinformatics tools showed significant differences in TIME and TIICs between high- and low-risk groups. Functional analysis revealed that the signature lncRNAs were associated with many cancer-related metabolic pathways. These findings strongly suggested a huge potential for cancer-related metabolic reprogramming in HCC.
A total of 9 hub metabolism-related lncRNAs (AC124798.1, TMCC1.AS1, AC145343.1, AC125437.1, SNHG4, AC004816.1, AL117336.2, POLH.AS1, and AC107959.3) were identified in our prognostic model. The lncRNA TMCC1-AS1 promotes epithelial mesenchymal transition for tumor metastasis in HCC (25). The lncRNA SNHG4 can promote cancer development as a competing endogenous RNA (ceRNA) (26,27). In addition, AC124798.1 (28), AC145343.1 (29), AC004816.1 (30), and AC107959.3 (31) were found to be strongly associated with poor prognosis of cancer by bioinformatics analysis. Although only a few lncRNAs have been experimentally reported as associated with cancer development, it is still possible to hypothesize that our prognostic model was negatively associated with prognosis of HCC based on the results of previous bioinformatic analysis and our results.
There is growing evidence that cancer-associated metabolic reprogramming not only plays a crucial role in cancer signaling, but also has broad implications in regulating antitumor immune responses through metabolite release and influencing the expression of immune molecules, such as lactate (32). Metabolic reprogramming is not unique to cancer cells; it is present in some immune cells, such as activated T cells, regulatory T cells (Tregs), and neutrophils (33) Like cancer cells, TIICs require certain nutrients from TME to maintain their own proliferation and differentiation. Previous study has shown that cancer cells limit glucose consumption by T cells through glycolysis, thereby inducing T cell failure and immune escape (34). In our study, immune infiltration analysis and enrichment analysis revealed a significant decrease in the percentage of immune activated cells in the high-risk group compared to the low-risk group. In Figure 1D and Figure 1E, we found that characteristic lncRNAs were significantly associated with glycolysis, oxidative phosphorylation, and amino acid metabolism. Glycolysis has been reported to be a major metabolic pathway for M1 macrophages, dendritic cells, naive T cells, and effector T cells (35). Tregs, M2 macrophages, and memory T cells rely mainly on OXPHOS, a source of fatty acid oxidation, for energy supply (36). Certain amino acids, such as glutamine, are essential for the maintenance and regulation of immune cell function (37). In addition, GSEA found that the high-risk group was significantly associated with the KRAS pathway. KRAS mutation aberrantly activates its downstream signaling pathways, including PI3K/AKT and MEK/ERK, thus contributing to the promotion and maintenance of cancer malignancy. Recently, KRAS has been reported to mediate immune escape in TME, which is consistent with our findings (38).
In addition, we explored the differences in drug sensitivity between high and low risk groups. Cisplatin and sorafenib are the most classical HCC drugs, and our results suggest that the high-risk group is less sensitive to both drugs. Both cisplatin and sorafenib were reported to promote immune activation to maintain the anticancer effect (39,40). This suggests that we can improve drug sensitivity to cisplatin and sorafenib by improving the immunosuppressive status of HCC patients. We are looking forward to see if it is the same with the new antitumoral drugs for HCC, such as lenvatinib, cabozantinib, or regorafenib, as well as the antiangiogenic antibody ramucirumab.
With the rapid development of bioinformatics technology, an array of models has been constructed to predict the prognosis of HCC based on lncRNA. However, in contrast to our study, these studies neglected the value of traditional clinical parameters. We combined clinical parameters with metabolism-related signature lncRNAs to predict the survival of HCC, which would provide great value to the clinic. In addition, we hypothesized a behind-the-scenes reason why signature lncRNAs affect prognosis of HCC-immunosuppression, which will provide new insights for future experimental design. However, our study still had some limitations. First, it was conducted retrospectively and therefore needs further validation in prospective studies. Second, HCC is highly heterogeneous and has different biological behaviors, the information from TCGA database was limited and incomplete. Third, to define the prognostic clinical outcome of patients with HCC there are different variables that have not been considered such has the histopathology of the tumor, level of alfa-fetoprotein and type of treatment (systemic or surgery as resection or transplantation). Fourth, more experiments are needed to further reveal the potential mechanisms by which the characteristic lncRNAs affect the prognosis of HCC.
In conclusion, our study identified 9 characteristic lncRNAs’ prognostic features and validated the accurate type and reliability of the model by ROC, DCA, and nomogram. Our prognostic model can help clinicians to provide personalized treatment strategies, and the 9 signature lncRNAs also provide some insights into new prognostic markers and potential therapeutic targets for HCC.
Acknowledgments
Funding: None.
Footnote
Reporting Checklist: The authors have completed the TRIPOD reporting checklist. Available at https://atm.amegroups.com/article/view/10.21037/atm-22-2194/rc
Conflicts of Interest: All authors have completed the ICMJE uniform disclosure form (available at https://atm.amegroups.com/article/view/10.21037/atm-22-2194/coif). The authors have no conflicts of interest to declare.
Ethical Statement: The authors are accountable for all aspects of the work in ensuring that questions related to the accuracy or integrity of any part of the work are appropriately investigated and resolved. The study was conducted in accordance with the Declaration of Helsinki (as revised in 2013).
Open Access Statement: This is an Open Access article distributed in accordance with the Creative Commons Attribution-NonCommercial-NoDerivs 4.0 International License (CC BY-NC-ND 4.0), which permits the non-commercial replication and distribution of the article with the strict proviso that no changes or edits are made and the original work is properly cited (including links to both the formal publication through the relevant DOI and the license). See: https://creativecommons.org/licenses/by-nc-nd/4.0/.
References
- Bray F, Ferlay J, Soerjomataram I, et al. Global cancer statistics 2018: GLOBOCAN estimates of incidence and mortality worldwide for 36 cancers in 185 countries. CA Cancer J Clin 2018;68:394-424. [Crossref] [PubMed]
- Kulik L, El-Serag HB. Epidemiology and Management of Hepatocellular Carcinoma. Gastroenterology 2019;156:477-91.e1. [Crossref] [PubMed]
- Ioannou GN. Epidemiology and risk-stratification of NAFLD-associated HCC. J Hepatol 2021;75:1476-84. [Crossref] [PubMed]
- Serper M, Taddei TH, Mehta R, et al. Association of Provider Specialty and Multidisciplinary Care With Hepatocellular Carcinoma Treatment and Mortality. Gastroenterology 2017;152:1954-64. [Crossref] [PubMed]
- Cancer Genome Atlas Research Network. Electronic address: wheeler@bcm.edu; Cancer Genome Atlas Research Network. Comprehensive and Integrative Genomic Characterization of Hepatocellular Carcinoma. Cell 2017;169:1327-1341.e23. [Crossref]
- Pavlova NN, Thompson CB. The Emerging Hallmarks of Cancer Metabolism. Cell Metab 2016;23:27-47. [Crossref] [PubMed]
- Kroemer G, Pouyssegur J. Tumor cell metabolism: cancer's Achilles' heel. Cancer Cell 2008;13:472-82. [Crossref] [PubMed]
- Koppenol WH, Bounds PL, Dang CV. Otto Warburg's contributions to current concepts of cancer metabolism. Nat Rev Cancer 2011;11:325-37. [Crossref] [PubMed]
- Miranda-Galvis M, Teng Y. Targeting Hypoxia-Driven Metabolic Reprogramming to Constrain Tumor Progression and Metastasis. Int J Mol Sci 2020;21:5487. [Crossref] [PubMed]
- Fan C, Zhang S, Gong Z, et al. Emerging role of metabolic reprogramming in tumor immune evasion and immunotherapy. Sci China Life Sci 2021;64:534-47. [Crossref] [PubMed]
- Kouidhi S, Ben Ayed F, Benammar Elgaaied A. Targeting Tumor Metabolism: A New Challenge to Improve Immunotherapy. Front Immunol 2018;9:353. [Crossref] [PubMed]
- Kietzmann T. Metabolic zonation of the liver: The oxygen gradient revisited. Redox Biol 2017;11:622-30. [Crossref] [PubMed]
- Lim LJ, Wong SYS, Huang F, et al. Roles and Regulation of Long Noncoding RNAs in Hepatocellular Carcinoma. Cancer Res 2019;79:5131-9. [Crossref] [PubMed]
- Malakar P, Stein I, Saragovi A, et al. Long Noncoding RNA MALAT1 Regulates Cancer Glucose Metabolism by Enhancing mTOR-Mediated Translation of TCF7L2. Cancer Res 2019;79:2480-93. [Crossref] [PubMed]
- Søndergaard JN, Sommerauer C, Atanasoai I, et al. CCT3-LINC00326 axis regulates hepatocarcinogenic lipid metabolism. Gut 2022; Epub ahead of print. [Crossref] [PubMed]
- Chen M, Zhang C, Liu W, et al. Long noncoding RNA LINC01234 promotes hepatocellular carcinoma progression through orchestrating aspartate metabolic reprogramming. Mol Ther 2022; Epub ahead of print. [Crossref] [PubMed]
- Dai X, Jiang W, Ma L, et al. A metabolism-related gene signature for predicting the prognosis and therapeutic responses in patients with hepatocellular carcinoma. Ann Transl Med 2021;9:500. [Crossref] [PubMed]
- Yao N, Zuo L, Yan X, et al. Systematic analysis of ferroptosis-related long non-coding RNA predicting prognosis in patients with lung squamous cell carcinoma. Transl Lung Cancer Res 2022;11:632-46. [Crossref] [PubMed]
- Zaal EA, Berkers CR. The Influence of Metabolism on Drug Response in Cancer. Front Oncol 2018;8:500. [Crossref] [PubMed]
- Elia I, Doglioni G, Fendt SM. Metabolic Hallmarks of Metastasis Formation. Trends Cell Biol 2018;28:673-84. [Crossref] [PubMed]
- Meacham CE, Morrison SJ. Tumour heterogeneity and cancer cell plasticity. Nature 2013;501:328-37. [Crossref] [PubMed]
- Hanahan D, Weinberg RA. Hallmarks of cancer: the next generation. Cell 2011;144:646-74. [Crossref] [PubMed]
- Hoxhaj G, Manning BD. The PI3K-AKT network at the interface of oncogenic signalling and cancer metabolism. Nat Rev Cancer 2020;20:74-88. [Crossref] [PubMed]
- Osthus RC, Shim H, Kim S, et al. Deregulation of glucose transporter 1 and glycolytic gene expression by c-Myc. J Biol Chem 2000;275:21797-800. [Crossref] [PubMed]
- Chen C, Su N, Li G, et al. Long non-coding RNA TMCC1-AS1 predicts poor prognosis and accelerates epithelial-mesenchymal transition in liver cancer. Oncol Lett 2021;22:773. [Crossref] [PubMed]
- Wang S, Zhu W, Qiu J, et al. lncRNA SNHG4 promotes cell proliferation, migration, invasion and the epithelial-mesenchymal transition process via sponging miR-204-5p in gastric cancer. Mol Med Rep 2021;23:85. [Crossref] [PubMed]
- Xu R, Feng F, Yu X, et al. LncRNA SNHG4 promotes tumour growth by sponging miR-224-3p and predicts poor survival and recurrence in human osteosarcoma. Cell Prolif 2018;51:e12515. [Crossref] [PubMed]
- Li Z, Qian Z, Chen F, et al. Identification of Key lncRNA-mRNA Pairs and Functional lncRNAs in Breast Cancer by Integrative Analysis of TCGA Data. Front Genet 2021;12:709514. [Crossref] [PubMed]
- Huang DP, Liao MM, Tong JJ, et al. Construction of a genome instability-derived lncRNA-based risk scoring system for the prognosis of hepatocellular carcinoma. Aging (Albany NY) 2021;13:24621-39. [Crossref] [PubMed]
- Wang Y, Wang Y, Di F, et al. Bioinformatic analysis of immune-related lncRNA based on TCGA database in patients with prostate cancer. Xi Bao Yu Fen Zi Mian Yi Xue Za Zhi 2021;37:113-8. [PubMed]
- Huang ZL, Li W, Chen QF, et al. Eight key long non-coding RNAs predict hepatitis virus positive hepatocellular carcinoma as prognostic targets. World J Gastrointest Oncol 2019;11:983-97. [Crossref] [PubMed]
- Hurley HJ, Dewald H, Rothkopf ZS, et al. Frontline Science: AMPK regulates metabolic reprogramming necessary for interferon production in human plasmacytoid dendritic cells. J Leukoc Biol 2021;109:299-308. [Crossref] [PubMed]
- Ghesquière B, Wong BW, Kuchnio A, et al. Metabolism of stromal and immune cells in health and disease. Nature 2014;511:167-76. [Crossref] [PubMed]
- Sukumar M, Roychoudhuri R, Restifo NP. Nutrient Competition: A New Axis of Tumor Immunosuppression. Cell 2015;162:1206-8. [Crossref] [PubMed]
- Pearce EL, Poffenberger MC, Chang CH, et al. Fueling immunity: insights into metabolism and lymphocyte function. Science 2013;342:1242454. [Crossref] [PubMed]
- Gardiner CM. NK cell metabolism. J Leukoc Biol 2019;105:1235-42. [Crossref] [PubMed]
- Coëffier M, Miralles-Barrachina O, Le Pessot F, et al. Influence of glutamine on cytokine production by human gut in vitro. Cytokine 2001;13:148-54. [Crossref] [PubMed]
- Hamarsheh S, Groß O, Brummer T, et al. Immune modulatory effects of oncogenic KRAS in cancer. Nat Commun 2020;11:5439. [Crossref] [PubMed]
- Hage C, Hoves S, Strauss L, et al. Sorafenib Induces Pyroptosis in Macrophages and Triggers Natural Killer Cell-Mediated Cytotoxicity Against Hepatocellular Carcinoma. Hepatology 2019;70:1280-97. [Crossref] [PubMed]
- Dasari S, Tchounwou PB. Cisplatin in cancer therapy: molecular mechanisms of action. Eur J Pharmacol 2014;740:364-78. [Crossref] [PubMed]
(English Language Editor: J. Jones)